-
PDF
- Split View
-
Views
-
Cite
Cite
Jorge Hidalgo, Daniela Lourenco, Shogo Tsuruta, Yutaka Masuda, Stephen Miller, Matias Bermann, Andre L S Garcia, Ignacy Misztal, Changes in genomic predictions when new information is added, Journal of Animal Science, Volume 99, Issue 2, February 2021, skab004, https://doi.org/10.1093/jas/skab004
- Share Icon Share
Abstract
The stability of genomic evaluations depends on the amount of data and population parameters. When the dataset is large enough to estimate the value of nearly all independent chromosome segments (~10K in American Angus cattle), the accuracy and persistency of breeding values will be high. The objective of this study was to investigate changes in estimated breeding values (EBV) and genomic EBV (GEBV) across monthly evaluations for 1 yr in a large genotyped population of beef cattle. The American Angus data used included 8.2 million records for birth weight, 8.9 for weaning weight, and 4.4 for postweaning gain. A total of 10.1 million animals born until December 2017 had pedigree information, and 484,074 were genotyped. A truncated dataset included animals born until December 2016. To mimic a scenario with monthly evaluations, 2017 data were added 1 mo at a time to estimate EBV using best linear unbiased prediction (BLUP) and GEBV using single-step genomic BLUP with the algorithm for proven and young (APY) with core group fixed for 1 yr or updated monthly. Predictions from monthly evaluations in 2017 were contrasted with the predictions of the evaluation in December 2016 or the previous month for all genotyped animals born until December 2016 with or without their own phenotypes or progeny phenotypes. Changes in EBV and GEBV were similar across traits, and only results for weaning weight are presented. Correlations between evaluations from December 2016 and the 12 consecutive evaluations were ≥0.97 for EBV and ≥0.99 for GEBV. Average absolute changes for EBV were about two times smaller than for GEBV, except for animals with new progeny phenotypes (≤0.12 and ≤0.11 additive genetic SD [SDa] for EBV and GEBV). The maximum absolute changes for EBV (≤2.95 SDa) were greater than for GEBV (≤1.59 SDa). The average(maximum) absolute GEBV changes for young animals from December 2016 to January and December 2017 ranged from 0.05(0.25) to 0.10(0.53) SDa. Corresponding ranges for animals with new progeny phenotypes were from 0.05(0.88) to 0.11(1.59) SDa for GEBV changes. The average absolute change in EBV(GEBV) from December 2016 to December 2017 for sires with ≤50 progeny phenotypes was 0.26(0.14) and for sires with >50 progeny phenotypes was 0.25(0.16) SDa. Updating the core group in APY without adding data created an average absolute change of 0.07 SDa in GEBV. Genomic evaluations in large genotyped populations are as stable and persistent as the traditional genetic evaluations, with less extreme changes.
Introduction
The stability of genomic evaluations depends on the amount of data and population parameters, provided that no changes are made to the model. When the data are large enough to estimate the value of nearly all independent chromosome segments, the accuracy of genomic predictions will be high (Pocrnic et al., 2019); hence, their persistency and stability will also be high. Independent chromosome segments are DNA regions that are inherited together in linked blocks (Stam, 1980) that are also called linkage disequilibrium blocks (Muir, 2007). The number of blocks that are inherited together depends on the past demographic history, the recombination landscape, the length of the genome (), and the mating structure of the population (Stam, 1980; Slatkin, 2008). The mating structure in finite populations under random mating is represented by the effective population size (), thus the independent chromosome segments can be quantified as 4 (Stam, 1980). In Angus beef cattle populations, the number of independent chromosome segments ranges from 10 to 15k (Pocrnic et al., 2016a). Given two populations with the same number of genotyped animals and phenotypes, the one with smaller would yield genomic predictions of greater accuracy because a lower number of independent chromosome segments would need to be estimated (Misztal et al., 2020a).
Pocrnic et al. (2016b) showed in a simulated dataset that the accuracy of genomic selection was maximized when the genomic estimated breeding values (GEBV) of genotyped animals were conditioned on GEBV from a number of genotyped animals equal to the number of independent chromosome segments (4 ), or equivalently, the number of the largest eigenvalues explaining 98% of the variation in the genomic relationship matrix (G). Using data from the American Angus beef cattle population, Pocrnic et al. (2016a) found that the accuracy of genomic predictions was marginally smaller using the number of the largest eigenvalues explaining 98% instead of 99% or 100% of the variation in G. The number of independent chromosome segments corresponds to the number of core animals in the algorithm for proven and young (APY; Misztal et al., 2014a; Pocrnic et al., 2016b). Pocrnic et al. (2019) reported that accuracies were marginally smaller when using 25% instead of 100% of the optimal number of core animals in APY, suggesting that genomic selection acts on clusters of independent chromosome segments rather than on individual independent chromosome segments. In the same study, the authors also showed that a small amount of phenotypic data allowed only the estimation of the largest clusters (i.e., eigenvalues), and that the four largest clusters explained 10% of the variation in G. This would yield only moderate genomic prediction accuracies; thus, many more phenotypes would be required for additional improvements in accuracy.
Accuracy and possible changes in predictions when more data are added to the evaluation system are both based on the standard error of prediction (Van Vleck, 2016). The larger the amount of data available for an animal, the more stable its prediction is, and the lower the size of changes that may occur. Estimated breeding values (EBV) from the traditional best linear unbiased prediction (BLUP) are very stable even for animals with moderate accuracies. This outcome generated a high level of confidence in this method. The stability of predictions when new data are included is a desirable feature of genomic evaluations for proven animals or animals without new data. However, changes may occur due to limited accuracies, higher number of links between animals through genomic than pedigree relationships, and decay of genomic information across generations. Considering the large amount of phenotypic and genomic data available and the limited dimensionality of the genomic information in the American Angus population (independent chromosome segments = 10,605; = 113), we hypothesized that all independent chromosome segments could be estimated accurately, and the stability of genomic predictions would be high for proven animals or animals without new data.
Genomic evaluations for large genotyped populations based on the single-step genomic BLUP (ssGBLUP) require special algorithms like APY for computational feasibility and efficiency. Recently, Misztal et al. (2019) showed that GEBV can change when the core group is updated, even if no extra data are added, and those changes can be as high as one additive genetic SD (SDa). Such changes happen because the APY relies on recursions of breeding values of noncore on core animals (Misztal et al., 2014a). The recursion formula for noncore animals in APY assumes that a fraction of variation in G (~98%) is due to information and the rest is noise (~2%). The noise is modeled in the formula by an error term, which varies with different random samples of core animals, leading to small changes in GEBV (Misztal et al., 2020b). Keeping the same core animals for a given time is a good strategy to minimize changes in GEBV.
The main objective of this study was to investigate changes in genomic predictions across monthly evaluations for 1 yr to assess the stability of predictions when new animals with phenotypic and genomic information are included in the evaluations. A second objective was to compare the changes in GEBV from adding new data and updating the core group in APY.
Materials and Methods
Animal Care and Use Committee approval was not needed because information was obtained from preexisting databases.
Data
The dataset was provided by the American Angus Association and contained phenotypes for birth weight, weaning weight (N = 8,881,124), and postweaning gain. The pedigree file consisted of 10,129,980 animals born between 1955 and 2017. A total of 484,074 animals had genotypes for 39,774 single nucleotide polymorphisms after quality control. The numbers of animals with genotypes, phenotypes for weaning weight, and pedigree records from December 2016 to December 2017 are presented in Table 1. Most of the data were added between January and April 2017 because the vast majority of calves are born in the spring. Therefore, the 2017 data were added based on the birth date of the animals because this information was more abundant than processing dates. It is important to highlight that the dynamics of the data inclusion in the official database is based on processing dates, and the amount of data added to the database could have a different pattern. The number of animals added to the database from December 2016 to December 2017 was 329,858, of which 91,075 had genomic information and 250,897 had phenotypes for weaning weight.
The number of animals in the pedigree, animals with genotypes, and animals with weaning weight in the American Angus population1
Period . | Pedigree . | Genotypes . | Weaning weight . |
---|---|---|---|
December 2016 | 9.800 | 0.393 | 8.630 |
January 2017 | 9.876 | 0.416 | 8.688 |
February 2017 | 9.965 | 0.443 | 8.763 |
March 2017 | 10.046 | 0.462 | 8.831 |
April 2017 | 10.081 | 0.467 | 8.860 |
May 2017 | 10.092 | 0.469 | 8.868 |
June 2017 | 10.095 | 0.469 | 8.869 |
July 2017 | 10.097 | 0.470 | 8.871 |
August 2017 | 10.104 | 0.474 | 8.874 |
September 2017 | 10.117 | 0.479 | 8.879 |
October 2017 | 10.125 | 0.482 | 8.881 |
November 2017 | 10.128 | 0.483 | 8.881 |
December 2017 | 10.130 | 0.484 | 8.881 |
Period . | Pedigree . | Genotypes . | Weaning weight . |
---|---|---|---|
December 2016 | 9.800 | 0.393 | 8.630 |
January 2017 | 9.876 | 0.416 | 8.688 |
February 2017 | 9.965 | 0.443 | 8.763 |
March 2017 | 10.046 | 0.462 | 8.831 |
April 2017 | 10.081 | 0.467 | 8.860 |
May 2017 | 10.092 | 0.469 | 8.868 |
June 2017 | 10.095 | 0.469 | 8.869 |
July 2017 | 10.097 | 0.470 | 8.871 |
August 2017 | 10.104 | 0.474 | 8.874 |
September 2017 | 10.117 | 0.479 | 8.879 |
October 2017 | 10.125 | 0.482 | 8.881 |
November 2017 | 10.128 | 0.483 | 8.881 |
December 2017 | 10.130 | 0.484 | 8.881 |
1In millions.
The number of animals in the pedigree, animals with genotypes, and animals with weaning weight in the American Angus population1
Period . | Pedigree . | Genotypes . | Weaning weight . |
---|---|---|---|
December 2016 | 9.800 | 0.393 | 8.630 |
January 2017 | 9.876 | 0.416 | 8.688 |
February 2017 | 9.965 | 0.443 | 8.763 |
March 2017 | 10.046 | 0.462 | 8.831 |
April 2017 | 10.081 | 0.467 | 8.860 |
May 2017 | 10.092 | 0.469 | 8.868 |
June 2017 | 10.095 | 0.469 | 8.869 |
July 2017 | 10.097 | 0.470 | 8.871 |
August 2017 | 10.104 | 0.474 | 8.874 |
September 2017 | 10.117 | 0.479 | 8.879 |
October 2017 | 10.125 | 0.482 | 8.881 |
November 2017 | 10.128 | 0.483 | 8.881 |
December 2017 | 10.130 | 0.484 | 8.881 |
Period . | Pedigree . | Genotypes . | Weaning weight . |
---|---|---|---|
December 2016 | 9.800 | 0.393 | 8.630 |
January 2017 | 9.876 | 0.416 | 8.688 |
February 2017 | 9.965 | 0.443 | 8.763 |
March 2017 | 10.046 | 0.462 | 8.831 |
April 2017 | 10.081 | 0.467 | 8.860 |
May 2017 | 10.092 | 0.469 | 8.868 |
June 2017 | 10.095 | 0.469 | 8.869 |
July 2017 | 10.097 | 0.470 | 8.871 |
August 2017 | 10.104 | 0.474 | 8.874 |
September 2017 | 10.117 | 0.479 | 8.879 |
October 2017 | 10.125 | 0.482 | 8.881 |
November 2017 | 10.128 | 0.483 | 8.881 |
December 2017 | 10.130 | 0.484 | 8.881 |
1In millions.
Analyses and computations
The analyses were carried out with the BLUP90IOD2OMP1 program (Misztal et al., 2014b) utilizing a three-trait model used by Angus Genetics Inc. (St. Joseph, MO) for routine genetic evaluations (Lourenco et al., 2015). To compute traditional pedigree-based predictions, the analyses included pedigree and phenotypes, and to compute genomic predictions, the analyses included pedigrees, phenotypes, and genotypes. When genotypes were included, GEBV were computed by ssGBLUP using APY. The G matrix was constructed based on VanRaden (2008), blended with 10% of the pedigree relationship matrix for genotyped animals () to avoid singularity problems, and then rescaled to have the same means of diagonals and off-diagonals as (Vitezica et al., 2011).
