-
PDF
- Split View
-
Views
-
Cite
Cite
Elham Khodayari Moez, Matthew T Warkentin, Yonathan Brhane, Stephen Lam, John K Field, Geoffrey Liu, Javier J Zulueta, Karmele Valencia, Miguel Mesa-Guzman, Andrea Pasquier Nialet, Sukhinder Atkar-Khattra, Michael P A Davies, Benjamin Grant, Kiera Murison, Luis M Montuenga, Christopher I Amos, Hilary A Robbins, Mattias Johansson, Rayjean J Hung, Circulating proteome for pulmonary nodule malignancy, JNCI: Journal of the National Cancer Institute, Volume 115, Issue 9, September 2023, Pages 1060–1070, https://doi.org/10.1093/jnci/djad122
- Share Icon Share
Abstract
Although lung cancer screening with low-dose computed tomography is rolling out in many areas of the world, differentiating indeterminate pulmonary nodules remains a major challenge. We conducted one of the first systematic investigations of circulating protein markers to differentiate malignant from benign screen-detected pulmonary nodules.
Based on 4 international low-dose computed tomography screening studies, we assayed 1078 protein markers using prediagnostic blood samples from 1253 participants based on a nested case-control design. Protein markers were measured using proximity extension assays, and data were analyzed using multivariable logistic regression, random forest, and penalized regressions. Protein burden scores (PBSs) for overall nodule malignancy and imminent tumors were estimated.
We identified 36 potentially informative circulating protein markers differentiating malignant from benign nodules, representing a tightly connected biological network. Ten markers were found to be particularly relevant for imminent lung cancer diagnoses within 1 year. Increases in PBSs for overall nodule malignancy and imminent tumors by 1 standard deviation were associated with odds ratios of 2.29 (95% confidence interval: 1.95 to 2.72) and 2.81 (95% confidence interval: 2.27 to 3.54) for nodule malignancy overall and within 1 year of diagnosis, respectively. Both PBSs for overall nodule malignancy and for imminent tumors were substantially higher for those with malignant nodules than for those with benign nodules, even when limited to Lung Computed Tomography Screening Reporting and Data System (LungRADS) category 4 (P < .001).
Circulating protein markers can help differentiate malignant from benign pulmonary nodules. Validation with an independent computed tomographic screening study will be required before clinical implementation.
Lung cancer continues to represent a significant public health burden worldwide as the leading cause of global cancer death (1-3). Low-dose computed tomography (LDCT) screening in smokers was shown to reduce lung cancer–related mortality by 20% to 30% (4-6); as a result, the United States Preventive Services Task Force recommends annual LDCT screening for those aged 50 to 80 years with at least a 20-pack-year smoking history who either currently smoke or have quit within the last 15 years (7). Challenges of implementing LDCT at the population level remain, however. One of the main challenges is that pulmonary abnormalities can be found in approximately 20% of the screening participants, but only a small fraction of these nodules are malignant (6,8).
The Lung CT Screening Reporting and Data System (LungRADS), developed by the American College of Radiology, is commonly used in the US screening program for risk assessment (9), but it is largely based on nodule diameter and solidity without considering other parameters, and currently there is still a wide range of nodule management protocols (8,10,11). To differentiate malignant from benign noncalcified pulmonary nodules, several malignancy probability models have been proposed (12-15). For example, the Pan-Canadian Early Detection of Lung Cancer (PanCan) model (also referred to as the Brock model) combines demographic, clinical, and specific nodule information to assess the probability of nodule malignancy (15); the model has been incorporated into the British Thoracic Society guidelines (16). Although beneficial for reducing false positives, these nodule malignancy assessment tools are highly dependent on nodule sizes and have suboptimal predictive performance in small nodules (17).
Given the clinical importance of accurately classifying nodules based on their probability of malignancy to minimize unnecessary follow-up procedures, we launched a systematic investigation of whether circulating proteins can help differentiate benign from malignant nodules in conjunction with the nodule features and an individual’s medical history. Circulating protein markers can be ideal biomarkers because they have been shown to predict cancer occurrence in prospective studies and can be obtained using a minimally invasive approach (18,19). While there have been a plethora of biomarker studies on lung cancer risk, the data on pulmonary nodule malignancy are much more sparse and often limited to either a few targeted markers or a single study (20). This study is the first to apply an extensive circulating proteomic approach using prediagnostic samples based on an international collaboration of 4 LDCT studies. Our goal was to identify circulating protein markers that can help differentiate malignant from benign pulmonary nodules beyond what current clinical classification systems such as LungRADS can achieve.
Methods
Study design and participants
As part of the Integrative Analysis of Lung Cancer Risk and Etiology (INTEGRAL) research program, this study was conducted based on 4 LDCT lung cancer screening programs in Canada, Spain, the United States, and the United Kingdom using a nested case-control design, including the PanCan, the UK Lung Screening trial (UKLS), the Toronto International Early Lung Cancer Action Program (IELCAP-Toronto), and the Pamplona International Early Lung Cancer Action Program (P-IELCAP) (21). Detailed designs for these studies have previously been reported (22-27). In brief, varying by individual studies, these studies were conducted between 2001 to 2020 among smokers aged 40 to 84 years, and the participants were followed for up to 17 years after enrollment. Participants completed a study questionnaire for health history at baseline. Blood samples were collected at baseline and at some of the follow-up screening rounds, depending on the study design (Supplementary Methods, available online).
A total of 425 patients with lung cancer and prediagnostic blood samples collected within 5 years of diagnosis and 430 frequency-matched controls (on age, sex, and follow time) with benign pulmonary nodules were included in this analysis. In addition, we selected 398 healthy controls without any nodules, frequency matched with cases on age of enrollment, sex, and follow-up time to provide information for background protein expression distribution in the study source population. Written informed consent was obtained from all participants. The ethics approvals were obtained by each local institute and the Mount Sinai Hospital Research Ethics Board.
Protein biomarkers
The circulating proteome in prediagnostic plasma samples was quantified using the proximity extension assay (PEA); the details of this assay have been reported previously (28). In brief, the proximity extension assay is an immunoassay based on pairs of oligonucleotide-coupled antibodies, and the abundance of target proteins is quantified by real-time quantitative polymerase chain reaction (29). A total of 1105 PEA assays representing 1078 unique protein markers were measured, for a total of 1253 participants (Supplementary Table 1, available online).
