-
PDF
- Split View
-
Views
-
Cite
Cite
Meghan E McGrady, Lisa A Prosser, Aimee N Thompson, Ahna L H Pai, Application of a Discrete Choice Experiment to Assess Adherence-Related Motivation Among Adolescents and Young Adults With Cancer, Journal of Pediatric Psychology, Volume 43, Issue 2, March 2018, Pages 172–184, https://doi.org/10.1093/jpepsy/jsx104
- Share Icon Share
Abstract
To develop and pilot test a discrete choice experiment (DCE) to assess the specific factors likely to motivate a given adolescent or young adult with cancer to adhere to medications included in treatment protocols.
A multimethod and iterative approach was used. Twenty adolescents and young adults with cancer participated in DCE pilot testing and refinement. Hierarchical Bayes was used to estimate attribute and attribute-level preference scores for each participant.
The final DCE included nine choice sets composed of four attributes. The resulting DCE was modified with feedback from 20 adolescents and young adults (M[SD] age = 18.66 [2.95]) with cancer. The DCE was understandable and relevant. The factors likely to motivate adolescents and young adults with cancer to be adherent differed across individuals.
DCEs including the measure developed in this manuscript offer a novel approach to understanding individual-level differences critical for informing adherence-promotion efforts.
Nonadherence to the oral chemotherapy and/or prophylactic (i.e., antibiotic; antifungal) medications included in cancer treatment protocols is demonstrated by 27–60% of adolescents and young adults (ages 15–24 years) with cancer (Butow et al., 2010) and is associated with treatment failure (i.e., relapse; Bhatia et al., 2015) and death (Kennard et al., 2004). Understanding and improving medication adherence, thus, have the potential to significantly improve health outcomes for thousands of the 11,000 adolescents and young adults diagnosed with cancer in the United States each year (Bleyer, O'Leary, Barr, & Ries, 2006). The only published clinical trial of an adherence-promotion intervention for adolescents and young adults with cancer represents a critical first step in this line of research but demonstrates limited effectiveness (d = 0.19), with participants still missing 37% of medication doses postintervention (Kato, Cole, Bradlyn, & Pollock, 2008). Improving on these efforts requires a comprehensive understanding of the modifiable factors that may lead adolescents and young adults with cancer to be nonadherent.
An extensive line of theoretically based research among adults with chronic medical conditions has found that the daily adherence decision requires a combination of information, social support, behavioral skills, and personal motivation (Fisher, Fisher, Amico, & Harman, 2006). Deficits in information, social support, and behavioral skills also play a role in adherence to cancer treatment regimens for adolescents and young adults with cancer (Butow et al., 2010). Personal motivation, however, has been largely neglected. Personal motivation is a promising target for investigation, as it was the most commonly cited reason for nonadherence in a study of adults with breast cancer (Guth, Myrick, Kilic, Eppenberger-Castori, & Schmid, 2012) and may serve as the catalyst that helps adolescents and young adults use tools provided in adherence-promotion interventions (Fisher et al., 2006). This hypothesis could explain why the only available intervention for adolescents and young adults with cancer increased knowledge but demonstrated a limited impact on adherence (Kato et al., 2008). A logical next step for the field is to develop a measure of motivation specific to adolescents and young adults with cancer to allow for the examination of this hypothesis.
Individuals may be motivated to adhere to a medication regimen when it results in a reward, leads to social approval, or prevents a negative consequence (Fisher et al., 2006). Neurodevelopmental changes (i.e., dorsal striatal intrinsic functional connectivity) beginning after the age of 14 years and stabilizing after the age of 24 years cause adolescents and young adults to process rewards and warning signals differently than children and older adults (Porter et al., 2015). As a result, adolescents and young adults are more likely to make choices that result in immediate rewards, particularly related to peer approval, and less likely to be deterred by future negative consequences than individuals at other ages (Ernst, Pine, & Hardin, 2006; Luciana & Collins, 2012). To account for the neurodevelopmental characteristics of adolescents and young adults, a measure of adherence-related motivation must capture the rewards, social factors, and consequences salient to adolescents and young adults ages 15–24 years.
Existing measures of adherence-related motivation for adolescents and young adults ages 15–24 years ask individuals to rate the presence or absence of factors hypothesized to increase motivation. While many available measures include motivators salient to adolescents and young adults (i.e., social approval), they also assume that rewards, social factors, and consequences are equally motivating to all adolescents and young adults (e.g., higher social approval = higher motivation). This measurement approach neglects the fact that even adolescents and young adults of the same age demonstrate different neurological and biological responses to rewards and consequences and, as a result, may experience varying levels of motivation in response to the same level of rewards or consequences. Accurately assessing motivation requires a novel measurement strategy that accounts for the degree to which rewards, social factors, and consequences are likely to motivate a given adolescent or young adult to be adherent.
One innovative approach to overcoming this limitation is to leverage discrete choice experiments (DCEs), a measurement strategy used by health economists to identify the specific rewards and consequences that motivate a given individual’s health-related decisions (Clark, Determann, Petrou, Moro, & de Bekker-Grob, 2014). In a discrete choice experiment, participants are presented with two or more options (termed a “choice set,” e.g., medications to treat type 2 diabetes) with multiple characteristics (termed “attributes,” e.g., side effects [weight gain], effectiveness) that have different values (termed “levels,” e.g., 0, 2.3, 4.5, or 9.0 kg weight gain; Hauber, Mohamed, Johnson, & Falvey, 2009). Participants are asked to consider all attributes and levels and choose the most desirable option. After the first response, another combination of levels for each attribute is presented and the participant again selects their preferred choice. The theory underlying DCEs assumes that choices are guided by the relative importance of each attribute and level (Lancaster, 1966). Thus, analyzing a participant’s responses can elucidate the specific rewards and consequences likely to motivate that individual to perform a given behavior (e.g., adhere to a medication).
