-
PDF
- Split View
-
Views
-
Cite
Cite
Teresa Gil-Lopez, Cuihua Shen, Grace A Benefield, Nicholas A Palomares, Michal Kosinski, David Stillwell, One Size Fits All: Context Collapse, Self-Presentation Strategies and Language Styles on Facebook, Journal of Computer-Mediated Communication, Volume 23, Issue 3, May 2018, Pages 127–145, https://doi.org/10.1093/jcmc/zmy006
- Share Icon Share
Abstract
This study empirically examines context collapse on Facebook by examining audience influences on content and language in self-disclosures. Context collapse is the process of disparate audiences being conjoined into one. Using a public longitudinal behavioral data set of 6,378 Facebook users, the study found that the size and heterogeneity of people’s networks were positively associated with the number of text status updates they posted, but negatively associated with language style variability of these updates during 12 months. Results suggest that people manage their online self-presentation in ways that are consistent with lowest common denominator, imagined audience, and accommodation propositions. Network size was positively associated with the proportion of positive emotional language and negatively with negative emotional language, whereas heterogeneity had the opposite effect.
About half the globe (76% of Internet users) regularly interacts on social network sites (SNSs) (Poushter, 2016) to stay in contact with family/friends, initiate relationships (Smith, 2011), maintain work ties (Olmstead, Lampe, & Ellison, 2016), etc. Because each of the diverse social goals may have a unique target audience, people may struggle to frame messages relevant to subsets of a network (e.g., vacation moments are more appropriate for family than colleagues).
This phenomenon—context collapse (boyd, 2002; Davis & Jurgenson, 2014; Litt, 2012; Meyrowitz, 1986)—occurs when some spheres of a network, mostly detached non-digitally, are combined to blur separation boundaries. Context collapse is more pervasive when online ego1 networks (henceforth, networks) increase in size and heterogeneity. SNSs may connect otherwise segmented groups, encouraging people to share content with their entire network, which could be awkward given a disconnect between appropriate behavior for an entire network versus different subgroups. While some SNSs provide custom privacy settings to restrict access to certain content for different people, users are unlikely to use them (Wisniewski, Knijnenburg, & Lipford, 2014).
Context collapse can potentially influence the amount and nature of self-disclosures on SNSs. Since online disclosures and context affect appropriateness judgments (Bazarova, 2012), individuals use varied self-presentation strategies to offset context collapse: self-censorship (Marwick & boyd, 2011; Vitak, Blasiola, Patil, & Litt, 2015), sophistication of design logic (Oh & LaRose, 2016), use of advanced privacy settings (Vitak, 2012), or audience-specific message adaptations (Bazarova, Choi, Schwanda Sosik, Cosley, & Whitlock, 2015; Lin & Qiu, 2013).
However, research to date has primarily focused on how context collapse may affect how much people disclose (Vitak, 2012; Wang, Burke, & Kraut, 2016). Fewer empirical studies examine if the content (what) and language (how) characteristics of such disclosures may change due to context collapse. Many studies corroborate the key role audiences play in the decision-making process prior to posting (Burke, Marlow, & Lento, 2009; Oeldorf-Hirsch, Hecht, Morris, Teevan, & Gergle, 2014; Sleeper et al., 2013). Others have explored emotion disclosures, which depend on audience composition (Bazarova et al., 2015; Burke & Develin, 2016). Yet, little is known about how audience affects the content and language of messages beyond their emotional valence. Finally, most studies have relied on self-report data (e.g., Binder, Howes, & Sutcliffe, 2009; Vitak, 2012). Empirical studies using behavioral data are scarce and have primarily used data-driven approaches such as machine learning (Wang et al., 2016).
We make important contributions to context collapse by integrating and testing theoretical processes of communication accommodation, lowest common denominator and imagined audience with longitudinal behavioral data. We assess how much users self-disclose and also how and what they disclose in Facebook text status updates by linking language-style variability, emotional expression and person-related content to the size and heterogeneity of networks. Linguistic data allow us to explore the direct product of impression management strategies regardless of users’ ability to self-report about them. In fact, we are the first to assess language style variation across multiple text status updates to observe audience adaptation over time, as well as the first to employ open behavioral data, allowing for verification/replication.
Theory and hypotheses
Understanding context collapse
Context collapse occurs when people, information, and norms from different settings meet (boyd, 2002; Marwick & boyd, 2011; Meyrowitz, 1986). Researchers have observed that, with the surge of SNSs, the boundaries separating group-based online communities began to blur (boyd & Heer, 2006). Collapsed contexts flatten multiple audiences into one, and challenge users to manage personal boundaries and online identities across diverse sets of norms (boyd, 2002).
Context can be understood in terms of role identities and associated networks (Davis & Jurgenson, 2014). Individuals relate to each other via different social roles and present themselves as a function of the context—constructing a situation-specific dimension of their identity. Because each situation entails different norms and expectations, presenting oneself appropriately for one context may not work for other contexts. This may also occur in offline communication: phone calls can interrupt conversations and bring role conflicts as “we are (…) left to juggle between the sensibilities of our two audiences” (Ling, 2008, p. 65). Even in face-to-face interactions (e.g., running into a colleague while having an intimate conversation), awkwardness may arise where one tries to optimally manage two disparate identities–personal and work. Yet, these situations are inherently synchronous and often require physical co-presence, whereas SNSs, having no contextual boundaries, can bring additional complications. Context collapse thus captures how different segments of a user’s social network come together in these platforms in potentially awkward ways (Marwick & boyd, 2011).
Herein, we examine context collapse employing measures of network size and heterogeneity. Size is the number of people in a person’s network, whereas heterogeneity is the extent to which a person’s connections are not friends with each other. Size naturally captures the degree of expansiveness of a network. Heterogeneity measures the degree to which a person’s network is fragmented. For example, Person A may have 100 Facebook friends who are all connected, whereas Person B may have the same number of friends but none are connected. Because friends from the same audience (i.e., school band) are likely to be connected amongst themselves, the lack of interaction among Person B’s friends suggests that these friends may belong to separate social spheres and possess unique information and resources, while dense connections among Person A’s friends indicate a single, close-knit group. This principle constitutes a pillar of the prominent “strength of weak ties” hypothesis (Granovetter, 1973) and has received consistent empirical support (e.g., Shen, Monge, & Williams, 2014).
