-
PDF
- Split View
-
Views
-
Cite
Cite
Marco Alings, Elisa Vireca, Dirk Bastian, Alexander Jacques Wardeh, Christopher Nimeth, Raymond Tukkie, Susanne Trinks, Walter Kainz, Colleen Delaney, Gert Kaltofen, for the AUTOMATICITY Study Investigators, Amphia Ziekenhuis, Poul Erik Bloch-Thomsen, Cestmir Cihalik, Thomas Lawo, Benaissa Agraou, Philippe Deutsch, Patrick Bazin, Yves Guyomar, Marc Bobillier, Pascal Defaye, Alain Amiel, Arnaud Lazarus, Maxime Guenoun, Pierre Le Franc, Fanny L. Oei, D. Nicastia, Stefan Hoenen, A.E. de Porto, Heiner Vontobel, Ramon Robles de Medina, Walter Kainz, Philippe Brunner, Javier Alzueta, Jose Antonio Santos, Alejandro Bellver Navarro, C. Hie, Josef Kautzner, Peter Ammann-Kardiol, Gert Kaltofen, Raymond Tukkie, Eduard Zeindlhofer, Christopher Nimeth, Martin Frömmel, Johannes Brachmann, Konrad Göhl, Susanne Trinks, Peter van der Meer, Gerald Zenker, Franz Gebetsberger, Tilmann Unger, Jacob H. Ruiter, Hemanth Ramanna, K.B. Hadj, Andreas Lang, Diethard Predel, Andreas Schnabel, Martin Martinek, Cheung-Wah Cheng, Kevin Ward, Gunnar Jensen, Sean O'Nunain, Shan Jiang, W.F. Terpstra, Erich Budschedl, D.J. van Doorn, Frédéric Marenne, Thorsten Wahlers, Tobias Zeus, Stefan Osswald, Birger Engby, Per Dahl Christensen, Ge Junbo, Maurits van der Veen, Clinical use of automatic pacemaker algorithms: results of the AUTOMATICITY registry, EP Europace, Volume 13, Issue 7, July 2011, Pages 976–983, https://doi.org/10.1093/europace/eur052
- Share Icon Share
Abstract
Follow-up of the ever-increasing numbers of patients with implantable cardiac devices places a heavy burden on clinical departments. Device automaticity may alleviate the follow-up burden by minimizing the time for physician involvement. The aim of the prospective, multicentre AUTOMATICITY registry was to examine the performance of a subset of programmed automatic algorithms during patient follow-up and their acceptance by implanting physicians.
The clinical use of automatic algorithms from the InsigniaTM pacemakers (PM; Boston Scientific, St Paul, MN, USA) was evaluated: atrial and ventricular AutoSense (sensitivity adjustment), ventricular Automatic Capture (threshold verification and output setting), AutoLifeStyleTM (sensor settings adjustment). The objective of the study was to assess the reprogramming rates within 12 months of implant, the reasons for reprogramming and relationship to adverse events. A total of 960 patients were enrolled in the study. The proportion of patients free from any algorithm reprogramming at 12 months was 86.1%. A total of 2736 algorithms were activated at enrolment, with 156 (5.7%) being reprogrammed in 115 patients at 12 months for any reason. Forty-nine reprogrammings (1.8%) were unintentional or related to changes in device settings such that the algorithm was no longer available, 33 (1.2%) were due to suspected sensing issues, and 22 (0.8%) were assumed related to the algorithm. The individual 12-month reprogramming-free rates were: ventricular AutoSense 94.3%, Atrial AutoSense 93.3%, AutoLifeStyleTM 93.9%, and Automatic Capture 95.9%.
The results of the AUTOMATICITY registry show that automatic measurement of key settings and automatic adjustment to optimal programming is feasible and safe. The simplicity of PM follow-up and avoidance of frequent reprogramming may contribute to a more effective use of hospital time and resources.
Introduction
The rapidly expanding indications for the implantation of permanent cardiac devices have resulted in an increased prevalence of patients implanted with implantable pacemakers (PM) or implantable cardioverter defibrillators (ICD). Over the course of the 1990s, the number of patients living in the USA with a PM increased by 22%.1 A similar trend is observed in Europe. The European Medical Technology Industry Association (EUCOMED) has measured a growth rate of ∼9% per year between 2005 and 2008 in the market of all cardiac implantable devices.2 Despite their increasing number, PM patients need to be offered a safe and good quality of medical care. The follow-up of these patients and their devices places a heavy burden on clinics and cardiology departments with significant implications for clinical and hospital resources.
In addition, cardiac PM have become more sophisticated, requiring adequately trained physicians in order to appropriately program and follow-up specific functions and algorithms implemented into these devices.
The option of automatic and continuous adjustments of PM functions is more necessary the more device complexity increases. In this context, device automaticity may alleviate the burden of follow-up visits, by minimizing the time for physician involvement, thus contributing to the optimization of healthcare resources and improving the effectiveness of hospital services.
The newest generations of PM and ICDs are able to automatically execute tests that are performed manually at the outpatient clinic, such as battery status, lead impedances, sensing, and capture thresholds.
These automatic features (collectively termed ‘automaticity algorithms’), include beat-to-beat capture verification, sensitivity threshold adjustment, and dynamic adaptation of maximum sensor rate. Although the safety, efficacy, and clinical benefit of the automaticity algorithms have been determined in previous studies,3–13 there is no large cohort of data available looking at the standard usage and acceptance of the combined suite of algorithms in the medical community. The AUTOMATICITY registry has been designed to examine the performance of a subset of programmed automatic algorithms during patient follow-up and their acceptance by implanting physicians.
