- Split View
-
Views
-
Cite
Cite
Savithry Namboodiripad, Daniel Lenzen, Ryan Lepic, Tessa Verhoef, Measuring conventionalization in the manual modality, Journal of Language Evolution, Volume 1, Issue 2, July 2016, Pages 109–118, https://doi.org/10.1093/jole/lzw005
- Share Icon Share
Abstract
Gestures produced by users of spoken languages differ from signs produced by users of sign languages in that gestures are more typically ad hoc and idiosyncratic, while signs are more typically conventionalized and shared within a language community. To measure how gestures may change over time as a result of the process of conventionalization, we used a social coordination game to elicit repeated silent gestures from hearing non-signers, and used Microsoft Kinect to unobtrusively track the movement of their bodies as they gestured. Our approach follows from a tradition of laboratory experiments designed to study language evolution and draws upon insights from sign language research on language emergence. Working with silent gesture, we were able to simulate and quantify hallmarks of conventionalization that have been described for sign languages, in the laboratory. With Kinect, we measured a reduction in the size of the articulatory space and a decrease in the distance traveled by the articulators, while communicative success increased between participants over time. This approach opens the door for more direct future comparisons between ad hoc gestures produced in the lab and natural sign languages in the world.
1. Background
Gestures produced by hearing non-signers and signs produced by signers of natural sign languages differ along many dimensions. However, gesture and sign have in common that both are ‘visible bodily actions’ that are standardly employed for the purposes of human communication (Kendon 2014). Among other things, this commonality has allowed sign linguists to look to gesture as a possible source for grammatical structures that are found in sign languages (e.g., Goldin-Meadow 1993; Senghas and Coppola 2001; Padden et al. 2013). The comparison between sign and gesture has also proven fruitful for gesture research, which has examined whether patterns that have been documented for sign languages can also be observed or elicited in gesture (e.g., Goldin-Meadow et al. 1996; Kita et al. 1998; Hall et al. 2013). Taken together, these complementary lines of research demonstrate that studies of non-sign gesture have the potential for direct comparison with studies of naturally emerging sign languages (e.g., Senghas et al. 2004; Sandler et al. 2005; Schouwstra et al. 2014). Despite these fruitful comparisons, however, it remains a challenge to quantify many aspects of the gestural signal. Accordingly, here we examine whether the processes that are known to change the forms of conventional lexical signs over time in a natural sign language can be quantified in communicative silent gestures elicited from hearing non-signers in a laboratory environment.
We focus here on two classes of diachronic reduction that have been documented for individual signs in American Sign Language (ASL): sign length and size of signing space (Frishberg 1975; Siple 1978; Klima and Bellugi 1979; see also Supalla and Clark 2015). For example, Frishberg (1975: 708–09) has demonstrated that over a span of approximately 55 years, between 1910 and 1965, certain signs in ASL reduced in form so as to become less transparent. Thus, while the ASL sign meaning ‘bird’ is recorded as a two-sign sequence in Long (1910), articulated first with the fingers on one hand opening and closing near the mouth and then with both hands flapping at the sides of the body, in Stokoe et al. (1965), ‘bird’ is recorded in a reduced form, with the second component of the earlier sign omitted completely. As a result, the 1965 version of the ASL sign meaning ‘bird’ is both shorter and smaller than its 1910 predecessor. Frishberg’s description of formal reduction in established ASL signs remains the state of the art, and no subsequent study has compared modern ASL signs with their 1965 counterparts. A potential barrier to progress in this domain is that, until relatively recently, it has been difficult to quantify general aspects of reduction in sign languages.