Context collapse and self-presentation strategies
To investigate online self-presentation and identity, understanding how individuals construe their identity in social interaction is imperative. A common lens used to explain identity in the social media era is Goffman’s dramaturgical metaphor in “Presentation of Self” (1959). For Goffman, presenting oneself in everyday social situations is an ongoing process of impression management and performance. Audiences are segregated, and different performances are presented to each, ensuring that individuals’ impressions are related to the specific setting in which an interaction occurs. SNSs offer a stage where users can perform their public identity by sharing what they want other people to see as representative of themselves. The problem arises when a performance is appropriate for one group but not others. In SNSs, performances are simultaneously displayed to multiple audiences, which are no longer segregated. A few outcomes are possible given these challenges.
Number of status updates
When individuals have smaller, more homogeneous networks, disclosures bring little conflict for self-presentation. Indeed, self-disclosures were positively associated with network density (Wang et al., 2016). As social networks increase in size and heterogeneity, users may experience more apprehension because managing impressions becomes more difficult as social spheres collide in the same online space. One strategy to mitigate such apprehension is to reduce the frequency with which information is shared on SNSs: by presenting one’s identity less frequently, people reduce the risk of sharing an aspect of it to an inappropriate portion of their network, thereby minimizing the possibility of embarrassment. Thus, as network size and heterogeneity increase, people will post less to SNSs. Although the above-mentioned explanation has a clear intuitive appeal, empirical results do not always support it: the amount of disclosures has been found to increase as both audience size and diversity increase (Vitak, 2012), whereas others found mixed results with respect to network size (Wang et al., 2016). This could result from a disconnect between privacy concerns and users’ self-disclosing behavior (Debatin, Lovejoy, Horn, & Hughes, 2009), which suggests that, even when users perceive a risk of inappropriate self-disclosure, they may not act upon it. Moreover, as networks increase in size and heterogeneity, the number of reasons to stay in contact with different groups of people increases, providing new opportunities to post. Accordingly, the more SNS contacts, the more active one should become on the platform. Considering these two alternative processes, we present two pairs of competing hypotheses on SNS network characteristics and the number of status updates:
H1: Users with a larger network have: (a) fewer, or (b) greater number of posted status updates.
H2: Users with a more heterogeneous network have: (a) fewer, or (b) greater number of posted status updates.
Linguistic style variability in text status updates
Online self-presentation strategies may depend not only on how much users disclose, but also on how they choose to disclose based on their audience. Past research shows that one of the closest and most direct reflections of a speaker’s adjustment to an audience is linguistic style (Bell, 1984; Danescu-Niculescu-Mizil, Gamon, & Dumais, 2011), which refers to aspects of language indicating how things are said, independent of content (i.e., what is said). We advance propositions about possible language-based audience adaptation strategies.
Communication accommodation theory (CAT) (Giles, 2008) suggests that people adjust their speech in response to group differences. People tend to converge more when they are motivated to seek approval from their conversation partners (Palomares, Giles, Soliz, & Gallois, 2016). When considering accommodation processes in relation to SNSs, CAT’s explanation relies on a sender’s perceptions of who the recipient is, and what is expected and normative. Depending on this perception, people will adapt their language accordingly. For example, language will differ between communicating with an ingroup member or an outgroup member, because the standards and conventions for what is appropriate change (Palomares et al., 2016). As a person’s network size increases, the number of conversational partners they need to accommodate to also increases. The linguistic style adopted to accommodate to one intended target could change for another because different individuals have distinct norms and expectations, which has implications for language use. Thus,
H3: Users with a larger network have greater linguistic style variability.
Although the need to adapt to diverse norms would intuitively be accompanied by greater linguistic style variability across status updates, other considerations may be at play when users face the need of addressing multiple or heterogeneous audiences. Often, users create a “mental conceptualization of the people with whom they are communicating” (Litt, 2012, p. 331). In other words, users construct an imagined audience, whereby they form judgments to delimit such an audience to their own desires to reach specific individuals or groups, in spite of being aware of the virtually unbounded nature of SNS audiences (Litt, 2012; Marwick & boyd, 2011). The imagined audience may not match the actual audience, but it is the former that arguably guides users’ self-presentation strategies on SNSs, allowing them to assess the normative character of their online behavior and disclosures. For example, those perceived to be one’s actual friends rather than Facebook acquaintances are more likely to be a part of such imagined audience. As a means to obtain a more nuanced and subjective perception of audience, researchers have started to consider actual, offline connections in addition to the total number of Facebook contacts to study SNS outcomes (Ellison, Steinfield, & Lampe, 2011).
Extending the idea of the imagined audience, Hogan’s (2010)lowest common denominator approach to online self-presentation posits that users deal with context collapse by sharing information that is optimally appropriate for all audience segments. However, “one need not consider everyone when submitting content but only two groups: those for whom we seek to present an idealized front and those who may find this front problematic” (p. 383). For example, when posting about a party on Facebook a teenager may have an intended audience (e.g., school friends), but she also has to consider that her message is visible to other recipients (e.g., parents), who have alternative standards of appropriateness. Unintended audiences could condition users’ self-presentation strategies to a similar extent that intended audiences do.
Integrating the rationales from Hogan (2010) and CAT, linguistic accommodation will depend on the degree of heterogeneity of the network and the disparities among segmented groups. SNS users adapt their language styles based on their perceptions of the intended and unintended audiences. For example, vulgarities may be more acceptable to one’s friends than to parents. The appropriateness concerns from multiple disconnected groups will place constraints on language use and its variation. Thus, to send messages optimally adapted to all segments of the network and increase its overall level of appropriateness, language variability will be low.
H4: Users with a more heterogeneous network have less linguistic style variability.
Expression of emotions and personal disclosure
Users communicate emotions and personal experiences on SNSs not only as a way of self-presentation but also to seek social support. The expression of positive and negative emotions has been found to be a reliable indicator of self-disclosure on SNSs (Wang et al., 2016). We seek to understand whether context collapse, as measured by the size and heterogeneity of people’s online social networks, has any bearing on emotional expressions, as users who disclose both positive and negative emotions might experience greater subjective well-being.
One possible strategy to manage online impressions is “positive self-presentation” (i.e., highlighting socially desirable parts of one’s identity), which was found to increase subjective well-being (Kim & Lee, 2011). However, audience composition may not always encourage positive self-presentation (Lee-Won, Shim, Joo, & Park, 2014), and sharing negative emotional experiences could generate benefits, too. Yet, negative self-disclosures can be judged less favorably than positive ones (Bazarova, 2012). These two strategies often conflict, given distinct costs and benefits of sharing positive versus negative emotions. Network size and heterogeneity may predict how these conflicting strategies relate to the expression of emotions.