The relative abundance of protein levels was measured as normalized protein expression values, calculated from the inverse amount of target nucleic acid based on the cycle threshold values and expressed in the log2 scale. The normalized protein expression values that did not pass quality control (described in the Supplementary Methods, available online) were excluded from the analysis.
Statistical analysis
The overview of our analytical pipeline is provided in Figure 1, and details are in the Supplementary Methods (available online). In brief, to assess the association between each protein marker and nodule malignancy while accounting for nodule characteristics, we applied multivariable logistic regression, adjusting for the PanCan/Brock score, which was computed based on demographics, family history of lung cancer, history of emphysema, and nodule characteristics, as previously described (15).
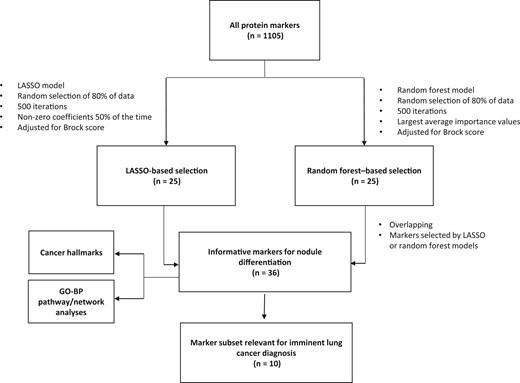
The overall analytical pipeline, by number of markers. GO-BP = Gene Ontology–Biological Process; LASSO = least absolute shrinkage and selection operator.
To select the top markers that can potentially inform nodule malignancy, 2 analytical methods—penalized regression (least absolute shrinkage and selection operator [LASSO]) and random forest—were performed in parallel (30,31). Using LASSO, the markers with non-zero coefficients in at least 50% of the random resamples were considered potentially informative. Twenty-five markers were selected using this approach. Using random forest, the markers were ranked by their importance values, which reflects their relevance in classifying cases vs controls (32). We selected the top 25 markers based on the importance values from random forest analysis to allow both analytical methods to be considered equally. With 14 markers selected by both LASSO and random forest, a total of 36 markers were considered informative for nodule malignancy.
To identify protein markers that were most informative for imminent lung cancer (defined as a diagnosis within 1 year of blood collection) and to assess whether the protein markers remain informative with longer lead time, we conducted stratified analysis by the time to diagnosis as 1 year or less, 1 to 3 years, and more than 3 years.
Pathway enrichment and network analysis
To assess whether any biological pathways were enriched in this selected set of 36 informative protein markers, we conducted pathway enrichment analyses using Gene Ontology–Biological Process pathway based on the hypergeometric test and assessed with the false discovery rate (FDR)–adjusted P values (details in the Supplementary Methods, available online) (33-35). A similar approach was used to assess cancer hallmarks (36).
Protein burden score
To aggregate the expression levels of the top protein markers, we constructed 2 protein burden scores (PBSs) based on a linear combination of the protein expression level weighted by their estimated coefficients, after adjustment for the PanCan/Brock score. The overall PBS (PBS-overall) was based on the expression data of all 36 informative markers. The imminent PBS (PBS-imminent) was estimated specifically based on the 10 protein markers that were most relevant for imminent lung cancer.
Sensitivity analysis
As a sensitivity analysis, we applied the incident case sampling design and for each case randomly selected up to 4 controls, matched based on the sex, age, and the screening cohort, whose members were not diagnosed with lung cancer at the time of diagnosis for each case (including those who developed lung cancers later). We then fitted conditional logistic regression to account for the matching structure of the data (37).
Results
The key characteristics of CT screening participants by study are summarized in Table 1. Compared with those with benign nodules (controls), the participants with malignant nodules (cases) had a higher proportion of current smokers and were more likely to have a history of emphysema or chronic obstructive pulmonary disease. As expected, the malignant nodules were, on average, larger than benign lesions.
Key characteristics of the study population, by nodule malignancy status and LDCT screening cohortsa
. | Participants with lung cancer . | Participants with benign nodules . | . | ||||||||
---|---|---|---|---|---|---|---|---|---|---|---|
. | IELCAP-Toronto . | PanCan . | P-IELCAP . | UKLS . | Total . | IELCAP-Toronto . | PanCan . | P-IELCAP . | UKLS . | Total . | Total . |
Total, No. | 79 | 169 | 76 | 101 | 425 | 87 | 169 | 82 | 92 | 430 | 855 |
Age at enrollment, mean (SD), y | 62.7 (7) | 63.4 (6) | 63.8 (89) | 68.6 (4) | 64.6 (7) | 64.5 (7) | 64.3 (6) | 62.7 (9) | 68.5 (4) | 64.9 (7) | 64.8 (7) |