While DCEs have been used to understand the factors that motivate adherence decision-making, available measures were designed for adults with chronic conditions other than cancer (e.g., diabetes, Hauber et al., 2009) and include rewards and consequences (i.e., side effects) that can only be addressed by switching medications. These DCEs are not applicable to adolescents and young adults with cancer whose treatment protocols often preclude medication changes. In addition, these DCEs neglect motivational factors like social approval that are particularly salient to adolescents and young adults. Assessing adherence-related motivation among adolescents and young adults with cancer, thus, requires a novel DCE.
The purpose of this study was to use criteria established by the International Society for Pharmacoeconomics and Outcomes Research (Bridges et al., 2011) to develop a DCE that can ultimately be used to identify the factors that motivate adherence among adolescents and young adults (ages 15–24 years) with cancer. To achieve this goal, a multimethod and iterative approach consistent with expert guidelines (Coast et al., 2012) was used to identify attributes and levels relevant to the medication adherence decision context and adolescents and young adults with cancer (Phase I). Next, attributes and levels were combined into choice sets to form the DCE (Phase II). Compliance of the DCE with measure guidelines (Bridges et al., 2011) was assessed by evaluating the statistical efficiency of the experimental design, and the phrases and images included in the DCE were refined with expert feedback (Phase II). Next, pilot testing and cognitive interviewing were used to examine participant burden, participant comprehension, content validity, and achievement of theoretical assumptions underlying DCEs (Phase III). Finally, patient-level responses were examined to identify the factors likely to motivate a given adolescent or young adult with cancer to be adherent.
General Method
The specific aims, procedures, and results corresponding to each phase of the study are detailed in Figure 1. Findings are described in accordance with reporting guidelines for DCEs (Bridges et al., 2011) and measures for use in pediatric psychology (Holmbeck & Devine, 2009). Study data were managed using REDCap electronic data capture tools hosted at our institution. All study procedures were approved by the institutional review board.
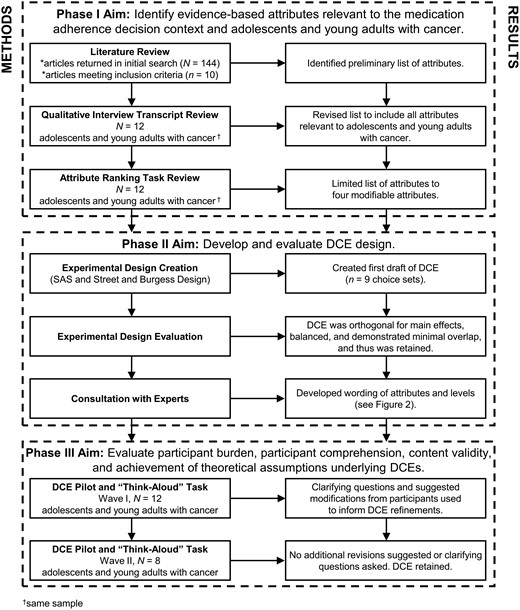
Phase I: Attribute and Level Selection
The purpose of Phase I was to identify the attributes and levels relevant to medication adherence decision-making among adolescents and young adults with cancer.
Method
Three recommended methods were used to develop attributes and levels relevant to the research question and context (Bridges et al., 2011). First, MEDLINE/PubMed was searched for DCEs designed to understand medication adherence decision-making using the following terms: “discrete choice experiment” or “conjoint analysis” and “adherence” or “compliance” or “self-management.” Of the resulting 144 articles, 10 reported on DCEs completed by patients prescribed a daily oral (pill/tablet) medication and were reviewed by the first author. The other 134 articles were excluded as they detailed DCEs completed by physicians or caregivers (as opposed to a patient), regarding a one-time or nonoral medication (i.e., vaccine), and/or regarding a health behavior other than medication adherence (i.e., cancer screening), and thus, unlikely to include attributes relevant to medication adherence decision-making among adolescents and young adults with cancer. Information was extracted to compile a list of potential attributes supported by previously published research.
To ensure the list of potential attributes was inclusive of those relevant to adolescents and young adults with cancer, a secondary analysis was conducted using data from a previously published qualitative study in which 12 adolescents and young adults with cancer described their daily adherence decision-making process (McGrady, Brown, and Pai, 2016). As part of this study, adolescents and young adults also classified each attribute as “not important,” “somewhat important,” or “very important” (see McGrady et al., 2016 for details regarding methods).
Themes were identified by two independent raters using a grounded theory approach and line-by-line coding as described previously (McGrady et al. 2016). Attributes relevant to adherence-related motivation were extracted from these themes and added to the list of potential attributes. Because the long-term goal of the DCE is to provide clinicians with potential targets for psychosocial intervention, nonmodifiable attributes (e.g., dosing schedule) were removed. Once an exhaustive list of potential modifiable attributes was generated, results of the ranking task were used to select relevant attributes for inclusion in the DCE (Hiligsmann et al., 2013).