Broadcasting to larger audiences may increase people’s motivations to manage their impressions, as larger group sizes often increase conformity and the desire to be liked (Insko, Smith, Alicke, Wade, & Taylor, 1985). Having a large online social network may, in turn, increase people’s need for impression management, as communicating with large groups encourages people to focus on themselves rather than their audience (Barasch & Berger, 2014). Indeed, people with a larger Facebook social network were more likely to express positive, rather than negative, emotions in status updates, although these effects may be recursive, considering that those who have a greater need to self-present themselves positively tend to maintain larger networks (Lin, Tov, & Qiu, 2014). In contrast, smaller networks may decrease people’s need to manage their impressions and encourage “narrowcasting,” which is a greater focus on interaction partners, in their communication (Barasch & Berger, 2014). With a lesser need to save face, people may choose to express more negative emotions—especially to gain social support. Also, because negative topics might contain more personal information, sharing them can be considered more appropriate in less public settings. On Facebook, people with a smaller network size tend to have a greater percentage of negative posts (Burke & Develin, 2016).
Beyond emotional valence, the extent to which people disclose personal matters such as work or home-related issues may be a better indicator of how much the composition of one’s audience in SNSs can condition self-presentation strategies. Personal disclosure in SNSs has become customary (Tsay-Vogel, Shanahan, & Signorielli, 2016), and even highly personal information is often included in user profiles (Nosko, Wood, & Molema, 2010). First person singular pronouns (e.g., I, my) also signal self-disclosure. Their use in status updates demonstrates their self-orientation, serving the goals of self-validation and self-expression (Bazarova & Choi, 2014). They also reflect verbal immediacy, often used in informal, socially-oriented communication (Pennebaker & King, 1999).
Although status updates can be a convenient and efficient tool for public self-expression, disclosing personal information via this relatively public channel may risk judgment from certain contacts in a network given a large audience and their perceptions about the appropriateness of private disclosures. This risk is arguably lower for more detached disclosures with lower personal involvement. Thus, users with larger networks may tend to minimize personal disclosure compared to those with smaller networks.
H5: Users with a larger network express: (a) more positive emotions, (b) fewer negative emotions, and (c) fewer person-related content words and first-person singular pronouns in text status updates.
Besides the size of a social network, fragmentation within networks could correlate with how people communicate their emotions. People embedded in a disconnected network tend to have greater instability and lower feelings of trust for their contacts (Granovetter, 1973). One reason for this fragility is a time constraint—when ties are not reinforced by mutual contacts, maintaining relationships becomes more time consuming and effortful (see Stovel, Golub, & Milgrom, 2011), which might lead to expressing negative emotions less often and positive emotions more often to maintain a positive self-presentation.
By contrast, in a network where the person’s contacts are connected to each other, this tie redundancy increases the feeling of trust amongst members in the social network as opportunistic behaviors will easily get caught (Coleman, 1988). People embedded in tightly connected networks tend to experience greater feelings of trust and social support. As a result, they may feel more comfortable self-disclosing more negative emotions as a strategy to receive support, whereas in more sparse and disconnected networks the norm is to focus on positive rather than negative emotions (Leary, 1995). Moreover, the expression of negative emotions is more private than that of positive emotions (Finkenauer & Rimé, 1998), and denser networks of strong ties may be better suited to accommodate them. Empirically, people with a denser online network make more emotional disclosures on Facebook (Burke & Develin, 2016).
Finally, heterogeneous networks are also likely to present challenges to personal disclosure. Baym and boyd (2012) argue that, when posting to diverse audiences, users’ roles relative to more or less intimate publics—family and close friends versus acquaintances—need to be negotiated every time they engage in private disclosures, as these may affect their relationships with certain individuals. In line with this, people may feel exposed when sharing about everyday life as they know multiple, distinct groups with diverse sets of appropriateness norms and expectations rather than a cohesive group compose the audience. Therefore,
H6: Users with a more heterogeneous network express: (a) more positive emotions, (b) fewer negative emotions, and (c) fewer person-related content words and first-person singular pronouns in text status updates.
Method
Data
We obtained access to anonymized databases from the myPersonality Project (mypersonality.org; Kosinski, Stillwell, & Graepel, 2013), which maintains public data for academic use.2 Data included user demographics, text status updates, and Facebook friendships. Only users with all three data types were included in analyses.
Network data
A single network was constructed using myPersonality’s main friendship table (224 million dyads). Users’ egocentric networks were then build from the main network. Network size and heterogeneity were calculated for the subset of users for which linguistic variables were also available, using the network analysis R package igraph (Csardi & Nepusz, 2006). Users who had very few connections were excluded, because their networks were too small (and likely incomplete) to calculate meaningful network measures. Following Kosinski, Bachrach, Kohli, Stillwell, and Graepel (2014), only users whose network size was at least 20 were included. The final data set contained 6,971 users and 2,063,500 status updates, which were aggregated and processed through Linguistic Inquiry and Word Count (LIWC)—a text analysis software—monthly from April 2010 to April 2011.
Text status updates and linguistic data
Linguistic analyses were carried out on only user-generated text status updates (henceforth, status updates). Shared links or media were disregarded, as our focus concerns users’ language use. The original data set includes 22 million text posts from 154,000 users from January 2009 to November 2011. We used LIWC to examine individuals’ linguistic style variability across status updates (Pennebaker, Booth, Boyd, & Francis, 2015). LIWC calculates the percentage of various language categories (e.g., function words, emotional tone) relative to the total number of words within a text (Linguistic Measures section). To calculate linguistic style variability over time, we included only consistently active users across 12 months by aggregating individuals’ status updates monthly, then calculating total word count for each month. A user was judged consistently active if their total word count each month reached at least 50 words for 12 consecutive months (Pennebaker, 2011). This helped ensure that linguistic variables would not yield interpretable values of zero, as there would be no way of differentiating between zero values indicating absence of linguistic variability and those occurring due to a lack of activity. We then selected the year containing the largest number of consistently active users, April 2010 to April 2011. Finally, since the myPersonality data set contains status updates in multiple languages, data were manually checked and non-English cases removed after all other filters were applied and our final sample was identified. These cases constituted approximately 4.7% of the sample.
