-
PDF
- Split View
-
Views
-
Cite
Cite
Yoonjin Kang, Suk Ho Sohn, Jae Woong Choi, Ho Young Hwang, Kyung Hwan Kim, Machine-learning-based prediction of survival and mitral regurgitation recurrence in patients undergoing mitral valve repair, Interdisciplinary CardioVascular and Thoracic Surgery, Volume 37, Issue 5, November 2023, ivad176, https://doi.org/10.1093/icvts/ivad176
- Share Icon Share
Abstract
This study was conducted to assess long-term clinical outcomes after mitral valve repair using machine-learning techniques.
We retrospectively evaluated 436 consecutive patients (mean age: 54.7 ± 15.4; 235 males) who underwent mitral valve repair between January 2000 and December 2017. Actuarial survival and freedom from significant (≥ moderate) mitral regurgitation (MR) were clinical end points. To evaluate the independent risk factors, random survival forest (RSF), extreme gradient boost (XGBoost), support vector machine, Cox proportional hazards model and general linear models with elastic net regularization were used. Concordance indices (C-indices) of each model were estimated.
The operative mortality was 0.9% (N = 4). Reoperation was required in 15 patients (3.5%). In terms of C-index, the overall performance of the XGBoost (C-index 0.806) and RSF models (C-index 0.814) was better than that of the Cox model (C-index 0.733) in overall survival. For the recurrent MR, the C-index for XGBoost was 0.718, which was the highest among the 5 models. Compared to the Cox model (C-index 0.545), the C-indices of the XGBoost (C-index 0.718) and RSF models (C-index 0.692) were higher.
Machine-learning techniques can be a useful tool for both prediction and interpretation in the survival and recurrent MR. From the machine-learning techniques examined here, the long-term clinical outcomes of mitral valve repair were excellent. The complexity of MV increased the risk of late mitral valve-related reoperation.
INTRODUCTION
Mitral valve repair is considered to have better survival and clinical outcomes compared to valve replacement [1–3]. As late survival after mitral valve repair is associated with the presence of symptoms of congestive heart failure or left ventricular (LV) dysfunction [4–6], the guidelines suggest that mitral valve repair should be considered in asymptomatic patients with severe mitral regurgitation (MR) and normal LV function if it can be performed safely [7]. Therefore, the number of patients who undergo mitral valve repair is increasing and valve repair failures have been also increasing [8–10]. Valve repair failure rates are known to be 0.5–4% according to the aetiologies [10–12]. Disease progression itself is known to be the most common cause of MR recurrence [13]. However, the data on the long-term outcomes of mitral valve repair regarding late failure are still limited.
The most commonly used survival analysis in the literature is the Cox proportional hazards regression model. However, as the conventional Cox model is a linear prognostic prediction model, it is based on hazard ratios being constant over time and linear effects of covariates on hazards, which is not suitable to real-life applications [14]. In a Cox model, nonlinear functions between the log-hazard ratio and static covariates would decrease prediction accuracy. Therefore, novel machine-learning techniques that include nonlinear variables are in great need to predict outcomes in various clinical settings. In this regard, machine-learning approaches including random forest, extreme gradient boost (XGBoost) and support vector machine (SVM) have recently been used to predict outcomes after cardiac surgery [15, 16]. These techniques have been successfully applied to censored survival data, such as random survival forest- (RSF) or XGBoost-based survival analysis. These machine-learning models for survival analyses show a better fit for variables with a nonlinear relationship, which reflects real-life factors. In large feature sets, those models can learn feature representation, easily handling censoring in survival data. However, so far only a single study has adopted machine-learning techniques for survival analysis within the field of cardiac surgery [17]. The present study was conducted to examine long-term clinical outcomes and recurrence of MR over a 20-year postoperative follow-up period using machine-learning techniques for survival analysis.
PATIENTS AND METHODS
Ethics statement
The study protocol was reviewed by the Institutional Review Board at our institution and approved as a minimal-risk retrospective study (approval number: H-2106-078-1226) that did not require individual consent.
Patient enrollment
A total of 461 consecutive patients who underwent mitral valve repair at our institution (a single-centre study). Among these patients between January 2000 and December 2017 were included in this study. Of these, 13 who underwent mitral valve repair for congenital heart disease and 5 who had connective tissue disorder were excluded. Seven patients with any missing data were excluded. Thus, a total of 436 patients (54.7 ± 15.4 years; 235 males and 201 females) were enrolled in the present study.
Operative strategy
All procedures were performed through a median sternotomy, with aorto-bicaval cannulation under moderate hypothermia and cold cardioplegic arrest. Concomitant procedures included coronary artery bypass grafting, arrhythmia surgery, tricuspid valve-related procedures and aortic valve-related procedures. Mitral valve repair techniques included quadrangular and triangular resections (27.5%), artificial chordae implantation (18.6%), chordae transfer (3.9%), commissurotomy (6.0%), secondary chordae resection (6.2%), papillary muscle splitting (2.8%), commissural obliteration (12.2%), chordae shortening (6.0%), papillary muscle shortening (0.9%) and the Alfieri procedure (1.1%). Most of the procedures were combined with prosthetic ring implantation (90.1%). Those who did not underwent prosthetic ring implantation had systolic anterior motion or small annulus; for all patients, the specific surgical repair techniques used were documented. Surgery was performed by 4 cardiac surgeons with experience of at least 100 open heart valve surgeries. Surgical video shows mitral valve repair (artificial chordae implantation) for rupture chordae with degenerative pathology.
Early and long-term clinical outcomes
Operative mortality was defined as death within 30 days of the operation or during the same hospitalization period. Postoperative low cardiac output syndrome was defined as the need for mechanical or inotropic support to maintain systolic blood pressure >90 mmHg despite the correction of reversible factors.
Regular (3- to 6-month intervals) postoperative clinical and echocardiographic follow-up was performed in the outpatient clinic. Patient condition was evaluated via telephone calls if they could not keep an appointment. In addition, survival data were obtained from Statistics Korea, a national organization that collects and aggregates quality data about the people and economy in Korea and has complete data regarding deaths through 31 December 2019. The clinical follow-up period ended on 28 February 2019. The median follow-up duration was 99 months (range: 1–295 months).
Postoperative echocardiography was performed at hospital discharge and then serially at 3-month intervals. Recurrent MV was diagnosed if echocardiography showed greater than mild MR. The median echocardiographic follow-up duration was 64.9 months (range: 1–200 months). Complex mitral valve lesions were defined as lesions involving >2 posterior leaflet scallops, anterior or both leaflets, commissures or those with severe annular or leaflet calcifications [18, 19].
Statistical analysis
The development of machine-learning models and statistical analyses were performed using R software (version 4.1.3; The R Foundation, Vienna, Austria; http://cran.r-project.org). Comparisons between the 2 groups (mortality versus non-mortality group and recurrence versus non-recurrence group) were performed using the Chi-square test or Fisher’s exact test and Student’s t-test for categorical and continuous variables, respectively. When >20% of cells have expected frequencies <5, Fisher's exact test was used. Normal distribution of continuous variables was determined by Shapiro–Wilk test. Survival rates were estimated using the Kaplan–Meier method. Risk factors for longitudinal data were analysed using a multivariable Cox proportional hazards model. Variables with a P-value of <0.10 in the univariate analyses were entered into multivariable models. A P-value of <0.05 was considered statistically significant.
