-
PDF
- Split View
-
Views
-
Cite
Cite
Sining Liu, Jiashu Chen, Yan Luo, Junpeng Ren, Yahan Chen, Zhengbing Yan, Wenxuan Han, Coordination between leaf pH and other leaf traits are divergent among life-forms and eco-geographic regions in plants of northern China, Journal of Plant Ecology, Volume 17, Issue 6, December 2024, rtae103, https://doi.org/10.1093/jpe/rtae103
- Share Icon Share
Abstract
As a key functional trait affecting many physiological processes, leaf pH is closely related to other leaf traits at the local scale. Nevertheless, whether and how leaf pH is linked with other leaf functional traits across geographic scales remains unclear. A field survey in northern China was conducted to investigate the relationships between leaf pH and some key leaf structural (specific leaf area, SLA; leaf dry matter content, LDMC) and chemical traits (elemental concentrations; total dissolved solids, TDS; practical salinity), as well as the effects of environmental factors on these relationships. Our results showed that the trait coordination may vary in degree or direction across eco-geographic regions (arid vs. non-arid regions) and life-forms (woody vs. herbaceous plants). Generally, leaf pH was negatively related to SLA, but positively related to TDS and salinity. However, leaf pH and LDMC were negatively correlated in arid regions but positively correlated in non-arid regions; leaf pH covaried with N (similarly, with Ca, Mg and Na) in the same direction for both herbaceous and woody plants in arid regions, but not in non-arid regions. Climatic factors mainly influenced the relationships of leaf pH with leaf Ca and Fe concentrations, while soil factors mostly affected those with leaf P, Ca and Mn concentrations. Our findings highlight the divergent coordination between leaf pH and other leaf traits across life-forms and eco-geographic regions and may shed light on the in-depth understanding of the role of acid-base balance in plant eco-physiological processes and ecological adaptation over biogeographic scales.
摘要
作为对植物许多生理过程存在重要影响的一种功能性状,叶pH值在局域尺度上与其他叶性状密切相关。然而,在地理尺度上植物叶pH值与其他叶性状的关联性尚不清楚。本研究基于实地调查,分析了中国北方植物叶pH值与某些关键叶结构性状(比叶面积,SLA;叶干物质含量,LDMC)和叶化学性状(叶元素浓度;叶总溶解固体,TDS;叶实际盐度)之间的协调关系,并探讨了环境因素对这些关系的影响。研究结果显示,不同生态地理区域(干旱区与非干旱区)和植物生活型(木本植物与草本植物)的叶pH值与其他叶性状间的协调关系在强度或方向上存在差异。叶pH值总体上与SLA呈负相关,与叶TDS和叶盐度呈正相关;但干旱区的植物叶pH值与LDMC显著负相关,而在非干旱区中这两个叶性状显著正相关;在干旱区, 草本植物的叶pH值与叶N、Ca、Mg和Na浓度的协变与木本植物中的协变方向一致,但在非干旱区的草本和木本植物中则不一致。叶pH值与叶Ca、Fe浓度间的协调性主要受气候影响,而叶pH值与叶P、Ca和Mn的协调关系主要受土壤因素影响。上述研究结果强调了不同生态地理区域和不同植物生活型的叶pH值与其他叶性状的协变关系会发生变化,该发现有助于深入理解酸碱平衡在植物生理生态过程和生物地理尺度上的生态适应中的作用。
INTRODUCTION
Plant functional traits refer to a series of plant properties closely associated with plant growth, mortality, environmental adaptation and ecosystem processes, such as physical forms, chemical substance contents and physiological features (Cornelissen et al. 2003; Kattge et al. 2019; Reich et al. 1997). Many studies on the leaf economics spectrum and hydraulic traits have shown tightly coordinated relationships among multiple traits which are closely associated with resource acquisition and utilization of plants (Liu et al. 2020; Maréchaux et al. 2015; Prentice et al. 2014; Villagra et al. 2013; Walls 2011; Wright et al. 2004; Zwieniecki et al. 2002). These closely related functional traits jointly determine plant survival strategies. Leaf pH, as an inner environment indicator, affects the plant enzymatic reaction and metabolism by regulating the enzyme activity and ion exchange (Husson 2013; Rengel 2002; Sattelmacher 2001). Furthermore, leaf pH, a species-specific trait has also been demonstrated to be linked with a variety of plant functional traits (Cornelissen et al. 2006; Jia and Davies 2007; Jiang 1991; Tao et al. 2019). Consequently, exploring the relationship between leaf pH and other plant traits can shed light on the in-depth understanding of the role of acid–base balance in plant physiological processes and ecological adaptation.