Sex, No. (%) | |||||||||||
Female | 56 (71) | 86 (51) | 13 (17) | 21 (21) | 176 (41) | 62 (71) | 87 (51) | 16 (20) | 22 (24) | 187 (43) | 363 (42) |
Male | 23 (29) | 83 (49) | 63 (83) | 80 (79) | 249 (59) | 25 (29) | 82 (49) | 66 (80) | 70 (76) | 243 (57) | 492 (58) |
Smoking status, No. (%) | |||||||||||
Current | 49 (62) | 109 (64) | 47 (62) | 60 (59) | 265 (62) | 30 (34) | 98 (58) | 44 (54) | 51 (55) | 223 (52) | 488 (57) |
Former | 30 (38) | 60 (36) | 29 (38) | 41 (41) | 160 (38) | 57 (66) | 71 (42) | 38 (46) | 41 (45) | 207 (48) | 367 (43) |
Nodule size, median (IQR), mm | 12.0 (11) | 11.0 (10) | 10.0 (6) | 18.5 (13) | 12.0 (11) | 7.0 (2) | 4.9 (4) | 7.0 (12) | 6.9 (4) | 6.1 (4) | 8 (7) |
Emphysema/COPD, No. (%) | 16 (20) | 36 (21) | 62 (82) | 31 (31) | 145 (34) | 14 (16) | 31 (18) | 46 (56) | 20 (22) | 111 (26) | 256 (30) |
Family history of lung cancer, No. (%) | 13 (16) | 64 (38) | — | 23 (23) | 100 (23) | 15 (17) | 49 (29) | — | 22 (24) | 86 (20) | 186 (22) |
Histology, No. (%) | |||||||||||
Adenocarcinoma | 46 (58) | 97 (57) | 36 (47) | 48 (47) | 227 (53) | — | — | — | — | — | — |
Small cell | 2 (2) | 11 (6) | 5 (7) | 10 (10) | 28 (7) | — | — | — | — | — | — |
Squamous cell | 7 (9) | 21 (12) | 15 (20) | 26 (26) | 69 (16) | — | — | — | — | — | — |
Stage, No. (%) | |||||||||||
Stage I | 59 (81) | 109 (70) | 57 (78) | 49 (50) | 274 (68) | — | — | — | — | — | — |
Stage II | 5 (7) | 9 (6) | 3 (4) | 9 (9) | 26 (6) | — | — | — | — | — | — |
Stage III | 4 (5) | 21 (13) | 7 (10) | 14 (14) | 46 (11) | — | — | — | — | — | — |
Stage IV | 5 (7) | 17 (11) | 6 (8) | 26 (27) | 54 (13) | — | — | — | — | — | — |
Time to diagnosis, No. (%), y | |||||||||||
≤1 | 36 (46) | 65 (39) | 36 (47) | 49 (49) | 186 (44) | — | — | — | — | — | — |
1-3 | 29 (37) | 79 (47) | 23 (30) | 27 (27) | 158 (37) | — | — | — | — | — | — |
>3 | 14 (18) | 25 (15) | 17 (22) | 25 (25) | 81 (19) | — | — | — | — | — | — |
. | Participants with lung cancer . | Participants with benign nodules . | . | ||||||||
---|---|---|---|---|---|---|---|---|---|---|---|
. | IELCAP-Toronto . | PanCan . | P-IELCAP . | UKLS . | Total . | IELCAP-Toronto . | PanCan . | P-IELCAP . | UKLS . | Total . | Total . |
Total, No. | 79 | 169 | 76 | 101 | 425 | 87 | 169 | 82 | 92 | 430 | 855 |
Age at enrollment, mean (SD), y | 62.7 (7) | 63.4 (6) | 63.8 (89) | 68.6 (4) | 64.6 (7) | 64.5 (7) | 64.3 (6) | 62.7 (9) | 68.5 (4) | 64.9 (7) | 64.8 (7) |
Sex, No. (%) | |||||||||||
Female | 56 (71) | 86 (51) | 13 (17) | 21 (21) | 176 (41) | 62 (71) | 87 (51) | 16 (20) | 22 (24) | 187 (43) | 363 (42) |
Male | 23 (29) | 83 (49) | 63 (83) | 80 (79) | 249 (59) | 25 (29) | 82 (49) | 66 (80) | 70 (76) | 243 (57) | 492 (58) |
Smoking status, No. (%) | |||||||||||
Current | 49 (62) | 109 (64) | 47 (62) | 60 (59) | 265 (62) | 30 (34) | 98 (58) | 44 (54) | 51 (55) | 223 (52) | 488 (57) |
Former | 30 (38) | 60 (36) | 29 (38) | 41 (41) | 160 (38) | 57 (66) | 71 (42) | 38 (46) | 41 (45) | 207 (48) | 367 (43) |
Nodule size, median (IQR), mm | 12.0 (11) | 11.0 (10) | 10.0 (6) | 18.5 (13) | 12.0 (11) | 7.0 (2) | 4.9 (4) | 7.0 (12) | 6.9 (4) | 6.1 (4) | 8 (7) |
Emphysema/COPD, No. (%) | 16 (20) | 36 (21) | 62 (82) | 31 (31) | 145 (34) | 14 (16) | 31 (18) | 46 (56) | 20 (22) | 111 (26) | 256 (30) |
Family history of lung cancer, No. (%) | 13 (16) | 64 (38) | — | 23 (23) | 100 (23) | 15 (17) | 49 (29) | — | 22 (24) | 86 (20) | 186 (22) |
Histology, No. (%) | |||||||||||
Adenocarcinoma | 46 (58) | 97 (57) | 36 (47) | 48 (47) | 227 (53) | — | — | — | — | — | — |
Small cell | 2 (2) | 11 (6) | 5 (7) | 10 (10) | 28 (7) | — | — | — | — | — | — |
Squamous cell | 7 (9) | 21 (12) | 15 (20) | 26 (26) | 69 (16) | — | — | — | — | — | — |
Stage, No. (%) | |||||||||||
Stage I | 59 (81) | 109 (70) | 57 (78) | 49 (50) | 274 (68) | — | — | — | — | — | — |
Stage II | 5 (7) | 9 (6) | 3 (4) | 9 (9) | 26 (6) | — | — | — | — | — | — |
Stage III | 4 (5) | 21 (13) | 7 (10) | 14 (14) | 46 (11) | — | — | — | — | — | — |
Stage IV | 5 (7) | 17 (11) | 6 (8) | 26 (27) | 54 (13) | — | — | — | — | — | — |
Time to diagnosis, No. (%), y | |||||||||||
≤1 | 36 (46) | 65 (39) | 36 (47) | 49 (49) | 186 (44) | — | — | — | — | — | — |
1-3 | 29 (37) | 79 (47) | 23 (30) | 27 (27) | 158 (37) | — | — | — | — | — | — |
>3 | 14 (18) | 25 (15) | 17 (22) | 25 (25) | 81 (19) | — | — | — | — | — | — |
LDCT = low-dose computed tomography; COPD = chronic obstructive pulmonary disease; IELCAP-Toronto = Toronto International Early Lung Cancer Action Program; PanCan = Pan-Canadian Early Detection of Lung Cancer; P-IELCAP = Pamplona International Early Lung Cancer Action Program; UKLS = UK Lung Screening.