We developed the Cox model and machine-learning model using 39 variables from electronic health record to analyse overall mortality and MR recurrence. Preoperative variables, including age, sex, body mass index, smoking, hypertension, diabetes mellitus, history of stroke, chronic kidney disease, coronary artery disease, dyslipidaemia, emergency surgery, infective endocarditis, congestive heart failure before operation, aetiology, complexity of the mitral valve lesion, previous history of cardiac surgery, atrial fibrillation and LV ejection fraction, left atrium size, LV end-diastolic dimension, the presence of preoperative/intraoperative extracorporeal membrane oxygenation and operative techniques, were included in the analysis. Postoperative complications, including low cardiac output syndrome, reoperation due to bleeding, acute kidney injury, mediastinitis, stroke and pneumonia, were also included in the model.
The machine-learning algorithms used were RSF-, XGBoost- and SVM-based survival analyses. RSF uses a decision tree to predict and rank variables according to the importance to identify risk factors of the outcomes [20]. It is suitable for the analysis of correlated complex survival data with time-dependent outcomes. SVM is a model that defines decision boundary, which is the boundary for classification [21]. XGBoost selects decision tree as a base learner. Using gradient boosting, it has complemented weakness of the previous models, providing higher precision and accuracy [22]. These machine-learning techniques have been applied to survival analysis, yielding improved outcomes. The machine-learning models were compared to 2 statistical models using the Cox proportional hazards model and generalized linear models with elastic net regularization.
We estimated the performance of the models with stratified five-fold cross-validation in terms of concordance index (C-index) according to the patients with recurrent MR. The total population was randomly divided into 5 equal groups. Each fold was used as a test set separately, while the remaining four-fold was used as the training set. Thus, there were a total of 5 C-indices for a single model algorithm or statistical model. We estimated the average of these 5 C-index values and drew a box plot for the distribution of the averages.
The hyperparameters for RSF models included ntree (number of trees), mtry (number of variables used in each tree) and nodesize (minimum size of nodes, which determines depth).
For the XGB model, we determined the best hyperparameter consisting of nrounds, eta (step size shrinkage used in the update process to prevent overfitting) and gamma (minimum loss reduction required to make a further partition). The hyperparameters for SVM included margin and gamma.mu. The hyperparameters that would give the best results were optimized by configuring a set of hyperparameter choices manually with a usage of grid search.
In the RSF model, variable importance was analysed by assigning the out-of-bag cases to the opposite split. In the XGB model, variable importance was analysed using Gain, which implies the relative contribution of the corresponding feature to the model, calculated by taking each feature’s contribution towards each tree. We determined the variable importance for each of the 5 models from RSF and XGB using five-fold cross-validation; we chose the top 10 most important variables with the highest variable importance values for each feature.
RESULTS
Baseline characteristics
Table 1 shows demographic data of the study patients. A total of 187 patients were identified as complex disease. The mean cardiopulmonary bypass and aortic cross-clamp times were 132 ± 43 and 89 ± 32 min, respectively (Table 2). Concomitant cardiac procedures, including tricuspid valve operations (n = 82), aortic valve procedures (n = 20), arrhythmia surgery (n = 120) and coronary artery bypass grafting (n = 16), were performed.
Variables . | Total (n = 436) . | Mortality (n = 94) . | Non-mortality (n = 342) . | P-value . | Recurrence (n = 53) . | Non-recurrence (n = 383) . | P-value . |
---|---|---|---|---|---|---|---|
Age (years), mean ± SD | 54.7 ± 15.4 | 64.2 ± 11.4 | 52.2 ± 15.3 | <0.001 | 53.1 ± 15.3 | 55.0 ± 15.4 | 0.395 |
Female, n (%) | 235 (53.9%) | 51 (54.3%) | 184 (53.8%) | >0.999 | 30 (56.6%) | 205 (53.5%) | 0.784 |
BMI, mean ± SD | 23.4 ± 3.4 | 23.17 ± 3.45 | 23.40 ± 3.44 | 0.569 | 23.0 ± 3.1 | 23.4 ± 3.5 | 0.488 |
Risk factors, n (%) | |||||||
Hypertension | 125 (28.7%) | 39 (41.5%) | 86 (25.1%) | 0.003 | 6 (11.3%) | 119 (31.1%) | 0.005 |
Diabetes mellitus | 39 (8.9%) | 12 (12.8%) | 27 (7.9%) | 0.207 | 1 (1.9%) | 38 (9.9%) | 0.096 |
History of stroke | 19 (4.4%) | 7 (7.4%) | 12 (3.5%) | 0.170 | 0 (0.0%) | 19 (5.0%) | 0.194 |
CKDa | 24 (5.5%) | 15 (16%) | 9 (2.6%) | <0.001 | 2 (3.8%) | 22 (5.7%) | 0.789 |
Coronary artery disease | 29 (6.7%) | 11 (11.7%) | 18 (5.3%) | 0.047 | 2 (3.8%) | 27 (7.0%) | 0.547 |
Dyslipidaemia | 22 (5.0%) | 6 (6.4%) | 16 (4.7%) | 0.687 | 1 (1.9%) | 21 (5.5%) | 0.432 |
Emergency operation | 2 (0.5%) | 1 (1.1%) | 1 (0.3%) | 0.906 | 0 (0.0%) | 2 (0.5%) | >0.999 |
Previous cardiac surgery | 152 (34.9%) | 29 (30.9%) | 123 (36%) | 0.424 | 30 (56.6%) | 122 (31.9%) | 0.001 |