Results
Previously published studies included attributes related to medication effectiveness, potentially life-threatening adverse effects, mild to moderate side effects, dosing schedule, cost, medication delivery method and characteristics (e.g., pill size), restrictions on daily activities (e.g., dietary restrictions), barriers to medication access, medication administration time, convenience of transporting medication, and overall burden (Supplementary Tables S1 and S2).
Twelve adolescents and young adults with cancer with an average age of 19.91 years (SD = 4.86) completed qualitative interviews and the ranking task (McGrady et al. 2016). Results of the qualitative interviews informed additional revisions to ensure the DCE included attributes relevant to adolescents and young adults with cancer (Supplementary Table S2). First, the attribute “restrictions on daily activities” was modified to account for the fact that participants indicated that these restrictions were often because of medication side effects. Second, the attribute “medication effectiveness” was revised to clarify that participants typically obtained this information from their physician. Third, six additional attributes were added to reflect constructs identified by adolescents and young adults with cancer but not included in previous DCEs.
Nonmodifiable attributes were then removed to enhance the likelihood that DCE results can ultimately be used to inform psychological adherence-promotion interventions. Finally, results of the ranking task (described previously [McGrady et al. 2016]) were used to identify the most important attributes to adolescents and young adults with cancer and limit the comprehensive list to those included in the DCE. The final list included four attributes: parent or significant other emotional support and encouragement; perceived impact of medication adherence on long-term goals; perceived medication effectiveness (per discussion with physician); and perceived impact of medication adherence on daily activities.
Phase II: DCE Experimental Design and Evaluation
The purpose of Phase II was to use the attributes and levels identified in Phase I to design a DCE, evaluate its statistical efficiency, and obtain expert feedback.
Method
In a DCE, participants are presented with multiple choice sets including two profiles. The SAS macro %mktruns was used to identify a reasonable number of choice sets, given the number of attributes and levels (Kuhfeld, 2010). The Street and Burgess theoretical framework for producing optimally efficient DCE designs (Johnson et al., 2013; Street & Burgess, 2007) was used to identify the specific combination of attributes and levels to comprise each profile. An opt-out option (“I would take neither medication”) was included to reflect the fact that medication adherence is a choice and account for the possibility that adolescents and young adults may anticipate not being adherent to either hypothetical medication (see Lancsar & Louviere, 2008 for a discussion of opt-out options).
Statistically efficient DCE designs are orthogonal (all attribute levels vary independently), balanced (each attribute level occurs the same number of times), and demonstrate minimal overlap (two alternatives should not exhibit the same level for a given attribute; Johnson et al., 2013). The statistical efficiency of the resulting DCE was examined using software developed by Burgess (2007).
Once an initial draft of the DCE was compiled, expert feedback was solicited from the three licensed pediatric psychologists (i.e., coauthors A. N. T. and A. L. H. P.) who were providing or overseeing clinical care to adolescents and young adults with cancer at our institution. The first author explained the purpose of the DCE and administered the current draft (see Supplemental Figure S1) of the DCE (as it would be administered to adolescents and young adults) to each expert. Experts were asked to provide feedback on the instructions, wording, and/or images to enhance clarity and potential clinical utility.
Results
Results of the SAS macro %mktruns indicated that orthogonal DCE designs could be developed using 9 or 18 choice sets. The nine choice set option was selected to minimize participant burden and was considered feasible as it falls below the number of choice sets used in a DCE designed to understand support service preferences among adolescents and young adults with cancer (n = 16, Goodall, King, Ewing, Smith, & Kenny, 2012). Results of the matrix of canonical correlations between factors indicated that the DCE design was orthogonal for main effects. The profiles generated using the Street and Burgess theoretical framework were balanced and without overlap, with each attribute level occurring only once in a choice set and in three of nine profiles. In sum, the DCE design met all of the criteria for statistical efficiency (D-efficiency = 100%) and was not modified further before pilot testing.
Feedback provided by the three experts included modifications to level descriptions (e.g., “encourages me to take it” revised to read “encourages me to take it and is proud of me when I do”), addition of graphics to depict levels, and formatting suggestions (e.g., differentiate response options from attributes/levels by using a different color). All suggested revisions were made, resulting in the attributes, levels, and formatting depicted in Figure 2.
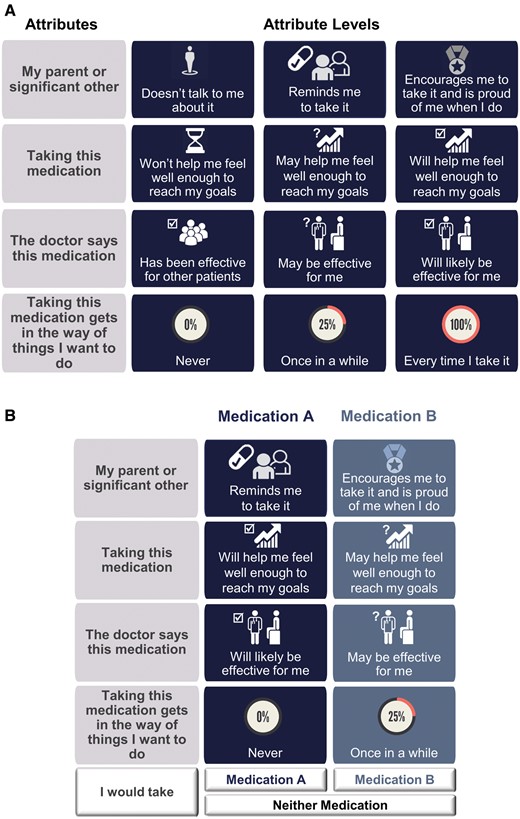
DCE attributes and levels. (A) DCE attributes (column 1) and attribute levels (columns 2–4). (B) Sample DCE choice set.