To mimic a system with monthly evaluations, phenotypes, genotypes, and pedigree information were added every month, from January to December 2017. We computed traditional BLUP and ssGBLUP evaluations monthly and compared the resulting EBV and GEBV for genotyped animals born until December 2016 with the values obtained in the evaluation from December 2016 or with the values obtained in the evaluation of the previous month. For example, we compared the evaluations obtained with data until May 2017 with the evaluations obtained with data until December 2016 and also with the evaluations obtained with data until April 2017. We computed correlations between EBV in December 2016 and EBV in the 12 monthly evaluations in 2017 as well as correlations between GEBV in December 2016 and GEBV from the 12 monthly evaluations in 2017. In addition, we obtained the distribution of changes for EBV and GEBV when comparing monthly evaluations in 2017 with evaluations in December 2016 and computed average, top 1%, and maximum absolute changes in EBV and GEBV expressed as SDa and kg. Correlations and changes were evaluated separately for six groups of animals: all genotyped animals born until December 2016 (n = 392,999), genotyped animals born until December 2016 with (n = 387,743) and without (n = 5,256) phenotypes, genotyped animals born until December 2016 with (n = 45,848) and without (n = 347,151) new progeny phenotypes after December 2016, and for young genotyped animals born in 2016 with neither phenotypes nor progeny (n = 1,444).
To implement ssGBLUP with APY, two scenarios were used. In the first scenario, 20k genotyped animals from December 2016 dataset were randomly selected to be the core group, and this group remained unchanged in all the genomic evaluations of 2017. In the second scenario, updated core groups were utilized from December 2016 to December 2017, meaning that every month the core was updated considering also the genotyped animals newly added to the database, which means 20k animals were again randomly selected from all the genotyped animals available at that point in time. This second scenario was useful to investigate the impact of adding new data vs. the impact of changing the core group. The comparison between the two evaluations in December 2016 using different core groups was termed contrast zero. The evaluations obtained with fixed and updated core are hereafter referred to as GEBV and GEBV_UC, respectively.
Results and Discussion
Only results for weaning weight are presented here because monthly changes in EBV and GEBV were similar among traits. Correlations between evaluations in December 2016 and the 12 subsequent monthly evaluations in 2017 were greater than or equal to 0.97 for EBV and 0.99 for GEBV. Figure 1a shows the distribution of cumulative changes in EBV for weaning weight between the evaluations in December 2016 and the 12 monthly evaluations in 2017 for the set of all genotyped animals born until December 2016. Similarly, Figure 1b shows the corresponding distribution of cumulative changes in GEBV between these 12 contrasts. The main cumulative changes occurred in the first 4 mo of 2017 because approximately 90% of the phenotypes and genotypes were added during this period (spring calving season; Table 1). An increasing dispersion was observed from January to April (Figure 1 and Table 3). Changes from May to December 2017 were negligible in comparison with the previous months (Figure 1 and Table 4) because substantially less pedigree, phenotypic, and genotypic information were added to the dataset (Table 1).
![Distribution of cumulative changes in EBV (a) and GEBV (b) contrasting the evaluation for weaning weight in December 2016 with subsequent monthly evaluations in 2017 for all genotyped animals (Cx = contrast between December 2016 and month x of 2017; with x varying from 1 [January] to 12 [December]). All changes and statistics are presented in SDa units. The major changes occurred in the first four evaluations due to a considerable increase in the number of phenotypes and genotypes from January to April 2017.](https://oup.silverchair-cdn.com/oup/backfile/Content_public/Journal/jas/99/2/10.1093_jas_skab004/12/m_skab004_fig1.jpeg?Expires=1750420378&Signature=KvYXDJZlBfguVPTB-rW8GtjPZe9A~KMBeVVUgzYxzd7L1vB~kdwQ-fCZZEYC0O-QkRLTjpk2U1PsfHGQd2~Mc61gDFtAFz9PRSJRB48X2YcKOx2fqfFCyXmcAfhRln3S~6niY6xsm~7GZNQugP1d2sT7kN1kfP-BPsI9NxMt2nRGOzne~6g7Jl26SmywgFoSmiF76sbsPoYfI8bWon5BWqt8gk1DV7spMeoe8YdoVfSuGNMVkYpdcFbfPWLTUxUlj2aUcMk8FQsRSDFrKNLGsCV7mgUUGjI8LpoMsHTqRiNGl5-Ft5ed8d3iaH~Xs-ob2jXXdSh5MNnQ~P4DMArkTw__&Key-Pair-Id=APKAIE5G5CRDK6RD3PGA)
Distribution of cumulative changes in EBV (a) and GEBV (b) contrasting the evaluation for weaning weight in December 2016 with subsequent monthly evaluations in 2017 for all genotyped animals (Cx = contrast between December 2016 and month x of 2017; with x varying from 1 [January] to 12 [December]). All changes and statistics are presented in SDa units. The major changes occurred in the first four evaluations due to a considerable increase in the number of phenotypes and genotypes from January to April 2017.
Figure 2 shows average, top 1%, and maximum absolute changes in EBV and GEBV for weaning weight across evaluations for all genotyped animals born until December 2016 in the American Angus population. Table 2 presents average and maximum (in parenthesis) absolute changes between evaluations in December 2016 and evaluations in January 2017 and between evaluations in December 2016 and evaluations in December 2017 for the six comparison groups of genotyped animals born until December 2016. For all the genotyped animals, the average, top 1%, and maximum absolute changes between December 2016 and January 2017 were 0.02, 0.19, and 2.20 SDa for EBV and 0.05, 0.15, and 0.88 SDa for GEBV, respectively (Figure 2). The corresponding average, top 1%, and maximum absolute changes between December 2016 and December 2017 were 0.06, 0.37, and 2.95 SDa for EBV and 0.10, 0.35, and 1.59 SDa for GEBV (Figure 2). As expected, EBV and GEBV absolute changes were larger over a 12-mo period than over a 1-mo period.
Average (maximum) absolute changes in EBV and genomic EBV for weaning weight between December 2016 and January 2017 and between December 2016 and December 2017 for genotyped animals born until 2016 in the American Angus population1
Contrast2 . | Genotyped animals . | |||||
---|---|---|---|---|---|---|
. | All . | With records . | Without records . | With new records after 2016 . | Without new records after 2016 . | Young3 . |
C1_EBV | 0.02 (2.20) | 0.02 (2.20) | 0.01 (0.70) | 0.04 (2.20) | 0.02 (1.15) | 0.02 (0.70) |
C1_GEBV | 0.05 (0.88) | 0.05 (0.88) | 0.04 (0.25) | 0.05 (0.88) | 0.05 (0.45) | 0.05 (0.25) |
C12_EBV | 0.06 (2.95) | 0.06 (2.95) | 0.04 (1.44) | 0.12 (2.95) | 0.05 (1.39) | 0.05 (0.69) |
C12_GEBV | 0.10 (1.59) | 0.10 (1.59) | 0.10 (0.53) | 0.11 (1.59) | 0.10 (0.75) | 0.10 (0.53) |
Contrast2 . | Genotyped animals . | |||||
---|---|---|---|---|---|---|
. | All . | With records . | Without records . | With new records after 2016 . | Without new records after 2016 . | Young3 . |
C1_EBV | 0.02 (2.20) | 0.02 (2.20) | 0.01 (0.70) | 0.04 (2.20) | 0.02 (1.15) | 0.02 (0.70) |
C1_GEBV | 0.05 (0.88) | 0.05 (0.88) | 0.04 (0.25) | 0.05 (0.88) | 0.05 (0.45) | 0.05 (0.25) |
C12_EBV | 0.06 (2.95) | 0.06 (2.95) | 0.04 (1.44) | 0.12 (2.95) | 0.05 (1.39) | 0.05 (0.69) |
C12_GEBV | 0.10 (1.59) | 0.10 (1.59) | 0.10 (0.53) | 0.11 (1.59) | 0.10 (0.75) | 0.10 (0.53) |
1Absolute changes in SDa units.
2C1, contrast between December 2016 and January 2017; C12, contrast between December 2016 and December 2017.
3Born in 2016 with neither phenotypes nor progeny.
Average (maximum) absolute changes in EBV and genomic EBV for weaning weight between December 2016 and January 2017 and between December 2016 and December 2017 for genotyped animals born until 2016 in the American Angus population1
Contrast2 . | Genotyped animals . | |||||
---|---|---|---|---|---|---|
. | All . | With records . | Without records . | With new records after 2016 . | Without new records after 2016 . | Young3 . |
C1_EBV | 0.02 (2.20) | 0.02 (2.20) | 0.01 (0.70) | 0.04 (2.20) | 0.02 (1.15) | 0.02 (0.70) |
C1_GEBV | 0.05 (0.88) | 0.05 (0.88) | 0.04 (0.25) | 0.05 (0.88) | 0.05 (0.45) | 0.05 (0.25) |
C12_EBV | 0.06 (2.95) | 0.06 (2.95) | 0.04 (1.44) | 0.12 (2.95) | 0.05 (1.39) | 0.05 (0.69) |
C12_GEBV | 0.10 (1.59) | 0.10 (1.59) | 0.10 (0.53) | 0.11 (1.59) | 0.10 (0.75) | 0.10 (0.53) |
Contrast2 . | Genotyped animals . | |||||
---|---|---|---|---|---|---|
. | All . | With records . | Without records . | With new records after 2016 . | Without new records after 2016 . | Young3 . |
C1_EBV | 0.02 (2.20) | 0.02 (2.20) | 0.01 (0.70) | 0.04 (2.20) | 0.02 (1.15) | 0.02 (0.70) |
C1_GEBV | 0.05 (0.88) | 0.05 (0.88) | 0.04 (0.25) | 0.05 (0.88) | 0.05 (0.45) | 0.05 (0.25) |
C12_EBV | 0.06 (2.95) | 0.06 (2.95) | 0.04 (1.44) | 0.12 (2.95) | 0.05 (1.39) | 0.05 (0.69) |
C12_GEBV | 0.10 (1.59) | 0.10 (1.59) | 0.10 (0.53) | 0.11 (1.59) | 0.10 (0.75) | 0.10 (0.53) |
1Absolute changes in SDa units.
2C1, contrast between December 2016 and January 2017; C12, contrast between December 2016 and December 2017.
3Born in 2016 with neither phenotypes nor progeny.
![Maximum (Max), top 1% (Top1), and average (Avg) absolute changes in EBV and GEBV contrasting the evaluation for weaning weight in December 2016 with subsequent monthly evaluations in 2017 for all genotyped animals (Cx = contrast between December 2016 and month x of 2017; with x varying from 1 [January] to 12 [December]). All changes and statistics are presented in SDa units. Average absolute changes in GEBV were approximately two times greater than those in EBV, but maximum and top 1% absolute changes were larger in EBV.](https://oup.silverchair-cdn.com/oup/backfile/Content_public/Journal/jas/99/2/10.1093_jas_skab004/12/m_skab004_fig2.jpeg?Expires=1750420378&Signature=THWpHcQ0TTKzuNphPTQ6FagD8wWZ8Iul8nGacKn02sQ0lIrpX6JFItYuQlQ1GKExQUoFuBGzHavAHSv1U~9q0-ZFTTCWwDN1BecU39z1glCxyVKapBAmDNSq9kWNf5yVqVYfwZN~LCx~gIc2PCc1eHxjZvaRc0pgylK0TGwemCnhh9NRCHHd5Dnq4PyVc97-7vT5oatwNjPhbSkPVSjaUAdyDGPT5Jco6GdPVePML3~VF4FCRKeSr7EpDnrMdw970dAnsmeAUDQNt5iEjOR9CFOjbgTYmn2caVeSpq42JhtC6zmol5sC4SuK2xxjmG-5x4a7sW6zJaAdwLBrhkuNLQ__&Key-Pair-Id=APKAIE5G5CRDK6RD3PGA)
Maximum (Max), top 1% (Top1), and average (Avg) absolute changes in EBV and GEBV contrasting the evaluation for weaning weight in December 2016 with subsequent monthly evaluations in 2017 for all genotyped animals (Cx = contrast between December 2016 and month x of 2017; with x varying from 1 [January] to 12 [December]). All changes and statistics are presented in SDa units. Average absolute changes in GEBV were approximately two times greater than those in EBV, but maximum and top 1% absolute changes were larger in EBV.