Previous studies have been reported that leaf pH is closely related to various plant nutrient (e.g. C, N, K, Ca and Mg) concentrations in the evergreen woody species in a tropical mountain seasonal rainforest (Jiang 1991) and a diverse set of subarctic plant species and types (Cornelissen et al. 2006); and these elements can regulate leaf pH by influencing the intracellular and apoplastic cation–anion balance (Brady and Weil 2010; Gollan et al. 1992; Marschner 2012). Leaf chemical traits, such as total dissolved solids (TDS) and practical salinity (Salinity), are closely related to the soluble and readily dissolvable substances in the extract (Sparks et al. 1996), and various solute concentrations (e.g. base cations, K+, Ca2+, Mg2+ and Na+). These chemical traits jointly regulate the pH value of the solution (Li 2001). For leaf solution, the relationship between TDS and pH, which are both closely related to the solute, remains unclear yet. Leaf structural traits, such as specific leaf area (SLA) and leaf lignin content, are also closely related to leaf pH (Cornelissen et al. 2006; Jia and Davies 2007). SLA has been examined to be positively correlated with leaf pH for only a small number of species (Cornelissen et al. 2006). Nevertheless, the current understanding of the relationships between leaf pH and other leaf chemical traits, and structural traits, was mainly based on studies focusing on the constrained plant populations and regions, with less known about the generalizability of the leaf trait coordination over large biogeographic extents.
Leaf trait relationships have been found to change across different growing environments (e.g. those with different water conditions) (Liu et al. 2021; Westoby and Wright 2003), and among plant functional types (PFTs, e.g. plant life-forms) (Bloomfield et al. 2018; Garnier and Laurent 1994; Lambers et al. 2008; Messier et al. 2017). For example, the relationship between leaf dry matter content (LDMC) and SLA is negative in slow-growing species with sclerophyllous leaves, but positive in succulent plants (Pérez-Harguindeguy et al. 2013). Leaf economics trait relationships have been reported at the global scale (Wright et al. 2004) but can be shifted by climatic conditions (Wright et al. 2005a, 2006) and PFTs (Messier et al. 2017; Santiago and Wright 2007). Based on literature data reported by Jiang (1991), we found that this phenomenon may also exist between leaf pH and other traits: leaf pH was positively correlated with leaf P concentration for evergreen broad-leaved woody plants in Jianfengling, Hainan, China, which is inconsistent with our recent results reported for terrestrial and aquatic deciduous woody and herbaceous plants in temperate areas in China (P > 0.05; Chang et al. 2024; Liu et al. 2021). These results imply that the relationship between leaf pH and nutrients may depend on PFTs. Studies showed that the leaf pH of terrestrial plants was significantly negatively correlated with leaf C concentration and positively correlated with leaf N concentration (Cornelissen et al. 2006; Liu et al. 2019), which are not applicable to aquatic and helophytic herbaceous macrophytes (Liu et al. 2021). However, it is not clear whether these differences are caused by environmental water conditions (terrestrial vs. aquatic) or the shift of PFTs. We speculate that the relationships between leaf pH and other traits associated with diverse survival strategies (e.g. conserved vs. competitive), may indeed exhibit different responses to environmental changes and vary among PFTs. However, it is unknown whether and how the relationships between leaf pH and other tightly associated plant functional traits change at large spatial scales spanning various environmental conditions and species compositions.
Therefore, to explore how the relationships between leaf pH and other plant functional traits vary among PFTs and across regions with contrast water conditions, we investigated the leaf pH and multiple functional traits in arid and non-arid regions of northern China, and compared the relationship between leaf pH and other leaf traits across different PFTs (life-forms: herbaceous vs. woody plants) and eco-geographic regions with different water conditions (arid vs. non-arid regions). A variety of environmental factors including climatic and soil properties, which can reflect the environmental water conditions and soil nutrient availability (possibly affecting the leaf-juice chemical properties), were also investigated to explore their possible contribution to the variation of these relationships. Accordingly, we hypothesize that: (i) leaf pH has a close relationship with multiple leaf chemical (i.e. leaf nutrient (C, N, P, K, Ca, Mg, Fe, Mn and Na) concentrations, TDS and practical salinity (Salinity, the ratio of the mass of dissolved substances to the mass of seawater/solvent, which is a dimensionless quantity with typical seawater having a practical salinity of 35)) and structural traits (SLA and LDMC) at the large geographic scale; and these relationships vary with life-forms (herbaceous vs. woody plants) and eco-geographic regions (arid vs. non-arid regions) due to divergent adaptation strategies in plants, (ii) because of the similar habitat and different survival strategies, different life-forms in the same region are expected to differ in the height of correlations but show the same direction. Similarly, because of the contrasting water conditions between regions, which have great effects on plant morphology, physiology and survival strategy, the same life-forms are expected to differ in the height of correlations but show the same direction. Based on our previous study (Liu et al. 2019), the east-west transect of northern China (EWTNC), which is mainly characterized by a water availability gradient according to the aridity index (AI) (i.e. the ratio of precipitation to potential evapotranspiration), was selected as the survey region to test these hypotheses.