For the present study, we designed a communication game that encouraged hearing non-signers to create and use a shared set of referential gestures. Because this type of communication game has previously been shown to elicit the emergence of shared communicative systems (e.g., Garrod et al. 2007; Healey et al. 2007; Theisen et al. 2010; Caldwell and Smith 2012; Fay et al. 2013), we predicted this process would result in rapid conventionalization here as well. We also expected to see a reduction in the signal comparable to the reductive processes which have been observed diachronically for individual lexical signs in ASL and simplification of drawings observed in a laboratory setting (Caldwell and Smith 2012). Crucially, here we measured this reduction for the first time in gesture using body-tracking with the Microsoft Kinect, which allowed us to quantify the predicted change in the communicative signal in a novel way.
2. Methods
In order to bring about rapid conventionalization of ad hoc gestures, we designed a communication game in which participants took turns either giving gestural clues about (the Communicator) or guessing (the Guesser) items from a set of English nouns. Our approach follows a tradition that allows for the study of the role of social coordination and transmission in the emergence of linguistic structure (Scott-Phillips and Kirby 2010). We used a laboratory set-up modeled after previous studies that have observed rapid conventionalization of the signals that participants create. In these studies, participants typically communicate in dyads or small groups about a limited set of concepts, such that the influence from the language(s) they already speak is minimized. For instance, participants have been asked to communicate using drawings (Garrod et al. 2007; Healey et al. 2007; Theisen et al. 2010; Caldwell and Smith 2012), non-linguistic vocalizations (Perlman et al. 2015), and gestures (Fay et al. 2013; Schouwstra et al. 2014). In our communication game, the gestural signal was tracked with the Microsoft Kinect.
The Microsoft Kinect is a camera and body-tracking sensor system designed for video game play. In addition to recording RGB (red-green-blue) images and audio, the Kinect uses an infrared light projector to create a depth field in front of it. From this depth field, recognition algorithms locate human shapes and estimate locations of human joints in three dimensions. Following the setup and methods of Lenzen (2015) and Weibel et al. (2016), we captured Kinect-estimated positions of joints of participants in an experimental setting.
The Communicator was seated on a stool placed directly in front of a Microsoft Kinect for Windows v.1 sensor. The Kinect sensor was mounted on a tripod and placed on a raised surface at roughly the eye-level of a seated participant and 1.7 m from the front of the stool. The experiment room had covered windows and overhead fluorescent lighting so as not to disrupt the Kinect’s infrared field. Using ChronoSense software (Weibel et al. 2015) we recorded audio, RGB video frames, depth frames, and 10 upper-body joint estimations (∼30 samples per second) from the Kinect sensor.
A laptop was placed on the same surface as the Kinect sensor, slightly to the left of the sensor, and positioned so as to be visible only to the Communicator. Experimental items, to be described below, were displayed on the laptop screen. The Guesser sat on a stool to the left and slightly behind the laptop. The Guesser held a USB keyboard that was connected to the laptop, resting it on their own lap. This set-up allowed the Communicator and Guesser to face one another, with the laptop screen visible only to the Communicator, and with the Communicator’s movements clearly visible to both the Guesser and the Kinect sensor (Fig. 1).
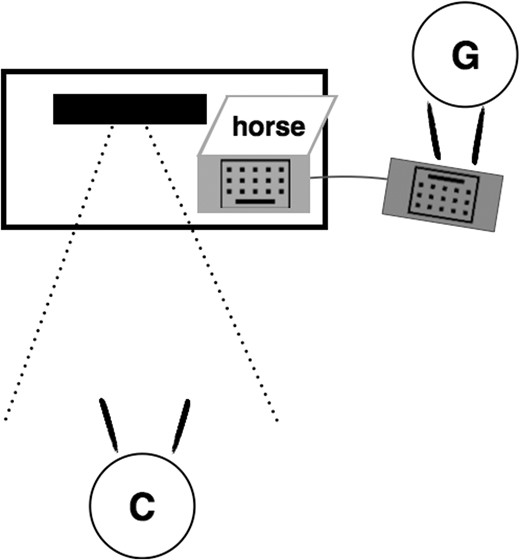
Experimental Set-up. The black solid box represents the Kinect sensor. The Communicator (C) is seated in the Kinect’s infrared field (represented by the dotted lines) and in full view of the Guesser (G), who controls the laptop without being able to see the stimulus on the screen.