The average absolute changes for EBV were approximately two times smaller than the average absolute changes for GEBV for all genotyped animals, genotyped animals with and without own records, genotyped animals without new progeny records after December 2016, and genotyped young animals (Table 2). Genotyped animals with new progeny records after December 2016 had similar average absolute changes for EBV and GEBV (Table 2).
The average absolute changes for EBV were greater for the group of animals with new progeny phenotypes added after December 2016, about two times compared with the other groups. Therefore, in this case, the changes were driven by the new phenotypes. The average absolute changes for GEBV were similar across the six comparison groups; thus, in this case, not just the new phenotypes but also the new genotypes were the main forces driving the changes (Tables 2–4). In general, the group of animals with new progeny phenotypes added after December 2016 presented the greater average absolute changes, but, even in this case, and after 1 yr of adding new data, the average absolute changes were small (≤0.12 SDa for EBV and ≤0.11 for GEBV). The maximum absolute changes were always greater for EBV than for GEBV and in line with the theory of outliers in the normal distribution (≤2.59 SDa for EBV and ≤1.95 SDa for GEBV). Lastly, the maximum absolute changes were smaller for young animals, a desirable feature because this group represents selection candidates. The changes in (G)EBV are bounded by the accuracy or precision of the estimates; therefore, smaller maximum changes for GEBV, when compared with EBV, are likely because the genomic information is expected to reduce the prediction error variance (Misztal et al., 2020b). The reason for this is the more accurate estimation of Mendelian sampling effects with genomics (Hayes et al., 2009; Cole and VanRaden, 2011).
Figure 3 shows the distribution of the changes in EBV (a), GEBV_UC (b), and GEBV (c) when contrasting the predictions from December 2016 with those from December 2017 for genotyped sires (n = 7,299) with own weaning weight phenotype and progeny phenotypes. Average absolute changes for sires with 50 or less progeny were 0.26, 0.15, and 0.14 SDa for EBV, GEBV_UC, and GEBV, respectively. Average absolute changes for sires with more than 50 progeny were 0.25, 0.17, and 0.16 SDa, in the same order. Maximum absolute changes in EBV, GEBV_UC, and GEBV were 2.95, 1.62, and 1.59 SDa for sires with 50 or less progeny and 2.11, 1.19, and 1.17 SDa for sires with more than 50 progeny. In general, changes were greater for sires with no or few progeny before December 2016. Proven sires with more than 50 progeny had similar average absolute changes but smaller maximum absolute changes than sires with 50 or less progeny. For this group of sires with own phenotype and progeny phenotypes, the average and maximum absolute changes in GEBV were smaller than in EBV. Additionally, updating the core group in APY generated a marginal increase in the absolute average and maximum changes.
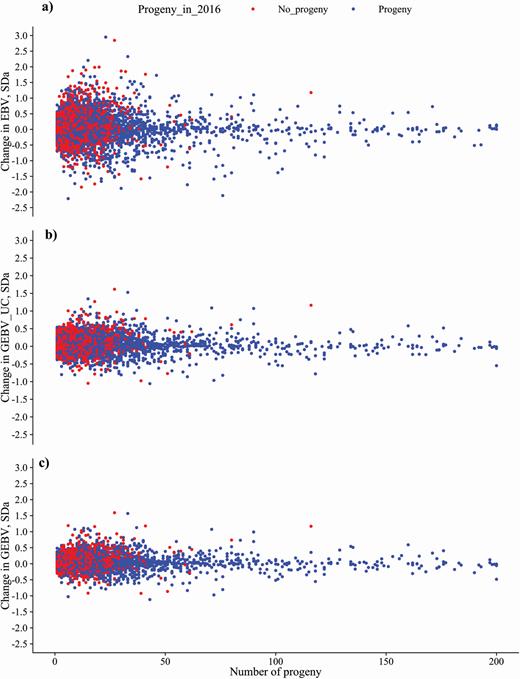
Distribution of changes in EBV (a), GEBV_UC (b), and GEBV (c) as a function of the number of progeny for genotyped sires with own weaning weight phenotype and progeny with weaning weight phenotypes, when contrasting the evaluations for weaning weight in December 2016 with the evaluations in December 2017. Changes are presented in SDa units. Average and maximum absolute changes in GEBV were smaller than those changes in EBV; updating the core group in APY generated a marginal increase in the absolute average and maximum changes.
Looking at the decomposition of EBV and GEBV might help to understand the changes. The EBV can be decomposed into a parental average, a yield deviation, and a progeny contribution (VanRaden and Wiggans, 1991). Additionally, the decomposition of GEBV also includes a direct genomic value and a pedigree prediction, and the last one is needed to avoid double counting of relationships (Aguilar et al., 2010; Lourenco et al., 2015). Without new information for an animal (i.e., new progeny phenotypes), the EBV based on parental average is expected to be stable (assuming that the parents had high accuracy EBV). However, the addition of new progeny phenotypes can result in large changes in EBV for an animal because, in the absence of large progeny groups (i.e., few or no progeny), the additional information is sizeable respective to the parental average. In this case, the magnitude of the change in EBV has a direct relationship with the phenotypic information that is added.
In the case of GEBV, every genotyped animal with phenotypic information influences the direct genomic value of all genotyped animals. Consequently, the GEBV of animals with no additional phenotypic information of their own or their relatives could change. However, if the reference population is large, the accuracy of the direct genomic value will be high (Lourenco et al., 2015); thus, additional phenotypic records would have a lower impact on GEBV because they would contribute with less information than the direct genomic value. In our study, average absolute changes for GEBV in the group of genotyped animals with new progeny records after December 2016 were similar compared with the remaining groups, indicating that the accuracy is high, which was expected because of the large reference population.
For milk yield in Holsteins, the information from the sire and the dam, both with 99% reliability, is equivalent to having 14 daughters with phenotypic records (VanRaden and Wiggans, 1991). With genomic information, the genotype of an animal provides information equivalent to 37.5 daughters for milk yield, 240.6 daughters for daughter pregnancy rate, and 780.2 daughters for heifer conception rate (https://queries.uscdcb.com/eval/summary/comparexml_menu.cfm?R_menu=v_2004.v_Young_Bulls.v_Holstein_wddx#StartBody). For weaning weight in Angus beef cattle, having the genotype of an unproven bull is equivalent to having 27 calves with weaning weight records (https://www.angus.org/AGI/GenomicEnhancedEPDs.pdf).
Genotypes create stronger relationship ties among animals and at the same time reduce the prediction error variance of GEBV as demonstrated by Misztal et al. (2020b) in a research study carried out to investigate the magnitude of changes in relation to accuracy. In the preceding research study, the authors compared two genomic evaluations with the same amount of data but different core groups in APY and concluded that the largest differences were for animals with accuracy lower than 0.7; the animals with greater accuracy (smaller prediction error variance) had considerably smaller changes. In our study, the stronger relationship ties and the reduced prediction error variance resulted in greater average absolute changes in GEBV but lower maximum absolute changes than those from EBV. In the group of animals with new progeny phenotypes after December 2016, the average absolute changes in GEBV were similar to the average absolute changes in EBV, and the maximum absolute changes were smaller for GEBV than those for EBV. Additionally, genotyped sires of progeny with weaning weight phenotypes had smaller changes for GEBV than for EBV, indicating that genomic evaluations were as stable as traditional evaluations and with less extreme changes because of their greater accuracy when animals had new phenotypic information.
An implicit assumption in this study was that the amount of information was sufficient to estimate the values of nearly all independent chromosome segments and their clusters with high accuracy. The predictivity of GEBV (Legarra et al., 2008), calculated as the correlation between phenotypes adjusted for fixed effects (using the whole dataset) and GEBV (estimated using a partial dataset where the phenotypes for validation animals were removed), for animals with weaning weight records and born in 2017 (n = 250,897) was 0.44. Assuming a heritability of weaning weight (0.20), the population accuracy calculated as would be very high (0.98). This formula depends on the heritability of the trait in the validation population and the adjustments to phenotypes. Although the computed accuracy seems overly high, the actual accuracy was likely high, thus explaining the high persistency of GEBV in this study. Hidalgo et al. (2020) found that the heritability for traits under strong genomic selection change over time, which could also lead to changes in GEBV accuracies. Thus, validation methods that do not explicitly depend on heritabilities (e.g., linear regression [LR] validation from Legarra and Reverter, 2018) may provide better estimates of GEBV accuracies. The LR method assumes that the additive genetic variance for the subset of validation animals is known. This additive genetic variance can be computed and is smaller than the population-based estimate in populations undergoing selection; however, its computation is intricate and requires a special method based on Gibbs Sampling (Sorensen et al., 2001). The implementation of this method is out of the scope of this research study and deserves future research.
Table 3 presents the average and maximum absolute changes in EBV, GEBV, and GEBV_UC (i.e., updated core) for all genotyped animals born until December 2016, those that had new progeny records added after December 2016, and the young ones. All changes are shown in SDa and in kg, but discussions are in SDa for simplicity. The contrast zero represents absolute changes between initial evaluations in December 2016 and evaluations in December 2016 with updated core. The contrasts 1 to 12 represent absolute changes among evaluations in December 2016 and evaluations from January to December 2017 adding data, and either with fixed or updated core.