Atrial fibrillation | 98 (22.5%) | 31 (33%) | 67 (19.6%) | 0.009 | 7 (13.2%) | 91 (23.8%) | 0.121 |
Aetiology | |||||||
Rheumatic | 28 | 1 | 27 | 28 | 2 | 26 | |
Degenerative | 399 | 47 | 352 | 399 | 28 | 371 | |
Ischaemic | 3 | 2 | 1 | 3 | 0 | 3 | |
Functional | 3 | 0 | 3 | 3 | 0 | 3 | |
Endocarditis, n (%) | 3 (0.7%) | 0 (0.0%) | 3 (0.9%) | 0.836 | 0 (0.0%) | 3 (0.8%) | >0.999 |
LVESD, mean ± SD | 39.2 ± 7.8 | 41.5 ± 8.8 | 38.6 ± 7.4 | 0.003 | 40.2 ± 9.2 | 39.1 ± 7.6 | 0.400 |
LVEDD, mean ± SD | 61.1 ± 8.1 | 62.8 ± 8.4 | 60.7 ± 8.0 | 0.025 | 62.5 ± 9.4 | 60.9 ± 7.9 | 0.181 |
EF, mean ± SD | 60.0 ± 9.0 | 57.3 ± 10.3 | 60.6 ± 8.4 | 0.006 | 60.9 ± 10.7 | 59.7 ± 8.7 | 0.440 |
LA, mean ± SD | 55.8 ± 10.9 | 59.2 ± 10.2 | 54.9 ± 11.0 | 0.001 | 57.0 ± 10.9 | 55.7 ± 11.0 | 0.418 |
Complex disease | 187 (42.9%) | 38 (40.4%) | 149 (43.6%) | 0.669 | 36 (67.9%) | 151 (39.4%) | <0.001 |
IABP, n (%) | 15 (3.4%) | 4 (4.3%) | 11 (3.2%) | 0.865 | 1 (1.9%) | 22 (5.7%) | 0.937 |
ECMO, n (%) | 4 (0.9%) | 2 (2.1%) | 2 (0.6%) | 0.103 | 0 (0.0%) | 4 (1.0%) | >0.999 |
Variables . | Total (n = 436) . | Mortality (n = 94) . | Non-mortality (n = 342) . | P-value . | Recurrence (n = 53) . | Non-recurrence (n = 383) . | P-value . |
---|---|---|---|---|---|---|---|
Age (years), mean ± SD | 54.7 ± 15.4 | 64.2 ± 11.4 | 52.2 ± 15.3 | <0.001 | 53.1 ± 15.3 | 55.0 ± 15.4 | 0.395 |
Female, n (%) | 235 (53.9%) | 51 (54.3%) | 184 (53.8%) | >0.999 | 30 (56.6%) | 205 (53.5%) | 0.784 |
BMI, mean ± SD | 23.4 ± 3.4 | 23.17 ± 3.45 | 23.40 ± 3.44 | 0.569 | 23.0 ± 3.1 | 23.4 ± 3.5 | 0.488 |
Risk factors, n (%) | |||||||
Hypertension | 125 (28.7%) | 39 (41.5%) | 86 (25.1%) | 0.003 | 6 (11.3%) | 119 (31.1%) | 0.005 |
Diabetes mellitus | 39 (8.9%) | 12 (12.8%) | 27 (7.9%) | 0.207 | 1 (1.9%) | 38 (9.9%) | 0.096 |
History of stroke | 19 (4.4%) | 7 (7.4%) | 12 (3.5%) | 0.170 | 0 (0.0%) | 19 (5.0%) | 0.194 |
CKDa | 24 (5.5%) | 15 (16%) | 9 (2.6%) | <0.001 | 2 (3.8%) | 22 (5.7%) | 0.789 |
Coronary artery disease | 29 (6.7%) | 11 (11.7%) | 18 (5.3%) | 0.047 | 2 (3.8%) | 27 (7.0%) | 0.547 |
Dyslipidaemia | 22 (5.0%) | 6 (6.4%) | 16 (4.7%) | 0.687 | 1 (1.9%) | 21 (5.5%) | 0.432 |
Emergency operation | 2 (0.5%) | 1 (1.1%) | 1 (0.3%) | 0.906 | 0 (0.0%) | 2 (0.5%) | >0.999 |
Previous cardiac surgery | 152 (34.9%) | 29 (30.9%) | 123 (36%) | 0.424 | 30 (56.6%) | 122 (31.9%) | 0.001 |
Atrial fibrillation | 98 (22.5%) | 31 (33%) | 67 (19.6%) | 0.009 | 7 (13.2%) | 91 (23.8%) | 0.121 |
Aetiology | |||||||
Rheumatic | 28 | 1 | 27 | 28 | 2 | 26 | |
Degenerative | 399 | 47 | 352 | 399 | 28 | 371 | |
Ischaemic | 3 | 2 | 1 | 3 | 0 | 3 | |
Functional | 3 | 0 | 3 | 3 | 0 | 3 | |
Endocarditis, n (%) | 3 (0.7%) | 0 (0.0%) | 3 (0.9%) | 0.836 | 0 (0.0%) | 3 (0.8%) | >0.999 |
LVESD, mean ± SD | 39.2 ± 7.8 | 41.5 ± 8.8 | 38.6 ± 7.4 | 0.003 | 40.2 ± 9.2 | 39.1 ± 7.6 | 0.400 |
LVEDD, mean ± SD | 61.1 ± 8.1 | 62.8 ± 8.4 | 60.7 ± 8.0 | 0.025 | 62.5 ± 9.4 | 60.9 ± 7.9 | 0.181 |
EF, mean ± SD | 60.0 ± 9.0 | 57.3 ± 10.3 | 60.6 ± 8.4 | 0.006 | 60.9 ± 10.7 | 59.7 ± 8.7 | 0.440 |
LA, mean ± SD | 55.8 ± 10.9 | 59.2 ± 10.2 | 54.9 ± 11.0 | 0.001 | 57.0 ± 10.9 | 55.7 ± 11.0 | 0.418 |
Complex disease | 187 (42.9%) | 38 (40.4%) | 149 (43.6%) | 0.669 | 36 (67.9%) | 151 (39.4%) | <0.001 |
IABP, n (%) | 15 (3.4%) | 4 (4.3%) | 11 (3.2%) | 0.865 | 1 (1.9%) | 22 (5.7%) | 0.937 |
ECMO, n (%) | 4 (0.9%) | 2 (2.1%) | 2 (0.6%) | 0.103 | 0 (0.0%) | 4 (1.0%) | >0.999 |
CKD is adapted from the definition of chronic kidney disease by The Kidney Disease: Improving Global Outcomes Work Group.
BMI: body mass index; CKD: chronic kidney disease; ECMO: extracorporeal membrane oxygenation; EF: ejection fraction; IABP: intra-aortic balloon pump; LA: left atrium; LVEDD: left ventricular end-diastolic dimension; LVESD: left ventricular end-systolic dimension; SD: standard deviation.
Variables . | Total (n = 436) . | Mortality (n = 94) . | Non-mortality (n = 342) . | P-value . | Recurrence (n = 53) . | Non-recurrence (n = 383) . | P-value . |
---|---|---|---|---|---|---|---|
Age (years), mean ± SD | 54.7 ± 15.4 | 64.2 ± 11.4 | 52.2 ± 15.3 | <0.001 | 53.1 ± 15.3 | 55.0 ± 15.4 | 0.395 |
Female, n (%) | 235 (53.9%) | 51 (54.3%) | 184 (53.8%) | >0.999 | 30 (56.6%) | 205 (53.5%) | 0.784 |
BMI, mean ± SD | 23.4 ± 3.4 | 23.17 ± 3.45 | 23.40 ± 3.44 | 0.569 | 23.0 ± 3.1 | 23.4 ± 3.5 | 0.488 |
Risk factors, n (%) | |||||||
Hypertension | 125 (28.7%) | 39 (41.5%) | 86 (25.1%) | 0.003 | 6 (11.3%) | 119 (31.1%) | 0.005 |
Diabetes mellitus | 39 (8.9%) | 12 (12.8%) | 27 (7.9%) | 0.207 | 1 (1.9%) | 38 (9.9%) | 0.096 |
History of stroke | 19 (4.4%) | 7 (7.4%) | 12 (3.5%) | 0.170 | 0 (0.0%) | 19 (5.0%) | 0.194 |
CKDa | 24 (5.5%) | 15 (16%) | 9 (2.6%) | <0.001 | 2 (3.8%) | 22 (5.7%) | 0.789 |
Coronary artery disease | 29 (6.7%) | 11 (11.7%) | 18 (5.3%) | 0.047 | 2 (3.8%) | 27 (7.0%) | 0.547 |
Dyslipidaemia | 22 (5.0%) | 6 (6.4%) | 16 (4.7%) | 0.687 | 1 (1.9%) | 21 (5.5%) | 0.432 |
Emergency operation | 2 (0.5%) | 1 (1.1%) | 1 (0.3%) | 0.906 | 0 (0.0%) | 2 (0.5%) | >0.999 |
Previous cardiac surgery | 152 (34.9%) | 29 (30.9%) | 123 (36%) | 0.424 | 30 (56.6%) | 122 (31.9%) | 0.001 |
