-
PDF
- Split View
-
Views
-
Cite
Cite
Saki Takahashi, Bryan Greenhouse, Isabel Rodríguez-Barraquer, Are Seroprevalence Estimates for Severe Acute Respiratory Syndrome Coronavirus 2 Biased?, The Journal of Infectious Diseases, Volume 222, Issue 11, 1 December 2020, Pages 1772–1775, https://doi.org/10.1093/infdis/jiaa523
- Share Icon Share
Abstract
Biased seroprevalence estimates can occur using serological assays optimized with validation sets unrepresentative of disease spectrum in the general population. Correct interpretation of serosurveys for severe acute respiratory syndrome coronavirus 2 requires quantifying variations in sensitivity with disease severity and over time.
Serosurveys are needed to understand how many people have been infected by severe acute respiratory syndrome coronavirus 2 (SARS-CoV-2) and where we are in the epidemic curve. Unprecedented serosurveillance efforts have been launched (eg, Solidarity II, National Institutes of Health) to generate local and global infection estimates to guide social distancing policies. Recently published results suggest that the proportion of the population that has been infected, even in places with explosive outbreaks such as Spain or New York City, is low and far from the levels required for herd immunity [1–3].
Multiple serological assays and rapid tests are now available and, as of 16 July 2020, the Food and Drug Administration has authorized the use of 29, which report a range of test performance characteristics (ie, sensitivity and specificity, as well as positive and negative predictive values) [4]. Assay validation requires samples from individuals with known infection status in order to determine test performance characteristics. Owing to potential cross-reactivity of antibody responses to seasonal coronaviruses, much of the focus of assay development has been on ensuring near-perfect specificity, to minimize the risk of false positive results. This is particularly important during early stages of the epidemic, when the number of true positives is expected to be very low. However, if the purpose of deploying a serological assay is to quantify the proportion of the population that has been infected by SARS-CoV-2 (ie, serosurveillance), adequate characterization of assay sensitivity to detect prior infection in the general population is important as well. We raise this issue because in the absence of such characterization, it will not be possible to generate accurate estimates of population-level exposure to this novel pathogen [5].
A growing body of evidence suggests that asymptomatic and mild SARS-CoV-2 infections, together making up >95% of all infections, may be associated with lower antibody titers than more severe infections [6–16]. Similarly, it is known that antibody levels peak a few weeks after infection and then decay gradually [17–19]. Yet, positive controls used for assay optimization and validation are usually limited to early convalescence samples from hospitalized patients with severe disease, leading to what is commonly known as spectrum bias. Sensitivities estimated from these sample sets may therefore overestimate the actual sensitivity that the assay would have when applied to the general population, leading to underestimates of the true seroprevalence.
To illustrate this point, we quantified the amount of bias in estimating population seroprevalence potentially introduced by the choice of positive controls used to evaluate assay sensitivity. We evaluated the impact of: (1) using validation sets with different proportions of severe, mild, and asymptomatic infections (Figure 1A), and (2) using validation sets with samples from recent infections only (Figure 2A and 2B). While the relationship between disease severity and assay sensitivity has yet to be quantified for most assays, we assumed that sensitivity was highest for severe infections and considered a range of values for asymptomatic and mild infections. Similarly, we assumed that sensitivity peaked early after infection and then decayed over time (Figure 2C). For simplicity, we fixed test specificity at 100%.
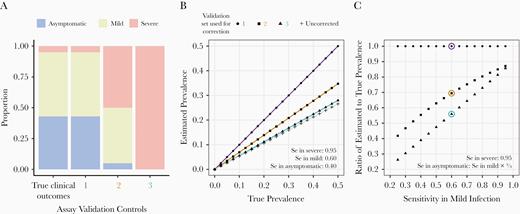
Impact of assay validation set and symptom-dependent sensitivity on estimation of seroprevalence. A, True distribution of clinical outcomes observed among severe acute respiratory syndrome coronavirus 2 (SARS-CoV-2)–infected individuals (left bar), classified as asymptomatic, mild, or severe infection, and 3 simulated sets of positive controls used in assay validation. B, For a range of true prevalence, we calculated the prevalence that would be estimated in the population under the scenarios of no correction for test characteristics (+ symbols), and corrections for test sensitivity as determined from each validation set (circles, squares, and triangles). We assumed values of symptom-dependent sensitivities (Se), as shown in the panel, and a specificity of 100%, leading to crude sensitivities of 53.15% (set 1), 76.5% (set 2), and 95% (set 3) in the validation sets. For all of these scenarios, the true sensitivity in the general population would be 53.15%. C, For a range of sensitivities in mild and asymptomatic infections (assuming sensitivity in asymptomatic infections is two-thirds of the sensitivity in mild infections), we calculated the ratio of estimated to true prevalence for each validation set used for correction. The scenarios in B are circled.