Phase III: Pilot Testing
The purpose of Phase III was to use two iterative waves of cognitive interviews to assess participant burden, participant comprehension, content validity, and achievement of assumptions underlying DCEs (Willis, 2015). Participant responses were also analyzed to explore the factors likely to motivate a given individual to adhere to his/her medication regimen.
Method
Participants
Twenty adolescents and young adults with cancer receiving care at a Midwestern children’s hospital participated in one of two waves of DCE pilot testing (Wave I, N = 12; Wave II, N = 8) including DCE questionnaire completion and an individual cognitive interview. In the absence of formal sample size guidelines for DCE pilot testing (Lancsar & Louviere, 2008), a sample size of 20 was selected as it is sufficient to identify ∼70% of high-impact problems using cognitive interviewing (Blair & Conrad, 2011) and is consistent with sample sizes included in previous DCE pilot tests identified via literature review (N = 6–24; Bennette et al., 2013; Whitty, Walker, Golenko, & Ratcliffe, 2014). Wave sizes were selected based on published guidelines for measure development and refinement via iterative cognitive interviewing (recommended wave size N = 8–12; Willis, 2015).
Eligible participants included adolescents and young adults between 15 and 24 years of age with a cancer diagnosis prescribed a treatment regimen including oral chemotherapy and/or prophylactic medication. As the DCE was designed to be administered to adolescents and young adults with a range of oncologic diagnoses and medication regimens, purposive sampling was used to maximize sample heterogeneity. Adolescents and young adults were recruited across all primary cancer groups and oral medication regimens, with efforts made to target adolescents and young adults with less common diagnoses and/or medication regimens (e.g., patients enrolled in Phase I clinical trials). In total, 22 of the 28 adolescents and young adults approached consented to participate (enrollment rate = 79%). Two participants discontinued study procedures because of changes in eligibility or availability, resulting in a final sample of 20.
Demographic and Clinical Information
Participants completed a demographic questionnaire. Two trained study staff independently reviewed electronic medical records to obtain clinical information (i.e., cancer diagnosis). Data were entered into two separate databases and compared by the first author for agreement. Interrater agreement was high (97%) and discrepancies were resolved via discussion and consultation of the electronic medical record.
DCE Administration
The DCE was administered to participants via a tablet. Participants in Wave I were administered the DCE developed in Phase II. Results of Wave I cognitive interviews were analyzed as detailed below and used to modify the DCE. The resulting DCE was administered to Wave II participants.
For all participants, in addition to the original nine choice sets included in the DCE, two randomly selected choice sets were included twice to assess response consistency (Ryan, Watson, & Entwistle, 2009). An example choice set included in the DCE is presented in Figure 2. Instructions were modeled after those provided in previous studies of adherence-related DCEs (Hauber et al., 2009) and refined with expert feedback. Specifically, participants were asked to imagine that their medical team was going to prescribe a new medication as part of their cancer treatment protocol. Participants were told they would be presented with two choices and provided with an overview of the four ways in which the medications could differ (attributes). Participants were presented with a “practice” DCE choice set and asked to consider the attributes and their levels and select the medication he or she would be more likely to take as prescribed. After the practice response, participants were provided with 11 choice sets (i.e., nine original and two repeated choice sets) and asked to select their preferred medication in each scenario. Participants were also instructed to assume that any attributes not included in the DCE (e.g., dosing schedule) were consistent across choices. A trained research coordinator was present during DCE administration and encouraged participants to ask clarifying questions as necessary. Responses to DCE items were recorded to explore patterns of responding and the relative importance of each attribute to a given participant (Lancsar & Louviere, 2008). A number of items completed and completion time were recorded to assess participant burden.
Cognitive Interviews
Cognitive interviews including a concurrent think-aloud protocol and retrospective probing were conducted to explore participant comprehension, content validity, and achievement of assumptions underlying DCEs (Drennan, 2003). Before beginning the DCE, participants were asked to “think aloud” while completing each choice set (Ericsson & Simon, 1980). The purpose of this exercise was to explore the assumption underlying DCEs that participants consider all attributes and make trade-offs among them, selecting the profile with the factors likely to motivate them to be adherent. Follow-up prompts were used to encourage continued verbalization of thoughts as necessary. Following DCE completion, retrospective probing was used to gather additional information regarding comprehension (e.g., “What was it like to answer these questions?”) and content validity (e.g., “Are they missing anything that would change your decision?”; Drennan, 2003). In addition, a “writing” function was enabled on the tablet, and participants were asked to mark words, phrases, or images in the DCE that were confusing. All responses were audio-recorded and transcribed verbatim.
Analysis
Descriptive statistics and frequencies were used to summarize demographic and clinical characteristics and measures of burden (items completed; completion time). Qualitative data were coded in NVivo (NVivo, 2012) using an a priori coding scheme. To assess participant burden, a category was created to code comments regarding DCE length and/or duration. To assess participant comprehension, categories were created to code clarifying questions asked during DCE completion, suggested modifications to wording/images, and comments regarding clarity. To assess content validity, categories were created to code relevance/representativeness of attributes, and suggested additional attributes (Haynes, Richard, & Kubany, 1995). Finally, a category was created to explore the extent to which participant response patterns reflected trade-offs that would be consistent with theoretical constructs underlying DCEs (Ryan et al., 2009; Whitty et al., 2014). As DCEs assume that participants will make judgments by considering all attributes, assigning a value to each attribute, and making trade-offs among attributes, a category was created to code verbalizations of this process. Consistent with recommendations for using iterative rounds of cognitive interviewing to inform measure development by Willis (2015), data were analyzed after each of the two waves.