Atrial fibrillation | 98 (22.5%) | 31 (33%) | 67 (19.6%) | 0.009 | 7 (13.2%) | 91 (23.8%) | 0.121 |
Aetiology | |||||||
Rheumatic | 28 | 1 | 27 | 28 | 2 | 26 | |
Degenerative | 399 | 47 | 352 | 399 | 28 | 371 | |
Ischaemic | 3 | 2 | 1 | 3 | 0 | 3 | |
Functional | 3 | 0 | 3 | 3 | 0 | 3 | |
Endocarditis, n (%) | 3 (0.7%) | 0 (0.0%) | 3 (0.9%) | 0.836 | 0 (0.0%) | 3 (0.8%) | >0.999 |
LVESD, mean ± SD | 39.2 ± 7.8 | 41.5 ± 8.8 | 38.6 ± 7.4 | 0.003 | 40.2 ± 9.2 | 39.1 ± 7.6 | 0.400 |
LVEDD, mean ± SD | 61.1 ± 8.1 | 62.8 ± 8.4 | 60.7 ± 8.0 | 0.025 | 62.5 ± 9.4 | 60.9 ± 7.9 | 0.181 |
EF, mean ± SD | 60.0 ± 9.0 | 57.3 ± 10.3 | 60.6 ± 8.4 | 0.006 | 60.9 ± 10.7 | 59.7 ± 8.7 | 0.440 |
LA, mean ± SD | 55.8 ± 10.9 | 59.2 ± 10.2 | 54.9 ± 11.0 | 0.001 | 57.0 ± 10.9 | 55.7 ± 11.0 | 0.418 |
Complex disease | 187 (42.9%) | 38 (40.4%) | 149 (43.6%) | 0.669 | 36 (67.9%) | 151 (39.4%) | <0.001 |
IABP, n (%) | 15 (3.4%) | 4 (4.3%) | 11 (3.2%) | 0.865 | 1 (1.9%) | 22 (5.7%) | 0.937 |
ECMO, n (%) | 4 (0.9%) | 2 (2.1%) | 2 (0.6%) | 0.103 | 0 (0.0%) | 4 (1.0%) | >0.999 |
Variables . | Total (n = 436) . | Mortality (n = 94) . | Non-mortality (n = 342) . | P-value . | Recurrence (n = 53) . | Non-recurrence (n = 383) . | P-value . |
---|---|---|---|---|---|---|---|
Age (years), mean ± SD | 54.7 ± 15.4 | 64.2 ± 11.4 | 52.2 ± 15.3 | <0.001 | 53.1 ± 15.3 | 55.0 ± 15.4 | 0.395 |
Female, n (%) | 235 (53.9%) | 51 (54.3%) | 184 (53.8%) | >0.999 | 30 (56.6%) | 205 (53.5%) | 0.784 |
BMI, mean ± SD | 23.4 ± 3.4 | 23.17 ± 3.45 | 23.40 ± 3.44 | 0.569 | 23.0 ± 3.1 | 23.4 ± 3.5 | 0.488 |
Risk factors, n (%) | |||||||
Hypertension | 125 (28.7%) | 39 (41.5%) | 86 (25.1%) | 0.003 | 6 (11.3%) | 119 (31.1%) | 0.005 |
Diabetes mellitus | 39 (8.9%) | 12 (12.8%) | 27 (7.9%) | 0.207 | 1 (1.9%) | 38 (9.9%) | 0.096 |
History of stroke | 19 (4.4%) | 7 (7.4%) | 12 (3.5%) | 0.170 | 0 (0.0%) | 19 (5.0%) | 0.194 |
CKDa | 24 (5.5%) | 15 (16%) | 9 (2.6%) | <0.001 | 2 (3.8%) | 22 (5.7%) | 0.789 |
Coronary artery disease | 29 (6.7%) | 11 (11.7%) | 18 (5.3%) | 0.047 | 2 (3.8%) | 27 (7.0%) | 0.547 |
Dyslipidaemia | 22 (5.0%) | 6 (6.4%) | 16 (4.7%) | 0.687 | 1 (1.9%) | 21 (5.5%) | 0.432 |
Emergency operation | 2 (0.5%) | 1 (1.1%) | 1 (0.3%) | 0.906 | 0 (0.0%) | 2 (0.5%) | >0.999 |
Previous cardiac surgery | 152 (34.9%) | 29 (30.9%) | 123 (36%) | 0.424 | 30 (56.6%) | 122 (31.9%) | 0.001 |
Atrial fibrillation | 98 (22.5%) | 31 (33%) | 67 (19.6%) | 0.009 | 7 (13.2%) | 91 (23.8%) | 0.121 |
Aetiology | |||||||
Rheumatic | 28 | 1 | 27 | 28 | 2 | 26 | |
Degenerative | 399 | 47 | 352 | 399 | 28 | 371 | |
Ischaemic | 3 | 2 | 1 | 3 | 0 | 3 | |
Functional | 3 | 0 | 3 | 3 | 0 | 3 | |
Endocarditis, n (%) | 3 (0.7%) | 0 (0.0%) | 3 (0.9%) | 0.836 | 0 (0.0%) | 3 (0.8%) | >0.999 |
LVESD, mean ± SD | 39.2 ± 7.8 | 41.5 ± 8.8 | 38.6 ± 7.4 | 0.003 | 40.2 ± 9.2 | 39.1 ± 7.6 | 0.400 |
LVEDD, mean ± SD | 61.1 ± 8.1 | 62.8 ± 8.4 | 60.7 ± 8.0 | 0.025 | 62.5 ± 9.4 | 60.9 ± 7.9 | 0.181 |
EF, mean ± SD | 60.0 ± 9.0 | 57.3 ± 10.3 | 60.6 ± 8.4 | 0.006 | 60.9 ± 10.7 | 59.7 ± 8.7 | 0.440 |
LA, mean ± SD | 55.8 ± 10.9 | 59.2 ± 10.2 | 54.9 ± 11.0 | 0.001 | 57.0 ± 10.9 | 55.7 ± 11.0 | 0.418 |
Complex disease | 187 (42.9%) | 38 (40.4%) | 149 (43.6%) | 0.669 | 36 (67.9%) | 151 (39.4%) | <0.001 |
IABP, n (%) | 15 (3.4%) | 4 (4.3%) | 11 (3.2%) | 0.865 | 1 (1.9%) | 22 (5.7%) | 0.937 |
ECMO, n (%) | 4 (0.9%) | 2 (2.1%) | 2 (0.6%) | 0.103 | 0 (0.0%) | 4 (1.0%) | >0.999 |
CKD is adapted from the definition of chronic kidney disease by The Kidney Disease: Improving Global Outcomes Work Group.
BMI: body mass index; CKD: chronic kidney disease; ECMO: extracorporeal membrane oxygenation; EF: ejection fraction; IABP: intra-aortic balloon pump; LA: left atrium; LVEDD: left ventricular end-diastolic dimension; LVESD: left ventricular end-systolic dimension; SD: standard deviation.
Variables . | N = 436 . |
---|---|
CPB time (min), mean ± SD | 132 ± 43 |
ACC time (min), mean ± SD | 89 ± 32 |
Repair technique, n (%) | |
Annuloplasty | 393 (90.1%) |
Artificial chordae implantation | 87 (20.0%) |
Triangular resection | 49 (11.2%) |
Quadrangular resection | 81 (18.6%) |
Commissural obliteration | 53 (12.2%) |
Secondary chordae resection | 27 (6.2%) |
Commissurotomy | 26 (6.0%) |
Chordae transfer | 17 (3.9%) |
Concomitant procedure, n (%) | |
Aortic valve-related procedure | 20 (4.6%) |
Tricuspid valve-related procedure | 82 (18.8%) |
Arrhythmia surgery | 120 (27.5%) |
CABG | 16 (3.6%) |
Aorta | 7 (1.6%) |
Variables . | N = 436 . |
---|---|
CPB time (min), mean ± SD | 132 ± 43 |
ACC time (min), mean ± SD | 89 ± 32 |
Repair technique, n (%) | |
Annuloplasty | 393 (90.1%) |
Artificial chordae implantation | 87 (20.0%) |
Triangular resection | 49 (11.2%) |
Quadrangular resection | 81 (18.6%) |
Commissural obliteration | 53 (12.2%) |
Secondary chordae resection | 27 (6.2%) |
Commissurotomy | 26 (6.0%) |
Chordae transfer | 17 (3.9%) |
Concomitant procedure, n (%) | |
Aortic valve-related procedure | 20 (4.6%) |
Tricuspid valve-related procedure | 82 (18.8%) |
Arrhythmia surgery | 120 (27.5%) |
CABG | 16 (3.6%) |
Aorta | 7 (1.6%) |
ACC: aortic cross-clamp; CABG: coronary artery bypass grafting; CPB: cardiopulmonary bypass; SD: standard deviation.