Key characteristics of the study population, by nodule malignancy status and LDCT screening cohortsa
. | Participants with lung cancer . | Participants with benign nodules . | . | ||||||||
---|---|---|---|---|---|---|---|---|---|---|---|
. | IELCAP-Toronto . | PanCan . | P-IELCAP . | UKLS . | Total . | IELCAP-Toronto . | PanCan . | P-IELCAP . | UKLS . | Total . | Total . |
Total, No. | 79 | 169 | 76 | 101 | 425 | 87 | 169 | 82 | 92 | 430 | 855 |
Age at enrollment, mean (SD), y | 62.7 (7) | 63.4 (6) | 63.8 (89) | 68.6 (4) | 64.6 (7) | 64.5 (7) | 64.3 (6) | 62.7 (9) | 68.5 (4) | 64.9 (7) | 64.8 (7) |
Sex, No. (%) | |||||||||||
Female | 56 (71) | 86 (51) | 13 (17) | 21 (21) | 176 (41) | 62 (71) | 87 (51) | 16 (20) | 22 (24) | 187 (43) | 363 (42) |
Male | 23 (29) | 83 (49) | 63 (83) | 80 (79) | 249 (59) | 25 (29) | 82 (49) | 66 (80) | 70 (76) | 243 (57) | 492 (58) |
Smoking status, No. (%) | |||||||||||
Current | 49 (62) | 109 (64) | 47 (62) | 60 (59) | 265 (62) | 30 (34) | 98 (58) | 44 (54) | 51 (55) | 223 (52) | 488 (57) |
Former | 30 (38) | 60 (36) | 29 (38) | 41 (41) | 160 (38) | 57 (66) | 71 (42) | 38 (46) | 41 (45) | 207 (48) | 367 (43) |
Nodule size, median (IQR), mm | 12.0 (11) | 11.0 (10) | 10.0 (6) | 18.5 (13) | 12.0 (11) | 7.0 (2) | 4.9 (4) | 7.0 (12) | 6.9 (4) | 6.1 (4) | 8 (7) |
Emphysema/COPD, No. (%) | 16 (20) | 36 (21) | 62 (82) | 31 (31) | 145 (34) | 14 (16) | 31 (18) | 46 (56) | 20 (22) | 111 (26) | 256 (30) |
Family history of lung cancer, No. (%) | 13 (16) | 64 (38) | — | 23 (23) | 100 (23) | 15 (17) | 49 (29) | — | 22 (24) | 86 (20) | 186 (22) |
Histology, No. (%) | |||||||||||
Adenocarcinoma | 46 (58) | 97 (57) | 36 (47) | 48 (47) | 227 (53) | — | — | — | — | — | — |
Small cell | 2 (2) | 11 (6) | 5 (7) | 10 (10) | 28 (7) | — | — | — | — | — | — |
Squamous cell | 7 (9) | 21 (12) | 15 (20) | 26 (26) | 69 (16) | — | — | — | — | — | — |
Stage, No. (%) | |||||||||||
Stage I | 59 (81) | 109 (70) | 57 (78) | 49 (50) | 274 (68) | — | — | — | — | — | — |
Stage II | 5 (7) | 9 (6) | 3 (4) | 9 (9) | 26 (6) | — | — | — | — | — | — |
Stage III | 4 (5) | 21 (13) | 7 (10) | 14 (14) | 46 (11) | — | — | — | — | — | — |
Stage IV | 5 (7) | 17 (11) | 6 (8) | 26 (27) | 54 (13) | — | — | — | — | — | — |
Time to diagnosis, No. (%), y | |||||||||||
≤1 | 36 (46) | 65 (39) | 36 (47) | 49 (49) | 186 (44) | — | — | — | — | — | — |
1-3 | 29 (37) | 79 (47) | 23 (30) | 27 (27) | 158 (37) | — | — | — | — | — | — |
>3 | 14 (18) | 25 (15) | 17 (22) | 25 (25) | 81 (19) | — | — | — | — | — | — |
. | Participants with lung cancer . | Participants with benign nodules . | . | ||||||||
---|---|---|---|---|---|---|---|---|---|---|---|
. | IELCAP-Toronto . | PanCan . | P-IELCAP . | UKLS . | Total . | IELCAP-Toronto . | PanCan . | P-IELCAP . | UKLS . | Total . | Total . |
Total, No. | 79 | 169 | 76 | 101 | 425 | 87 | 169 | 82 | 92 | 430 | 855 |
Age at enrollment, mean (SD), y | 62.7 (7) | 63.4 (6) | 63.8 (89) | 68.6 (4) | 64.6 (7) | 64.5 (7) | 64.3 (6) | 62.7 (9) | 68.5 (4) | 64.9 (7) | 64.8 (7) |
Sex, No. (%) | |||||||||||
Female | 56 (71) | 86 (51) | 13 (17) | 21 (21) | 176 (41) | 62 (71) | 87 (51) | 16 (20) | 22 (24) | 187 (43) | 363 (42) |
Male | 23 (29) | 83 (49) | 63 (83) | 80 (79) | 249 (59) | 25 (29) | 82 (49) | 66 (80) | 70 (76) | 243 (57) | 492 (58) |
Smoking status, No. (%) | |||||||||||
Current | 49 (62) | 109 (64) | 47 (62) | 60 (59) | 265 (62) | 30 (34) | 98 (58) | 44 (54) | 51 (55) | 223 (52) | 488 (57) |
Former | 30 (38) | 60 (36) | 29 (38) | 41 (41) | 160 (38) | 57 (66) | 71 (42) | 38 (46) | 41 (45) | 207 (48) | 367 (43) |
Nodule size, median (IQR), mm | 12.0 (11) | 11.0 (10) | 10.0 (6) | 18.5 (13) | 12.0 (11) | 7.0 (2) | 4.9 (4) | 7.0 (12) | 6.9 (4) | 6.1 (4) | 8 (7) |
Emphysema/COPD, No. (%) | 16 (20) | 36 (21) | 62 (82) | 31 (31) | 145 (34) | 14 (16) | 31 (18) | 46 (56) | 20 (22) | 111 (26) | 256 (30) |
Family history of lung cancer, No. (%) | 13 (16) | 64 (38) | — | 23 (23) | 100 (23) | 15 (17) | 49 (29) | — | 22 (24) | 86 (20) | 186 (22) |
Histology, No. (%) | |||||||||||
Adenocarcinoma | 46 (58) | 97 (57) | 36 (47) | 48 (47) | 227 (53) | — | — | — | — | — | — |
Small cell | 2 (2) | 11 (6) | 5 (7) | 10 (10) | 28 (7) | — | — | — | — | — | — |
Squamous cell | 7 (9) | 21 (12) | 15 (20) | 26 (26) | 69 (16) | — | — | — | — | — | — |
Stage, No. (%) | |||||||||||
Stage I | 59 (81) | 109 (70) | 57 (78) | 49 (50) | 274 (68) | — | — | — | — | — | — |
Stage II | 5 (7) | 9 (6) | 3 (4) | 9 (9) | 26 (6) | — | — | — | — | — | — |
Stage III | 4 (5) | 21 (13) | 7 (10) | 14 (14) | 46 (11) | — | — | — | — | — | — |
Stage IV | 5 (7) | 17 (11) | 6 (8) | 26 (27) | 54 (13) | — | — | — | — | — | — |
Time to diagnosis, No. (%), y | |||||||||||
≤1 | 36 (46) | 65 (39) | 36 (47) | 49 (49) | 186 (44) | — | — | — | — | — | — |
1-3 | 29 (37) | 79 (47) | 23 (30) | 27 (27) | 158 (37) | — | — | — | — | — | — |
>3 | 14 (18) | 25 (15) | 17 (22) | 25 (25) | 81 (19) | — | — | — | — | — | — |
LDCT = low-dose computed tomography; COPD = chronic obstructive pulmonary disease; IELCAP-Toronto = Toronto International Early Lung Cancer Action Program; PanCan = Pan-Canadian Early Detection of Lung Cancer; P-IELCAP = Pamplona International Early Lung Cancer Action Program; UKLS = UK Lung Screening.