MATERIALS AND METHODS
Study sites
This study was conducted in northern China, and 22 sampling sites were investigated along the longitudinal gradient of northern China. For each site, 1–5 plots were set up depending on the local conditions. Given a uniform vegetation cover composition and limited variability of the desert scrub, only one plot (10 m × 10 m) was established. For the substantial variations in vegetation of mountain forests and grasslands, multiple plots (20 m × 30 m) were established. This transect followed EWTNC in Liu et al. (2019), but the artificial vegetation in North China Plain and relatively isolated sample plot in Qilian Mountain in the transect were removed to reduce special point and artificial interference. These two samples were replaced by two natural grassland samples, which were relatively few in the original transect. Except for these two new sites, all leaf pH data and samples were the same as in Liu et al. (2019). The longitude and latitude of the new EWTNC spanned 45.7° (83.5°–129.2° E) and 16.5° (35.4°–51.9° N), respectively, and the elevation ranged from 259 m in the Xinjiang (Sinkiang) desert shrubland to 2467 m in the Luya Mountains. The EWTNC nearly contained all the representative vegetation types (e.g. forest, grassland and desert shrubland) in northern China (Supplementary Fig. S1).
The vegetation composition of forests in northern China tends to be straightforward, typically characterized by one to three dominant tree species alongside associated shrubs and herbs. The dominant and common species of the representative vegetation types in arid and non-arid regions were investigated. We collected all tree species present in the forest, along with associated shrubs and herbs with large biomass. In grasslands and desert scrubs, based on prior vegetation surveys, we sampled species comprising 80% of the above-ground biomass within the quadrats. Due to the sparse vegetation and low species richness of desert shrubs, the number of species collected per site typically ranged from 2 to 5. Conversely, mountain forests and grasslands exhibit greater species richness, with the number of species ranging from 4 to 16 (see more details in Liu et al. 2019 and Supplementary Table S1).
Sampling and measurement
Fully expanded sunlit leaves were sampled from all investigated mature and healthy plants (herb, shrub and tree), and four or five replicates were collected for each species. Each individual of trees and shrubs represented a replicate. For small shrubs in desert scrub and for those herbaceous plants that have a low individual biomass, the amount of leaf material from a single individual was insufficient to measure all traits, so each replicate consisted of multiple individuals, with a minimum sampling standard of 10 g of fresh leaf weight (see more details in Liu et al. 2019).
The collected samples were separately stored in ziplock bags and kept in a freezer or cooling box during the collection process. The fresh weight (electronic balance with 1% accuracy) and leaf area (with a flatbed scanner) of 20 leaves were measured, and then oven-dried or refrigerated for the further measurement of plant traits. Leaf samples frozen in a freezer (c. −16 °C, such preservation method was proved to have no significant effect on the pH value of fresh leaves, (Liu et al. 2022)) were used to measure leaf pH, TDS (ppm) and practical salinity (Salinity). Samples were pretreated and measured according to Liu et al. (2019). Leaf pH, leaf TDS and Salinity were all measured by a multi-functional pH meter with different electrodes (Bante902 multi-parameter meters, Bante). The other leaf sample was oven-dried first at 105 °C for 30 min and subsequently at 65 °C to a constant weight. The dry weight was obtained to calculate the SLA, cm2 g−1 and LDMC, %. It is noteworthy that the SLA data of arid regions and monocot herbaceous plants were excluded from the analysis. Arid region plants often possess succulent, columnar or needle-shaped leaves, or even replaced by assimilation branches, while monocot herbaceous plants tend to curl their leaves severely upon collection, resulting in significant measurement errors. Therefore, these data were ultimately omitted from our study. In addition, other missing specific data are shown in Supplementary Table S2.
The oven-dried samples were ground with a ball mill (NM200, Retsch, Haan, Germany). 100.0 ± 1.0 mg of the oven-dried samples was used for assaying the leaf C and N concentrations using an elemental analyzer (vario MACRO cube, Elementar, Germany). 300.0 ± 10.0 mg of the samples was digested by HNO3–H2O2 digestive solution with a Microwave Digestion System (MARS Xpress, CEM, American) for the measurement of leaf P and other elemental (K, Ca, Mg, Fe, Mn and Na) concentrations, which were determined by an inductively coupled plasma emission spectrometer (ICP-OES 7300DV, PerkinElmer, American).
Three topsoil samples were randomly collected and thoroughly mixed for each sample plot. The ground soil (through a 2-mm sieve) was extracted with deionized water in 1:2.5 soil–water mass ratio suspension at 250 rpm for 10 min, then stationary for 30 min to solid–liquid separation. Soil pH and soil TDS were measured by a multi-functional pH meter (Bante902 multi-parameter meters, Bante, China).
Geographic and environmental factors
Geographic (longitude, latitude and altitude) and environmental (climatic and soil) factors were investigated to explore their effects on the variation of relationships between leaf pH and other leaf traits. Longitude, latitude and altitude data were recorded contemporaneously during sampling with Handheld Global Positioning System receiver (GPS). Climatic indicators included mean annual temperature (MAT, °C), mean annual precipitation (MAP, mm) and AI, which were used to examine the effects of temperature and moisture conditions. MAT and MAP were obtained from WorldClim Version 2.0 (http://worldclim.org/version2, Fick and Hijmans 2017), while AI was extracted from the database of CGIAR-CSI (http://www.cgiar-csi.org). Soil factors included soil physical composition, nutrient concentration, pH, TDS and ion-exchange capacity, which were used to explore the possible effects of soil properties on relationships between leaf pH and other traits. Soil pH and TDS were measured in the laboratory. Soil nutrient concentration (soil total N, P and K), physical composition (sand, silt and clay content) and ion-exchange capacity (exchangeable K, Ca, Na, Mg and cation exchange capacity (CEC)) were extracted from the database of the gridded Global Soil Dataset for use in Earth System Models (GSDE, 0–15 cm layer; Shangguan et al. 2014). All the extracted data were interpolated at 0.5° resolution. (see more details in Supplementary Tables S1 and S3).