Measures
Demographic controls
Users’ age (M = 24.74, SD = 8.65) and gender, which were self-reported information available on profiles, served as demographic variables. Gender was coded as dichotomous (1 = female) and age was calculated by subtracting the year of birth from 2011 (when status updates were collected). Only users between 13- and 100-years-old were included in the analysis. The upper limit was set to 100 because people reporting over 100 were less likely to provide accurate information. About 31% of the users were male (Table 1). These demographics are consistent with general myPersonality samples, with an over-representation of young and female users in particular (Bachrach, Kosinski, Graepel, Kohli, & Stillwell, 2012). Compared to the entire myPersonality sample, our sample of 6,378 users is slightly younger (25.31 versus 24.73; p < .001), and includes more females (64.73% versus 69.57%, p < .001).
Variable . | M . | Median . | SD . |
---|---|---|---|
Age | 24.73 | 22 | 8.63 |
Number of updates | 300.6 | 247 | 192.06 |
Network size | 418.5 | 330.5 | 371.38 |
Heterogeneity (original measure) | .940 | .963 | .135 |
Heterogeneity (residual) | .012 | .021 | .107 |
Function words (%) | 46.89 | 47.72 | 5.64 |
Function words (SD) | .041 | .038 | .018 |
Cognitive processes (%) | 9.84 | 9.87 | 1.87 |
Cognitive processes (SD) | .024 | .023 | .008 |
Informal speech (%) | 4.03 | 3.58 | 2.28 |
Informal speech (SD) | .016 | .014 | .008 |
Positive/Affect ratio | .672 | .676 | .098 |
Negative/Affect ratio | .322 | .317 | .097 |
Person-related words (%) | 5.55 | 5.50 | 1.48 |
First person sing. pronouns (%) | 5.83 | 5.86 | 1.87 |
Gender | 69.57% (F) |
Variable . | M . | Median . | SD . |
---|---|---|---|
Age | 24.73 | 22 | 8.63 |
Number of updates | 300.6 | 247 | 192.06 |
Network size | 418.5 | 330.5 | 371.38 |
Heterogeneity (original measure) | .940 | .963 | .135 |
Heterogeneity (residual) | .012 | .021 | .107 |
Function words (%) | 46.89 | 47.72 | 5.64 |
Function words (SD) | .041 | .038 | .018 |
Cognitive processes (%) | 9.84 | 9.87 | 1.87 |
Cognitive processes (SD) | .024 | .023 | .008 |
Informal speech (%) | 4.03 | 3.58 | 2.28 |
Informal speech (SD) | .016 | .014 | .008 |
Positive/Affect ratio | .672 | .676 | .098 |
Negative/Affect ratio | .322 | .317 | .097 |
Person-related words (%) | 5.55 | 5.50 | 1.48 |
First person sing. pronouns (%) | 5.83 | 5.86 | 1.87 |
Gender | 69.57% (F) |
Variable . | M . | Median . | SD . |
---|---|---|---|
Age | 24.73 | 22 | 8.63 |
Number of updates | 300.6 | 247 | 192.06 |
Network size | 418.5 | 330.5 | 371.38 |
Heterogeneity (original measure) | .940 | .963 | .135 |
Heterogeneity (residual) | .012 | .021 | .107 |
Function words (%) | 46.89 | 47.72 | 5.64 |
Function words (SD) | .041 | .038 | .018 |
Cognitive processes (%) | 9.84 | 9.87 | 1.87 |
Cognitive processes (SD) | .024 | .023 | .008 |
Informal speech (%) | 4.03 | 3.58 | 2.28 |
Informal speech (SD) | .016 | .014 | .008 |
Positive/Affect ratio | .672 | .676 | .098 |
Negative/Affect ratio | .322 | .317 | .097 |
Person-related words (%) | 5.55 | 5.50 | 1.48 |
First person sing. pronouns (%) | 5.83 | 5.86 | 1.87 |
Gender | 69.57% (F) |
Variable . | M . | Median . | SD . |
---|---|---|---|
Age | 24.73 | 22 | 8.63 |
Number of updates | 300.6 | 247 | 192.06 |
Network size | 418.5 | 330.5 | 371.38 |
Heterogeneity (original measure) | .940 | .963 | .135 |
Heterogeneity (residual) | .012 | .021 | .107 |
Function words (%) | 46.89 | 47.72 | 5.64 |
Function words (SD) | .041 | .038 | .018 |
Cognitive processes (%) | 9.84 | 9.87 | 1.87 |
Cognitive processes (SD) | .024 | .023 | .008 |
Informal speech (%) | 4.03 | 3.58 | 2.28 |
Informal speech (SD) | .016 | .014 | .008 |
Positive/Affect ratio | .672 | .676 | .098 |
Negative/Affect ratio | .322 | .317 | .097 |
Person-related words (%) | 5.55 | 5.50 | 1.48 |
First person sing. pronouns (%) | 5.83 | 5.86 | 1.87 |
Gender | 69.57% (F) |
User data were not consistently available across all the accessed data sets. Missing data were especially problematic for age. Comparing cases with and without age revealed differences in size (M = 99.59, SD = 145.21 and M = 418.35, SD = 371.05, respectively; t(1,381.5) = −41.675, p < .001), heterogeneity (M = .61, SD = .19 versus M = .94, SD = .13; t(620.76) = −40.233, p < .001), and number of updates (M = 245.16, SD = 117.63 versus M = 300.54, SD = 192.58; t(868.61) = −10.1, p < .001). Finally, 25 cases had missing data on gender. Given these differences, results are reported for the 6,378 cases for which complete data exist (Table 1).
Network measures
Network size: Size (hypotheses 1, 3 and 5) was measured by counting total contacts in a person’s Facebook network (Wasserman & Faust, 1994), with a log transformation due to skewness (3.28).
Linguistic measures
Number of status updates
This outcome measure was calculated by summing the number of Facebook status updates each user published in the time frame. As with most count data, this variable had a non-normal distribution with many low-frequency occurrences (see Data Analysis). In addition to the total number of status updates, LIWC analysis was applied to monthly aggregated status updates by user to generate two distinct linguistic variables: linguistic style variability and the cumulative percentage of linguistic categories.
Linguistic style variability
Because this study focuses on linguistic style audience-based adaptation strategies, linguistic categories were chosen that reflect style rather than content, to clearly differentiate between how and what people disclose. The distinction has existed since the early development of LIWC (Tausczik & Pennebaker, 2010) to show that style words, such as pronouns or articles, are the most common in regular communicative exchanges, and reflect psychological processes which are independent of the content of a specific communication. The same distinction has proved meaningful in recent work on linguistic style accommodation (see Danescu-Niculescu-Mizil et al., 2011). Hence, variability in linguistic style is a meaningful measure of audience adaptation. A high degree of variability across users’ status updates may indicate that different disclosures are being individually addressed to various rather than one specific audience. Caution is needed to interpret the psychological implications of the measures. The aim of the present project is not to understand individual differences in communication styles, but rather to measure the degree to which those styles vary in relation to context collapse.