The communication game consisted of four rounds, and participants switched roles halfway through each round. In the first round, both participants were allowed to use gesture and speech when taking on the role of the Communicator. This was done to facilitate guessing the correct item and to establish a common ground in the first round. Subsequent rounds were gesture-only. Other than these communicative constraints, the Communicator was allowed to give whatever clues they could think of (aside from the name of the item itself), and the Guesser was allowed to guess aloud as many times as needed. Once the Communicator confirmed that the Guesser had correctly named the item on the screen, the Guesser pressed the spacebar on the keyboard in front of them to advance to the next item.
Experimental items (Table 1) consisted of a set of thirty-two English words, eight each from four categories: Animals, Professions, Shapes, and Sports. All items appeared once per round, in a randomized order. Because each experimental item appeared every round, we anticipated that, as participants repeatedly referred to the same set of items, they would converge on efficient ways to communicate about items in the set. Moreover, we hypothesized that we would be able to track these changes automatically using the Kinect.
Animals . | Professions . | Shapes . | Sports . |
---|---|---|---|
bird | author | circle | archery |
cat | chauffeur | diamond | cycling |
elephant | dentist | octagon | golf |
giraffe | doctor | oval | gymnastics |
horse | drummer | spiral | karate |
monkey | painter | square | skiing |
raccoon | scientist | star | tennis |
walrus | waiter | triangle | weightlifting |
Animals . | Professions . | Shapes . | Sports . |
---|---|---|---|
bird | author | circle | archery |
cat | chauffeur | diamond | cycling |
elephant | dentist | octagon | golf |
giraffe | doctor | oval | gymnastics |
horse | drummer | spiral | karate |
monkey | painter | square | skiing |
raccoon | scientist | star | tennis |
walrus | waiter | triangle | weightlifting |
Animals . | Professions . | Shapes . | Sports . |
---|---|---|---|
bird | author | circle | archery |
cat | chauffeur | diamond | cycling |
elephant | dentist | octagon | golf |
giraffe | doctor | oval | gymnastics |
horse | drummer | spiral | karate |
monkey | painter | square | skiing |
raccoon | scientist | star | tennis |
walrus | waiter | triangle | weightlifting |
Animals . | Professions . | Shapes . | Sports . |
---|---|---|---|
bird | author | circle | archery |
cat | chauffeur | diamond | cycling |
elephant | dentist | octagon | golf |
giraffe | doctor | oval | gymnastics |
horse | drummer | spiral | karate |
monkey | painter | square | skiing |
raccoon | scientist | star | tennis |
walrus | waiter | triangle | weightlifting |
Eleven pairs of undergraduate students participated in the study, in exchange for course credit. In order to avoid the possibility that communicative success between pairs could result from knowledge of a sign language, and because we expect interaction to happen differently if participants are friends, participant pairs were excluded if they knew each other and/or had reported experience with a sign language. One pair was excluded for these reasons, so our analysis is based on the remaining 10 pairs.
For every experimental session, the ChronoSense software was started first for the Kinect, and then the experiment program (written in Processing) was started on the laptop. The experiment program displayed a written summary of the experiment instructions, and instructed the Communicator to tell the Guesser to press the spacebar once they were both ready to begin. A fixation cross was shown for one second before each item appeared. The experiment program also began with an identifiable sound, which was recorded by the Kinect and facilitated synchronization of the log file from the experiment program with the Kinect output file. The experiment program log file recorded an exact timestamp, in milliseconds, each time the spacebar was pressed to advance to the next item. These timestamps were used to automatically divide the data from the Kinect recordings into segments that corresponded with individual item trials.