Variables . | N = 436 . |
---|---|
CPB time (min), mean ± SD | 132 ± 43 |
ACC time (min), mean ± SD | 89 ± 32 |
Repair technique, n (%) | |
Annuloplasty | 393 (90.1%) |
Artificial chordae implantation | 87 (20.0%) |
Triangular resection | 49 (11.2%) |
Quadrangular resection | 81 (18.6%) |
Commissural obliteration | 53 (12.2%) |
Secondary chordae resection | 27 (6.2%) |
Commissurotomy | 26 (6.0%) |
Chordae transfer | 17 (3.9%) |
Concomitant procedure, n (%) | |
Aortic valve-related procedure | 20 (4.6%) |
Tricuspid valve-related procedure | 82 (18.8%) |
Arrhythmia surgery | 120 (27.5%) |
CABG | 16 (3.6%) |
Aorta | 7 (1.6%) |
Variables . | N = 436 . |
---|---|
CPB time (min), mean ± SD | 132 ± 43 |
ACC time (min), mean ± SD | 89 ± 32 |
Repair technique, n (%) | |
Annuloplasty | 393 (90.1%) |
Artificial chordae implantation | 87 (20.0%) |
Triangular resection | 49 (11.2%) |
Quadrangular resection | 81 (18.6%) |
Commissural obliteration | 53 (12.2%) |
Secondary chordae resection | 27 (6.2%) |
Commissurotomy | 26 (6.0%) |
Chordae transfer | 17 (3.9%) |
Concomitant procedure, n (%) | |
Aortic valve-related procedure | 20 (4.6%) |
Tricuspid valve-related procedure | 82 (18.8%) |
Arrhythmia surgery | 120 (27.5%) |
CABG | 16 (3.6%) |
Aorta | 7 (1.6%) |
ACC: aortic cross-clamp; CABG: coronary artery bypass grafting; CPB: cardiopulmonary bypass; SD: standard deviation.
Early outcomes
Operative mortality occurred in 4 patients (0.9%; Supplementary Material, Table S1). Postoperative complications were as follows: low cardiac output syndrome (n = 23, 5.3%), respiratory complications (n = 31, 7.1%), acute kidney injury (n = 22, 5.0%), postoperative bleeding that required reoperation (n = 11, 2.5%), stroke (n = 8, 1.8%) and mediastinitis (n = 5, 1.1%).
Long-term survival
Late death occurred in 88 patients. The 10- and 20-year overall survival rates were 86.1% and 69.9%, respectively. Multivariate Cox analyses showed that age, diabetes mellitus, chronic kidney disease, left ventricle ejection fraction and emergency surgery were significantly associated with overall survival (Table 3). The evaluation results for each model are shown in Table 4. The C-index of RSF was 0.815, which was the highest among 5 models studied. Compared to that of the Cox model, the C-indices of the RSF model and XGBoost-based survival models were higher. In terms of C-index, the overall performance of the XGBoost and RSF models was better than that of the Cox model.
Cox proportional hazards models for factors associated with overall survival and mitral regurgitation recurrence, respectively
Variablesa . | Univariate analysis . | Multivariate analysis . | ||||
---|---|---|---|---|---|---|
HR . | 95% CI . | P-value . | HR . | 95% CI . | P-value . | |
Factors associated with overall survival | ||||||
Age (years) | 1.110 | 1.077–1.124 | <0.001 | 1.093 | 1.068–1.119 | <0.001 |
Diabetes mellitus | 2.120 | 1.147–3.916 | 0.016 | 2.642 | 1.196–5.838 | 0.018 |
Hypertension | 2.725 | 1.784 –4.162 | <0.001 | 1.501 | 0.952–2.367 | 0.081 |
Stroke | 4.011 | 1.819–8.845 | <0.001 | 0.949 | 0.368–2.447 | 0.914 |
Dyslipidaemia | 2.425 | 1.048 –5.612 | 0.039 | 0.565 | 0.216–1.473 | 0.243 |
Chronic kidney disease | 8.516 | 4.790–15.140 | <0.001 | 7.135 | 3.535–14.401 | <0.001 |
CAD | 3.534 | 1.850–6.752 | <0.001 | 1.707 | 0.781–3.727 | 0.180 |
Atrial fibrillation | 4.269 | 2.636–6.914 | <0.001 | 1.201 | 0.683–2.113 | 0.524 |
Emergency operation | 7.425 | 1.026–53.750 | 0.047 | 11.581 | 1.040–128.968 | 0.046 |
ECMO | 18.920 | 5.772–62.000 | <0.001 | 4.222 | 0.517–34.444 | 0.179 |
Ejection fraction | 0.965 | 0.945–0.986 | <0.001 | 0.971 | 0.949–0.993 | 0.010 |
LA size | 1.034 | 1.016–1.052 | <0.001 | 1.018 | 0.999–1.037 | 0.058 |
Ischaemic aetiology | 7.719 | 2.777–21.460 | <0.001 | 0.597 | 0.045–8.000 | 0.697 |
Factors associated with recurrence | ||||||
Age (years) | 1.016 | 1.000–1.032 | 0.058 | 1.019 | 1.001–1.038 | 0.043 |
CHF | 3.787 | 1.123–12.77 | 0.032 | 3.029 | 0.717–12.806 | 0.132 |
Complexity | 1.811 | 0.983–3.338 | 0.057 | 2.058 | 1.112–3.808 | 0.022 |
MAP | 0.576 | 0.306–1.082 | 0.086 | 0.412 | 0.185–0.921 | 0.031 |
LA size | 1.020 | 0.997–1.043 | 0.094 | 1.019 | 0.994–1.044 | 0.145 |
Variablesa . | Univariate analysis . | Multivariate analysis . | ||||
---|---|---|---|---|---|---|
HR . | 95% CI . | P-value . | HR . | 95% CI . | P-value . | |
Factors associated with overall survival | ||||||
Age (years) | 1.110 | 1.077–1.124 | <0.001 | 1.093 | 1.068–1.119 | <0.001 |
Diabetes mellitus | 2.120 | 1.147–3.916 | 0.016 | 2.642 | 1.196–5.838 | 0.018 |
Hypertension | 2.725 | 1.784 –4.162 | <0.001 | 1.501 | 0.952–2.367 | 0.081 |
Stroke | 4.011 | 1.819–8.845 | <0.001 | 0.949 | 0.368–2.447 | 0.914 |
Dyslipidaemia | 2.425 | 1.048 –5.612 | 0.039 | 0.565 | 0.216–1.473 | 0.243 |
Chronic kidney disease | 8.516 | 4.790–15.140 | <0.001 | 7.135 | 3.535–14.401 | <0.001 |
CAD | 3.534 | 1.850–6.752 | <0.001 | 1.707 | 0.781–3.727 | 0.180 |
Atrial fibrillation | 4.269 | 2.636–6.914 | <0.001 | 1.201 | 0.683–2.113 | 0.524 |
Emergency operation | 7.425 | 1.026–53.750 | 0.047 | 11.581 | 1.040–128.968 | 0.046 |
ECMO | 18.920 | 5.772–62.000 | <0.001 | 4.222 | 0.517–34.444 | 0.179 |
Ejection fraction | 0.965 | 0.945–0.986 | <0.001 | 0.971 | 0.949–0.993 | 0.010 |
LA size | 1.034 | 1.016–1.052 | <0.001 | 1.018 | 0.999–1.037 | 0.058 |
Ischaemic aetiology | 7.719 | 2.777–21.460 | <0.001 | 0.597 | 0.045–8.000 | 0.697 |
Factors associated with recurrence | ||||||
Age (years) | 1.016 | 1.000–1.032 | 0.058 | 1.019 | 1.001–1.038 | 0.043 |
CHF | 3.787 | 1.123–12.77 | 0.032 | 3.029 | 0.717–12.806 | 0.132 |
Complexity | 1.811 | 0.983–3.338 | 0.057 | 2.058 | 1.112–3.808 | 0.022 |
MAP | 0.576 | 0.306–1.082 | 0.086 | 0.412 | 0.185–0.921 | 0.031 |
LA size | 1.020 | 0.997–1.043 | 0.094 | 1.019 | 0.994–1.044 | 0.145 |
All variables in Table 1 were analysed and factors that entered into the multivariable analysis were shown.