Three LIWC dimensions were selected for the purposes of studying linguistic style variability: function words, cognitive processes, and informal speech. Function words encompass pronouns, prepositions, or conjunctions, among other style words (e.g., anyway, you or does). Cognitive processes, including subcategories such as causal words (e.g., because, hence) and insight words (e.g., think, know), reflect thinking styles. Finally, informal speech refers to colloquial language markers such as swear words or fillers (e.g., crap, like). As an example, the status update “So like um I’ve just been told that my friends are pissed at me cuz I don’t give a damn” has 65% of function words, 10% of cognitive processes, and 15% informal speech.
Linguistic style variability across status updates was obtained by calculating the standard deviation of LIWC percentages for each selected language category on the monthly status updates aggregates across the 12-month period. A large standard deviation indicated that a user varied language styles considerably over the 12-month period. We chose to aggregate status updates monthly, then perform LIWC analysis each month and calculate the standard deviation, rather than across individual status updates for two reasons. First, LIWC analysis requires at least 50 words to be reliable, yet most status updates tend to be rather short. Second, the standard deviation requires users who are consistently active during each period. Thus, we secured a large enough sample of consistently active users with enough data points for each user to calculate standard deviations.
Cumulative percentage of linguistic style categories
For monthly aggregated status updates, we calculated the cumulative percentage by dividing the number of words in each of the selected LIWC categories by the total word count, then multiplying it by 100. This measure was used as control variable in models predicting linguistic style variability, in order to account for individual differences in language use (Pennebaker, et al., 2015).
Ratio of positive/negative emotions over total affect words
These two measures were calculated by dividing the number of positive/negative words by total affect words.
Person-related content words and first person singular pronouns
Person-related words indicates the proportion of words in each monthly aggregate that correspond to any of the six LIWC word categories for personal concerns: work, leisure, home, money, religion, and death (e.g., bar, bill, God, or roomie). First-person singular pronouns indicates the aggregated proportion of words corresponding to the LIWC word category “first personal singular.” This category includes various articulations of such pronouns, for instance: I’d, me, myself.
Data analysis
For H1 and H2, the mean number of status updates (DV) was substantially smaller than the variance, with dispersion parameters that were highly significant (t statistics 28.47 and 28.52, respectively). This suggested that a negative binomial model was more appropriate than a Poisson model. H3–H6 were tested via seven regressions with language style variability (function words, cognitive-processes words, informal speech), emotion ratios, percentage of person-related words and first person singular pronouns as dependent variables, respectively.
Results
Two competing hypotheses, H1a and H1b, were tested to examine whether an increase in the size of person’s network size is associated with: (a) fewer, or (b) greater number of status updates. Controlling for age and gender, network size was positively related to the number of status updates (z = 9.34, p < .001), supporting H1b (Table 2). H2a and H2b tested whether people with greater network heterogeneity tend to post fewer or more status updates (Table 2). Controlling for age, gender, and network size, heterogeneity was positively associated with the total number of status updates (z = 6.94, p < .001), supporting H2b.
Negative Binomial Regression Models Predicting Number of Text Status Updates (N = 6,378)
. | N updates H1 . | N updates H2 . | ||
---|---|---|---|---|
. | coef . | z . | coef . | z . |
Age | −.009*** | .0008 | −.010*** | .0008 |
Female | .066*** | .0151 | .061*** | .0150 |
Size (log) | .071*** | .0076 | .082*** | .0077 |
Disconnect. | .463*** | .0666 | ||
Model AIC | 81,865 | 81,819 |
. | N updates H1 . | N updates H2 . | ||
---|---|---|---|---|
. | coef . | z . | coef . | z . |
Age | −.009*** | .0008 | −.010*** | .0008 |
Female | .066*** | .0151 | .061*** | .0150 |
Size (log) | .071*** | .0076 | .082*** | .0077 |
Disconnect. | .463*** | .0666 | ||
Model AIC | 81,865 | 81,819 |
*** < .001 ** < .01 * < .05 † < .1
Negative Binomial Regression Models Predicting Number of Text Status Updates (N = 6,378)
. | N updates H1 . | N updates H2 . | ||
---|---|---|---|---|
. | coef . | z . | coef . | z . |
Age | −.009*** | .0008 | −.010*** | .0008 |
Female | .066*** | .0151 | .061*** | .0150 |
Size (log) | .071*** | .0076 | .082*** | .0077 |
Disconnect. | .463*** | .0666 | ||
Model AIC | 81,865 | 81,819 |
. | N updates H1 . | N updates H2 . | ||
---|---|---|---|---|
. | coef . | z . | coef . | z . |
Age | −.009*** | .0008 | −.010*** | .0008 |
Female | .066*** | .0151 | .061*** | .0150 |
Size (log) | .071*** | .0076 | .082*** | .0077 |
Disconnect. | .463*** | .0666 | ||
Model AIC | 81,865 | 81,819 |
*** < .001 ** < .01 * < .05 † < .1
H3 tested whether users’ network size is positively associated with their linguistic style variability in status updates. The model ran on three linguistic dimensions: function words, words about cognitive processes, and words using informal speech (Table 3). We found no significant association between network size and function words (t = −.495, p = .62), but associations were significant and negative for cognitive processes (t = −3.82, p < .001) and informal speech (t = −4.53, p < .001). H3 was not supported. H4 tested whether network heterogeneity is negatively associated with variability in language styles. H4 was supported on all three observed language dimensions (t = −5.07, p < .001 for function words; t = −6.77, p < .001 for cognitive processes; t = −7.12, p < .001 for informal speech) (Table 3, Figure 1).