Statistical analysis
The AI is a widely used index to evaluate the dryness of the climate at a given region. According to AI, six subtypes of drylands are defined: cold (potential evapotranspiration < 400 mm), hyper-arid (AI < 0.05), arid (0.05 ≤ AI < 0.2), semi-arid (0.2 ≤ AI < 0.5), dry subhumid (0.5 ≤ AI < 0.65) and humid (AI ≥ 0.65) (Middleton and Thomas 1997). In simplifying the above classification and considering rainfall levels, we categorized sampling sites into arid (AI < 0.2 and MAP < 200 mm) and non-arid (AI > 0.2 and MAP > 200 mm) regions for comparison. The relationships between plant traits were analyzed at the individual level. The terrestrial plants were classified into herbs and woody plants according to life-form. Before the statistical analysis, except for leaf pH, all trait data were log10-transformed approach a normal distribution. One-way analysis of variance was performed to examine the effects of life-forms and eco-geographic regions on leaf pH and other leaf chemical and structural traits (i.e. SLA, LDMC, TDS, Salinity and the nutrient concentrations) involved here. Standard major axis (SMA) regression was performed to examine and compare the relationships between leaf pH and other leaf chemical (leaf nutrient (C, N, P, K, Ca, Mg, Fe, Mn and Na) concentrations, TDS and Salinity) and structural traits (LDMC and SLA) of plants across different life-forms (woody and herbaceous plant) and eco-geographic regions (arid and non-arid regions). This analysis was employed to evaluate the first hypothesis. In addition, the regression slope differences between life-forms and regions were also analyzed using the smart package of R (Warton and Weber 2015; Westoby and Wright 2003). The comparison analysis of the regression slopes was dedicated to explore divergent relationships between leaf pH and other traits, which was the content of the second hypothesis.
To assess the effects of geographic and environmental factors on the leaf pH–trait relationships, intra-site SMA slopes of these relationships were calculated for 22 sampling sites. Dicotyledon with a wide distribution and a slightly larger number of species were selected as research objects, for each site zero to eight monocotyledon and gymnosperms species were removed, and a total of 23.8% of the data was removed. Pearson correlation analysis was adopted to analyze the relationship between geographic and environmental factors (all factors mentioned in Geographic and environmental factors) and intra-site SMA slopes. Only the significant SMA slopes (P < 0.05) were incorporated in this analysis. All the statistical analyses were performed in R 4.1.2 (R Core Team 2017) and IBM SPSS Statistics 20 (2011, ver. 20; SPSS Inc.).
RESULTS
Relationship between leaf pH and other leaf traits in the arid region
Leaf pH was closely related to leaf chemical and structural traits in arid regions, but their relationships differ significantly between life-forms (Figs 1 and 2). For both herbaceous and woody plants, leaf pH was negatively correlated with leaf C and Ca concentrations and LDMC; and positively correlated with leaf N, Na and Fe concentrations, TDS and Salinity; and weakly correlated with leaf P concentration, but the significance level, direction and slope of the SMA regression differed significantly between these two life-forms, except for those relationships with leaf N concentration and LDMC (Supplementary Table S4). For woody plants, the relationship between leaf pH and leaf P, K, Mg and Fe was not significant, but only the leaf pH–leaf P relationship was insignificant for herbaceous plants. The SMA regression slopes of woody plants relative to herbs were significantly higher for those of leaf pH against leaf Ca and Na concentrations, TDS and Salinity (Supplementary Table S4; Figs 1 and 2). In contrast, the regression slopes of leaf pH against leaf K, Mg and Mn concentrations showed opposite trends between these two life-forms, with significantly negative values for herbs and positive values for woody plants (P > 0.05 for K, P = 0.001 for Mn and P = 0.08 for Mg) (Fig. 1; Supplementary Table S4).