The Kinect output was in the form of a CSV (comma-separated values) file with time-stamped XYZ depth estimations for ten regions of interest (forehead, sternum, and right and left hands, wrists, elbows, and shoulders), sampled at a rate of ∼30 estimations per second. This output file was imported to MATLAB and synchronized and segmented according to the measurements that were recorded in the experiment program output file.
3. Results
Because we are interested in changes in gesture forms over the course of the experiment, we report comparisons across Rounds 2–4, in which the Communicator was gesturing without speech about a set of items known to both participants. First, we report an oft-cited correlate of conventionalization, reduction of trial length, and then we move on to the results obtained from the Kinect measurements.
As expected, we observed a rapid increase in communicative success between participant pairs across rounds in this social coordination game; as participants became familiar with the items in the game and converged on a shared communicative system to refer to those items, they correctly guessed the items at faster rates. Across participant pairs, trial lengths (in seconds) started longer in Round 2 (M = 11.54 s), and became shorter in Round 3 (M = 5.65 s) and Round 4 (M = 4.39 s). A linear mixed-effects model (random effects = pair, item, participant) showed that trial length reduced by about 3.57 s (S.E.+/−0.37) each round. A model comparison showed that round significantly affected trial length (χ2 = 87.09, p < 0.0001). Figure 2 shows the length of each trial by round for each of our ten pairs, with a linear model fit and 95% confidence intervals for each pair.
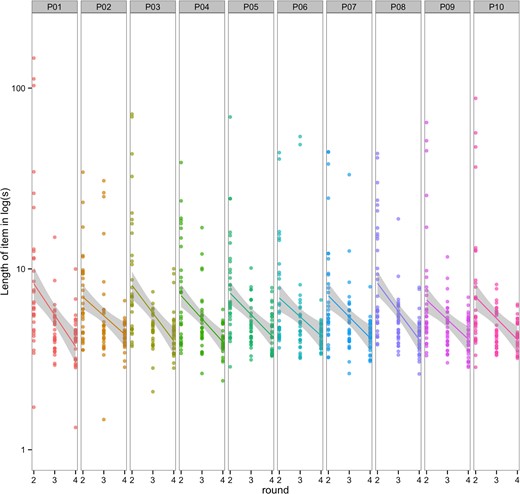
The length of item across rounds, by communication pair. The data is plotted on a log scale for clarity. Each dot represents the number of seconds taken for the Guesser to correctly name the item on the screen during a given item trial. The lines represent linear model fits, and the shaded area represents a 95% confidence interval.
Using Kinect as a diagnostic tool, we were able to track the movement of the bodies of participants in 3D space. In the analysis reported here, we only focus on the XYZ positions of the two wrists. The measurements reported below were computed based on sampled estimations for XYZ wrist location over the course of a given item trial. Accordingly, depth samples that were missing the right or left wrist estimations were excluded from further analyses (32220 of 343040 samples; 9.4 per cent). These missing samples result from the Kinect occasionally being unable to estimate locations due to issues such as occlusion of the target wrist, or occasionally being unable to distinguish separate parts of the body when they are located at similar depths. About 1280 item trials were recorded across all rounds for all participants, from which three were excluded due to no wrist data being recorded in the trial. Figure 3 shows one participant giving a gestural clue for the item ‘bird’, with the estimates of the different joints from the Kinect recording on the right.
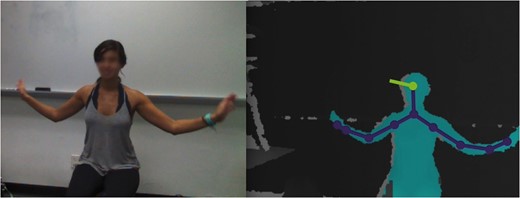
An RGB frame recorded by the Kinect (left), and a representation of joint locations in a human figure, taken from XYZ coordinates estimated by the Kinect (right).