. | Function words variability . | Cogn. processes variability . | Informal speech variability . | |||
---|---|---|---|---|---|---|
. | B . | t . | B . | t . | B . | t . |
Age | −.00007** | −3.07 | −.000006 | −.46 | −.00003 | −4.34 |
Female | −.001† | −1.84 | −.0005* | −2.29 | −.0005** | −3.21 |
Size (log) | −.0001 | −.495 | −.0004*** | −3.82 | −.0004*** | −4.53 |
Heterogeneity | −.01*** | −5.07 | −.007*** | −6.77 | −.005*** | −7.12 |
Cum. % | −.001*** | −32.95 | .0007*** | 11.78 | .0021*** | 60.24 |
Model F | 248.2 (df = 5, 6372) | 39.96 (df = 5, 6372) | 850.8 (df = 5, 6372) | |||
Adj. R2 | .16*** | .030*** | .40*** |
. | Function words variability . | Cogn. processes variability . | Informal speech variability . | |||
---|---|---|---|---|---|---|
. | B . | t . | B . | t . | B . | t . |
Age | −.00007** | −3.07 | −.000006 | −.46 | −.00003 | −4.34 |
Female | −.001† | −1.84 | −.0005* | −2.29 | −.0005** | −3.21 |
Size (log) | −.0001 | −.495 | −.0004*** | −3.82 | −.0004*** | −4.53 |
Heterogeneity | −.01*** | −5.07 | −.007*** | −6.77 | −.005*** | −7.12 |
Cum. % | −.001*** | −32.95 | .0007*** | 11.78 | .0021*** | 60.24 |
Model F | 248.2 (df = 5, 6372) | 39.96 (df = 5, 6372) | 850.8 (df = 5, 6372) | |||
Adj. R2 | .16*** | .030*** | .40*** |
*** < .001 ** < .01 * < .05 † < .1
. | Function words variability . | Cogn. processes variability . | Informal speech variability . | |||
---|---|---|---|---|---|---|
. | B . | t . | B . | t . | B . | t . |
Age | −.00007** | −3.07 | −.000006 | −.46 | −.00003 | −4.34 |
Female | −.001† | −1.84 | −.0005* | −2.29 | −.0005** | −3.21 |
Size (log) | −.0001 | −.495 | −.0004*** | −3.82 | −.0004*** | −4.53 |
Heterogeneity | −.01*** | −5.07 | −.007*** | −6.77 | −.005*** | −7.12 |
Cum. % | −.001*** | −32.95 | .0007*** | 11.78 | .0021*** | 60.24 |
Model F | 248.2 (df = 5, 6372) | 39.96 (df = 5, 6372) | 850.8 (df = 5, 6372) | |||
Adj. R2 | .16*** | .030*** | .40*** |
. | Function words variability . | Cogn. processes variability . | Informal speech variability . | |||
---|---|---|---|---|---|---|
. | B . | t . | B . | t . | B . | t . |
Age | −.00007** | −3.07 | −.000006 | −.46 | −.00003 | −4.34 |
Female | −.001† | −1.84 | −.0005* | −2.29 | −.0005** | −3.21 |
Size (log) | −.0001 | −.495 | −.0004*** | −3.82 | −.0004*** | −4.53 |
Heterogeneity | −.01*** | −5.07 | −.007*** | −6.77 | −.005*** | −7.12 |
Cum. % | −.001*** | −32.95 | .0007*** | 11.78 | .0021*** | 60.24 |
Model F | 248.2 (df = 5, 6372) | 39.96 (df = 5, 6372) | 850.8 (df = 5, 6372) | |||
Adj. R2 | .16*** | .030*** | .40*** |
*** < .001 ** < .01 * < .05 † < .1
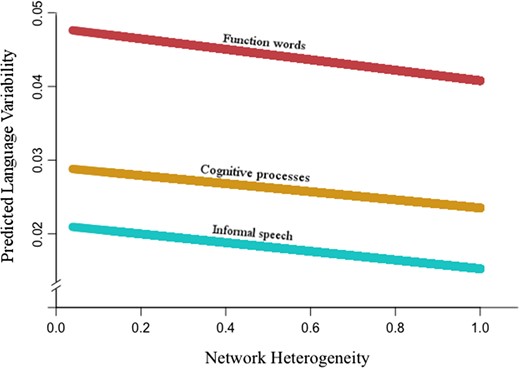
Negative relationship between network heterogeneity and linguistic style variability across three linguistic categories.
H5a and H5b tested if network size is associated with positive and negative emotions. Size was positively related to the ratio of positive emotions within affect words (t = 7.73, p < .001), and negatively related to the ratio of negative emotions (t = −7.67, p < .001), offering consistent support for H5a and H5b (Table 4). H5c tested whether network size is associated with words related to personal concerns and first person singular pronouns. Network size was not associated with the proportion of words indicating personal concerns (t = −1.26, ns) or first person singular pronouns (t = −1.24, ns), indicating network size does not predict disclosures of personal matters. H5c was not supported. H6a and H6b tested if network heterogeneity is associated with the use of positive and negative emotions. Heterogeneity was negatively related to the ratio of positive emotions (t = −9.19, p < .001) and positively related to the ratio of negative emotions (t = 9.22, p < .001), refuting H6a and H6b. H6c proposed a negative relationship between heterogeneity and disclosing personal matters and first person pronouns. Because heterogeneity was positively associated with both the proportion of words reflecting personal concerns (t = 2.21, p < .05) and first person pronouns (t = 6.57, p < .001), H6c was not supported.