The association of single protein markers
The single-marker results of all 1078 circulating protein markers are shown in Figure 2. The odds ratios (ORs) of the individual markers ranged from 0.75 to 1.49 after adjusting for the PanCan/Brock score. The most statistically significant protein markers were WAP 4-disulfide core domain 2 (WFDC2) (OR = 1.49; 95% confidence interval [CI]: 1.26 to 1.76; P < .001), tumor necrosis factor–related apoptosis-inducing ligand-receptor 2 (TRAIL-R2) (OR = 1.46; 95% CI: 1.22 to 1.76; P < .001), and C-X-C motif chemokine ligand 17 (CXCL17) (OR = 1.42; 95% CI: 1.19 to 1.70; P < .001). Some of the markers showed an inverse association with nodule malignancy—for example, fas ligand (FASLG) (OR = 0.77; 95% CI: 0.66 to 0.90; P < .001). In addition, keratin 19 (KRT19), matrix metalloproteinases 12 (MMP12), and carcinoembryogenic antigen cell adhesion molecule 5 (CEACAM5) associated with lung cancer diagnosed within 1 year of lead time (OR = 1.84; 95% CI: 1.43 to 2.38; P < .001; OR = 1.60; 95% CI: 1.27 to 2.04; P < .001; and OR = 1.43; 95% CI: 1.13 to 1.83; P = .002, respectively) (Supplementary Figure 1, available online).
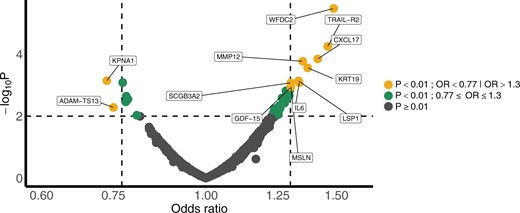
Volcano plot for single-marker analyses of nodule malignancy. The results of individual markers based on multivariable logistic regression adjusted for Brock/PanCan score. OR = odds ratio.
Biological relevance of informative protein markers
Based on LASSO and random forest analysis (Figure 1), we identified a total of 36 protein markers that were deemed informative of pulmonary nodule malignancy. Supplementary Table 1 (available online) provides the full list of the 36 informative markers and the subset of 10 markers, including MMP12, KRT19, S100 calcium binding protein A12 (a.k.a. EN-RAGE), CEACAM5, FASLG, integrin subunit alpha V (ITGAV), nicotinamide phosphoribosyltransferase (NAMPT), secretagogin (SCGN), thyroid stimulating hormone subunit beta (TSHB), and progestagen associated endometrial protein (PAEP), that are most relevant for imminent lung cancer diagnosis within 1 year. The biological pathways and network represented by these 36 potentially informative markers are shown in Figure 3. Ten pathways were statistically enriched with an FDR less than 0.01 (Figure 3, A). The most statistically significant pathway is response to external stimulus (FDR <0.001), and the representation of this pathway is approximately 7 times higher than expected by chance compared with other pathways. Other pathways that had enriched representation included cell death, immune response, and immune system development. The network analysis indicated that these 10 pathways are highly interconnected (Figure 3, B).

The results of Gene Ontology–Biological Process pathway enrichment analysis and network analysis based on the 36 informative markers. (A) The bar plot of the enriched pathway with statistical significance (FDR < 0.01) ordered by FDR levels, with fold enrichment value and pathway size (ie, number of genes) annotated. (B) The network of the significantly enriched pathways (FDR < 0.01), depicting the pathway size (circle), the significance level (gradient), and the connections between pathways (line types). FDR = false discovery rate.
Supplementary Figure 2 (available online) summarizes the allocations of our top protein markers to different cancer hallmarks (36). Cancer hallmarks include the instrumental milestones for a normal cell to become malignant and survive, proliferate, or spread. Fifteen of the 36 informative circulating protein markers were included in the cancer hallmarks previously described. Among those, FASLG and interleukin 6 (IL-6) are involved in more than half of those cancer hallmarks. The hallmarks that are most relevant for these circulating protein markers are inducing angiogenesis, resisting cell death, and tumor-promoting inflammation (Supplementary Figure 2, available online).
Protein burden scores
The PBS-overall was higher for the participants with malignant nodules (P < .001) (Supplementary Figure 3, A, available online). An increase in PBS-overall by 1 SD corresponded with an increased risk of nodule malignancy (OR = 2.29; 95% CI: 1.95 to 2.72) (Figure 4, A). Similarly, PBS-imminent was higher among malignant nodules detected within 1 year after blood collection (P < .001) (Supplementary Figure 3, B, available online). The risk of nodule malignancy within 1 year after blood collection was estimated to be 2.8 times higher per SD increase in PBS-imminent (Figure 4, B). The association between PBS (overall and imminent, per SD increase) and lung cancer risk among current smokers seems to be stronger than in former smokers (Figure 4). When stratified by LungRADS category, we observed that both the PBS-overall and PBS-imminent well differentiated benign and malignant nodules within the LungRADS category (≤3, 4), indicating the additional value of protein expression profiles beyond the clinical nodule classification system (Figures 4 and 5), where individuals with benign nodules consistently have lower PBSs than those with malignant nodules.