Hierarchical Bayes was conducted using Microsoft Excel XLSTAT to estimate attribute and attribute-level preference scores for each participant (Hauber et al., 2016). Attributes and attribute levels with the highest preference scores indicate the most preferred options for a given individual. It was hypothesized that the attributes with the highest preference scores would differ across participants, reflecting our hypothesis that the factors that motivate a given adolescent or young adult to be adherent are unique to that individual. The attribute levels for impact on long-term goals and impact on daily activities were designed to be interpreted consistently across adolescents and young adults, with a medication that “won’t help me feel well enough to reach my goals” being worse than a medication that “may help me feel well enough to reach my goals,” and both being worse than a medication that “will help me feel well enough to reach my goals.” However, because the preferred methods of providing social support and information regarding effectiveness may be influenced by individual preferences, it was hypothesized that the attribute levels with the highest preference scores would differ across participants. For example, it was hypothesized that some adolescents and young adults would be motivated to be adherent when their parent reminded them to take their medication, while others would be motivated by independent self-management (no parent involvement).
Results
Demographic and Clinical Characteristics
Twenty adolescents and young adults with cancer participated in DCE pilot testing. On average, adolescents and young adults were 18.66 years of age (SD = 2.95; range = 15.35–23.71, Table I) at the time of study participation, and most were currently enrolled in high school (n = 12, 60%). In total, 60% of participants were male and 95% were Caucasian. Owing to purposive sampling efforts to enroll participants with a wide range of diagnoses and medical regimens, the sample included participants with one of 17 cancers that could be classified in one of six diagnostic groups: leukemia (n = 6, 30%), lymphoma (n = 4, 20%), soft tissue sarcoma (n = 3, 15%), CNS tumor (n = 3, 15%), Ewing’s sarcoma (n = 2, 10%), or other cancer type (n = 2, 10%), 10 participants prescribed an oral chemotherapy medication (i.e., n = 3 prescribed a Phase I investigational medication), and 10 participants prescribed an oral antibiotic/antifungal prophylactic medication.
Variable . | Phase I (N = 12) . | Phase III (N = 20) . | ||
---|---|---|---|---|
. | N (%) . | M (SD) . | N (%) . | M (SD) . |
Age at study participation, years | 19.91 (4.86) | 18.66 (2.95) | ||
Gender, male | 8 (67) | 12 (60) | ||
Race | ||||
White or Caucasian | 11 (92) | 19 (95) | ||
Black or African-American | 1 (8) | 1 (5) | ||
Ethnicity | ||||
Hispanic or Latino | 1 (8) | 2 (10) | ||
Primary caregiver | ||||
Biological mother | 9 (75) | 16 (80) | ||
Biological father | 1 (8) | 2 (10) | ||
Significant other | 1 (8) | 1 (5) | ||
Other | 1 (8) | 1 (5) | ||
Educational level* | ||||
High school student | 5 (42) | 12 (60) | ||
High school graduate | 5 (42) | 1 (5) | ||
College student | 0 (0) | 5 (25) | ||
College graduate | 2 (16) | 1 (5) | ||
Oncology diagnosis | ||||
Leukemia | 7 (58) | 6 (30) | ||
Soft tissue sarcoma | 1 (8) | 3 (15) | ||
Lymphoma | 1 (8) | 4 (20) | ||
CNS tumor | 2 (17) | 3 (15) | ||
Ewing’s sarcoma | 0 (0) | 2 (10) | ||
Other | 1 (8) | 2 (10) | ||
Years since first oncology diagnosis | 3.62 (2.91) | 1.57 (1.44) | ||
Prescribed medications | ||||
Oral chemotherapy | 7 (58) | 10 (50) | ||
Oral antibiotic prophylaxis | 5 (42) | 10 (50) |
Variable . | Phase I (N = 12) . | Phase III (N = 20) . | ||
---|---|---|---|---|
. | N (%) . | M (SD) . | N (%) . | M (SD) . |
Age at study participation, years | 19.91 (4.86) | 18.66 (2.95) | ||
Gender, male | 8 (67) | 12 (60) | ||
Race | ||||
White or Caucasian | 11 (92) | 19 (95) | ||
Black or African-American | 1 (8) | 1 (5) | ||
Ethnicity | ||||
Hispanic or Latino | 1 (8) | 2 (10) | ||
Primary caregiver | ||||
Biological mother | 9 (75) | 16 (80) | ||
Biological father | 1 (8) | 2 (10) | ||
Significant other | 1 (8) | 1 (5) | ||
Other | 1 (8) | 1 (5) | ||
Educational level* | ||||
High school student | 5 (42) | 12 (60) | ||
High school graduate | 5 (42) | 1 (5) | ||
College student | 0 (0) | 5 (25) | ||
College graduate | 2 (16) | 1 (5) | ||
Oncology diagnosis | ||||
Leukemia | 7 (58) | 6 (30) | ||
Soft tissue sarcoma | 1 (8) | 3 (15) | ||
Lymphoma | 1 (8) | 4 (20) | ||
CNS tumor | 2 (17) | 3 (15) | ||
Ewing’s sarcoma | 0 (0) | 2 (10) | ||
Other | 1 (8) | 2 (10) | ||
Years since first oncology diagnosis | 3.62 (2.91) | 1.57 (1.44) | ||
Prescribed medications | ||||
Oral chemotherapy | 7 (58) | 10 (50) | ||
Oral antibiotic prophylaxis | 5 (42) | 10 (50) |
N = 1 missing for Phase III.
