-
PDF
- Split View
-
Views
-
Cite
Cite
Thomas M Atkinson, Aleksandr Petrov, Kathleen A Lynch, Login S George, Jennifer R Cracchiolo, Bobby Daly, Kristen L Fessele, James H Flory, Jun J Mao, Yuelin Li, Using GPT-4o to interpret patient-reported outcomes without training, JNCI: Journal of the National Cancer Institute, Volume 117, Issue 4, April 2025, Pages 809–811, https://doi.org/10.1093/jnci/djaf016
- Share Icon Share
Advances in large language models (LLMs) such as OpenAI’s Generative Pre-trained Transformer-4o (GPT-4o) have prompted interest regarding their use in oncology. LLMs distill vast amounts of text data such as postings in online forums, scientific articles, and educational materials.1 As these methods evolve, there is potential for leveraging their capabilities in summarizing patient-authored free text data to patient-reported outcomes (PROs).2,3 While conventional PROs use standardized questions to broadly assess treatment- and disease-related domains in clinical trials and routine care,4 free text data are ideal for capturing patients’ nuanced experiences “in their own words”.5
However, before LLMs can be applied in coding PROs, we sought to develop, as a proof of concept, methodological procedures for unsupervised learning to automatically interpret patient-generated free text phrases without pre-training by human coders (ie, “zero-shot learning [ZSL]”), and demonstrate that LLM performance is similar to traditional human qualitative analysis.
We used archived interview transcripts (N = 19) from 9 patients, 2 caregivers, and 7 physicians (891 total participant utterances) from a study of cancer patients’ prognostic awareness that were previously coded6 by 2 humans into 5 themes (Figure 1). The GPT-4o model licensed to our institution is comprised of over 1 trillion parameters, pre-trained by OpenAI and provided in Microsoft Azure through a secure enterprise cloud-based offering. The ZSL procedure instructed GPT-4o to match transcript entries to these 5 themes de novo, relying solely on semantic contents. Prompt engineering experimented with optimal queries: (1) a “base” prompt included basic descriptions of each theme; (2) a “comprehensive” prompt had very detailed descriptions; and (3) “hybrid” prompts featured mixed descriptions. Performance was evaluated by precision, recall, F1-score, and Kappa reliability using human analysts as the ground truth. Additionally, duplicated utterances (112 with >90% similarity) assessed internal consistency in human analysts and GPT-4o.
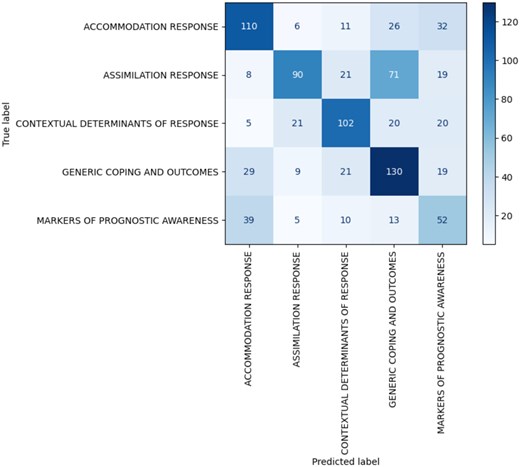
GPT-4o yielded acceptable performance in text classification using verbatim utterances alone; best performance was obtained with the “hybrid” prompt (56% precision, 54% recall, 55% F1-score, Κ = 0.43). The “comprehensive” and “base” prompts fared worse (57% precision, 54% recall, 54% F1-score, Κ = 0.42; 55% precision, 46% recall, 45% F1-score, Κ = 0.32, respectively). These metrics indicate better than chance classification (20%). However, longer prompts with detailed explanations and examples did not necessarily lead to the best performance. Encouragingly, internal consistency in GPT-4o nearly matched that of human coders (ie, Κ = 0.43 and 0.44).
As a necessary initial step for generalizing LLMs broadly to code PRO domains without pre-training, we have presented promising evidence that an unsupervised LLM can code patient-generated free text data. Ultimately, if a patient undergoing chemotherapy communicates with their doctor via a portal message and describes “I felt sick to my stomach…,” a further validated LLM may interpret this as signs of “mild nausea/vomiting” in real time and prompt clinicians for immediate action (eg, prescribing antiemetics) to minimize treatment-related adverse events and risk of chemotherapy interruptions. However, caution is advised, as current sub-optimal performance suggests that ZSL applications might be more suitable for initial screening, leaving uncertain classifications for human coders to detect nuanced meaning.
Acknowledgements
Role of the funder—support of investigator time and effort in relation to the design of the study, data collection, analysis, interpretation of the data, the writing of the correspondence, and the decision to submit the correspondence for publication.
Author contributions
Thomas Michael Atkinson, PhD (Conceptualization; Data curation; Formal analysis; Funding acquisition; Investigation; Methodology; Project administration; Resources; Software; Supervision; Validation; Visualization; Writing—original draft; Writing—review & editing), Aleksandr Petrov, MS (Conceptualization; Data curation; Formal analysis; Methodology; Project administration; Software; Validation; Visualization; Writing—original draft; Writing—review & editing), Kathleen A. Lynch, MPH (Conceptualization; Data curation; Formal analysis; Methodology; Validation; Writing—original draft; Writing—review & editing), Login S. George, PhD (Conceptualization; Data curation; Formal analysis; Investigation; Methodology; Project administration; Validation; Writing—original draft; Writing—review & editing), Jennifer R. Cracchiolo, MD (Conceptualization; Formal analysis; Investigation; Methodology; Validation; Writing—original draft; Writing—review & editing), Bobby Daly, MD (Conceptualization; Formal analysis; Investigation; Methodology; Validation; Writing—original draft; Writing—review & editing), Kristen L. Fessele, PhD, RN (Conceptualization; Formal analysis; Investigation; Methodology; Validation; Writing—original draft; Writing—review & editing), James H. Flory, MD (Conceptualization; Investigation; Methodology; Validation; Writing—original draft; Writing—review & editing), Jun J. Mao, MD (Conceptualization; Formal analysis; Investigation; Methodology; Validation; Writing—original draft; Writing—review & editing), Yuelin Li, PhD (Conceptualization; Data curation; Formal analysis; Funding acquisition; Investigation; Methodology; Project administration; Resources; Software; Supervision; Validation; Visualization; Writing—original draft; Writing—review & editing).
Funding
This research was funded in part through a National Institutes of Health/National Cancer Institute Cancer Center Support Grant P30 CA008748-58, which provides partial support for the Patient-Reported Outcomes, Community Engagement, and Language Core Facility used in this investigation. The content of this research is solely the responsibility of the authors and does not necessarily represent the official views of the National Cancer Institute or the National Institutes of Health.
Conflicts of interest
The authors have no conflicts of interest to disclose.
Data availability
De-identified data can be provided upon request to the corresponding author.