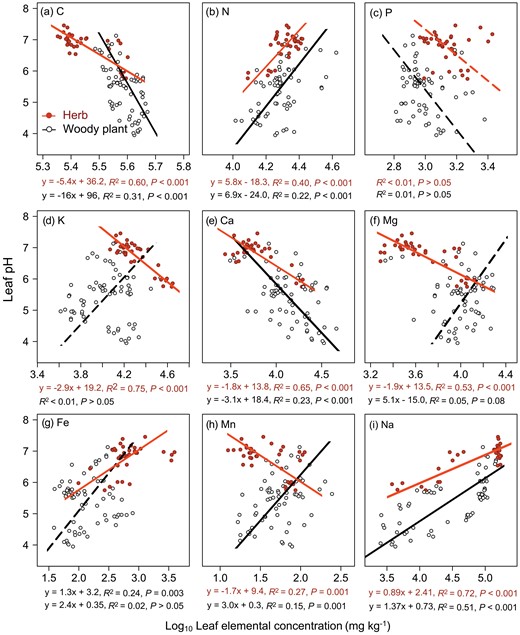
Relationship between leaf pH and leaf elemental concentrations of (a) C, (b) N, (c) P, (d) K, (e) Ca, (f) Mg, (g) Fe, (h) Mn and (i) Na for woody plants and herbs in arid regions. The fitted lines were determined by SMA regression model. Solid line denoted the relationship with P < 0.05, and dashed line denoted the relationship with P > 0.05.
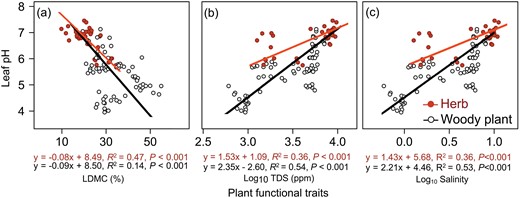
Relationship between leaf pH and (a) LDMC, (b) TDS and (c) salinity for woody plants and herbs in arid regions. The fitted lines were determined by SMA regression model. Solid line denoted the relationship with P < 0.05.
Relationship between leaf pH and other leaf traits in non-arid regions
Herbaceous plants showed inconsistent trends with woody plants in the relationship between leaf pH vs. leaf N, Ca, Mg or Na concentration: leaf pH of herbs increased with increasing leaf N (P = 0.016), Ca (P < 0.001), Mg (P = 0.001) and Na (P < 0.001) concentrations, while leaf pH of woody plants showed a completely opposite trend with the above elements (P < 0.001 for N, Ca and Mg, and P = 0.107 for Na). The relationship between leaf pH and leaf Mn concentration of both herbaceous and woody plants was weak (P > 0.05) in the non-arid regions. In addition, there was no significant correlation between leaf pH and leaf C, P, K and Fe in herbaceous plants, but only the relationship between leaf pH and Na of woody plants was insignificant. Woody plants showed stronger relationships (based on R2 of the regression model) between leaf pH and leaf C, P, K and Fe concentration than herbaceous plants: leaf pH of woody plants was extremely significantly negatively correlated with leaf C and P and positively correlated with leaf K and Fe; while there was no significant correlation between leaf pH and these traits in herbs (Fig. 3). In addition, leaf pH of both herbs and woody plants showed significantly negative correlations with SLA and increased significantly with increasing LDMC, TDS and practical salinity (Fig. 4).
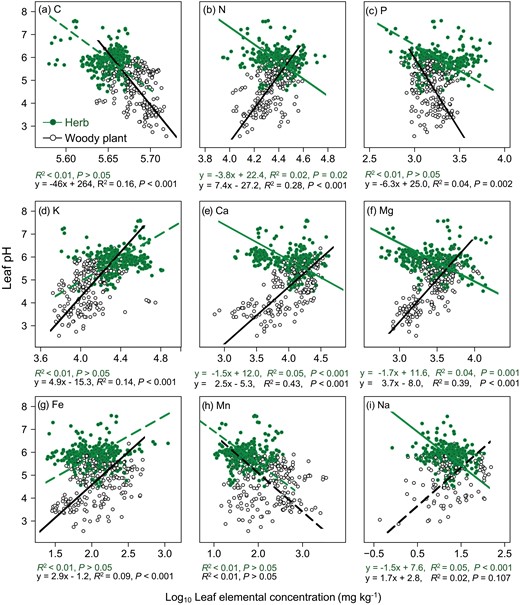
Relationship between leaf pH and leaf elemental concentrations of (a) C, (b) N, (c) P, (d) K, (e) Ca, (f) Mg, (g) Fe, (h) Mn and (i) Na for woody plants and herbs in non-arid regions. The fitted lines were determined by SMA regression model. Solid line denoted the relationship with P < 0.05, and dashed line denoted the relationship with P > 0.05.
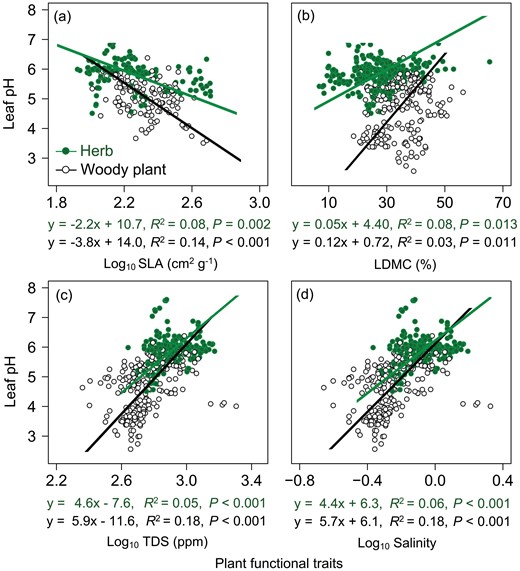
Relationship between leaf pH and (a) SLA, (b) LDMC, (c) TDS and (d) salinity for woody plants and herbs in non-arid regions. The fitted lines were determined by SMA regression model. Solid line denoted the relationship with P < 0.05.