Variable . | Phase I (N = 12) . | Phase III (N = 20) . | ||
---|---|---|---|---|
. | N (%) . | M (SD) . | N (%) . | M (SD) . |
Age at study participation, years | 19.91 (4.86) | 18.66 (2.95) | ||
Gender, male | 8 (67) | 12 (60) | ||
Race | ||||
White or Caucasian | 11 (92) | 19 (95) | ||
Black or African-American | 1 (8) | 1 (5) | ||
Ethnicity | ||||
Hispanic or Latino | 1 (8) | 2 (10) | ||
Primary caregiver | ||||
Biological mother | 9 (75) | 16 (80) | ||
Biological father | 1 (8) | 2 (10) | ||
Significant other | 1 (8) | 1 (5) | ||
Other | 1 (8) | 1 (5) | ||
Educational level* | ||||
High school student | 5 (42) | 12 (60) | ||
High school graduate | 5 (42) | 1 (5) | ||
College student | 0 (0) | 5 (25) | ||
College graduate | 2 (16) | 1 (5) | ||
Oncology diagnosis | ||||
Leukemia | 7 (58) | 6 (30) | ||
Soft tissue sarcoma | 1 (8) | 3 (15) | ||
Lymphoma | 1 (8) | 4 (20) | ||
CNS tumor | 2 (17) | 3 (15) | ||
Ewing’s sarcoma | 0 (0) | 2 (10) | ||
Other | 1 (8) | 2 (10) | ||
Years since first oncology diagnosis | 3.62 (2.91) | 1.57 (1.44) | ||
Prescribed medications | ||||
Oral chemotherapy | 7 (58) | 10 (50) | ||
Oral antibiotic prophylaxis | 5 (42) | 10 (50) |
Variable . | Phase I (N = 12) . | Phase III (N = 20) . | ||
---|---|---|---|---|
. | N (%) . | M (SD) . | N (%) . | M (SD) . |
Age at study participation, years | 19.91 (4.86) | 18.66 (2.95) | ||
Gender, male | 8 (67) | 12 (60) | ||
Race | ||||
White or Caucasian | 11 (92) | 19 (95) | ||
Black or African-American | 1 (8) | 1 (5) | ||
Ethnicity | ||||
Hispanic or Latino | 1 (8) | 2 (10) | ||
Primary caregiver | ||||
Biological mother | 9 (75) | 16 (80) | ||
Biological father | 1 (8) | 2 (10) | ||
Significant other | 1 (8) | 1 (5) | ||
Other | 1 (8) | 1 (5) | ||
Educational level* | ||||
High school student | 5 (42) | 12 (60) | ||
High school graduate | 5 (42) | 1 (5) | ||
College student | 0 (0) | 5 (25) | ||
College graduate | 2 (16) | 1 (5) | ||
Oncology diagnosis | ||||
Leukemia | 7 (58) | 6 (30) | ||
Soft tissue sarcoma | 1 (8) | 3 (15) | ||
Lymphoma | 1 (8) | 4 (20) | ||
CNS tumor | 2 (17) | 3 (15) | ||
Ewing’s sarcoma | 0 (0) | 2 (10) | ||
Other | 1 (8) | 2 (10) | ||
Years since first oncology diagnosis | 3.62 (2.91) | 1.57 (1.44) | ||
Prescribed medications | ||||
Oral chemotherapy | 7 (58) | 10 (50) | ||
Oral antibiotic prophylaxis | 5 (42) | 10 (50) |
N = 1 missing for Phase III.
Participant Burden
Participant burden was deemed acceptable, as all adolescents and young adults completed all 11 choice sets (100% completion) and completion time averaged 5.83 min (SD = 0.09 min, range = 3.58–11.87 min). This is consistent with qualitative data from the cognitive interviews in which no adolescents and young adults suggested removing items and participants describing length referred to it as “about right,” “okay,” or “not too long.”
Participant Comprehension
During DCE completion, no participants used the writing function enabled on the DCE to mark words or images included in the DCE as “confusing” or “unclear.” However, review of transcripts indicated that the first 12 participants (Wave I) were asking follow-up questions during DCE administration regarding the meaning of each attribute (e.g., “What does it mean to reach my goals?”; “What does that [gets in the way of other things] mean?”). Similarly, participants suggested that clarity could be enhanced by discussing the differences between attribute levels (e.g., “like…the uh different levels, it was hard to tell which one was more likely to be effective than the other”). After reviewing these queries and suggestions, the instructions were revised such that the DCE administrator explained each attribute using examples provided by the adolescent or young adult completing the DCE. For example, when describing the impact of the medication on goals, the DCE administrator would say: “One way the two medications can be different is whether or not taking it will help you feel well enough to reach your goals. Your goals could be things like going back to school, playing sports, or spending time with friends. What is one goal that you would like to achieve once you feel better?” If the participant responded he or she wants to feel well enough to return to high school, the DCE administrator would then explain that he/she will be presented with various options in which the medications “won’t,” “may,” or “will” help him/her feel well enough to return to high school.