Figure 4 is a visualization of this same participant gesturing the item ‘bird’ in Rounds 2, 3, and 4 (Figs 4a, b, and c, respectively). The gray ‘skeletons’ represent the average position of the participant’s body in that round, while gesturing that item. The skeletons are slightly different across rounds; this means that the participant’s body was moving slightly differently in each round. The red lines indicate the position of the wrist over the entire trial in which the participant was gesturing ‘bird’, and the orange squares represent the volume of the gesture space for each item trial, as viewed from the front. Impressionistically, the orange box in Fig. 4a appears larger than the orange boxes in Figs 4b and 4c, and in fact, across participants, gesture spaces started larger (in m3) in Round 2 (M = 0.15m3) and became smaller in Round 3 (M = 0.11m3) and Round 4 (M = 0.10m3).
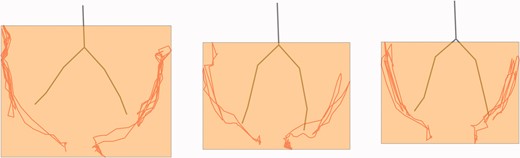
A front-view 2D image of ‘bird’ gestured by a single participant across all three gesture-only rounds. The gray skeleton represents the average position of the participant’s body in that round, while gesturing that item. The red lines indicate the position of the wrist over time in that item trial, and the orange squares represent a front-view of the volume of the gesture space for that item. (Colour online)
A linear mixed-effects model (random effects = pair, item, participant) showed that the volume of the gesture space reduced by about 0.03m3 (S.E.+/− 0.004) each round (Fig. 5), and a model comparison showed that the effect of round on gesture space was significant (χ2 = 54.01, p < 0.0001).
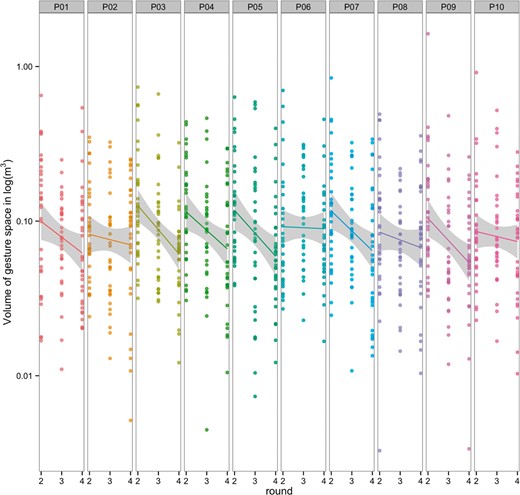
The volume of the gesture space across rounds, by communication pair. The data is plotted on a log scale for clarity. Each dot represents the distance (m3) traveled by both wrists of a single participant for a single item. The lines represent linear model fits, and the shaded area represents a 95% confidence interval.
Across participants, the total distance traveled by both hands added together (in m) also started longer in Round 2 (M = 11.58 m) and became shorter in Round 3 (M = 6.22 m) and Round 4 (M = 5.14 m). A linear mixed-effects model (random effects = pair, item, participant) showed that the distance that the hands traveled reduced by about 3.22 m (S.E.+/−0.36) each round (Fig. 6), and a model comparison showed that round had a significant effect on wrist travel distance (χ2 = 75.85, p < 0.0001). Trials in which the hands move a great deal (i.e., distances of approximately 100 m) result from the Communicator producing several gestures in order to get the Guesser to correctly guess the target word. This includes repetitions of the same gesture and elaborating with additional gestures, both of which also contributed to longer trial lengths (i.e., over 100 s).

The distance traveled by the hands (using the wrists as a proxy) across rounds, by communication pair. The data is plotted on a log scale for clarity. Each dot represents the meters traveled by both wrists by a single participant for a single item. The lines represent linear model fits, and the shaded area represents a 95% confidence interval.