Interregional variation in the relationship between leaf pH and other leaf traits
Compared with arid regions, non-arid regions had certain differences in the relationships between leaf pH and leaf chemical (Figs 1 and 3) and structural traits (Figs 2 and 4) for both herbaceous and woody plants. Specifically, the relationships of leaf pH with leaf C, P and Fe concentrations, TDS and practical salinity in non-arid regions showed the same direction but higher SMA regression slopes in comparison with those in arid regions (Figs 3 and 4; Supplementary Table S4).
In non-arid regions, leaf pH of both herbaceous and woody plants increased significantly with increasing LDMC (Fig. 4), which was opposite to that in arid regions. Meanwhile, the relationships of leaf pH with other chemical and structural traits showed divergent trends between these two regions. From arid to non-arid regions, the significant correlation between leaf pH and leaf K, Fe and Mn of herbaceous plants became insignificant, while the relationship between leaf pH and leaf P, K and Fe of woody plants showed an opposite variation tendency (from insignificant to significant, Figs 1 and 3).
Influence of environmental factors on the relationships of leaf pH vs. other leaf traits
Within-site slopepH-LP (the SMA slope for the relationship between leaf pH and leaf P concentration; the similar definition for the abbreviation as follows) and slopepH-LMn were mainly affected by soil physical and chemical properties; slopepH-LCa and slopepH-LFe were jointly affected by geography, climate and soil factors. Most slopes (slopepH-LDMC, slopepH-TDS, slopepH-Salinity, slopepH-LC, slopepH-LN, slopepH-LK and slopepH-LNa) were less affected by geographic and environmental factors, and mainly affected by soil physical and chemical properties (Fig. 5). In addition, slopepH-SLA and slopepH-LMg had no significant correlation with all these geographic and environmental factors studied.
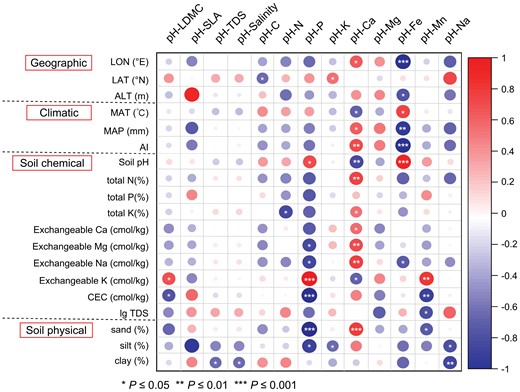
Relationships of SMA slope with geographic and environmental factors across sites. The SMA slope is derived from the relationships between leaf pH and other traits using the SMA regression. Please refer to Supplementary Table S2 for the data.
DISCUSSION
Our study focused on the variation of the relationships between leaf pH and multiple other leaf traits and explored the influences of plant life-forms, and eco-geographic regions on these relationships. Basically, the variation in water conditions shown between arid vs. non-arid regions, and the different strategies to cope with water stress shown between the life-forms of herbaceous vs. woody plants, may reflect the biophysical constraints and evolutionarily viable strategies. Our results confirmed that leaf pH was significantly correlated with the key traits in the leaf economics spectrum (e.g. leaf C, N and P concentration and SLA) and many other functional traits (e.g. leaf metal elemental concentration, LDMC and TDS). Meanwhile, the relationship between leaf pH and other leaf traits changed across plant life-forms and eco-geographic regions. These results partly supported the two hypotheses. However, in contrast with our expectation, even in the same eco-geographic region the leaf pH–other traits relationship for different life-forms (e.g. herbaceous vs. woody plants) could have opposite directions. These results suggested that the relationship between leaf pH of different functional plants and nutrient strategies varies inconsistently in response to environmental changes, reflecting distinct ecological adaptation strategies. The different covariant relationships between various leaf traits including leaf pH determined the different adaptation strategies of woody and herbaceous plants to the environment and their roles in the ecosystem (Garnier and Navas 2012; Wright et al. 2007, 2010).
Leaf chemical and structural traits are closely related to leaf pH at large geographic scales
Leaf pH was significantly correlated with various leaf nutrient concentrations, which verified our previous conjecture that the variation in leaf pH among plant life-forms was closely related to nutrient concentration (Liu et al. 2019). A small part of the relationship between leaf pH and traits (leaf C, TDS, practical salinity and SLA) showed the same direction (SMA slope) in different eco-geographic regions and life-forms (Figs 1–4; Supplementary Table S2). Leaf C concentration, as the main structural material of leaves, showed a negative correlation with leaf pH for all the plants investigated in non-arid regions (Figs 1 and 3), which was consistent with the results of Cornelissen et al. (2006). The positive correlations of leaf pH with leaf N and metal elemental concentrations of woody plants were consistent with those obtained via our analyzing the data from the previous study on woody plants in Jianfengling, Hainan Province, China (Jiang 1991). However, there was an opposite result in the correlation between leaf pH and leaf P compared with that according to the data from Jiang (1991). The leaf pH of different life-forms has inconsistent responses to environmental factors (soil pH) (Liu et al. 2019; Luo et al. 2021). The majority of the woody plants we investigated were deciduous broad-leaved plants, while most of the trees in Jiang (1991) were evergreen broad-leaved plants. These differences in life-forms, growing environments (e.g. water conditions) and leaf habits of plants might also affect the relationship between leaf pH and nutrient concentrations.