Average and maximum absolute changes in EBV and genomic EBV for weaning weight among evaluations in December 2016 and evaluations from January to December 2017 for genotyped animals born until December 2016 in the American Angus population1
. | . | Average . | Maximum . | ||||||||||
---|---|---|---|---|---|---|---|---|---|---|---|---|---|
Group . | Contrast2 . | EBV . | GEBV . | GEBV_UC3 . | EBV . | GEBV . | GEBV_UC . | ||||||
All | C0 | — | — | — | — | 0.07 | (0.71) | — | — | — | — | 0.76 | (7.97) |
C1 | 0.02 | (0.22) | 0.05 | (0.48) | 0.08 | (0.86) | 2.20 | (23.12) | 0.88 | (9.21) | 1.08 | (11.35) | |
C2 | 0.04 | (0.38) | 0.07 | (0.77) | 0.10 | (1.05) | 2.81 | (29.54) | 1.53 | (16.08) | 1.48 | (15.56) | |
C3 | 0.05 | (0.49) | 0.09 | (0.94) | 0.11 | (1.19) | 2.71 | (28.49) | 1.54 | (16.19) | 1.51 | (15.87) | |
C4 | 0.05 | (0.54) | 0.10 | (1.00) | 0.12 | (1.23) | 2.84 | (29.85) | 1.60 | (16.82) | 1.67 | (17.55) | |
C5 | 0.05 | (0.55) | 0.10 | (1.02) | 0.12 | (1.25) | 2.84 | (29.85) | 1.60 | (16.82) | 1.51 | (15.87) | |
C6 | 0.05 | (0.55) | 0.10 | (1.02) | 0.12 | (1.25) | 2.95 | (31.01) | 1.60 | (16.82) | 1.55 | (16.29) | |
C7 | 0.05 | (0.56) | 0.10 | (1.02) | 0.12 | (1.25) | 2.95 | (31.01) | 1.60 | (16.82) | 1.54 | (16.19) | |
C8 | 0.05 | (0.57) | 0.10 | (1.03) | 0.12 | (1.26) | 2.96 | (31.11) | 1.59 | (16.71) | 1.62 | (17.03) | |
C9 | 0.05 | (0.57) | 0.10 | (1.05) | 0.12 | (1.26) | 2.95 | (31.01) | 1.59 | (16.71) | 1.58 | (16.61) | |
C10 | 0.06 | (0.58) | 0.10 | (1.05) | 0.12 | (1.27) | 2.95 | (31.01) | 1.59 | (16.71) | 1.62 | (17.03) | |
C11 | 0.06 | (0.58) | 0.10 | (1.05) | 0.12 | (1.27) | 2.95 | (31.01) | 1.59 | (16.71) | 1.61 | (16.92) | |
C12 | 0.06 | (0.58) | 0.10 | (1.05) | 0.12 | (1.27) | 2.95 | (31.01) | 1.59 | (16.71) | 1.62 | (17.03) | |
New progeny records | C0 | — | — | — | — | 0.07 | (0.70) | — | — | — | — | 0.69 | (7.23) |
C1 | 0.04 | (0.41) | 0.05 | (0.48) | 0.08 | (0.87) | 2.20 | (23.12) | 0.88 | (9.21) | 0.88 | (9.22) | |
C2 | 0.07 | (0.78) | 0.08 | (0.80) | 0.10 | (1.07) | 2.81 | (29.54) | 1.53 | (16.08) | 1.48 | (15.56) | |
C3 | 0.10 | (1.05) | 0.10 | (1.01) | 0.12 | (1.24) | 2.71 | (28.49) | 1.54 | (16.19) | 1.51 | (15.87) | |
C4 | 0.11 | (1.16) | 0.10 | (1.08) | 0.12 | (1.29) | 2.84 | (29.85) | 1.60 | (16.82) | 1.67 | (17.55) | |
C5 | 0.11 | (1.19) | 0.11 | (1.10) | 0.13 | (1.31) | 2.84 | (29.85) | 1.60 | (16.82) | 1.51 | (15.87) | |
C6 | 0.11 | (1.19) | 0.11 | (1.10) | 0.13 | (1.31) | 2.95 | (31.01) | 1.60 | (16.82) | 1.55 | (16.29) | |
C7 | 0.11 | (1.20) | 0.11 | (1.10) | 0.13 | (1.32) | 2.95 | (31.01) | 1.60 | (16.82) | 1.54 | (16.19) | |
C8 | 0.12 | (1.23) | 0.11 | (1.11) | 0.13 | (1.33) | 2.96 | (31.11) | 1.59 | (16.71) | 1.62 | (17.03) | |
C9 | 0.12 | (1.26) | 0.11 | (1.14) | 0.13 | (1.33) | 2.95 | (31.01) | 1.59 | (16.71) | 1.58 | (16.61) | |
C10 | 0.12 | (1.28) | 0.11 | (1.15) | 0.13 | (1.35) | 2.95 | (31.01) | 1.59 | (16.71) | 1.62 | (17.03) | |
C11 | 0.12 | (1.29) | 0.11 | (1.15) | 0.13 | (1.36) | 2.95 | (31.01) | 1.59 | (16.71) | 1.61 | (16.92) | |
C12 | 0.12 | (1.29) | 0.11 | (1.15) | 0.13 | (1.36) | 2.95 | (31.01) | 1.59 | (16.71) | 1.62 | (17.03) | |
Young4 | C0 | — | — | — | — | 0.07 | (0.73) | — | — | — | — | 0.62 | (6.54) |
C1 | 0.02 | (0.21) | 0.05 | (0.50) | 0.08 | (0.88) | 0.70 | (7.31) | 0.25 | (2.59) | 0.37 | (3.87) | |
C2 | 0.04 | (0.37) | 0.08 | (0.81) | 0.10 | (1.09) | 0.71 | (7.44) | 0.36 | (3.73) | 0.47 | (4.97) | |
C3 | 0.05 | (0.48) | 0.09 | (0.99) | 0.12 | (1.23) | 0.69 | (7.23) | 0.52 | (5.41) | 0.69 | (7.25) | |
C4 | 0.05 | (0.53) | 0.10 | (1.03) | 0.12 | (1.25) | 0.69 | (7.25) | 0.55 | (5.77) | 0.75 | (7.90) | |
C5 | 0.05 | (0.53) | 0.10 | (1.04) | 0.12 | (1.24) | 0.69 | (7.25) | 0.54 | (5.63) | 0.73 | (7.63) | |
C6 | 0.05 | (0.53) | 0.10 | (1.04) | 0.12 | (1.25) | 0.69 | (7.25) | 0.53 | (5.54) | 0.57 | (5.95) | |
C7 | 0.05 | (0.53) | 0.10 | (1.05) | 0.12 | (1.25) | 0.69 | (7.27) | 0.52 | (5.51) | 0.60 | (6.32) | |
C8 | 0.05 | (0.53) | 0.10 | (1.05) | 0.12 | (1.26) | 0.69 | (7.28) | 0.52 | (5.41) | 0.59 | (6.16) | |
C9 | 0.05 | (0.54) | 0.10 | (1.07) | 0.12 | (1.25) | 0.69 | (7.28) | 0.53 | (5.53) | 0.64 | (6.67) | |
C10 | 0.05 | (0.54) | 0.10 | (1.08) | 0.12 | (1.26) | 0.69 | (7.22) | 0.53 | (5.56) | 0.55 | (5.77) | |
C11 | 0.05 | (0.54) | 0.10 | (1.07) | 0.12 | (1.28) | 0.69 | (7.22) | 0.53 | (5.58) | 0.62 | (6.50) | |
C12 | 0.05 | (0.54) | 0.10 | (1.08) | 0.12 | (1.29) | 0.69 | (7.22) | 0.53 | (5.55) | 0.77 | (8.06) |
. | . | Average . | Maximum . | ||||||||||
---|---|---|---|---|---|---|---|---|---|---|---|---|---|
Group . | Contrast2 . | EBV . | GEBV . | GEBV_UC3 . | EBV . | GEBV . | GEBV_UC . | ||||||
All | C0 | — | — | — | — | 0.07 | (0.71) | — | — | — | — | 0.76 | (7.97) |
C1 | 0.02 | (0.22) | 0.05 | (0.48) | 0.08 | (0.86) | 2.20 | (23.12) | 0.88 | (9.21) | 1.08 | (11.35) | |
C2 | 0.04 | (0.38) | 0.07 | (0.77) | 0.10 | (1.05) | 2.81 | (29.54) | 1.53 | (16.08) | 1.48 | (15.56) | |
C3 | 0.05 | (0.49) | 0.09 | (0.94) | 0.11 | (1.19) | 2.71 | (28.49) | 1.54 | (16.19) | 1.51 | (15.87) | |
C4 | 0.05 | (0.54) | 0.10 | (1.00) | 0.12 | (1.23) | 2.84 | (29.85) | 1.60 | (16.82) | 1.67 | (17.55) | |
C5 | 0.05 | (0.55) | 0.10 | (1.02) | 0.12 | (1.25) | 2.84 | (29.85) | 1.60 | (16.82) | 1.51 | (15.87) | |
C6 | 0.05 | (0.55) | 0.10 | (1.02) | 0.12 | (1.25) | 2.95 | (31.01) | 1.60 | (16.82) | 1.55 | (16.29) | |
C7 | 0.05 | (0.56) | 0.10 | (1.02) | 0.12 | (1.25) | 2.95 | (31.01) | 1.60 | (16.82) | 1.54 | (16.19) | |
C8 | 0.05 | (0.57) | 0.10 | (1.03) | 0.12 | (1.26) | 2.96 | (31.11) | 1.59 | (16.71) | 1.62 | (17.03) | |
C9 | 0.05 | (0.57) | 0.10 | (1.05) | 0.12 | (1.26) | 2.95 | (31.01) | 1.59 | (16.71) | 1.58 | (16.61) | |
C10 | 0.06 | (0.58) | 0.10 | (1.05) | 0.12 | (1.27) | 2.95 | (31.01) | 1.59 | (16.71) | 1.62 | (17.03) | |
C11 | 0.06 | (0.58) | 0.10 | (1.05) | 0.12 | (1.27) | 2.95 | (31.01) | 1.59 | (16.71) | 1.61 | (16.92) | |
C12 | 0.06 | (0.58) | 0.10 | (1.05) | 0.12 | (1.27) | 2.95 | (31.01) | 1.59 | (16.71) | 1.62 | (17.03) | |
New progeny records | C0 | — | — | — | — | 0.07 | (0.70) | — | — | — | — | 0.69 | (7.23) |
C1 | 0.04 | (0.41) | 0.05 | (0.48) | 0.08 | (0.87) | 2.20 | (23.12) | 0.88 | (9.21) | 0.88 | (9.22) | |
C2 | 0.07 | (0.78) | 0.08 | (0.80) | 0.10 | (1.07) | 2.81 | (29.54) | 1.53 | (16.08) | 1.48 | (15.56) | |
C3 | 0.10 | (1.05) | 0.10 | (1.01) | 0.12 | (1.24) | 2.71 | (28.49) | 1.54 | (16.19) | 1.51 | (15.87) | |
C4 | 0.11 | (1.16) | 0.10 | (1.08) | 0.12 | (1.29) | 2.84 | (29.85) | 1.60 | (16.82) | 1.67 | (17.55) | |
C5 | 0.11 | (1.19) | 0.11 | (1.10) | 0.13 | (1.31) | 2.84 | (29.85) | 1.60 | (16.82) | 1.51 | (15.87) | |
C6 | 0.11 | (1.19) | 0.11 | (1.10) | 0.13 | (1.31) | 2.95 | (31.01) | 1.60 | (16.82) | 1.55 | (16.29) | |
C7 | 0.11 | (1.20) | 0.11 | (1.10) | 0.13 | (1.32) | 2.95 | (31.01) | 1.60 | (16.82) | 1.54 | (16.19) | |
C8 | 0.12 | (1.23) | 0.11 | (1.11) | 0.13 | (1.33) | 2.96 | (31.11) | 1.59 | (16.71) | 1.62 | (17.03) | |
C9 | 0.12 | (1.26) | 0.11 | (1.14) | 0.13 | (1.33) | 2.95 | (31.01) | 1.59 | (16.71) | 1.58 | (16.61) | |
C10 | 0.12 | (1.28) | 0.11 | (1.15) | 0.13 | (1.35) | 2.95 | (31.01) | 1.59 | (16.71) | 1.62 | (17.03) | |
C11 | 0.12 | (1.29) | 0.11 | (1.15) | 0.13 | (1.36) | 2.95 | (31.01) | 1.59 | (16.71) | 1.61 | (16.92) | |
C12 | 0.12 | (1.29) | 0.11 | (1.15) | 0.13 | (1.36) | 2.95 | (31.01) | 1.59 | (16.71) | 1.62 | (17.03) | |
Young4 | C0 | — | — | — | — | 0.07 | (0.73) | — | — | — | — | 0.62 | (6.54) |
C1 | 0.02 | (0.21) | 0.05 | (0.50) | 0.08 | (0.88) | 0.70 | (7.31) | 0.25 | (2.59) | 0.37 | (3.87) | |
C2 | 0.04 | (0.37) | 0.08 | (0.81) | 0.10 | (1.09) | 0.71 | (7.44) | 0.36 | (3.73) | 0.47 | (4.97) | |
C3 | 0.05 | (0.48) | 0.09 | (0.99) | 0.12 | (1.23) | 0.69 | (7.23) | 0.52 | (5.41) | 0.69 | (7.25) | |
C4 | 0.05 | (0.53) | 0.10 | (1.03) | 0.12 | (1.25) | 0.69 | (7.25) | 0.55 | (5.77) | 0.75 | (7.90) | |
C5 | 0.05 | (0.53) | 0.10 | (1.04) | 0.12 | (1.24) | 0.69 | (7.25) | 0.54 | (5.63) | 0.73 | (7.63) | |
C6 | 0.05 | (0.53) | 0.10 | (1.04) | 0.12 | (1.25) | 0.69 | (7.25) | 0.53 | (5.54) | 0.57 | (5.95) | |
C7 | 0.05 | (0.53) | 0.10 | (1.05) | 0.12 | (1.25) | 0.69 | (7.27) | 0.52 | (5.51) | 0.60 | (6.32) | |
C8 | 0.05 | (0.53) | 0.10 | (1.05) | 0.12 | (1.26) | 0.69 | (7.28) | 0.52 | (5.41) | 0.59 | (6.16) | |
C9 | 0.05 | (0.54) | 0.10 | (1.07) | 0.12 | (1.25) | 0.69 | (7.28) | 0.53 | (5.53) | 0.64 | (6.67) | |
C10 | 0.05 | (0.54) | 0.10 | (1.08) | 0.12 | (1.26) | 0.69 | (7.22) | 0.53 | (5.56) | 0.55 | (5.77) | |
C11 | 0.05 | (0.54) | 0.10 | (1.07) | 0.12 | (1.28) | 0.69 | (7.22) | 0.53 | (5.58) | 0.62 | (6.50) | |
C12 | 0.05 | (0.54) | 0.10 | (1.08) | 0.12 | (1.29) | 0.69 | (7.22) | 0.53 | (5.55) | 0.77 | (8.06) |
1Absolute changes in additive genetic standard deviation units (in kg inside the parenthesis).