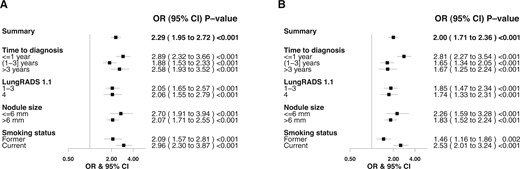
Forest plots of protein burden scores illustrating the association with nodule malignancy, stratified by time to diagnosis; LungRADS, version 1.1, categories; nodule sizes; and smoking status. The odds ratios and 95% confidence intervals per 1 SD increase are presented. (A) PBS-overall: PBSs are calculated based on the top 36 markers associated with nodule malignancy. (B) PBS-imminent: PBSs are calculated based on the top 10 markers associated with lung cancer diagnosis within 1 year after blood sample collection. CI = confidence interval; LungRADS = Lung Computed Tomography Reporting & Data System; OR = odds ratio; PBS-imminent = protein burden scores for imminent tumors; PBS-overall = protein burden scores for overall nodule malignancy.
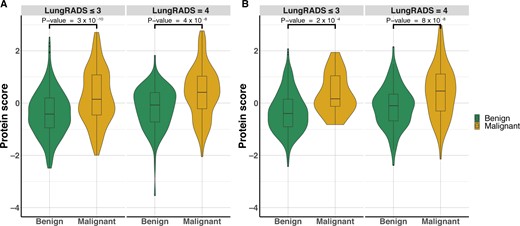
The differential expression of the top protein markers quantified by PBS in patients with benign and malignant nodules in LungRADS, version 1.1, category 4 vs other categories. (A) PBS-overall calculated based on the top 36 informative protein markers. (B) PBS-imminent calculated for the 10 markers relevant for lung cancer diagnosis within 1 year after blood collection. LungRADS = Lung Computed Tomography Reporting & Data System; PBS = protein burden score; PBS-imminent = protein burden scores for imminent tumors; PBS-overall = protein burden scores for overall nodule malignancy.
Figure 6 shows the time trend of PBS by lead time to lung cancer diagnosis. The PBS-overall based on 36 informative proteins among those with malignant nodules were consistently higher than those with benign nodules, even at 5 years before cancer diagnosis (Figure 6, A). In contrast, the PBS-imminent displayed an upward trend, and the differences between individuals with malignant vs benign nodules became more distinctive when approaching time of lung cancer diagnosis (Figure 6, B).
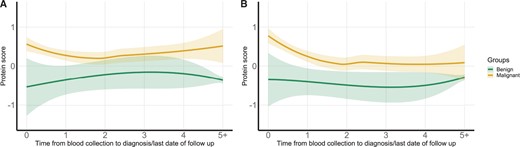
Time trend of PBS by lead time from blood collection to lung cancer diagnosis or end of follow-up in individuals with benign vs malignant nodules. (A) PBS-overall calculated based on the top 36 informative protein markers. (B) PBS-imminent calculated for the 10 markers relevant for lung cancer diagnosis within 1 year after blood collection. PBS = protein burden score; PBS-imminent = protein burden scores for imminent tumors; PBS-overall = protein burden scores for overall nodule malignancy.
Consideration of key factors
Specifically for the 10 protein-coding genes relevant for imminent lung cancer diagnosis, more information regarding their messenger RNA (mRNA) and protein expression in lung- and immune system–related tissues are summarized in Supplementary Table 2 (available online) (38-41). Based on the Human Protein Atlas and ProteomicsDB, all 10 genes are expressed in lung cancer and normal lung cells at the transcriptional level. As for protein expression in different tissues, where data are available, most of these 10 proteins have been reported as expressing in lung tissues, except SCGN and FASLG, which showed expression in immune-related tissues, instead.
The distribution of the protein levels for selected markers in healthy individuals, individuals with benign pulmonary nodules, and patients with cancer are shown in Supplementary Figure 4 (available online). In general, the distribution of the protein expression in healthy individuals and those with benign nodules were comparable to some extent, except for CXCL17, WFDC2, CEACAM5, surfactant protein A1 (SFTPA1), alkaline phosphatase-placental type (ALPP), and lysosomal associated membrane protein 3 (LAMP3), where there were statistically significant trends of distribution in the 3 groups (trend P < 10‒6).
The detailed associations between each of these circulating protein markers and nodule malignancy, by CT screening studies, sex, smoking status, time to diagnosis, histology, and nodule size, are shown in Supplementary Figure 1 (available online). We did not observe strong heterogeneity across the 4 LDCT studies for most of the markers. Apart from the notable differences by time to diagnosis for the 10 markers indicating imminent tumors, we observed heterogenous associations across different histologic groups for most of the markers, except for TRAIL-R2, WFDC2, and ALPP, which showed statistically significant association with nodule malignancy in all histologic groups (Supplementary Figure 1, available online).
Sensitivity analysis
Supplementary Figure 5 (available online) shows the result comparison between nested case-control design vs incident case sampling. In general, the estimations are comparable between the 2 approaches. Not surprisingly, the estimates based on incident cases are slightly closer to the null because some individuals who would later develop lung cancer were included as controls at early time points. It is expected that the protein expression profiles for those patients with nodules that eventually develop into tumors, before their cancer diagnosis, would be closer to the protein expression profiles of the defined case group.
Discussion
This study represents the first systematic search for circulating protein markers for pulmonary nodule malignancy. Based on an extensive assessment of circulating proteomics in prediagnostic samples from 4 LDCT screening studies, we showed that the circulating protein markers can help differentiate benign from malignant pulmonary nodules detected during LDCT screening before clinical cancer diagnosis, with some markers indicating an imminent cancer diagnosis. Overall, the 36 informative markers predominately represent a tightly connected network of pathways related to response to stimulus, cell death, and the immune system.
Given the increasing use of LDCT screening for lung cancer, there has been a growing interest in differentiating malignant and benign pulmonary nodules using blood-based biomarkers (42-45). Most of previous work on biomarkers for nodule malignancy tended to have small sample sizes and focused on a limited number of markers, and none employed a comprehensive and systematic search strategy (42,46-50). The advantage of the systematic search we used in the current study is that it enabled us to extract the most informative signals, including novel biomarkers that had not previously been investigated. The protein burden scores that integrated the information of the top protein markers showed a strong association with nodule malignancy, even within LungRADS classes, indicating the potential value of protein markers in improving the performance of current tools for differentiation of benign and malignant nodules.