. | Pos. emotion ratio . | Neg. emotion ratio . | Person-related words . | 1st Person Sing. Pronouns . | ||||
---|---|---|---|---|---|---|---|---|
. | B . | t . | B . | t . | B . | t . | B . | t . |
Age | .002*** | 18.91 | −.002*** | −19.17 | .020*** | 9.643 | −.053*** | −20.505 |
Female | .050*** | 20.08 | −.049*** | −19.92 | −.199*** | −4.986 | .819*** | 16.901 |
Size (log) | .01*** | 7.73 | −.01*** | −7.67 | −.026 | −1.262 | −.031 | −1.242 |
Heterogeneity | −.10*** | −9.19 | .10*** | 9.22 | .392* | 2.213 | 1.411*** | 6.570 |
Model F | 225.5 (df = 4, 6373) | 226.1 (df = 4, 6373) | 32.52 (df = 4, 6373) | 177 (df = 4, 6373) | ||||
Adj. R2 | .12*** | .12*** | .02*** | .099*** |
. | Pos. emotion ratio . | Neg. emotion ratio . | Person-related words . | 1st Person Sing. Pronouns . | ||||
---|---|---|---|---|---|---|---|---|
. | B . | t . | B . | t . | B . | t . | B . | t . |
Age | .002*** | 18.91 | −.002*** | −19.17 | .020*** | 9.643 | −.053*** | −20.505 |
Female | .050*** | 20.08 | −.049*** | −19.92 | −.199*** | −4.986 | .819*** | 16.901 |
Size (log) | .01*** | 7.73 | −.01*** | −7.67 | −.026 | −1.262 | −.031 | −1.242 |
Heterogeneity | −.10*** | −9.19 | .10*** | 9.22 | .392* | 2.213 | 1.411*** | 6.570 |
Model F | 225.5 (df = 4, 6373) | 226.1 (df = 4, 6373) | 32.52 (df = 4, 6373) | 177 (df = 4, 6373) | ||||
Adj. R2 | .12*** | .12*** | .02*** | .099*** |
*** < .001 ** < .01 * < .05 † < .1
. | Pos. emotion ratio . | Neg. emotion ratio . | Person-related words . | 1st Person Sing. Pronouns . | ||||
---|---|---|---|---|---|---|---|---|
. | B . | t . | B . | t . | B . | t . | B . | t . |
Age | .002*** | 18.91 | −.002*** | −19.17 | .020*** | 9.643 | −.053*** | −20.505 |
Female | .050*** | 20.08 | −.049*** | −19.92 | −.199*** | −4.986 | .819*** | 16.901 |
Size (log) | .01*** | 7.73 | −.01*** | −7.67 | −.026 | −1.262 | −.031 | −1.242 |
Heterogeneity | −.10*** | −9.19 | .10*** | 9.22 | .392* | 2.213 | 1.411*** | 6.570 |
Model F | 225.5 (df = 4, 6373) | 226.1 (df = 4, 6373) | 32.52 (df = 4, 6373) | 177 (df = 4, 6373) | ||||
Adj. R2 | .12*** | .12*** | .02*** | .099*** |
. | Pos. emotion ratio . | Neg. emotion ratio . | Person-related words . | 1st Person Sing. Pronouns . | ||||
---|---|---|---|---|---|---|---|---|
. | B . | t . | B . | t . | B . | t . | B . | t . |
Age | .002*** | 18.91 | −.002*** | −19.17 | .020*** | 9.643 | −.053*** | −20.505 |
Female | .050*** | 20.08 | −.049*** | −19.92 | −.199*** | −4.986 | .819*** | 16.901 |
Size (log) | .01*** | 7.73 | −.01*** | −7.67 | −.026 | −1.262 | −.031 | −1.242 |
Heterogeneity | −.10*** | −9.19 | .10*** | 9.22 | .392* | 2.213 | 1.411*** | 6.570 |
Model F | 225.5 (df = 4, 6373) | 226.1 (df = 4, 6373) | 32.52 (df = 4, 6373) | 177 (df = 4, 6373) | ||||
Adj. R2 | .12*** | .12*** | .02*** | .099*** |
*** < .001 ** < .01 * < .05 † < .1
Discussion
Context collapse occurs when disparate audiences are conjoined into one, creating potentially uncomfortable situations when users broadcast messages to an entire social network with different appropriateness norms across diverse groups. Examining text status updates and networks of more than 6,000 Facebook users, we conducted one of the first empirical tests of context collapse using longitudinal behavioral data to assess the relationships among users’ network size, degree of heterogeneity, number of updates, and variability of language use. Both network size and heterogeneity were positively associated with the number of status updates. However, having larger and heterogeneous networks was generally associated with low levels of linguistic style variability. Finally, network size was positively associated with the proportion of positive emotions and negatively associated with the proportion of negative emotions individuals shared on Facebook, whereas heterogeneity had the complete opposite outcome. Heterogeneity was also associated with increased use of words reflecting personal concerns and first-person singular pronouns. Network size, however, was not significantly associated with these indicators.
The first implication from these findings is that results are consistent with past findings (Vitak, 2012) challenging the belief that people with larger and more fragmented social networks disclose less information than users with smaller, tightly connected networks. Whereas broader audiences could be challenging for honest self-presentations, potentially threating identity, our data show that users with larger and more heterogeneous networks post more status updates. A potential explanation for this is that large and diverse audiences may generate more chances to communicate, and posting to such audiences is rewarding given increased chances for audience feedback, interaction with distant others, or access to novel information (Granovetter, 1973).
Second, the size and heterogeneity of an SNS audience are not only associated with how much people disclose, but also the style and content of such disclosures. Although not entirely consistent, users tended to vary their linguistic style less when audience segments were disconnected, and also, contrary to our prediction, when their networks were larger. This suggests that subjective perceptions of audience may play a more important role for self-presentation than was anticipated by H3. Possibly independent of the specific norms dictated by group memberships, large audiences complicate self-presentation (Binder et al., 2009). In that case, one possible strategy is a “one size fits all” approach, which stems from a desire to limit expression exclusively to messages that do not put self-image at risk. Aware of audience diversity, or simply, its magnitude, users self-disclose only if it is be universally appropriate (a lowest common denominator strategy), which is consistent with users who actively avoid sharing content that could disrupt a network (Oeldorf-Hirsch et al., 2014). At the same time, users may post to intended audiences constituting their mental representations. Such imagined audiences influence language adaptation as a means to accommodate the audience and gain their approval, as CAT suggests (Palomares et al., 2016). Thus, the mental representation of an ideal audience may influence language use. In mediated spaces such as SNSs, this influence may become stronger as audiences become increasingly fragmented and thus less discernable. Indeed, such consequences may be unavoidable because “the less an actual audience is visible or known, the more individuals become dependent on their imagination” of it (Litt, 2012, p. 331).
Third, users with large networks on Facebook tend to share more positive and fewer negative emotions, suggesting increased selectivity in curating status updates for a positive self-image as network size increases. Whereas expanding one’s network may bring many benefits, it likely incurs costs as well. People may be less likely to seek social support from their network during stressful and difficult times, when support is most needed. Another cost is social comparison with one’s network. In fact, exposure to upward social comparison (i.e., someone who has a better self-image than the focal person) may diminish one’s self-esteem and self-evaluations (Vogel, Rose, Roberts, & Eckles, 2014). Contrary to our prediction, network heterogeneity was associated with fewer positive and more negative emotional disclosures. Although unexpected, this finding might also be consistent with the imagined audience perspective. Users with fragmented networks may choose to address their imagined audience by sharing negative emotions. Heterogeneous audiences may foster sharing more in general, and more negative emotions specifically, which may be a means to draw on audience diversity to gain social support. Thus, whereas large network size is associated with a more positive self-image and fewer opportunities for seeking social support, audience fragmentation may have an opposite effect. A more speculative proposition is that, faced with the reality of a fragmented audience, users prioritize negatively-valenced over positive disclosures, as the former may induce more numerous and stronger reactions across audience segments.