2C0, contrast between two evaluations in December of 2016 with different core; Cx, contrast between December of 2016 and month x of 2017; with x varying from 1 (January) to 12 (December).
3GEBV_UC, GEBV from a scenario where the core set is updated every month.
4Born in 2016 with neither phenotypes nor progeny.
Average and maximum absolute changes in EBV and genomic EBV for weaning weight among evaluations in December 2016 and evaluations from January to December 2017 for genotyped animals born until December 2016 in the American Angus population1
. | . | Average . | Maximum . | ||||||||||
---|---|---|---|---|---|---|---|---|---|---|---|---|---|
Group . | Contrast2 . | EBV . | GEBV . | GEBV_UC3 . | EBV . | GEBV . | GEBV_UC . | ||||||
All | C0 | — | — | — | — | 0.07 | (0.71) | — | — | — | — | 0.76 | (7.97) |
C1 | 0.02 | (0.22) | 0.05 | (0.48) | 0.08 | (0.86) | 2.20 | (23.12) | 0.88 | (9.21) | 1.08 | (11.35) | |
C2 | 0.04 | (0.38) | 0.07 | (0.77) | 0.10 | (1.05) | 2.81 | (29.54) | 1.53 | (16.08) | 1.48 | (15.56) | |
C3 | 0.05 | (0.49) | 0.09 | (0.94) | 0.11 | (1.19) | 2.71 | (28.49) | 1.54 | (16.19) | 1.51 | (15.87) | |
C4 | 0.05 | (0.54) | 0.10 | (1.00) | 0.12 | (1.23) | 2.84 | (29.85) | 1.60 | (16.82) | 1.67 | (17.55) | |
C5 | 0.05 | (0.55) | 0.10 | (1.02) | 0.12 | (1.25) | 2.84 | (29.85) | 1.60 | (16.82) | 1.51 | (15.87) | |
C6 | 0.05 | (0.55) | 0.10 | (1.02) | 0.12 | (1.25) | 2.95 | (31.01) | 1.60 | (16.82) | 1.55 | (16.29) | |
C7 | 0.05 | (0.56) | 0.10 | (1.02) | 0.12 | (1.25) | 2.95 | (31.01) | 1.60 | (16.82) | 1.54 | (16.19) | |
C8 | 0.05 | (0.57) | 0.10 | (1.03) | 0.12 | (1.26) | 2.96 | (31.11) | 1.59 | (16.71) | 1.62 | (17.03) | |
C9 | 0.05 | (0.57) | 0.10 | (1.05) | 0.12 | (1.26) | 2.95 | (31.01) | 1.59 | (16.71) | 1.58 | (16.61) | |
C10 | 0.06 | (0.58) | 0.10 | (1.05) | 0.12 | (1.27) | 2.95 | (31.01) | 1.59 | (16.71) | 1.62 | (17.03) | |
C11 | 0.06 | (0.58) | 0.10 | (1.05) | 0.12 | (1.27) | 2.95 | (31.01) | 1.59 | (16.71) | 1.61 | (16.92) | |
C12 | 0.06 | (0.58) | 0.10 | (1.05) | 0.12 | (1.27) | 2.95 | (31.01) | 1.59 | (16.71) | 1.62 | (17.03) | |
New progeny records | C0 | — | — | — | — | 0.07 | (0.70) | — | — | — | — | 0.69 | (7.23) |
C1 | 0.04 | (0.41) | 0.05 | (0.48) | 0.08 | (0.87) | 2.20 | (23.12) | 0.88 | (9.21) | 0.88 | (9.22) | |
C2 | 0.07 | (0.78) | 0.08 | (0.80) | 0.10 | (1.07) | 2.81 | (29.54) | 1.53 | (16.08) | 1.48 | (15.56) | |
C3 | 0.10 | (1.05) | 0.10 | (1.01) | 0.12 | (1.24) | 2.71 | (28.49) | 1.54 | (16.19) | 1.51 | (15.87) | |
C4 | 0.11 | (1.16) | 0.10 | (1.08) | 0.12 | (1.29) | 2.84 | (29.85) | 1.60 | (16.82) | 1.67 | (17.55) | |
C5 | 0.11 | (1.19) | 0.11 | (1.10) | 0.13 | (1.31) | 2.84 | (29.85) | 1.60 | (16.82) | 1.51 | (15.87) | |
C6 | 0.11 | (1.19) | 0.11 | (1.10) | 0.13 | (1.31) | 2.95 | (31.01) | 1.60 | (16.82) | 1.55 | (16.29) | |
C7 | 0.11 | (1.20) | 0.11 | (1.10) | 0.13 | (1.32) | 2.95 | (31.01) | 1.60 | (16.82) | 1.54 | (16.19) | |
C8 | 0.12 | (1.23) | 0.11 | (1.11) | 0.13 | (1.33) | 2.96 | (31.11) | 1.59 | (16.71) | 1.62 | (17.03) | |
C9 | 0.12 | (1.26) | 0.11 | (1.14) | 0.13 | (1.33) | 2.95 | (31.01) | 1.59 | (16.71) | 1.58 | (16.61) | |
C10 | 0.12 | (1.28) | 0.11 | (1.15) | 0.13 | (1.35) | 2.95 | (31.01) | 1.59 | (16.71) | 1.62 | (17.03) | |
C11 | 0.12 | (1.29) | 0.11 | (1.15) | 0.13 | (1.36) | 2.95 | (31.01) | 1.59 | (16.71) | 1.61 | (16.92) | |
C12 | 0.12 | (1.29) | 0.11 | (1.15) | 0.13 | (1.36) | 2.95 | (31.01) | 1.59 | (16.71) | 1.62 | (17.03) | |
Young4 | C0 | — | — | — | — | 0.07 | (0.73) | — | — | — | — | 0.62 | (6.54) |
C1 | 0.02 | (0.21) | 0.05 | (0.50) | 0.08 | (0.88) | 0.70 | (7.31) | 0.25 | (2.59) | 0.37 | (3.87) | |
C2 | 0.04 | (0.37) | 0.08 | (0.81) | 0.10 | (1.09) | 0.71 | (7.44) | 0.36 | (3.73) | 0.47 | (4.97) | |
C3 | 0.05 | (0.48) | 0.09 | (0.99) | 0.12 | (1.23) | 0.69 | (7.23) | 0.52 | (5.41) | 0.69 | (7.25) | |
C4 | 0.05 | (0.53) | 0.10 | (1.03) | 0.12 | (1.25) | 0.69 | (7.25) | 0.55 | (5.77) | 0.75 | (7.90) | |
C5 | 0.05 | (0.53) | 0.10 | (1.04) | 0.12 | (1.24) | 0.69 | (7.25) | 0.54 | (5.63) | 0.73 | (7.63) | |
C6 | 0.05 | (0.53) | 0.10 | (1.04) | 0.12 | (1.25) | 0.69 | (7.25) | 0.53 | (5.54) | 0.57 | (5.95) | |
C7 | 0.05 | (0.53) | 0.10 | (1.05) | 0.12 | (1.25) | 0.69 | (7.27) | 0.52 | (5.51) | 0.60 | (6.32) | |
C8 | 0.05 | (0.53) | 0.10 | (1.05) | 0.12 | (1.26) | 0.69 | (7.28) | 0.52 | (5.41) | 0.59 | (6.16) | |
C9 | 0.05 | (0.54) | 0.10 | (1.07) | 0.12 | (1.25) | 0.69 | (7.28) | 0.53 | (5.53) | 0.64 | (6.67) | |
C10 | 0.05 | (0.54) | 0.10 | (1.08) | 0.12 | (1.26) | 0.69 | (7.22) | 0.53 | (5.56) | 0.55 | (5.77) | |
C11 | 0.05 | (0.54) | 0.10 | (1.07) | 0.12 | (1.28) | 0.69 | (7.22) | 0.53 | (5.58) | 0.62 | (6.50) | |
C12 | 0.05 | (0.54) | 0.10 | (1.08) | 0.12 | (1.29) | 0.69 | (7.22) | 0.53 | (5.55) | 0.77 | (8.06) |
. | . | Average . | Maximum . | ||||||||||
---|---|---|---|---|---|---|---|---|---|---|---|---|---|
Group . | Contrast2 . | EBV . | GEBV . | GEBV_UC3 . | EBV . | GEBV . | GEBV_UC . | ||||||
All | C0 | — | — | — | — | 0.07 | (0.71) | — | — | — | — | 0.76 | (7.97) |
C1 | 0.02 | (0.22) | 0.05 | (0.48) | 0.08 | (0.86) | 2.20 | (23.12) | 0.88 | (9.21) | 1.08 | (11.35) | |
C2 | 0.04 | (0.38) | 0.07 | (0.77) | 0.10 | (1.05) | 2.81 | (29.54) | 1.53 | (16.08) | 1.48 | (15.56) | |
C3 | 0.05 | (0.49) | 0.09 | (0.94) | 0.11 | (1.19) | 2.71 | (28.49) | 1.54 | (16.19) | 1.51 | (15.87) | |
C4 | 0.05 | (0.54) | 0.10 | (1.00) | 0.12 | (1.23) | 2.84 | (29.85) | 1.60 | (16.82) | 1.67 | (17.55) | |
C5 | 0.05 | (0.55) | 0.10 | (1.02) | 0.12 | (1.25) | 2.84 | (29.85) | 1.60 | (16.82) | 1.51 | (15.87) | |
C6 | 0.05 | (0.55) | 0.10 | (1.02) | 0.12 | (1.25) | 2.95 | (31.01) | 1.60 | (16.82) | 1.55 | (16.29) | |
C7 | 0.05 | (0.56) | 0.10 | (1.02) | 0.12 | (1.25) | 2.95 | (31.01) | 1.60 | (16.82) | 1.54 | (16.19) | |
C8 | 0.05 | (0.57) | 0.10 | (1.03) | 0.12 | (1.26) | 2.96 | (31.11) | 1.59 | (16.71) | 1.62 | (17.03) | |
C9 | 0.05 | (0.57) | 0.10 | (1.05) | 0.12 | (1.26) | 2.95 | (31.01) | 1.59 | (16.71) | 1.58 | (16.61) | |
C10 | 0.06 | (0.58) | 0.10 | (1.05) | 0.12 | (1.27) | 2.95 | (31.01) | 1.59 | (16.71) | 1.62 | (17.03) | |
C11 | 0.06 | (0.58) | 0.10 | (1.05) | 0.12 | (1.27) | 2.95 | (31.01) | 1.59 | (16.71) | 1.61 | (16.92) | |
C12 | 0.06 | (0.58) | 0.10 | (1.05) | 0.12 | (1.27) | 2.95 | (31.01) | 1.59 | (16.71) | 1.62 | (17.03) | |
New progeny records | C0 | — | — | — | — | 0.07 | (0.70) | — | — | — | — | 0.69 | (7.23) |
C1 | 0.04 | (0.41) | 0.05 | (0.48) | 0.08 | (0.87) | 2.20 | (23.12) | 0.88 | (9.21) | 0.88 | (9.22) | |
C2 | 0.07 | (0.78) | 0.08 | (0.80) | 0.10 | (1.07) | 2.81 | (29.54) | 1.53 | (16.08) | 1.48 | (15.56) | |
C3 | 0.10 | (1.05) | 0.10 | (1.01) | 0.12 | (1.24) | 2.71 | (28.49) | 1.54 | (16.19) | 1.51 | (15.87) | |
C4 | 0.11 | (1.16) | 0.10 | (1.08) | 0.12 | (1.29) | 2.84 | (29.85) | 1.60 | (16.82) | 1.67 | (17.55) | |
C5 | 0.11 | (1.19) | 0.11 | (1.10) | 0.13 | (1.31) | 2.84 | (29.85) | 1.60 | (16.82) | 1.51 | (15.87) | |
C6 | 0.11 | (1.19) | 0.11 | (1.10) | 0.13 | (1.31) | 2.95 | (31.01) | 1.60 | (16.82) | 1.55 | (16.29) | |
C7 | 0.11 | (1.20) | 0.11 | (1.10) | 0.13 | (1.32) | 2.95 | (31.01) | 1.60 | (16.82) | 1.54 | (16.19) | |
C8 | 0.12 | (1.23) | 0.11 | (1.11) | 0.13 | (1.33) | 2.96 | (31.11) | 1.59 | (16.71) | 1.62 | (17.03) | |
C9 | 0.12 | (1.26) | 0.11 | (1.14) | 0.13 | (1.33) | 2.95 | (31.01) | 1.59 | (16.71) | 1.58 | (16.61) | |
C10 | 0.12 | (1.28) | 0.11 | (1.15) | 0.13 | (1.35) | 2.95 | (31.01) | 1.59 | (16.71) | 1.62 | (17.03) | |
C11 | 0.12 | (1.29) | 0.11 | (1.15) | 0.13 | (1.36) | 2.95 | (31.01) | 1.59 | (16.71) | 1.61 | (16.92) | |
C12 | 0.12 | (1.29) | 0.11 | (1.15) | 0.13 | (1.36) | 2.95 | (31.01) | 1.59 | (16.71) | 1.62 | (17.03) | |
Young4 | C0 | — | — | — | — | 0.07 | (0.73) | — | — | — | — | 0.62 | (6.54) |
C1 | 0.02 | (0.21) | 0.05 | (0.50) | 0.08 | (0.88) | 0.70 | (7.31) | 0.25 | (2.59) | 0.37 | (3.87) | |
C2 | 0.04 | (0.37) | 0.08 | (0.81) | 0.10 | (1.09) | 0.71 | (7.44) | 0.36 | (3.73) | 0.47 | (4.97) | |
C3 | 0.05 | (0.48) | 0.09 | (0.99) | 0.12 | (1.23) | 0.69 | (7.23) | 0.52 | (5.41) | 0.69 | (7.25) | |
C4 | 0.05 | (0.53) | 0.10 | (1.03) | 0.12 | (1.25) | 0.69 | (7.25) | 0.55 | (5.77) | 0.75 | (7.90) | |
C5 | 0.05 | (0.53) | 0.10 | (1.04) | 0.12 | (1.24) | 0.69 | (7.25) | 0.54 | (5.63) | 0.73 | (7.63) | |
C6 | 0.05 | (0.53) | 0.10 | (1.04) | 0.12 | (1.25) | 0.69 | (7.25) | 0.53 | (5.54) | 0.57 | (5.95) | |
C7 | 0.05 | (0.53) | 0.10 | (1.05) | 0.12 | (1.25) | 0.69 | (7.27) | 0.52 | (5.51) | 0.60 | (6.32) | |
C8 | 0.05 | (0.53) | 0.10 | (1.05) | 0.12 | (1.26) | 0.69 | (7.28) | 0.52 | (5.41) | 0.59 | (6.16) | |
C9 | 0.05 | (0.54) | 0.10 | (1.07) | 0.12 | (1.25) | 0.69 | (7.28) | 0.53 | (5.53) | 0.64 | (6.67) | |
C10 | 0.05 | (0.54) | 0.10 | (1.08) | 0.12 | (1.26) | 0.69 | (7.22) | 0.53 | (5.56) | 0.55 | (5.77) | |
C11 | 0.05 | (0.54) | 0.10 | (1.07) | 0.12 | (1.28) | 0.69 | (7.22) | 0.53 | (5.58) | 0.62 | (6.50) | |
C12 | 0.05 | (0.54) | 0.10 | (1.08) | 0.12 | (1.29) | 0.69 | (7.22) | 0.53 | (5.55) | 0.77 | (8.06) |
1Absolute changes in additive genetic standard deviation units (in kg inside the parenthesis).