The “final” instructions were arrived at following modifications resulting from feedback from Wave I (n = 12), as the eight adolescents and young adults participating in Wave II did not ask any clarifying questions during DCE administration. Of note, all Wave II participants also described the task as “easy” or “pretty easy.” Participant comprehension was supported by response patterns, as all 20 adolescents and young adults responded consistently to at least one of the repeated items (repeated choice Set 1: n = 3 inconsistent responses; repeated choice Set 2: n = 0 inconsistent responses). A copy of the final instructions is available on request.
Content Validity
Attributes appeared to be relevant to adolescents and young adults, as participants did not suggest the removal of any attribute and noted “I think you guys hit the nail on the head” and “I feel like those categories were pretty good.” Additional attributes generated by adolescents and young adults included side effects (n = 4, described as “how you feel afterwards,” “being sick after you take it,” “how well you feel on it,” or “what the drug does to you”), medication timing (n = 3, described as “not having to take it in a certain amount of time,” “timing,” and “how often you have to take it”), medication size (n = 1), taste (n = 1), and current health state (n = 1, “if you’re sick before taking it”). Of note, all attributes except for current health state were included in our initial list of attributes. With the exception of taste, none of the new attributes are amenable to behavioral intervention. As a result, the current attributes were deemed relevant and representative of the factors likely to motivate adolescents and young adults with cancer to be adherent, and retained.
DCE Assumptions: Trade-Offs
While not all participants were able to articulate their underlying thought process during DCE completion, several adolescents and young adults verbalized evidence of making trade-offs. For example, one participant discussed that she would be willing to receive less parent/significant other support if she felt taking her medication would help her reach her goals, noting “…even though I don’t get encouraged to take it, it says it may, uh, help me reach my goals instead of it absolutely won’t.” Another participant with a history of significant treatment-related side effects noted he would be willing to take a medication with less desirable attributes as long as it did not impact his daily activities. Specifically, he reported “I am going to go with medication B because it doesn’t get in the way. No side effects, which…I’ve had pretty bad ones in the past…I guess I am mainly looking at the side effect part of it.”
DCE Results
Consistent with our hypothesis, the attribute most likely to motivate adolescents and young adults to be adherent differed across individuals. Figure 3A includes the individual preferences for each participant, with longer bar segments indicating greater salience of a given attribute. For half of all adolescents and young adults surveyed (n = 10), the decision to adhere to their medication regimen was influenced most by the perceived impact of medication adherence on long-term goals. The most salient motivator was perceived medication effectiveness for 35% of participants (n = 7), parent/significant other support/encouragement for two participants (10%), and perceived impact of medication adherence on daily activities for one participant (5%).
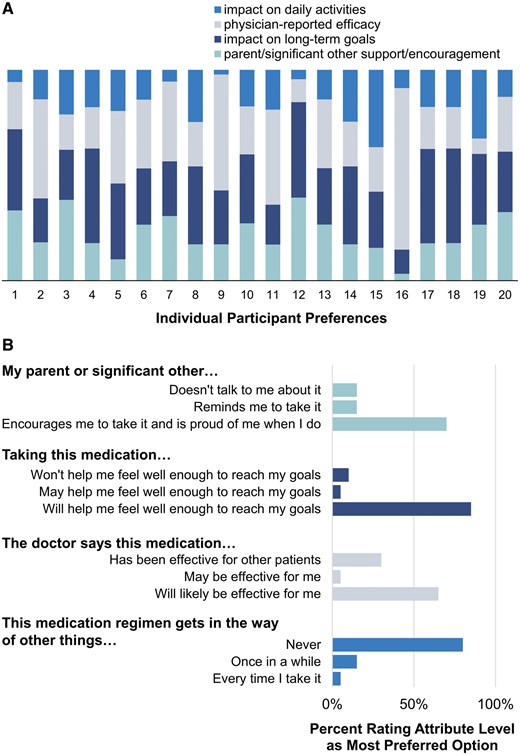
Results of DCE pilot testing. (A) Importance of DCE attributes for each adolescent and young adult. Longer bars represent an attribute of greater importance. (B) Attribute levels with highest preference weight for each adolescent and young adult.
As hypothesized, the types of support and information provision methods most likely to motivate adolescents and young adults to be adherent differed across individuals (Figure 3B). The majority of participants (n = 14, 70%) reported that they would be most likely to take a medication when their parent/significant other encourages them to take their medication and is proud of them when they do (supporting quote: “…and someone encouraging me to take it will definitely help me take it”). However, some participants (n = 3, 15%) would be most likely to take a medication when their parent/significant other reminds them (supporting quote: “…they don’t need to be, like, proud…as long as they just say, like, ‘yeah, do it’.”) and others (n = 3, 15%) would be most likely to take a medication when their parent or significant other does not talk to them about it (supporting quote: “It’d be easier to just take it and not, like, talk about it”). Similarly, 65% of participants (n = 13) would be most likely to take a medication that their doctor has said will likely be effective for them (supporting quote: “…I’d rather them say this, like I don’t care about the other patients, I’d rather focus on what happens with me”) but nearly a third (n = 6, 30%) would be most likely to take a medication that their doctor said had been effective for other patients (supporting quote: “…and with this one I would probably pick B, because they’ve seen good results in other patients”).
Discussion
This multimethod iterative study resulted in the development of a new measure of adherence-related motivation designed specifically for adolescents and young adults with cancer prescribed oral medication. Results of cognitive interviews confirm that parent/significant other support/encouragement, impact on long-term goals, physician-reported effectiveness, and impact on daily activities are salient motivators for medication adherence among adolescents and young adults with cancer. These attributes are consistent with factors included in theoretical models of adherence-related motivation (Fisher et al., 2006).