Impact of assay validation set and time-dependent sensitivity on estimation of seroprevalence. A, Epidemic curve from an Susceptible-Exposed-Infectious-Recovered (SEIR) transmission model with severe acute respiratory syndrome coronavirus 2 (SARS-CoV-2)–like parameters in a completely susceptible population, with mean latent period of 3.5 days, mean infectious period of 7 days, and a basic reproductive number (R0) of 2.1. We simulated cross-sectional seroprevalence surveys performed at 60, 180, and 300 days into the epidemic (labeled points on the curve). B, Proportion of the ever-infected individuals that experienced infection within 0–60 , 60–180 , or ≥180 days of the serosurveys performed at days 60, 180, and 300. The last bar shows a simulated set of positive controls used in assay validation, which includes only recent samples obtained within 0–60 days after infection. We assume for these simulations that the validation controls, and all infections, represent severe infections with a baseline test sensitivity of 95%. C, For a range of true prevalence, we calculated the prevalence values that would be estimated in the population at days 60, 180, and 300—assuming that sensitivity (Se) is reduced to 80% of the baseline level in infections that are 60–180 days old, and to 60% of the baseline level in infections that are ≥180 days old—and a specificity of 100%), correcting for test characteristics using the positive control set from the last bar in B. We used the procedure described in the Supplementary Methods to calculate the estimated prevalences. We also considered prevalence values that would be estimated in the population if the 2 sources of spectrum bias (both clinical outcomes and time since infection) are present. For this simulation (filled squares), we calculated the prevalence that would be estimated at day 180, now assuming that the distribution of true clinical outcomes and their test sensitivities is equal to that in B (ie, in terms of severity, 43% asymptomatic, 52% mild, and 5% severe infection, and in terms of sensitivity, 95% in severe, 60% in mild, and 40% in asymptomatic infection), and that the positive controls are all severe, recent infections.
Assays with imperfect sensitivity lead to underestimates of the true seroprevalence (Figure 1B, + symbols), but can be easily corrected for if the actual sensitivity of the assay in the sampled population is known (Figure 1B, circles) [20]. However, if test sensitivity has been determined from positive control sets skewed towards those with severe clinical outcomes (high antibody levels), the estimated prevalence, even after correction, will still underestimate the true prevalence (Figure 1B, triangles and squares). The magnitude of the underestimate will depend on how biased the distribution of positive controls is relative to the population, and on how much assay sensitivity varies with disease severity (Figure 1C). Similarly, corrected estimates of prevalence will equal the true prevalence only if decreases in sensitivity due to waning antibody responses over time can be accounted for (Figure 2C). If spectrum bias stemming from clinical outcomes as well as spectrum bias stemming from times since infection are both present, underestimation of the true prevalence will be greater.
These results have important implications for assay development and for the interpretation of SARS-CoV-2 seroprevalence studies. First, they highlight the need to quantify the extent to which the sensitivity of the assays used in ongoing serosurveillance studies varies with disease severity and over time, and possibly differentially over time by disease severity. Incorporating loss of sensitivity with increasing time since infection will gain importance as the pandemic progresses. More importantly, these results highlight the need for long-term studies characterizing kinetics of antibody responses to SARS-CoV-2 across the severity spectrum. If antibody responses are significantly lower in milder cases, or if there is significant waning in the months after infection, assays for seroprevalence studies should be optimized to detect these lower titers. Finally, these results caution against accepting aggregate sensitivities and specificities reported by assay manufacturers at face value. Ideally, sensitivities and specificities should be stratified by disease severity and time since infection, and the characteristics of the validation set should be reported at a minimum.
Based on these results, we propose the following recommendations to improve the optimization, validation, and interpretation of SARS-CoV-2 seroprevalence studies. First, we emphasize the need for studies measuring antibody levels longitudinally in individuals who have had confirmed SARS-CoV-2 infection. Such studies should include individuals with a range of disease severity, as discussed here, as well as other factors that may affect assay sensitivity such as age and immunodeficiency. Second, a set of standards should be established for assay validation. Importantly, positive controls should include samples across the spectrum of severity and times since infection. To these ends, a SARS-CoV-2 reference serum bank of well-characterized and representative positive control sets could be created. This reference panel would also facilitate direct comparability of test performance across assays. Third, we recommend developing a set of criteria for reporting the results of assay validation. In particular, characteristics of validation samples used to determine test performance should be reported, as well as any heterogeneities in performance identified in the validation process.
Correct interpretation of SARS-CoV-2 seroprevalence studies will not be possible until we know the sensitivity of serological assays to detect mild and asymptomatic infections and infections occurring months beforehand. We urge the Food and Drug Administration to revisit reporting requirements for performance characteristics of SARS-CoV-2 serological assays with this in mind.
Supplementary Information
Supplementary materials are available at The Journal of Infectious Diseases online. Consisting of data provided by the authors to benefit the reader, the posted materials are not copyedited and are the sole responsibility of the authors, so questions or comments should be addressed to the corresponding author.
Notes
Author contributions. S. T., B. G., and I. R. B. conceived the study, performed the simulations, and wrote the manuscript.
Disclaimer. The funders had no role in the writing of the manuscript or the decision to submit it for publication.
Financial support. S. T. was supported by the Schmidt Science Fellows, in partnership with the Rhodes Trust.
Potential conflicts of interest. All authors: No reported conflicts. All authors have submitted the ICMJE Form for Disclosure of Potential Conflicts of Interest. Conflicts that the editors consider relevant to the content of the manuscript have been disclosed.
References