2C0, contrast between two evaluations in December of 2016 with different core; Cx, contrast between December of 2016 and month x of 2017; with x varying from 1 (January) to 12 (December).
3GEBV_UC, GEBV from a scenario where the core set is updated every month.
4Born in 2016 with neither phenotypes nor progeny.
The average absolute changes updating the core group for APY and adding a new pedigree, genotypic, and phenotypic information (contrasts one to twelve) were about 0.02 SDa greater than with the fixed core group. The maximum absolute changes were similar for the groups of all genotyped animals born until December 2016 and those that had new progeny records added after December 2016 but about 0.12 SDa greater for young animals when compared with using a fixed core group. The average absolute changes for contrast zero were 0.07 for all groups of animals, which was similar to those changes in contrast two with fixed core group. This means that the impact of changing the core animals in the estimation of GEBV is the same as the impact of adding 2 mo of data, but keeping the same core, it is important to highlight that we refer to those months with the majority of data. Therefore, the changes in GEBV because of core updating may have more impact when no data or a small amount of data is added from one evaluation to the next. To avoid larger changes, the core group can be fixed for a period of time, that is, 1 yr, and updates should be done when large amounts of data are added to the evaluation system.
Table 4 contains average and maximum absolute changes in EBV, GEBV, and GEBV_UC for all genotyped animals born until December 2016, those that had new progeny records added after December 2016, and the young ones. All changes are shown in SDa and in kg, but discussions are in SDa for simplicity. The contrasts 1 to 12 represent absolute changes among evaluations of 2017 in the current and the precedent month. The largest average absolute changes in both EBV and GEBV were observed in the first four contrasts (from January to April 2017) and were marginal afterward. The maximum absolute changes were larger in the first four contrasts and decreased afterward, as expected, because less information was added from May to December 2017. Updating the core group in APY caused an average absolute change in GEBV of ~0.7 SDa. The maximum absolute changes for GEBV updating the core group in APY ranged from 0.61 to 1.17 SDa for all the genotyped animals and those with new progeny phenotypes. Maximum absolute changes were smaller for the group of young animals (~0.4 SDa).
Average and maximum absolute changes in EBV and genomic EBV for weaning weight among monthly evaluations in 2017 and evaluations in the previous month for genotyped animals born until December 2016 in the American Angus population1
. | . | Average . | Maximum . | ||||||||||
---|---|---|---|---|---|---|---|---|---|---|---|---|---|
Group . | Contrast2 . | EBV . | GEBV . | GEBV_UC3 . | EBV . | GEBV . | GEBV_UC . | ||||||
All | C1 | 0.02 | (0.22) | 0.05 | (0.48) | 0.08 | (0.86) | 2.20 | (23.12) | 0.88 | (9.21) | 1.08 | (11.35) |
C2 | 0.02 | (0.25) | 0.05 | (0.55) | 0.09 | (0.91) | 2.06 | (21.65) | 1.15 | (12.09) | 1.10 | (11.56) | |
C3 | 0.02 | (0.22) | 0.04 | (0.46) | 0.08 | (0.85) | 1.91 | (20.08) | 1.07 | (11.25) | 0.97 | (10.21) | |
C4 | 0.01 | (0.11) | 0.02 | (0.26) | 0.07 | (0.76) | 1.63 | (17.13) | 0.66 | (6.98) | 0.85 | (8.97) | |
C5 | 0.00 | (0.04) | 0.01 | (0.13) | 0.07 | (0.73) | 1.33 | (13.98) | 0.45 | (4.69) | 0.96 | (10.12) | |
C6 | 0.00 | (0.01) | 0.01 | (0.07) | 0.07 | (0.73) | 1.68 | (17.66) | 1.09 | (11.46) | 1.17 | (12.30) | |
C7 | 0.00 | (0.02) | 0.01 | (0.06) | 0.07 | (0.73) | 1.55 | (16.29) | 0.72 | (7.54) | 0.95 | (10.00) | |
C8 | 0.00 | (0.04) | 0.01 | (0.12) | 0.07 | (0.73) | 1.08 | (11.35) | 0.44 | (4.60) | 0.84 | (8.81) | |
C9 | 0.01 | (0.06) | 0.01 | (0.15) | 0.07 | (0.73) | 1.29 | (13.56) | 0.70 | (7.35) | 0.88 | (9.26) | |
C10 | 0.00 | (0.03) | 0.01 | (0.11) | 0.07 | (0.72) | 1.00 | (10.51) | 0.44 | (4.61) | 0.94 | (9.88) | |
C11 | 0.00 | (0.01) | 0.00 | (0.05) | 0.07 | (0.72) | 0.43 | (4.53) | 0.18 | (1.85) | 0.84 | (8.81) | |
C12 | 0.00 | (0.00) | 0.00 | (0.02) | 0.07 | (0.72) | 0.27 | (2.80) | 0.11 | (1.10) | 0.87 | (9.09) | |
New progeny records | C1 | 0.04 | (0.41) | 0.05 | (0.48) | 0.08 | (0.87) | 2.20 | (23.12) | 0.88 | (9.21) | 0.88 | (9.22) |
C2 | 0.05 | (0.51) | 0.05 | (0.58) | 0.09 | (0.93) | 2.06 | (21.65) | 1.15 | (12.09) | 1.10 | (11.56) | |
C3 | 0.05 | (0.48) | 0.05 | (0.51) | 0.08 | (0.88) | 1.91 | (20.08) | 1.07 | (11.25) | 0.83 | (8.72) | |
C4 | 0.02 | (0.23) | 0.03 | (0.28) | 0.07 | (0.77) | 1.63 | (17.13) | 0.66 | (6.98) | 0.70 | (7.32) | |
C5 | 0.01 | (0.09) | 0.01 | (0.14) | 0.07 | (0.73) | 1.33 | (13.98) | 0.45 | (4.69) | 0.72 | (7.53) | |
C6 | 0.00 | (0.03) | 0.01 | (0.07) | 0.07 | (0.73) | 1.68 | (17.66) | 1.09 | (11.46) | 1.17 | (12.30) | |
C7 | 0.00 | (0.03) | 0.01 | (0.06) | 0.07 | (0.73) | 1.55 | (16.29) | 0.72 | (7.54) | 0.95 | (10.00) | |
C8 | 0.01 | (0.07) | 0.01 | (0.12) | 0.07 | (0.73) | 1.08 | (11.35) | 0.44 | (4.60) | 0.84 | (8.81) | |
C9 | 0.01 | (0.10) | 0.01 | (0.15) | 0.07 | (0.73) | 1.29 | (13.56) | 0.70 | (7.35) | 0.72 | (7.58) | |
C10 | 0.01 | (0.06) | 0.01 | (0.11) | 0.07 | (0.73) | 1.00 | (10.51) | 0.44 | (4.61) | 0.64 | (6.73) | |
C11 | 0.00 | (0.02) | 0.00 | (0.05) | 0.07 | (0.72) | 0.43 | (4.53) | 0.18 | (1.85) | 0.61 | (6.42) | |
C12 | 0.00 | (0.01) | 0.00 | (0.02) | 0.07 | (0.72) | 0.27 | (2.80) | 0.11 | (1.10) | 0.65 | (6.84) | |
Young4 | C1 | 0.02 | (0.21) | 0.05 | (0.50) | 0.08 | (0.88) | 0.70 | (7.31) | 0.25 | (2.59) | 0.37 | (3.87) |
C2 | 0.02 | (0.23) | 0.05 | (0.57) | 0.09 | (0.97) | 0.58 | (6.09) | 0.25 | (2.62) | 0.42 | (4.46) | |
C3 | 0.02 | (0.21) | 0.04 | (0.46) | 0.08 | (0.87) | 0.56 | (5.87) | 0.23 | (2.43) | 0.61 | (6.45) | |
C4 | 0.01 | (0.11) | 0.03 | (0.27) | 0.07 | (0.77) | 0.22 | (2.34) | 0.14 | (1.51) | 0.53 | (5.58) | |
C5 | 0.00 | (0.04) | 0.01 | (0.13) | 0.07 | (0.74) | 0.12 | (1.23) | 0.06 | (0.58) | 0.40 | (4.20) | |
C6 | 0.00 | (0.01) | 0.01 | (0.07) | 0.07 | (0.75) | 0.04 | (0.41) | 0.03 | (0.29) | 0.34 | (3.62) | |
C7 | 0.00 | (0.01) | 0.01 | (0.06) | 0.07 | (0.74) | 0.14 | (1.46) | 0.05 | (0.56) | 0.37 | (3.93) | |
C8 | 0.00 | (0.03) | 0.01 | (0.13) | 0.07 | (0.75) | 0.07 | (0.73) | 0.08 | (0.82) | 0.40 | (4.15) | |
C9 | 0.00 | (0.05) | 0.01 | (0.15) | 0.07 | (0.74) | 0.12 | (1.24) | 0.07 | (0.74) | 0.46 | (4.79) | |
C10 | 0.00 | (0.04) | 0.01 | (0.11) | 0.07 | (0.72) | 0.12 | (1.25) | 0.05 | (0.49) | 0.39 | (4.08) | |
C11 | 0.00 | (0.01) | 0.01 | (0.05) | 0.07 | (0.70) | 0.05 | (0.49) | 0.02 | (0.24) | 0.41 | (4.32) | |
C12 | 0.00 | (0.00) | 0.00 | (0.02) | 0.07 | (0.70) | 0.02 | (0.22) | 0.01 | (0.12) | 0.47 | (4.97) |
. | . | Average . | Maximum . | ||||||||||
---|---|---|---|---|---|---|---|---|---|---|---|---|---|
Group . | Contrast2 . | EBV . | GEBV . | GEBV_UC3 . | EBV . | GEBV . | GEBV_UC . | ||||||
All | C1 | 0.02 | (0.22) | 0.05 | (0.48) | 0.08 | (0.86) | 2.20 | (23.12) | 0.88 | (9.21) | 1.08 | (11.35) |
C2 | 0.02 | (0.25) | 0.05 | (0.55) | 0.09 | (0.91) | 2.06 | (21.65) | 1.15 | (12.09) | 1.10 | (11.56) | |