In addition to leaf nutrient status, other structural and chemical traits were also closely related to leaf pH. The leaf pH of all plants increased significantly with increasing leaf TDS and practical salinity. This result suggested that the increasing soluble substances in leaves were more neutral or alkaline, resulting in a decrease in the leaf acidity. Compared with non-arid regions, arid regions showed higher leaf pH, TDS and practical salinity, which might be attributed to the increasing soluble alkaline cations in arid regions, especially the Na concentration, and ultimately led to higher leaf pH (Supplementary Table S5; Bacon et al. 1998; Cornelissen and Thompson 1997; Gollan et al. 1992; Makkonen et al. 2012). This pattern may also be closely related to the enhancement of water conservation, drought and salinity resistance capability of plants in arid regions (Xi et al. 2006; Zhao and Fan 2005). However, our results also showed that those single alkaline cations (Ca, Mg or Na) were not necessarily positively correlated with leaf pH (Fig. 1).
SLA was a key structural trait in the leaf economic spectrum, which was closely related to light acquisition efficiency and plant growth rate (Butler et al. 2017; Milla and Reich 2007; Wright et al. 2004). Our results about the relation between leaf pH and SLA (significantly negative) were opposite to those of Cornelissen et al. (2006) (significantly positive). Differences in climatic conditions might explain this inconsistent relationship between SLA and pH between our study and that of Cornelissen et al. (2006): the research of Cornelissen et al. (2006) was carried out in subarctic terrestrial ecosystems, while our study was conducted in temperate zones. In the subarctic zone, to resist harsh environments such as severe cold, relatively higher leaf pH was required (Felle et al. 2005), meanwhile, plants would also have a higher SLA under cold conditions (Poorter et al. 2009; Wright et al. 2004). This might result in a positive correlation between leaf pH and SLA in the subarctic zone. In addition, SLA that reflected the potential light acquisition ability per unit leaf mass was positively correlated with the relative growth rate (Reich et al. 1997), and the rapid leaf elongation of fast-growing plants was accompanied by the rapid elongation and acidification of the apoplast and cell walls (Barbez et al. 2017; Lin et al. 2021; Mühling and Läuchli 2000; Rayle and Cleland 1992), which might contribute to the lower leaf pH and higher SLA in fast-growing plants in temperate zones.
Divergent relationships between leaf pH and chemical and structural traits among different life-forms
Herbs and woody plants showed opposite relationships between leaf pH and leaf N, Ca or Mg concentration in non-arid regions (Fig. 3). Moreover, the relationships of leaf pH with leaf elemental concentrations in woody plants always had significantly higher absolute SMA regression slopes than those in herbs (Figs 1 and 3). This finding suggests that the leaf pH of woody plants relative to herbs is more susceptible to the variation in leaf elemental concentration. Relatively fast-growing herbs (r-strategist) allocate more substances to metabolize active ingredients, while relatively slow-growing perennial woody plants (K-strategist) distribute more substances for cell wall or storage (Lambers et al. 2008; Pianka 1970; Van Arendonk and Poorter 1994). The functional differences in nutrients between these two life-forms might be responsible for the above results.
Plants of the same life-form in different regions also showed inconsistent relationships between leaf pH and other traits. The response tendency of leaf pH against leaf N or Na concentrations in herbs was inconsistent in different regions (i.e. arid and non-arid regions). The above differences were also found in the relationship between leaf pH and leaf Ca concentration in woody plants (Figs 1 and 3).
Effect of environmental factors on the relationships between leaf pH and traits
The environmental factors were mainly manifested in their impact on slopepH-LP, slopepH-LCa, slopepH-LFe and slopepH-LMn, and mostly had no effect on the other slopes (Fig. 5). This phenomenon might be the reason for the different relationships of leaf pH with leaf P, Ca, Fe and Mn concentrations, and the consistent relationships of leaf pH with leaf TDS, practical salinity and C concentration of plants of the same life-form (but different regions, Figs 1 and 3). The different influence strengths might be related to the function, stability and plasticity of the trait, the response difference of multiple traits to the environment and the strength of associations between traits (Han et al. 2011; Hofhansl et al. 2021). In addition, the defects of our study might also lead to the inaccurate or insignificant effects of geographical and environmental factors on some leaf pH–trait relationships. For example, in SLA, there was too many missing data, and in N, there were many insignificant leaf pH–N relationships (no slope data were available). These limited data might result in a weak correlation between environmental factors and leaf pH–trait (SLA and N) relationships. This phenomenon might also lead to the inaccuracy of some results, and it is worth our follow-up study.