CAD: coronary artery disease; CHF: congestive heart failure; CI: confidence interval; ECMO: extracorporeal membrane oxygenator; HR: hazard ratio; LA: left atrium; MAP: mitral annuloplasty; LA: left atrium.
Cox proportional hazards models for factors associated with overall survival and mitral regurgitation recurrence, respectively
Variablesa . | Univariate analysis . | Multivariate analysis . | ||||
---|---|---|---|---|---|---|
HR . | 95% CI . | P-value . | HR . | 95% CI . | P-value . | |
Factors associated with overall survival | ||||||
Age (years) | 1.110 | 1.077–1.124 | <0.001 | 1.093 | 1.068–1.119 | <0.001 |
Diabetes mellitus | 2.120 | 1.147–3.916 | 0.016 | 2.642 | 1.196–5.838 | 0.018 |
Hypertension | 2.725 | 1.784 –4.162 | <0.001 | 1.501 | 0.952–2.367 | 0.081 |
Stroke | 4.011 | 1.819–8.845 | <0.001 | 0.949 | 0.368–2.447 | 0.914 |
Dyslipidaemia | 2.425 | 1.048 –5.612 | 0.039 | 0.565 | 0.216–1.473 | 0.243 |
Chronic kidney disease | 8.516 | 4.790–15.140 | <0.001 | 7.135 | 3.535–14.401 | <0.001 |
CAD | 3.534 | 1.850–6.752 | <0.001 | 1.707 | 0.781–3.727 | 0.180 |
Atrial fibrillation | 4.269 | 2.636–6.914 | <0.001 | 1.201 | 0.683–2.113 | 0.524 |
Emergency operation | 7.425 | 1.026–53.750 | 0.047 | 11.581 | 1.040–128.968 | 0.046 |
ECMO | 18.920 | 5.772–62.000 | <0.001 | 4.222 | 0.517–34.444 | 0.179 |
Ejection fraction | 0.965 | 0.945–0.986 | <0.001 | 0.971 | 0.949–0.993 | 0.010 |
LA size | 1.034 | 1.016–1.052 | <0.001 | 1.018 | 0.999–1.037 | 0.058 |
Ischaemic aetiology | 7.719 | 2.777–21.460 | <0.001 | 0.597 | 0.045–8.000 | 0.697 |
Factors associated with recurrence | ||||||
Age (years) | 1.016 | 1.000–1.032 | 0.058 | 1.019 | 1.001–1.038 | 0.043 |
CHF | 3.787 | 1.123–12.77 | 0.032 | 3.029 | 0.717–12.806 | 0.132 |
Complexity | 1.811 | 0.983–3.338 | 0.057 | 2.058 | 1.112–3.808 | 0.022 |
MAP | 0.576 | 0.306–1.082 | 0.086 | 0.412 | 0.185–0.921 | 0.031 |
LA size | 1.020 | 0.997–1.043 | 0.094 | 1.019 | 0.994–1.044 | 0.145 |
Variablesa . | Univariate analysis . | Multivariate analysis . | ||||
---|---|---|---|---|---|---|
HR . | 95% CI . | P-value . | HR . | 95% CI . | P-value . | |
Factors associated with overall survival | ||||||
Age (years) | 1.110 | 1.077–1.124 | <0.001 | 1.093 | 1.068–1.119 | <0.001 |
Diabetes mellitus | 2.120 | 1.147–3.916 | 0.016 | 2.642 | 1.196–5.838 | 0.018 |
Hypertension | 2.725 | 1.784 –4.162 | <0.001 | 1.501 | 0.952–2.367 | 0.081 |
Stroke | 4.011 | 1.819–8.845 | <0.001 | 0.949 | 0.368–2.447 | 0.914 |
Dyslipidaemia | 2.425 | 1.048 –5.612 | 0.039 | 0.565 | 0.216–1.473 | 0.243 |
Chronic kidney disease | 8.516 | 4.790–15.140 | <0.001 | 7.135 | 3.535–14.401 | <0.001 |
CAD | 3.534 | 1.850–6.752 | <0.001 | 1.707 | 0.781–3.727 | 0.180 |
Atrial fibrillation | 4.269 | 2.636–6.914 | <0.001 | 1.201 | 0.683–2.113 | 0.524 |
Emergency operation | 7.425 | 1.026–53.750 | 0.047 | 11.581 | 1.040–128.968 | 0.046 |
ECMO | 18.920 | 5.772–62.000 | <0.001 | 4.222 | 0.517–34.444 | 0.179 |
Ejection fraction | 0.965 | 0.945–0.986 | <0.001 | 0.971 | 0.949–0.993 | 0.010 |
LA size | 1.034 | 1.016–1.052 | <0.001 | 1.018 | 0.999–1.037 | 0.058 |
Ischaemic aetiology | 7.719 | 2.777–21.460 | <0.001 | 0.597 | 0.045–8.000 | 0.697 |
Factors associated with recurrence | ||||||
Age (years) | 1.016 | 1.000–1.032 | 0.058 | 1.019 | 1.001–1.038 | 0.043 |
CHF | 3.787 | 1.123–12.77 | 0.032 | 3.029 | 0.717–12.806 | 0.132 |
Complexity | 1.811 | 0.983–3.338 | 0.057 | 2.058 | 1.112–3.808 | 0.022 |
MAP | 0.576 | 0.306–1.082 | 0.086 | 0.412 | 0.185–0.921 | 0.031 |
LA size | 1.020 | 0.997–1.043 | 0.094 | 1.019 | 0.994–1.044 | 0.145 |
All variables in Table 1 were analysed and factors that entered into the multivariable analysis were shown.
CAD: coronary artery disease; CHF: congestive heart failure; CI: confidence interval; ECMO: extracorporeal membrane oxygenator; HR: hazard ratio; LA: left atrium; MAP: mitral annuloplasty; LA: left atrium.