C3 | 0.02 | (0.22) | 0.04 | (0.46) | 0.08 | (0.85) | 1.91 | (20.08) | 1.07 | (11.25) | 0.97 | (10.21) | |
C4 | 0.01 | (0.11) | 0.02 | (0.26) | 0.07 | (0.76) | 1.63 | (17.13) | 0.66 | (6.98) | 0.85 | (8.97) | |
C5 | 0.00 | (0.04) | 0.01 | (0.13) | 0.07 | (0.73) | 1.33 | (13.98) | 0.45 | (4.69) | 0.96 | (10.12) | |
C6 | 0.00 | (0.01) | 0.01 | (0.07) | 0.07 | (0.73) | 1.68 | (17.66) | 1.09 | (11.46) | 1.17 | (12.30) | |
C7 | 0.00 | (0.02) | 0.01 | (0.06) | 0.07 | (0.73) | 1.55 | (16.29) | 0.72 | (7.54) | 0.95 | (10.00) | |
C8 | 0.00 | (0.04) | 0.01 | (0.12) | 0.07 | (0.73) | 1.08 | (11.35) | 0.44 | (4.60) | 0.84 | (8.81) | |
C9 | 0.01 | (0.06) | 0.01 | (0.15) | 0.07 | (0.73) | 1.29 | (13.56) | 0.70 | (7.35) | 0.88 | (9.26) | |
C10 | 0.00 | (0.03) | 0.01 | (0.11) | 0.07 | (0.72) | 1.00 | (10.51) | 0.44 | (4.61) | 0.94 | (9.88) | |
C11 | 0.00 | (0.01) | 0.00 | (0.05) | 0.07 | (0.72) | 0.43 | (4.53) | 0.18 | (1.85) | 0.84 | (8.81) | |
C12 | 0.00 | (0.00) | 0.00 | (0.02) | 0.07 | (0.72) | 0.27 | (2.80) | 0.11 | (1.10) | 0.87 | (9.09) | |
New progeny records | C1 | 0.04 | (0.41) | 0.05 | (0.48) | 0.08 | (0.87) | 2.20 | (23.12) | 0.88 | (9.21) | 0.88 | (9.22) |
C2 | 0.05 | (0.51) | 0.05 | (0.58) | 0.09 | (0.93) | 2.06 | (21.65) | 1.15 | (12.09) | 1.10 | (11.56) | |
C3 | 0.05 | (0.48) | 0.05 | (0.51) | 0.08 | (0.88) | 1.91 | (20.08) | 1.07 | (11.25) | 0.83 | (8.72) | |
C4 | 0.02 | (0.23) | 0.03 | (0.28) | 0.07 | (0.77) | 1.63 | (17.13) | 0.66 | (6.98) | 0.70 | (7.32) | |
C5 | 0.01 | (0.09) | 0.01 | (0.14) | 0.07 | (0.73) | 1.33 | (13.98) | 0.45 | (4.69) | 0.72 | (7.53) | |
C6 | 0.00 | (0.03) | 0.01 | (0.07) | 0.07 | (0.73) | 1.68 | (17.66) | 1.09 | (11.46) | 1.17 | (12.30) | |
C7 | 0.00 | (0.03) | 0.01 | (0.06) | 0.07 | (0.73) | 1.55 | (16.29) | 0.72 | (7.54) | 0.95 | (10.00) | |
C8 | 0.01 | (0.07) | 0.01 | (0.12) | 0.07 | (0.73) | 1.08 | (11.35) | 0.44 | (4.60) | 0.84 | (8.81) | |
C9 | 0.01 | (0.10) | 0.01 | (0.15) | 0.07 | (0.73) | 1.29 | (13.56) | 0.70 | (7.35) | 0.72 | (7.58) | |
C10 | 0.01 | (0.06) | 0.01 | (0.11) | 0.07 | (0.73) | 1.00 | (10.51) | 0.44 | (4.61) | 0.64 | (6.73) | |
C11 | 0.00 | (0.02) | 0.00 | (0.05) | 0.07 | (0.72) | 0.43 | (4.53) | 0.18 | (1.85) | 0.61 | (6.42) | |
C12 | 0.00 | (0.01) | 0.00 | (0.02) | 0.07 | (0.72) | 0.27 | (2.80) | 0.11 | (1.10) | 0.65 | (6.84) | |
Young4 | C1 | 0.02 | (0.21) | 0.05 | (0.50) | 0.08 | (0.88) | 0.70 | (7.31) | 0.25 | (2.59) | 0.37 | (3.87) |
C2 | 0.02 | (0.23) | 0.05 | (0.57) | 0.09 | (0.97) | 0.58 | (6.09) | 0.25 | (2.62) | 0.42 | (4.46) | |
C3 | 0.02 | (0.21) | 0.04 | (0.46) | 0.08 | (0.87) | 0.56 | (5.87) | 0.23 | (2.43) | 0.61 | (6.45) | |
C4 | 0.01 | (0.11) | 0.03 | (0.27) | 0.07 | (0.77) | 0.22 | (2.34) | 0.14 | (1.51) | 0.53 | (5.58) | |
C5 | 0.00 | (0.04) | 0.01 | (0.13) | 0.07 | (0.74) | 0.12 | (1.23) | 0.06 | (0.58) | 0.40 | (4.20) | |
C6 | 0.00 | (0.01) | 0.01 | (0.07) | 0.07 | (0.75) | 0.04 | (0.41) | 0.03 | (0.29) | 0.34 | (3.62) | |
C7 | 0.00 | (0.01) | 0.01 | (0.06) | 0.07 | (0.74) | 0.14 | (1.46) | 0.05 | (0.56) | 0.37 | (3.93) | |
C8 | 0.00 | (0.03) | 0.01 | (0.13) | 0.07 | (0.75) | 0.07 | (0.73) | 0.08 | (0.82) | 0.40 | (4.15) | |
C9 | 0.00 | (0.05) | 0.01 | (0.15) | 0.07 | (0.74) | 0.12 | (1.24) | 0.07 | (0.74) | 0.46 | (4.79) | |
C10 | 0.00 | (0.04) | 0.01 | (0.11) | 0.07 | (0.72) | 0.12 | (1.25) | 0.05 | (0.49) | 0.39 | (4.08) | |
C11 | 0.00 | (0.01) | 0.01 | (0.05) | 0.07 | (0.70) | 0.05 | (0.49) | 0.02 | (0.24) | 0.41 | (4.32) | |
C12 | 0.00 | (0.00) | 0.00 | (0.02) | 0.07 | (0.70) | 0.02 | (0.22) | 0.01 | (0.12) | 0.47 | (4.97) |
1Absolute changes in additive genetic standard deviation units (in kg inside the parenthesis).
2Cx, contrast between month x of 2017 and the precedent month, with x varying from 1 (January) to 12 (December).
3GEBV_UC, GEBV from a scenario where the core set is updated every month.
4Born in 2016 with neither phenotypes nor progeny.
Average and maximum absolute changes in EBV and genomic EBV for weaning weight among monthly evaluations in 2017 and evaluations in the previous month for genotyped animals born until December 2016 in the American Angus population1
. | . | Average . | Maximum . | ||||||||||
---|---|---|---|---|---|---|---|---|---|---|---|---|---|
Group . | Contrast2 . | EBV . | GEBV . | GEBV_UC3 . | EBV . | GEBV . | GEBV_UC . | ||||||
All | C1 | 0.02 | (0.22) | 0.05 | (0.48) | 0.08 | (0.86) | 2.20 | (23.12) | 0.88 | (9.21) | 1.08 | (11.35) |
C2 | 0.02 | (0.25) | 0.05 | (0.55) | 0.09 | (0.91) | 2.06 | (21.65) | 1.15 | (12.09) | 1.10 | (11.56) | |
C3 | 0.02 | (0.22) | 0.04 | (0.46) | 0.08 | (0.85) | 1.91 | (20.08) | 1.07 | (11.25) | 0.97 | (10.21) | |
C4 | 0.01 | (0.11) | 0.02 | (0.26) | 0.07 | (0.76) | 1.63 | (17.13) | 0.66 | (6.98) | 0.85 | (8.97) | |
C5 | 0.00 | (0.04) | 0.01 | (0.13) | 0.07 | (0.73) | 1.33 | (13.98) | 0.45 | (4.69) | 0.96 | (10.12) | |
C6 | 0.00 | (0.01) | 0.01 | (0.07) | 0.07 | (0.73) | 1.68 | (17.66) | 1.09 | (11.46) | 1.17 | (12.30) | |
C7 | 0.00 | (0.02) | 0.01 | (0.06) | 0.07 | (0.73) | 1.55 | (16.29) | 0.72 | (7.54) | 0.95 | (10.00) | |
C8 | 0.00 | (0.04) | 0.01 | (0.12) | 0.07 | (0.73) | 1.08 | (11.35) | 0.44 | (4.60) | 0.84 | (8.81) | |
C9 | 0.01 | (0.06) | 0.01 | (0.15) | 0.07 | (0.73) | 1.29 | (13.56) | 0.70 | (7.35) | 0.88 | (9.26) | |
C10 | 0.00 | (0.03) | 0.01 | (0.11) | 0.07 | (0.72) | 1.00 | (10.51) | 0.44 | (4.61) | 0.94 | (9.88) | |
C11 | 0.00 | (0.01) | 0.00 | (0.05) | 0.07 | (0.72) | 0.43 | (4.53) | 0.18 | (1.85) | 0.84 | (8.81) | |
C12 | 0.00 | (0.00) | 0.00 | (0.02) | 0.07 | (0.72) | 0.27 | (2.80) | 0.11 | (1.10) | 0.87 | (9.09) | |
New progeny records | C1 | 0.04 | (0.41) | 0.05 | (0.48) | 0.08 | (0.87) | 2.20 | (23.12) | 0.88 | (9.21) | 0.88 | (9.22) |
C2 | 0.05 | (0.51) | 0.05 | (0.58) | 0.09 | (0.93) | 2.06 | (21.65) | 1.15 | (12.09) | 1.10 | (11.56) | |
C3 | 0.05 | (0.48) | 0.05 | (0.51) | 0.08 | (0.88) | 1.91 | (20.08) | 1.07 | (11.25) | 0.83 | (8.72) | |
C4 | 0.02 | (0.23) | 0.03 | (0.28) | 0.07 | (0.77) | 1.63 | (17.13) | 0.66 | (6.98) | 0.70 | (7.32) | |
C5 | 0.01 | (0.09) | 0.01 | (0.14) | 0.07 | (0.73) | 1.33 | (13.98) | 0.45 | (4.69) | 0.72 | (7.53) | |
C6 | 0.00 | (0.03) | 0.01 | (0.07) | 0.07 | (0.73) | 1.68 | (17.66) | 1.09 | (11.46) | 1.17 | (12.30) | |
C7 | 0.00 | (0.03) | 0.01 | (0.06) | 0.07 | (0.73) | 1.55 | (16.29) | 0.72 | (7.54) | 0.95 | (10.00) | |
C8 | 0.01 | (0.07) | 0.01 | (0.12) | 0.07 | (0.73) | 1.08 | (11.35) | 0.44 | (4.60) | 0.84 | (8.81) | |
C9 | 0.01 | (0.10) | 0.01 | (0.15) | 0.07 | (0.73) | 1.29 | (13.56) | 0.70 | (7.35) | 0.72 | (7.58) | |
C10 | 0.01 | (0.06) | 0.01 | (0.11) | 0.07 | (0.73) | 1.00 | (10.51) | 0.44 | (4.61) | 0.64 | (6.73) | |
C11 | 0.00 | (0.02) | 0.00 | (0.05) | 0.07 | (0.72) | 0.43 | (4.53) | 0.18 | (1.85) | 0.61 | (6.42) | |
C12 | 0.00 | (0.01) | 0.00 | (0.02) | 0.07 | (0.72) | 0.27 | (2.80) | 0.11 | (1.10) | 0.65 | (6.84) | |
Young4 | C1 | 0.02 | (0.21) | 0.05 | (0.50) | 0.08 | (0.88) | 0.70 | (7.31) | 0.25 | (2.59) | 0.37 | (3.87) |
C2 | 0.02 | (0.23) | 0.05 | (0.57) | 0.09 | (0.97) | 0.58 | (6.09) | 0.25 | (2.62) | 0.42 | (4.46) | |