In addition, we also found that the slope (Fig. 5; e.g. slopepH-LMg) was even not affected by environmental factors, but diverged among life-forms. Similar to stoichiometric scaling relationships (Tian et al. 2017), the relationships of leaf pH with leaf chemical and structural traits were indeed inconsistent across different life-forms. Besides, the function consistency and the synergistic/antagonistic effects among multiple metal elements might also result in a difference in the pH–trait relationship that was not affected by the environment (Li 2001; Tang and Luan 2017). As we know, many kinds of cations, such as Mg2+, K+, Ca2+ and Na+, had commonality and interaction in absorption, storage and physiological function, and most of the cations were stored in vacuoles and played a role in charge compensation and osmotic regulation (Li 2001; Marschner 2012; Tränkner et al. 2018). Mg2+, Ca2+ and K+ were antagonistic to each other (Lenz et al. 2013; Tang and Luan 2017); the K+ and Ca2+ under water stress could enhance the tolerance of plants to water stress (Maathuis 2008; Shi et al. 2024); the synergistic absorption of K+ and Na+ was of great significance to the study of stomatal morphology and conductance (Guo et al. 2016). Therefore, the relationship (leaf pH and LCa) greatly affected by the environment was likely to cause the synergistic or antagonistic variation of other metal elements.
According to our study, the relationships between leaf pH and leaf chemical and structural traits across different plant life-forms and eco-geographic regions were inconsistent and dynamically changeable. Specifically, the relationships between leaf pH and leaf N, Ca and Na concentrations were inconsistent between herbs and woody plants in non-arid regions, but became consistent in arid regions. Leaf pH significantly increased with increasing TDS, practical salinity and decreasing SLA, but the relationship between leaf pH and LDMC was inconsistent across different regions. The difference in ecological strategies among life-forms, growing environments (e.g. water conditions) and leaf habits of plants might be the reason for the above results. Environmental factors were mainly manifested in the centralized impact on the relationships of leaf pH with leaf P, Ca, Fe and Mn concentrations. The different influence strength might be related to the function, stability and plasticity of the trait.
Although the world-wide leaf economics spectrum implies the convergent leaf trait relationships across different life-forms, biomes or habitats, climate conditions still regulate the relationship between leaf lifespan and leaf mass per area (the reciprocal of SLA) (Wright et al. 2004, 2005b). These findings together indicate that the coordinated relationships between leaf traits are susceptible to the diverse PFTs including life-forms and growing environments. The variation of plant functional traits mainly depends on plant PFTs and environmental factors (Reich et al. 1997; Wang et al. 2016), which also affect the scaling relationship between traits (Bruelheide et al. 2018; Yan et al. 2018). For more kinds of PFTs, their leaf pH–trait coordinated relationships, and the impacts of survival strategies and environmental changes on these relationships still remain poorly addressed and require further more explorations in detail. Our findings encourage researchers to explore deeply about the scaling relationships between plant traits.
Supplementary Material
Supplementary material is available at Journal of Plant Ecology online.
Figure S1: Distribution map of sample sites in arid and non-arid regions.
Table S1: The location, environmental conditions and the number of species of each sampling site in northern China.
Table S2: Significant regression slope (P < 0.05) of the standard major axis (SMA) regression models relating leaf pH to other plant functional traits for dicotyledons of each site.
Table S3: Soil physical and chemical properties of each sampling site in northern China.
Table S4: Regression slope and its 95% confidence interval of the standard major axis (SMA) regression models relating leaf pH to other plant functional traits for woody plants and herbs in arid and non-arid regions.
Table S5: Variation in leaf pH and other leaf chemical and structural traits across different PFTs (plant life-form) and eco-geographic regions in northern China.
Authors' Contributions
Wenxuan Han conceived this project. Sining Liu, Jiashu Chen and Junpeng Ren collected the plant samples in the field and conducted the chemical and statistical analyses of leaf pH, element concentrations and traits. Sining Liu, Wenxuan Han, Yan Luo, Zhengbing Yan and Yahan Chen wrote the manuscript.
Funding
This research was supported by the National Natural Science Foundation of China (32001165; 41473068), the Natural Science Foundation of Sichuan Province (2022NSFSC1753), the Strategic Priority Research Program of the Chinese Academy of Sciences (XDA26040202) and the Free Exploration Project of the Dual Support Plan for Disciplinary Development of Sichuan Agricultural University (2024ZYTS016).
Acknowledgements
We thank Dr M.X. Zhang, Dr S.Q. An, Dr F.Y. Ge, Dr X.Q. Lu and Miss J. Chen for their help in sample collection, and M.X. Zhang and F.Y. Ge for the sample measurement. And we thank Dr C.Y. Zheng and F.X. Zeng for providing us with some basic experimental facilities and reliable information of the sampling sites at Weichang, Hebei Province, China. We gratefully acknowledge the three anonymous referees and the associate editor, Prof. Helge Bruelheide, for their insightful comments on this manuscript.
Conflict of interest statement. The authors declare that they have no conflict of interest.