The Kinect records joint locations in three dimensions, and so to illustrate what these measures look like in 3D, Fig. 7 shows the position of the two wrists, represented as traces in XYZ space, for a single participant in each of their Rounds 2 (7 a), 3 (7 b) and 4 (7 c). The traces in these visualizations take up less space in Round 3 and 4 as compared to Round 2, and there are fewer and shorter traces in the later rounds, showing the correlates of conventionalization captured by our Kinect measures.
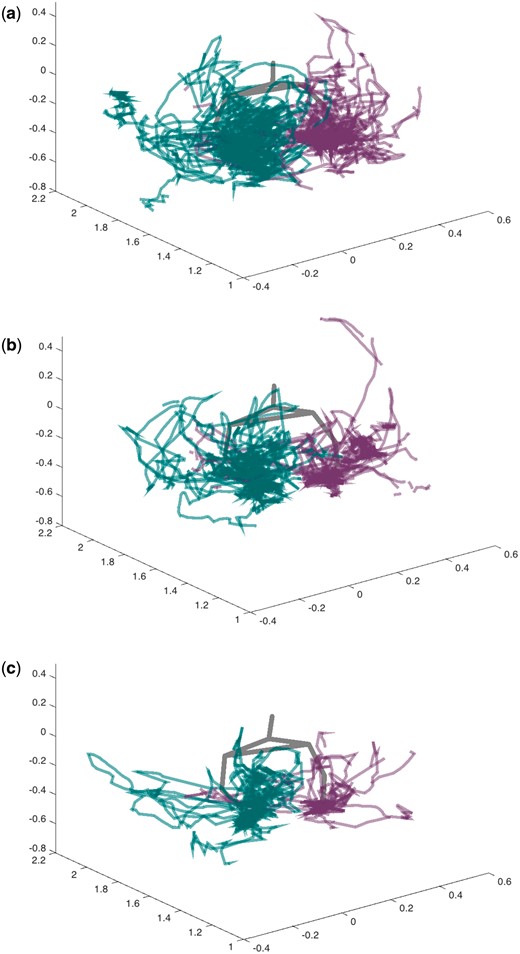
3D representation of the position of the two wrists in XYZ space as traces over the time span of a whole round for the same participant in (7 a) Round 2, (7 b) Round 3, and (7 c) Round 4.
4. Discussion
The language evolution literature has demonstrated that, in a laboratory setting, it is possible to create conditions under which participants will innovate and regularize novel communication systems. In these circumstances, the signals participants use seem to become less transparent, that is, more difficult to interpret for outside observers who have not been part of the development of the system, and less complex, exhibiting an overall reduction in form compared to previous tokens (e.g., Caldwell and Smith 2012). In these laboratory contexts, communication between partners also typically becomes more reliable, with faster interactions and improved accuracy, providing an overall sense of increased communicative efficiency. Given these findings, we anticipated our setup would lead to increased communicative success, and this prediction was confirmed. We measured a decrease in the amount of time it took for participants to correctly guess the experimental items over rounds, demonstrating that our participants converged on an ad hoc communicative system.
Additionally, previous research on formational reduction in sign language has demonstrated that individual lexical signs tend to become more arbitrary and less transparent, by virtue of the fact that they are conventional linguistic symbols in a language community (Frishberg 1975; Woodward 1976; Liddell and Johnson 1986), and as a function of their use in different social or grammatical contexts (e.g., Brentari and Poizner 1994; Tyrone and Mauk 2010; Russell et al. 2011). Specifically, the changes that affect the forms of conventional signs can be seen as resulting from the routinization of familiar articulatory targets, with diachronic variation typically reflecting the frozen product of previous synchronic variation (see Bybee 2007, 2010). Along these lines, we anticipated that the creation and use of a shared system during our experiment would be accompanied by changes in form, namely reduction, that are typically associated with naturally conventionalized signals. Our measures showed that the size of the communicative space and the distance traveled by the articulators decreased significantly over the rounds, a change which is directly comparable to described changes due to conventionalization in established languages.