C3 | 0.02 | (0.21) | 0.04 | (0.46) | 0.08 | (0.87) | 0.56 | (5.87) | 0.23 | (2.43) | 0.61 | (6.45) | |
C4 | 0.01 | (0.11) | 0.03 | (0.27) | 0.07 | (0.77) | 0.22 | (2.34) | 0.14 | (1.51) | 0.53 | (5.58) | |
C5 | 0.00 | (0.04) | 0.01 | (0.13) | 0.07 | (0.74) | 0.12 | (1.23) | 0.06 | (0.58) | 0.40 | (4.20) | |
C6 | 0.00 | (0.01) | 0.01 | (0.07) | 0.07 | (0.75) | 0.04 | (0.41) | 0.03 | (0.29) | 0.34 | (3.62) | |
C7 | 0.00 | (0.01) | 0.01 | (0.06) | 0.07 | (0.74) | 0.14 | (1.46) | 0.05 | (0.56) | 0.37 | (3.93) | |
C8 | 0.00 | (0.03) | 0.01 | (0.13) | 0.07 | (0.75) | 0.07 | (0.73) | 0.08 | (0.82) | 0.40 | (4.15) | |
C9 | 0.00 | (0.05) | 0.01 | (0.15) | 0.07 | (0.74) | 0.12 | (1.24) | 0.07 | (0.74) | 0.46 | (4.79) | |
C10 | 0.00 | (0.04) | 0.01 | (0.11) | 0.07 | (0.72) | 0.12 | (1.25) | 0.05 | (0.49) | 0.39 | (4.08) | |
C11 | 0.00 | (0.01) | 0.01 | (0.05) | 0.07 | (0.70) | 0.05 | (0.49) | 0.02 | (0.24) | 0.41 | (4.32) | |
C12 | 0.00 | (0.00) | 0.00 | (0.02) | 0.07 | (0.70) | 0.02 | (0.22) | 0.01 | (0.12) | 0.47 | (4.97) |
. | . | Average . | Maximum . | ||||||||||
---|---|---|---|---|---|---|---|---|---|---|---|---|---|
Group . | Contrast2 . | EBV . | GEBV . | GEBV_UC3 . | EBV . | GEBV . | GEBV_UC . | ||||||
All | C1 | 0.02 | (0.22) | 0.05 | (0.48) | 0.08 | (0.86) | 2.20 | (23.12) | 0.88 | (9.21) | 1.08 | (11.35) |
C2 | 0.02 | (0.25) | 0.05 | (0.55) | 0.09 | (0.91) | 2.06 | (21.65) | 1.15 | (12.09) | 1.10 | (11.56) | |
C3 | 0.02 | (0.22) | 0.04 | (0.46) | 0.08 | (0.85) | 1.91 | (20.08) | 1.07 | (11.25) | 0.97 | (10.21) | |
C4 | 0.01 | (0.11) | 0.02 | (0.26) | 0.07 | (0.76) | 1.63 | (17.13) | 0.66 | (6.98) | 0.85 | (8.97) | |
C5 | 0.00 | (0.04) | 0.01 | (0.13) | 0.07 | (0.73) | 1.33 | (13.98) | 0.45 | (4.69) | 0.96 | (10.12) | |
C6 | 0.00 | (0.01) | 0.01 | (0.07) | 0.07 | (0.73) | 1.68 | (17.66) | 1.09 | (11.46) | 1.17 | (12.30) | |
C7 | 0.00 | (0.02) | 0.01 | (0.06) | 0.07 | (0.73) | 1.55 | (16.29) | 0.72 | (7.54) | 0.95 | (10.00) | |
C8 | 0.00 | (0.04) | 0.01 | (0.12) | 0.07 | (0.73) | 1.08 | (11.35) | 0.44 | (4.60) | 0.84 | (8.81) | |
C9 | 0.01 | (0.06) | 0.01 | (0.15) | 0.07 | (0.73) | 1.29 | (13.56) | 0.70 | (7.35) | 0.88 | (9.26) | |
C10 | 0.00 | (0.03) | 0.01 | (0.11) | 0.07 | (0.72) | 1.00 | (10.51) | 0.44 | (4.61) | 0.94 | (9.88) | |
C11 | 0.00 | (0.01) | 0.00 | (0.05) | 0.07 | (0.72) | 0.43 | (4.53) | 0.18 | (1.85) | 0.84 | (8.81) | |
C12 | 0.00 | (0.00) | 0.00 | (0.02) | 0.07 | (0.72) | 0.27 | (2.80) | 0.11 | (1.10) | 0.87 | (9.09) | |
New progeny records | C1 | 0.04 | (0.41) | 0.05 | (0.48) | 0.08 | (0.87) | 2.20 | (23.12) | 0.88 | (9.21) | 0.88 | (9.22) |
C2 | 0.05 | (0.51) | 0.05 | (0.58) | 0.09 | (0.93) | 2.06 | (21.65) | 1.15 | (12.09) | 1.10 | (11.56) | |
C3 | 0.05 | (0.48) | 0.05 | (0.51) | 0.08 | (0.88) | 1.91 | (20.08) | 1.07 | (11.25) | 0.83 | (8.72) | |
C4 | 0.02 | (0.23) | 0.03 | (0.28) | 0.07 | (0.77) | 1.63 | (17.13) | 0.66 | (6.98) | 0.70 | (7.32) | |
C5 | 0.01 | (0.09) | 0.01 | (0.14) | 0.07 | (0.73) | 1.33 | (13.98) | 0.45 | (4.69) | 0.72 | (7.53) | |
C6 | 0.00 | (0.03) | 0.01 | (0.07) | 0.07 | (0.73) | 1.68 | (17.66) | 1.09 | (11.46) | 1.17 | (12.30) | |
C7 | 0.00 | (0.03) | 0.01 | (0.06) | 0.07 | (0.73) | 1.55 | (16.29) | 0.72 | (7.54) | 0.95 | (10.00) | |
C8 | 0.01 | (0.07) | 0.01 | (0.12) | 0.07 | (0.73) | 1.08 | (11.35) | 0.44 | (4.60) | 0.84 | (8.81) | |
C9 | 0.01 | (0.10) | 0.01 | (0.15) | 0.07 | (0.73) | 1.29 | (13.56) | 0.70 | (7.35) | 0.72 | (7.58) | |
C10 | 0.01 | (0.06) | 0.01 | (0.11) | 0.07 | (0.73) | 1.00 | (10.51) | 0.44 | (4.61) | 0.64 | (6.73) | |
C11 | 0.00 | (0.02) | 0.00 | (0.05) | 0.07 | (0.72) | 0.43 | (4.53) | 0.18 | (1.85) | 0.61 | (6.42) | |
C12 | 0.00 | (0.01) | 0.00 | (0.02) | 0.07 | (0.72) | 0.27 | (2.80) | 0.11 | (1.10) | 0.65 | (6.84) | |
Young4 | C1 | 0.02 | (0.21) | 0.05 | (0.50) | 0.08 | (0.88) | 0.70 | (7.31) | 0.25 | (2.59) | 0.37 | (3.87) |
C2 | 0.02 | (0.23) | 0.05 | (0.57) | 0.09 | (0.97) | 0.58 | (6.09) | 0.25 | (2.62) | 0.42 | (4.46) | |
C3 | 0.02 | (0.21) | 0.04 | (0.46) | 0.08 | (0.87) | 0.56 | (5.87) | 0.23 | (2.43) | 0.61 | (6.45) | |
C4 | 0.01 | (0.11) | 0.03 | (0.27) | 0.07 | (0.77) | 0.22 | (2.34) | 0.14 | (1.51) | 0.53 | (5.58) | |
C5 | 0.00 | (0.04) | 0.01 | (0.13) | 0.07 | (0.74) | 0.12 | (1.23) | 0.06 | (0.58) | 0.40 | (4.20) | |
C6 | 0.00 | (0.01) | 0.01 | (0.07) | 0.07 | (0.75) | 0.04 | (0.41) | 0.03 | (0.29) | 0.34 | (3.62) | |
C7 | 0.00 | (0.01) | 0.01 | (0.06) | 0.07 | (0.74) | 0.14 | (1.46) | 0.05 | (0.56) | 0.37 | (3.93) | |
C8 | 0.00 | (0.03) | 0.01 | (0.13) | 0.07 | (0.75) | 0.07 | (0.73) | 0.08 | (0.82) | 0.40 | (4.15) | |
C9 | 0.00 | (0.05) | 0.01 | (0.15) | 0.07 | (0.74) | 0.12 | (1.24) | 0.07 | (0.74) | 0.46 | (4.79) | |
C10 | 0.00 | (0.04) | 0.01 | (0.11) | 0.07 | (0.72) | 0.12 | (1.25) | 0.05 | (0.49) | 0.39 | (4.08) | |
C11 | 0.00 | (0.01) | 0.01 | (0.05) | 0.07 | (0.70) | 0.05 | (0.49) | 0.02 | (0.24) | 0.41 | (4.32) | |
C12 | 0.00 | (0.00) | 0.00 | (0.02) | 0.07 | (0.70) | 0.02 | (0.22) | 0.01 | (0.12) | 0.47 | (4.97) |
1Absolute changes in additive genetic standard deviation units (in kg inside the parenthesis).
2Cx, contrast between month x of 2017 and the precedent month, with x varying from 1 (January) to 12 (December).
3GEBV_UC, GEBV from a scenario where the core set is updated every month.
4Born in 2016 with neither phenotypes nor progeny.
Conclusions
When new pedigrees, genomic, and phenotypic data were included in the genomic evaluations of animals in the American Angus population, the average absolute changes in GEBV were about twice as large as those in EBV for most of the genotyped animals but for the ones with new progeny phenotypic records. The most extreme maximum absolute changes were observed for EBV. The deep pedigree and ample phenotypic and genomic data from the American Angus population yielded accurate estimates for all independent chromosome segments and their clusters, contributing to high persistency of genomic evaluations and leading to stable genomic evaluations during the year of the study. Genomic evaluations were as stable as traditional evaluations for animals with new progeny phenotypic records. If the APY is used, changes in GEBV due to changes in the core group can be avoided by keeping the same core group for 1 yr and updating it when a large amount of data is added.
Abbreviations
- APY
algorithm for proven and young
- BLUP
best linear unbiased prediction
- EBV
estimated breeding value
- G
genomic relationship matrix
- GEBV
genomic estimated breeding value
- L
length of the genome
- Ne
effective population size
- SDa
additive genetic standard deviation
- ssGBLUP
single-step genomic best linear unbiased prediction
Acknowledgments
This study was partly supported by Angus Genetics Inc. (St. Joseph, MO) and by the Agriculture and Food Research Initiative Competitive Grant (2020-67015-31030) from the US Department of Agriculture’s National Institute of Food and Agriculture. We gratefully acknowledge the editing of the manuscript by Mauricio Elzo. J.H. acknowledges the support during his PhD studies by the National Council for Science and Technology (CONACYT), Mexico.
Conflict of interest statement
The authors declare no real or perceived conflicts of interest.