Finally, posting to a diverse audience does not seem to deter users from engaging in personal disclosures, as higher levels of network heterogeneity were associated with a greater use of person-related content words and first-person pronouns. Personal stories in which users are the protagonist, for example about their school life or leisure time, may be deemed more pertinent to share with online audiences relative to other types of disclosures. Perhaps, under the pressure of having to negotiate one’s identity with multiple audience groups at a time, one safe strategy is to limit self-disclosures to ordinary, day-to-day personal anecdotes. Users may choose to disclose bits of personal information which they judge safe to share with everyone, building an honest “public” (to the extent that is visible to the entire network) online identity. This is also conceivable under the three theoretical perspectives considered in this text: presenting oneself in a coherent way may not only entail using an invariable linguistic style across status updates but also consistently keep disclosures within an array of safe, subjective stories that do not jeopardize the identity negotiated with the multiple audience groups.
Conclusion
In conclusion, the present study makes an important contribution by connecting and advancing theoretical propositions to understand context collapse, and by providing initial empirical evidence for the crucial influence audiences exert on users’ actual content-sharing strategies and message features such as linguistic style variability, emotional expression and person-related content, which previous studies did not explore (Burke et al., 2009; Sleeper et al., 2013). Our data demonstrated empirical support for lowest common denominator, imagined audience, and communication accommodation propositions. Yet it is still unclear whether users adapt their language to a unique and stable imagined audience, regardless of their actual readers, or if they consider all possible audiences and attendant consequences for a message.
Limitations and future research
The first limitations is that, although we took advantage of longitudinal Facebook language data over 12 months, both network and demographic data were collected as one snapshot. Therefore, analyses are cross-sectional (no causality). Second, we examined only users’ text status updates, but not photos or links, because it specifically focused on the audience-adaptation linguistic strategies directly attributable to the user and data on shared links or photos were not available to us. Links and photos also reveal important choices of impression management and should be considered in future research. Third, our analyses using LIWC were based on the monthly aggregation of users’ linguistic data over 12 months. As much as it allowed for a more reliable exploration of language variability across time, this method inevitably fails to recognize nuanced language variations from one status update to the next. Considering that the imagined (and actual) audience may be different each time users post on Facebook (Litt & Hargittai, 2016), a fruitful future research direction is to conduct a systematic empirical observation of each Facebook post in relation to the user’s intended audience groups. Further, as with similar linguistic analysis software, LIWC itself entails some limitations, the most notable being that it solely explores individual word usage, but does not analyze words in context. In addition, although the identification of some word categories is straightforward, especially those related to grammar, others such as negative and positive emotion are more subjective (Pennebaker, Boyd, Jordan, & Blackburn, 2015). Yet, numerous studies have shown LIWC’s validity in categorizing emotional and other words (see Tausczik & Pennebaker, 2010, for a review). Future research is advised to use human coders to cross-validate LIWC results.
This study is also limited due to the characteristics of the myPersonality data set. First, the data used in this study are from 2010–11. As a rapidly evolving SNS, Facebook has implemented various changes in the last few years. Thus, variables such as SNS-use frequency, expertise, or privacy-related measures were not present at the time of data collection, despite their relevance. Second, the myPersonality data set represents a convenience sample of motivated participants who opted to share their Facebook profile and network information. Additional biases were also introduced when we filtered the original sample to include only those fitting our criteria (e.g., word count). The resulting sample had relatively more young, female and active users who wrote more status updates than the original sample (M = 300.55 and M = 93.34, from April 2010 to April 2011; t (6,566.4) = 85.53, p < .001). Further, the filtered-out cases may be indicative of these users’ reluctance to engage in the platform or their coping strategy of context collapse on its own. To address these limitations and mitigate possible bias in the sample, we applied demographic and behavioral controls.3 Studies also showed that demographic variables in myPersonality are distributed more closely to the general population than traditional samples (Kosinski et al., 2013). Still, our sample may belong to a specific, distinctive subset of individuals, and further research is warranted to qualify how the impression management strategies employed by our sample may differ from those that other populations use.
The age of the myPersonality data set introduces many limitations. Whereas more recent Facebook behavioral data would be ideal, academic institutions do not have direct access to such data. If such data are used, they tend to be private, preventing replication. The benefits of using a public behavioral data set outweigh the drawbacks due to age. First, our central argument focuses on theoretical mechanisms for managing self-disclosure and context collapse, which remain relevant and apply beyond specific privacy-management tools that have since become available. Users create mental representations of the audience, which constrains choices inherent to any act of self-disclosure. If our data had included information on users’ privacy control practices, we speculate that they could be important moderators between context collapse and self-disclosure strategies. Users who actively manage privacy controls may be more aware of appropriateness norms across network segments, and have a more concrete idea of their intended audience for each individual status update. Yet, the direction of effects would likely remain the same, as self-presentation concerns are still at play: even if actively employing privacy controls, such control tends to be large and coarse-grained, since a total, exhaustive stratification is too costly and restrictive to implement. Context collapse could be reduced to a certain extent, but never eliminated despite advanced privacy tools. Second, because specific privacy features (e.g., Smartlists) were made available after the study period (September 2011), they were not as functional as they are now. Still, past research suggests that Facebook users often behave in ways that do not align with their privacy concerns (Stutzman & Kramer-Duffield, 2010), and that few users take advantage of these tools to actively manage context collapse (Vitak, 2012; Wisniewski et al., 2014). These patterns have been found even in the presence of advanced privacy controls currently supported by Facebook, which many avoid using (Kelley, Brewer, Mayer, Cranor, & Sadeh, 2011). Our study thus provides important lasting insights into the mechanisms surrounding context collapse and users’ coping strategies in today’s SNS environments.
Given our correlational data, future experiments might assess causality, wherein users’ awareness of disparate segments of their network is manipulated before writing and sharing a message on their network. Such experimental work could be done in conjunction with surveys. Studying discrete emotions (e.g., anger, joy), as opposed to valence, could also help understand the relationship between heterogeneity and emotional disclosure. Finally, research could confirm if improved features to effectively manage context collapse: (a) are actually leveraged, and if so, (b) how they may differ based on user characteristics such as self-presentation apprehension (Litt, 2013) or macro variables such as culture.
Acknowledgments
The authors would like to thank Wenjing Pan for her invaluable help with text analysis, and the Associate Editor and reviewers at the Journal of Computer-Mediated Communication for the crucial comments they provided during the process of reviewing this article, which have undoubtedly helped shape its current logic.
Footnotes
An ego network, or egocentric network, refers to a person’s total direct social ties.
MyPersonality data were already collected with explicit user consent and anonymized before our project; no IRB was needed for the present study.
We included number of status updates and number of likes as proxy behavioral measures of SNS activity, which we deleted from the final models given that they did not impact the results.
References
Author notes
Accepted by Nicole Ellison