Some previous studies used prediagnostic samples, but none were beyond 2 years before diagnosis (43,49,51,52). We included an expanded time horizon to 5 years before diagnosis, which enabled us to investigate biomarkers by time intervals, and we observed that 36 circulating protein markers can differentiate nodule malignancy even more than 3 years before diagnosis (eg, WFDC2, CXL17, TRAIL-R2, secretoglobin 3A2 (SCGB3A2)). Being able to assess nodule malignancy more than 3 years before clinical diagnosis would help physicians devise a better patient management strategy. Among these top 36 markers, we identified a subset of 10 markers most relevant for imminent lung cancers. For these markers, we observed a more distinguishing pattern of the protein expressions as the patients approached the diagnosis time. These markers indicating imminent tumors have the potential to facilitate more immediate clinical follow-up to improve patient prognosis.
Evaluating biomarkers in a longer prediagnostic time window also enabled identification of biological pathways beyond inflammatory-related or cancer-related proteins, which were often the focus of previous studies on imminent lung tumor (42). Given the longer lead time, some of the informative protein markers are likely not directly linked to the presence of the tumor itself but represent system dysregulation or a cellular environment that promotes tumor initiation and growth later (53). Overall, our data show that a tight-knit network of pathways that appear to have a role in differentiating malignant from benign nodules is related to response to stimulus, apoptosis, immune system, and regulators of these biological steps.
Several circulating biomarker tests are commercially available for indeterminate pulmonary nodules, such as EarlyCDT Lung (Oncimmune Holdings plc, Nottingham, UK) and Nodify XL2 (Biodesix, Boulder, CO, USA) (47, 48, 54, 55). However, EarlyCDT Lung, which consists of 7 autoantibodies, has shown insufficient sensitivity and currently is not recommended by the National Institute for Health and Care Excellence guidelines for nodule classification because of weak evidence (56,57). Nodify XL2, which consists of 2 biomarkers (LG3BP and C163A), has yet to be further validated in the clinical setting (48).
Some of the previous circulating protein panels proposed for indeterminate pulmonary nodules were adapted from initial discovery studies for lung cancer that did not use benign nodules as the comparison group (47). Although the protein expression levels can be comparable for some protein markers, we observed that the expression levels of some specific protein markers are different based on the presence of pulmonary nodules, regardless of their subsequent malignancy status, which supports the rationale of using patients with benign nodules as the primary comparison group and healthy controls only to assess background expression level. A notable example is WFDC2, whose expression level increased incrementally from healthy controls to benign nodule to malignant nodules. Using healthy controls without nodules would likely result in overestimation of the association when the goal is to differentiate benign from malignant nodules.
Some of our 36 informative markers were previously reported to be associated with lung cancer risk—for example, IL-6, CEACAM5 and KRT19—but many were novel markers that have not been previously reported in lung cancer (46,52,58). For example, CEACAM5, a member of the human carcinoembryonic antigen (CEA) protein family, is often used to monitor treatment response (59). Substantial previous work showed that CEACAM5 can be used to predict lung cancer risk, and some suggested the utility of distinguishing benign from malignant pulmonary nodules (43,49,52,54,60). We only observed an association between CEACAM5 and lung cancer within 1 year of lead time. In accordance with our finding regarding the overexpression of CEACAM5 protein in imminent lung cancer tumor, CEACAM5 is known to accelerate tumor growth and is specifically identified as a driver of metastasis (61).
Similarly, KRT19 (or cytokeratin 19) is responsible for structural integrity of epithelial cells, and its fragment antigen (CYFRA21-1) is frequently used to monitor tumor presence, although its specificity for cancer is considered low. It has previously been suggested as a biomarker for both lung cancer risk and nodule malignancy (43,49,50,52,54,60). We found that KRT19 is a predictor of nodule malignancy only for the imminent lung tumors, and this finding is compatible with a previous study showing that KRT19 is released into the blood circulation when necrosis occurs in lung tumor tissue (62).
As one of the most studied multifunctional cytokines, IL-6 is known for its immunosuppressive role in tumorigenesis. It is widely reported that the elevated serum level of cytokine family, including IL-6, is associated with lung cancer incidence (63,64). However, its role for classifying indeterminate nodules has not been previously investigated.
Overall, WFDC2 (also known as human epididymis protein 4 [HE4]) exhibited the strongest association with nodule malignancy in our study. It is known to be expressed in pulmonary epithelial cells and was previously shown to be associated with innate immunity, with a particular role in defense of the lung epithelial cells (39,65). Several recent studies have suggested its involvement in chronic obstructive pulmonary disease and lung cancer through proinflammatory responses, and high concentration of HE4 in serum and lung cancer tissues has previously been reported (66-68). HE4 was shown to be associated with lung cancer risk (58), but the associations with nodule malignancy was inconclusive (69).
The remaining top markers have not been previously investigated for nodule differentiation, but some were suggested to have a role in lung carcinogenesis. As expected, several of the top markers are involved in the inflammation pathway. MMP12, secreted by inflammatory macrophages, was shown to be highly expressed in lung cancers, can trigger angiogenesis, and results in inflammatory cell infiltration and epithelial growth (70,71). MMP12 was also identified as a tumor-associated antigen that triggers immune response and was suggested for use in a diagnostic autoantibody panel (72,73). CXCL17 is a mucosal chemokine that also exhibits an angiogenic effect (74); it was shown to be expressed in lung airways and in lung cancer cells (74). SCGB3A2, a multifunctional secreted protein, can activate inflammasome pathways and lead to pyroptosis (75-77).
As a member of the tumor necrosis ligand superfamily, FASLG plays a role in apoptosis signaling and tissue homeostasis, and its loss of expression was often observed in non-small cell lung cancer [78]. The lower activation of the FAS/FASLG signaling pathway implies resisting activation-induced cell death and hence weakens the immune response (79-81). We observed a lower FASLG level in circulation among patients with lung cancer, consistent with these previous experimental data. TRAIL-R2, related to apoptosis, was recently suggested as a negative regulator of the tumor suppressor p53 and is highly expressed in many types of tumor cells (82). Given its key role in TNR-related apoptosis, several clinical trials were conducted using TRAIL-R2–agonistic antibodies (83-85).