In language evolution experiments, various modalities for signal production have been used. Among these are drawings (Garrod et al. 2007; Healey et al. 2007; Theisen et al. 2010; Caldwell and Smith 2012), non-speech vocalizations (Perlman et al. 2015), whistles (Verhoef 2012; Verhoef et al. 2014), and virtual one-dimensional sliders (Verhoef et al. 2015). The advantages common to all of these methods are that they (1) prevent participants from resorting to the language(s) that they already know, and (2) are easy to record and quantify at the level of the signal. However, some of these modalities are quite far removed from actual linguistic signals. Experiments using gesture share the first advantage since most hearing participants have no previous experience with sign language. As Schouwstra et al. (2014) point out, the additional advantage of studying changes in gesture over time in the laboratory is that it creates the opportunity to make direct comparisons with changes in real sign languages. But in order to get quantitative measures of gesture data, typically a lot of hand coding is required, and properties like volume of gesture space and distance traveled by separate joints are hard to code by hand. Here we have presented an alternative for obtaining quantitative results from a gesture experiment with the possibility of measuring factors that are relevant in processes of (sign) language evolution.
Using the Kinect to measure visible bodily actions elicited in the laboratory, we have demonstrated that it is possible to quantify new dependent measures, namely 3D movement paths and volume, that would otherwise be costly and impractical to measure with different tools (e.g., Mauk and Tyrone 2012; McNeill 1992: ch3). Additionally, relative to traditional gesture coding methods, we have found that the Kinect allows us to automatically digitize information about bodily movement. This is important because though it is known to be fundamental to sign language structure, movement has proven difficult to quantify previously.
The present study focuses primarily on movement. However, the types of questions we can answer using automatic body-tracking with the Kinect are not limited to the measures we report here. Here, we have analyzed only those data points that correspond to the left and right wrists. However, the wrists are only two out of ten areas of interest whose locations the Kinect can estimate. Because we are able to measure joints other than the wrist, future studies will be in a position to probe more fine-grained changes in movement over time. For example, we anticipate that distalization of movement (i.e., transfer of the main locus of movement to more distal joints) will be attested in conventionalized signals. Furthermore, we did not analyze the two hands as separate articulators, but given that sign languages most typically distinguish between the two hands as dominant and non-dominant articulators, a reasonable next line of inquiry is to examine whether there will be a shift toward a dominant hand as gesturers create a shared inventory of signals.
Though we have sought to demonstrate the advantages inherent to using the Kinect to automatically yet unobtrusively (as opposed to motion capture, cf. Lu and Huenerfauth 2010) record bodily movement, we are also aware that there are limits to the automatic quantification approach we have pursued here. For example, we are not in a position to measure handshapes or facial expressions, two linguistically relevant formational parameters that have been successfully quantified through more traditional hand-coding methods (e.g., Nespor and Sandler 1999; Emmorey et al. 2003). The automatic measurements demonstrated here will not supplant hand-coding, but rather supplement it. Although features like handshape are, for now, best coded by hand, we can integrate body-tracking measures from Kinect with these hand-coded parameters to provide a more nuanced characterization of the linguistic signal. We are confident that the integration of automatic measures with hand-coding will lead to more specific predictions about and theoretical accounts of dynamic patterns in the manual modality.
With Kinect we were able to measure changes in gesture that are also the hallmarks of conventionalization in sign language. This approach opens the door for more direct future comparisons between ad hoc gestures produced in the lab with natural sign languages in the world.
Acknowledgments
We wish to thank Carol Padden, Jim Hollan, and Jeremy Karnowski for support and guidance. We are grateful to Nick Gibson for assistance with pre-processing of the data. Thanks to Gary Lupyan and two anonymous reviewers for their valuable comments. This work was supported in part by the UC San Diego Chancellor’s Interdisciplinary Collaboratories Fellowship to DL, RL, and SN, and the Netherlands Organisation for Scientific Research (NWO) Rubicon grant to TV.
References