Model performance measured by concordance index of 5 models in stratified five-fold cross-validation
Methods . | C-index . |
---|---|
Overall survival | |
Cox | 0.733207 |
RSF | 0.814996 |
XGBoost | 0.806113 |
SVM | 0.771163 |
GLMnet | 0.785287 |
Recurrence | |
Cox | 0.545301 |
RSF | 0.692364 |
XGBoost | 0.718107 |
SVM | 0.612838 |
GLMnet | 0.602545 |
Methods . | C-index . |
---|---|
Overall survival | |
Cox | 0.733207 |
RSF | 0.814996 |
XGBoost | 0.806113 |
SVM | 0.771163 |
GLMnet | 0.785287 |
Recurrence | |
Cox | 0.545301 |
RSF | 0.692364 |
XGBoost | 0.718107 |
SVM | 0.612838 |
GLMnet | 0.602545 |
C-index: concordance index; RSF: random survival forest; SVM: support vector machine; XGBoost: extreme gradient boost.
Model performance measured by concordance index of 5 models in stratified five-fold cross-validation
Methods . | C-index . |
---|---|
Overall survival | |
Cox | 0.733207 |
RSF | 0.814996 |
XGBoost | 0.806113 |
SVM | 0.771163 |
GLMnet | 0.785287 |
Recurrence | |
Cox | 0.545301 |
RSF | 0.692364 |
XGBoost | 0.718107 |
SVM | 0.612838 |
GLMnet | 0.602545 |
Methods . | C-index . |
---|---|
Overall survival | |
Cox | 0.733207 |
RSF | 0.814996 |
XGBoost | 0.806113 |
SVM | 0.771163 |
GLMnet | 0.785287 |
Recurrence | |
Cox | 0.545301 |
RSF | 0.692364 |
XGBoost | 0.718107 |
SVM | 0.612838 |
GLMnet | 0.602545 |
C-index: concordance index; RSF: random survival forest; SVM: support vector machine; XGBoost: extreme gradient boost.
To determine factors affecting the overall survival of patients who underwent mitral valve repair, we evaluated the importance of features using XGBoost and RSF models. The top 10 most important features ranked in the RSF model were age, chronic kidney disease, ischaemic MR, postoperative pneumonia, LV end-systolic dimension, LV ejection fraction, rheumatic MR atrial fibrillation, postoperative stroke and emergency surgery (Fig. 1). The highest-ranked predictor was age. The top 10 most important features ranked in the XGBoost model were age, rheumatic MR, chronic kidney disease, atrial fibrillation, left atrium size, degenerative MR, hypertension, LV end-systolic dimension, history of previous operation and LV ejection fraction (Fig. 2).
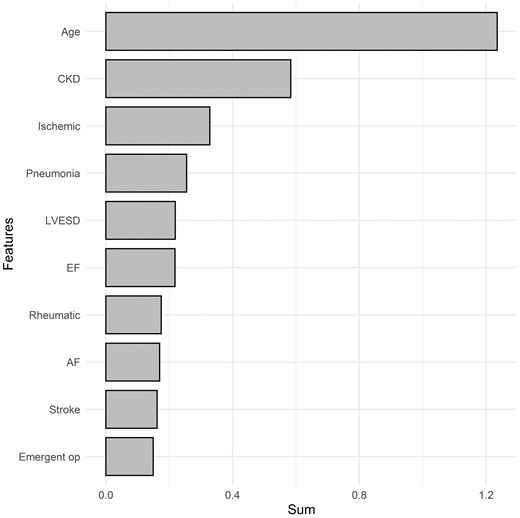
Variable importance of features included in the random survival forest model for the prediction of overall mortality after mitral valve repair. CKD: chronic kidney disease; EF: ejection fraction; Emergent op: emergency operation; IABP: usage of preoperative intra-aortic balloon pump; Ischaemic: ischaemic aetiology; LVESD: left ventricular end-systolic dimension; Rheumatic: rheumatic aetiology. The X-axis represents the sum of variable importance values.
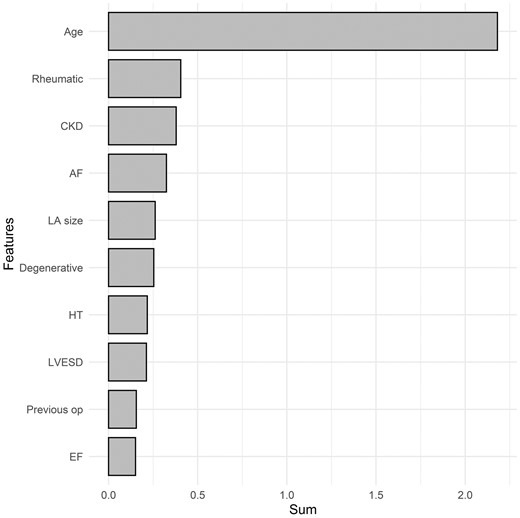
Variable importance of features included in the XGBoost-based survival model for the prediction of overall mortality after mitral valve repair. AF: atrial fibrillation; BMI: body mass index; CKD: chronic kidney disease; Degenerative: degenerative aetiology; EF: ejection fraction; HT: hypertension; LA: left atrium; Previous op: the presence of previous operation. The X-axis represents the sum of variable importance values.
Recurrence of mitral regurgitation
The 10- and 20-year rates of freedom from MR recurrence were 79.6% and 43.4%, respectively. Recurrent MR occurred in 17 (6.8%) and 36 (19.3%) patients with the simple and complex lesions, respectively. The multivariate Cox model showed that age (P = 0.043), complexity of the lesion (P = 0.022) and mitral annuloplasty (P = 0.031) were associated with recurrent MR (Table 3).
The results of each model for recurrent MR are shown in Table 4. The C-index for XGBoost was 0.718, which was the highest among the 5 models. Compared to the Cox model (C-index = 0.545), the C-indices of the XGBoost and RSF models were higher. In terms of C-index, the overall performance of the XGBoost and RSF models was better than Cox model.
To determine the factors influencing MR recurrence among patients who underwent mitral valve repair, we evaluated the importance of features using RSF and XGBoost models. The top 10 most important features in the RSF model were age, LV end-systolic dimension, LV end-diastolic dimension, ejection fraction, artificial chordae implantation, history of previous surgery, left atrium size, body mass index, complexity of the lesion and mitral annuloplasty (Fig. 3). The top 10 most important features in the XGBoost model were age, body mass index, LV end-diastolic dimension, left atrium size, ejection fraction, LV end-systolic volume, sex, artificial chordae implantation, sex, history of previous surgery and hypertension (Fig. 4).
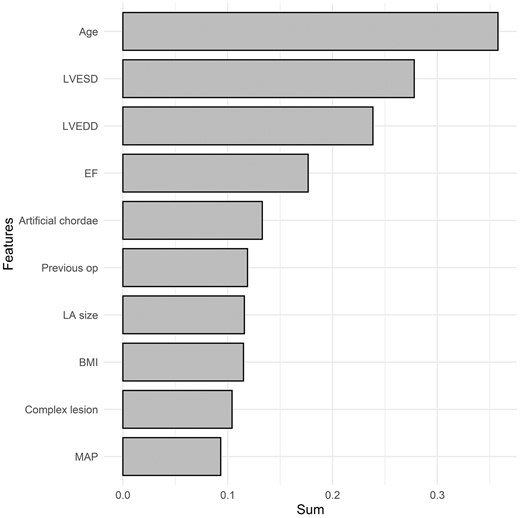
Variable importance of features included in the random survival forest model for the prediction of mitral valve regurgitation after mitral valve repair. BMI: body mass index; CHF: preoperative congestive heart failure; EF: ejection fraction; LA: left atrium; LVEDD: left ventricular end-diastolic dimension; LVESD: left ventricular end-systolic dimension; Previous op: the presence of previous operation. The X-axis represents the sum of variable importance values.