There are several limitations to our study. First, even with a total of 4 LDCT studies, our sample size is modest, which may lead to some degree of false-negative findings. To address the issue of statistical instability, we applied a resampling approach as well as FDR to adjust for multiple comparisons. Second, because this study was conducted using lung cancer screening cohorts, the results are not generalizable to people at low risk for lung cancer, including never-smokers. Third, because the normalized protein expression values are based on relative abundance, it is not ideal for building a prediction algorithm that can be deployed for clinical implementation. Therefore, our main goal was to identify the potential informative markers and the biological pathways represented and to assess the time trend based on lead time. Nonetheless, working toward to the clinical translation, the findings reported here are now contributing to the configuration of a customized panel for both lung cancer risk and pulmonary nodule malignancy that will enable absolute quantifications of the targeted protein markers. If validated, the customized protein panel may be useful in the real-life clinical setting to characterize pulmonary nodules found in the context of lung cancer screening and also to provide malignancy assessment of incidental pulmonary nodules found in other clinical situations.
There are notable strengths of our study. This study represents the first systematic investigation of circulating protein for pulmonary nodule malignancy based on an international collaboration of multiple screening cohorts. With prediagnostic samples, we were able to identify several novel and informative protein markers for pulmonary nodule malignancy. In general, we have observed consistency of results across studies, which suggests the robustness of our results. The findings from our study clearly demonstrated an added value of circulating protein markers beyond LungRADS classifications. This study paves the way to analyze the top markers to be measured in absolute quantifications as the next step and will allow the establishment and calibration of the risk prediction algorithm as well as the external validation of an independent study (21).
In conclusion, we demonstrated that circulating protein markers can help differentiate malignant from benign pulmonary nodules detected in LDCT screening. Clinically, this means that the protein markers may be useful for devising a better management plan for patients beyond the clinical classification system. Our study provides a road map for developing protein marker panels for use in pulmonary nodule management after LDCT screening.
Data availability
The dataset presented in this study is available from the corresponding author upon request and committee approval.
Author contributions
Elham Khodayari Moez, PhD (Conceptualization; Data curation; Formal analysis; Funding acquisition; Investigation; Methodology; Project administration; Resources; Software; Supervision; Validation; Visualization; Writing—original draft; Writing—review & editing), Hilary A. Robbins, PhD (Data curation; Funding acquisition; Investigation; Methodology; Project administration; Resources; Supervision; Writing—original draft; Writing—review & editing), Christopher I. Amos, PhD (Conceptualization; Data curation; Funding acquisition; Investigation; Methodology; Project administration; Resources; Supervision; Writing—original draft; Writing—review & editing), Luis M. Montuenga, PhD (Conceptualization; Data curation; Funding acquisition; Investigation; Resources; Supervision; Writing—original draft; Writing—review & editing), Kiera Murison, MSc (Data curation; Formal analysis; Methodology; Project administration; Writing—original draft; Writing—review & editing), Benjamin Grant, MSc (Data curation; Project administration; Resources; Writing—original draft; Writing—review & editing), Michael P. A. Davies, PhD (Conceptualization; Data curation; Funding acquisition; Investigation; Methodology; Resources; Supervision; Writing—original draft; Writing—review & editing), Sukhinder Atkar-Khattra, BSc (Conceptualization; Data curation; Investigation; Project administration; Resources; Writing—original draft; Writing—review & editing), Mattias Johansson, PhD (Conceptualization; Data curation; Funding acquisition; Investigation; Methodology; Project administration; Resources; Supervision; Validation; Writing—original draft; Writing—review & editing), Andrea Pasquier Nialet, MSc (Data curation; Investigation; Project administration; Resources; Writing—original draft; Writing—review & editing), Karmele Valencia, PhD (Data curation; Project administration; Resources; Writing—original draft; Writing—review & editing), Javier J. Zulueta, MD, PhD (Data curation; Investigation; Project administration; Resources; Writing—original draft; Writing—review & editing), Geoffrey Liu, MD (Conceptualization; Data curation; Funding acquisition; Investigation; Methodology; Resources; Supervision; Writing—original draft; Writing—review & editing), John K. Field, PhD (Conceptualization; Data curation; Funding acquisition; Investigation; Methodology; Resources; Supervision; Writing—original draft; Writing—review & editing), Stephen Lam, MD (Conceptualization; Data curation; Funding acquisition; Investigation; Methodology; Project administration; Resources; Supervision; Writing—original draft; Writing—review & editing), Yonathan Brhane, MSc (Conceptualization; Data curation; Formal analysis; Investigation; Methodology; Resources; Software; Validation; Visualization; Writing—original draft; Writing—review & editing), Matthew T. Warkentin, PhD (Conceptualization; Data curation; Formal analysis; Investigation; Methodology; Software; Validation; Visualization; Writing—original draft; Writing—review & editing), Miguel Mesa-Guzman, MD, PhD (Data curation; Project administration; Resources; Writing—original draft; Writing—review & editing), Rayjean J. Hung, PhD (Conceptualization; Data curation; Formal analysis; Funding acquisition; Investigation; Methodology; Project administration; Resources; Software; Supervision; Validation; Visualization; Writing—original draft; Writing—review & editing).
Funding
This work was supported by National Institute of Health (NIH U19 CA203654 (INTEGRAL) and Canadian Institute for Health Research (FDN 167273). L.M.M. was also supported by ISCIII Fondo de Investigación Sanitaria-Fondo Europeo de Desarrollo Regional (PI19/00098; PI22/00451), Lung Ambition Alliance and Fundación Roberto Arnal Planelles.
Conflicts of interests
Authors declared no conflict of interest.
Acknowledgement
Disclaimer: Where authors are identified as personnel of the International Agency for Research on Cancer/World Health Organization, the authors alone are responsible for the views expressed in this article and they do not necessarily represent the decisions, policy or views of the International Agency for Research on Cancer/World Health Organization.
The funders had no role in study design, data collection, analysis, decision to publish, or manuscript preparation.
This Study was approved by the Research Ethics Board of the Mount Sinai Hospital. The Ethics approval was also obtained from local institutes at each study sites.
References
Surveillance, Epidemiology, and End Results Program.