During the cognitive interviews, adolescents and young adults verbalized that they were simultaneously considering multiple attributes and weighing the relative importance of each potential motivator. Coupled with response consistency rates similar to those exhibited by adults (92%; Ryan et al., 2009; Whitty et al., 2014), these results suggest that adolescents and young adults as young as 15 years of age may demonstrate the cognitive capabilities necessary to complete DCEs. Adolescents and young adults comprehended the majority of DCE content and participant feedback was used to inform additional refinements to enhance readability.
Response patterns support our hypothesis that the factors likely to motivate adolescents and young adults to be adherent differ across individuals. No two participants evidenced the same preference weights, suggesting that the relative importance of each factor in motivating adherence is unique to each individual. Further, as the most important attribute levels also differed across adolescents and young adults, the most effective method for targeting specific factors to increase adherence likely differs across individuals. For example, some adolescents and young adults reported that they would be most motivated by parent/significant other encouragement and praise, while others would be motivated by increased independence and decreased reminders/discussion of medication. For the individual who is motivated by parent/significant other encouragement and praise, the parent/significant other should be included in the intervention, so that support can be maximized through planned regular discussion and praise of patient adherence. In contrast, if the individual demonstrates a desire for increased independence, the clinician could use intervention strategies such as behavioral planning/contracting with “rewards” of decreased parent/significant other involvement following achievement of treatment goals (e.g., 95% of prescribed doses taken). As other available measures do not provide this type of individual-level data, DCEs have the potential to significantly change our approach to assessment of treatment targets. Specifically, DCEs could provide clinicians with the information necessary to tailor their treatment to the most salient motivators for a given adolescent or young adult with cancer, thereby potentially increasing the efficiency of adherence-promotion intervention delivery. Although beyond the scope of this manuscript, data from DCEs can also be analyzed using latent mixture class models to identify segments of individuals with similar preference profiles (e.g., groups of adolescents and young adults likely to be motivated by similar psychological interventions; Hauber et al., 2016). If demographic (e.g., age) and clinical characteristics (e.g., cancer diagnosis, time since diagnosis) are included and significantly predict segment membership, tailoring intervention content based on these variables may provide one method of matching intervention content to patient preferences.
This study has several limitations. First, as the goal of this study was to develop a clinically relevant measure that could be administered to adolescents and young adults with a range of oncologic diagnoses and medication regimens, the participant population included a heterogeneous group. As a result, attributes particularly relevant to one medication regimen or disease group (e.g., nausea postchemotherapy) may not be included in the DCE, and individual differences in responding may, in part, be attributable to clinical characteristics (e.g., treatment duration, age at diagnosis). Second, despite the use of multiple methods (i.e., literature review; qualitative interviews) to identify attributes, the DCE may not include all factors likely to motivate adolescents and young adults to be adherent. Third, while the demographic characteristics of the sample are generally consistent with our patient population, results may not generalize to more ethnically or racially diverse samples. Fourth, in the absence of sample size guidelines for DCE pilot testing, guidelines for cognitive interviewing and previously published pilot tests of DCEs were used to inform sample size selection. While no new issues were identified in Wave II, a larger sample may have informed additional refinements to the DCE. In all, while results of this pilot study suggest that the DCE has the potential to be a clinically useful instrument for assessing adherence-related motivation among adolescents and young adults with cancer, measure validation (i.e., evaluation of reliability, validity, and utility; evaluation of demographic and clinical predictors of responding) will require additional large-scale testing (Holmbeck & Devine, 2009). Given the heterogeneity inherent in the adolescent and young adult oncology population, the samples comprising these studies should include the distribution necessary to explore potential variations in responding by demographic (i.e., ethnicity; race) and clinical (i.e., cancer diagnosis, time since diagnosis) characteristics.
In conclusion, the DCE appears to capture some of the salient factors that motivate adolescents and young adults with cancer to adhere to oral medications. Participants demonstrated little difficulty in completing the measure and provided helpful feedback to inform modifications. The resulting instrument is currently undergoing large-scale testing as part of a longitudinal observational study of adolescents and young adults with cancer. If further supported, the DCE instrument could provide clinicians with insight into how to best motivate an individual patient. Given the prevalence and life-threatening implications of nonadherence among adolescents and young adults with cancer and the dearth of evidence-based adherence-promotion interventions, developing and evaluating interventions using this DCE may have the potential to transform clinical care.
Supplementary Data
Supplementary data can be found at Journal of Pediatric Psychology online.
Acknowledgements
The authors gratefully acknowledge the adolescents and young adults who participated in this study. Naomi E. Joffe, PhD is also thanked for her feedback on the measure described in this manuscript. In addition, the authors would like to thank Gabriella Breen for her assistance with data collection and manuscript formatting and Vincent D’Anniballe for his assistance with manuscript formatting.
Funding
This study was supported by a Targeted Research Grant from the Society of Pediatric Psychology for M.E.M. M.E.M. is supported by the National Cancer Institute of the National Institutes of Health under award number K07CA200668. Data management (REDCap) was supported by the University of Cincinnati Center for Clinical and Translational Science and Training (CCTST) with funding from the National Institutes of Health Clinical and Translational Science Award (CTSA) number 1ULTR001425-01. The content is solely the responsibility of the authors and does not necessarily represent the official views of the National Institutes of Health.
Conflicts of interest: None declared.
References