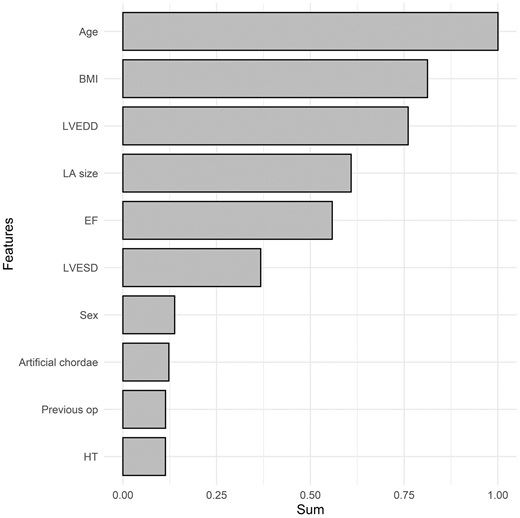
Variable importance of features included in the XGBoost-based survival model for the prediction of mitral valve regurgitation after mitral valve repair. BMI: body mass index; EF: ejection fraction; LA: left atrium; LVEDD: left ventricular end-diastolic dimension; LVESD: left ventricular end-systolic dimension; Previous op: the presence of previous operation. The X-axis represents the sum of variable importance values.
DISCUSSION
In the present study, we developed a machine-learning-based prediction model to assess overall mortality and MR recurrence after mitral valve repair. We found that RSF had the best discrimination for overall mortality. For MR recurrence, XGBoost-based survival model had the best discrimination. Our results are compelling because both models were superior to the Cox model in terms of C-index for the prediction of overall mortality and MR recurrence.
Early surgical correction of severe MR provides a survival benefit [4, 6]. Mitral valve repair is increasingly becoming the standard treatment for patients with MR. However, it remains unclear which clinical parameters are the most important predictors of late mortality and durability after mitral valve repair. For these clinical outcomes, the most commonly applied model is the Cox proportional hazards regression model. However, it is limited to the proportional hazards assumption. Machine-leaning techniques are assumption-free which is effectively used for modelling complex data. Rather than adapting fixed assumptions on data behaviour and variable preselection, machine-learning techniques are able to make the model by detecting underlying patterns [14–16]. Machine learning for big data analysis has revolutionized the conventional cardiovascular research. Its ability to manage large multidimensional and various data could expose novel associations for important outcomes and identify trends that would not have been apparent.
We found several factors associated with overall mortality using multivariable and machine-learning models (Table 4 and Figs 1 and 2). Age, chronic kidney disease and LV ejection fraction were most associated with overall mortality, consistent with previous studies [5, 23]. Although the Cox model included only 3 variables of significance for recurrent MR in this study, the machine-learning models revealed more variables. Complex valve lesions, non-use of annuloplasty rings and lack of chordae implantation were the associated factors, consistent with previous studies [24–26]. Kim et al. [26] have shown that after implementation of artificial chordae implantation, recurrent MR and mitral reoperation both decreased. The techniques of artificial chordae implantation to adjust the appropriate length are demanding and require sufficient time and experience for surgeons (Video 1). Moreover, age, body mass index and sex have been reported as factors associated with recurrent MR [25, 27, 28]. Other echocardiographic factors, including LV end-diastolic dimension, ejection fraction, left atrium size and LV end-systolic volume, have also been reported as independent determinants of recurrent MR [29–32]. Relatively higher recurrence of MR in this study may be attributed to the higher proportion of complex disease and larger LV end-systolic and end-diastolic dimensions (Table 1). Previous studies included lower proportion of complex disease (19.0–38.0%) and smaller mean LV end-systolic and end-diastolic dimensions [30, 31, 33].
Surgical video of mitral valve repair.
Valve repair is a reasonable option even for complex mitral valve pathology [10]. However, based on the results from our study, if the valve pathology is too complex to repair the valve and the patient is old, the surgeon can consider valve replacement rather than repair regarding higher risk of MV recurrence. Gillinov et al. [34] also reported that when repair seems to be infeasible due to severe valvar pathology, valve replacement does not diminish long-term survival.
There have been several mortality prediction models for patients undergoing open heart surgery. However, these prediction models have only relied on conventional statistical methods and did not focus on patients with mitral valve repair. Therefore, a new strategy for patients undergoing mitral valve repair using machine-learning algorithms was necessary.
Machine learning has been used in various fields from diagnosis to prediction. A previous study used machine-learning prediction models for mitral valve reparability and MR recurrence in patients undergoing surgical mitral valve repair [35]. However, they compared logistic machine-learning analysis models (decision tree, random forest, SVM, naive Bayes, extreme gradient boosted trees and multi-layer perception models) with the statistical logistic regression model. To identify time-dependent risk factors for mortality and the durability of mitral valve repair, we adopted a novel survival analysis from data mining, called RSF and compared it to commonly used Cox model. We also used XGB- and SVM-based survival analyses in this study. To our knowledge, this is the first study to use machine-learning-based survival analysis for patients undergoing mitral valve repair.
Limitations
There are several limitations to the present study that must be noted. First, this study was limited by its retrospective design. Second, it was a single-centre study; the models used may not be directly applicable to other centres with different postoperative management or follow-up strategies. However, this study used machine-learning techniques to predict the mortality and MR recurrence rather than to develop a final model for general clinical use. Third, the present study consisted of a relatively modest sample size. However, the median sample size of the previous >200 studies, which used machine learning in intensive care units, was 488 [36], which is similar to our sample size. Therefore, our sample size may be acceptable for a preliminary approach to predict outcomes after mitral valve repair. Fourth, the complexity of machine-learning model may result in difficult interpretation and application into the standard practice. However, we think that the adoption of machine-learning technique, especially artificial intelligence, will soon become widespread in clinical medicine. Fourth, as patients in the early study period with missing values were excluded (n = 7), this may have affected the clinical outcomes. Fifth, further research is required to optimize and validate model performance.
CONCLUSION
In conclusion, this study shows that machine-learning models were able to predict overall mortality and MR recurrence after mitral valve repair. The C-indices of machine-learning models were higher than those of the Cox model. Further validation and application of machine learning into the everyday clinical practice are still warranted for better understanding of its importance.
SUPPLEMENTARY MATERIAL
Supplementary material is available at ICVTS online.
FUNDING
None.
Conflict of interest: none declared.
DATA AVAILABILITY
The data underlying this article cannot be shared publicly due to privacy issues; however, the data will be shared on reasonable request to the corresponding author.
Author Contributions
Yoonjin Kang: Conceptualization; Data curation; Formal analysis; Investigation; Methodology; Project administration; Validation; Writing—original draft; Writing—review & editing. Suk Ho Sohn: Data curation; Supervision; Validation. Jae Woong Choi: Data curation; Supervision; Validation. Ho Young Hwang: Data curation; Formal analysis; Project administration; Supervision; Validation. Kyung Hwan Kim: Conceptualization; Data curation; Formal analysis; Investigation; Methodology; Project administration; Supervision; Validation; Writing—review & editing.
Reviewer information
Interdisciplinary CardioVascular and Thoracic Surgery thanks Rashmi Nedadur, Guido Ascione and the other anonymous reviewer(s) for their contribution to the peer review process of this article.
Presented at the EACTS 36th Annual Meeting in Milan, Italy.
REFERENCES
ABBREVIATIONS
- C-index
Concordance index
- LV
Left ventricular
- MR
Mitral regurgitation
- RSF
Random survival forest
- SVM
Support vector machine
- XGBoost
Extreme gradient boost