Methods
The AUTOMATICITY registry is an observational, prospective, multi-site, non-randomized study that was conducted at 62 European and 3 Chinese centres. The study population consisted of patients with indication for permanent pacing, who were implanted with any models of Insignia I™/Nexus I™ Ultra or Insignia I™/Nexus I™ AVT pacemakers (Boston Scientific, Cardiac Rhythm Management, St Paul, MN, USA). Eligible patients had at least one automaticity algorithm programmed to the automatic mode (ON/AUTO) at the pre-discharge visit. The actual number of automaticity algorithms that could have been programmed ON/AUTO in a given patient was dependent on the individually implanted device model. Patients whose device did not feature any automaticity algorithms, or patients who were discharged with no automaticity algorithm programmed ON/AUTO, per physician discretion, were not eligible for inclusion in this study. The study was conducted in accordance with the stipulations of the Declaration of Helsinki. All eligible patients were required to provide written informed consent prior to enrolment and the registry was approved by the responsible Ethics Committee/Institutional Review Boards as applicable. The study design has been previously described in detail.14
Briefly, the objective of the registry was to assess the reprogramming rate of any of the automaticity algorithms within 12 months after device implant, as well as the reprogramming rates per each individual algorithm. Therefore, percentage changes in programming, time to first reprogramming and adverse events were collected and prospectively evaluated with respect to the automaticity algorithms. The settings of each algorithm were documented by means of device printouts and all algorithm settings entered in the study database were verified against this source.
Patients were followed according to the standard clinical practice of the enrolling institution both in terms of visits scheduling and device management. Device programming was collected at each follow-up for 12-month post-implant.
The observational nature of the study design was intended to reduce the study influences on programming modifications of these algorithms and as such, to mimic, as closely as possible, the standard clinical practice regarding the usage of these features.
The automaticity algorithms to be evaluated in this study enable right-ventricular (RV) automatic capture verification and threshold testing (Automatic Capture), automatic sensitivity adjustment (AutoSense), and automatic adjustment of rate response sensors (both minute ventilation and accelerometer; AutoLifeStyleTM). Detailed algorithm description is included in the study design publication.14
Finally, in order to better understand the physician's perception of the automatic algorithms, investigators were asked to provide a reason for each reprogramming that was performed. Details about reprogramming were cross-linked to the reported adverse events to collect information on the causes of the programming change.
Statistical analysis
Statistical methods have been previously published.14 The original statistical plan of the study defined that a standard error of 1% was appropriately sensitive to provide validation of the endpoint. Originally, a maximum enrolment of 2000 patients was planned, assuming that the standard error of the proportion of patients who would need to be reprogrammed to a manual setting would be 0.012 in the absence of censoring. To account for the possibility that the standard error would approach this value at a lower sample size, interim analyses were foreseen to recalculate the standard error and therefore re-estimate the sample size. Based on the interim analysis performed on March 2008 and including 504 patients, the maximum sample size was re-estimated to be 960 patients.
A Kaplan–Meier analysis was performed to estimate both the overall (all algorithms) and the individual algorithm reprogramming-free rates at 12 months. To be included in the overall analysis, patients must have had all the algorithms programmed to ON/AUTO at pre-discharge visit, based on the feature availability in the device and the patient's clinical indication. If a particular algorithm was available, but not turned on at pre-discharge, these patients were removed from the overall analysis and from that individual algorithm analysis. They were only included in the individual analyses for those algorithms that were turned ON/AUTO.
Device reprogramming of any algorithm during follow-up was considered as an event. Patients who did not have any of the algorithms reprogrammed were censored either at 12 months of follow-up or at their death/withdrawal time if they died or withdrew prior to 12 months of follow-up. Due to the observational design of the study, those follow-up visits falling into a window of 12 ± 3 months were considered acceptable to document the patient's programmed parameters at 12 months. Kaplan–Meier analyses were also performed for each of the individual algorithms. To be included in the individual analyses, patients must have had each individual algorithm programmed to ON/AUTO at pre-discharge. Censoring rules were the same for these individual analyses as for the overall analysis.
Results
Patient data
Nine hundred and sixty patients were implanted with an Insignia/Nexus pacemaker between December 2006 and July 2009 in the centres participating in the study; the patients signed an approved Patient Informed Consent and were enrolled in the study. Nineteen patients were excluded from data analysis due to premature closure of two study sites, as the investigators left the study centre. Twenty-six patients were excluded from analyses as they did not have any automatic algorithm programmed to ON/AUTO at hospital pre-discharge but only at a later time. The remaining 915 patients had at least one automatic algorithm active at hospital discharge and were included in study analyses; of them, 817 had all available automaticity algorithms programmed to ON/AUTO at the time of discharge from the hospital and were considered for the overall analysis. Based on the algorithms that were available in the implanted devices and were activated based on the clinical indications of the patients, the numbers of patients included in the individual algorithm analyses are as follows: 535 patients for atrial AutoSense; 884 patients for ventricular AutoSense; 887 patients for Automatic Capture; and 370 patients for AutoLifeStyleTM. Patient characteristics (age, gender, and rhythm disease) and programmed pacing modes are shown in Table 1, leads characteristics are presented in Table 2.
Patient characteristics and programmed pacing modes for the enrolled patients
Characteristic . | Measurement . | Result . |
---|---|---|
Age at implant (years) | Mean ± SD | 75.3 ± 10.5 |
Range | 23.0 − 98.0 | |
Gender [n (%)] | Male | 530 (58%) |
Female | 385 (42%) | |
Rhythm disease | n | % of patientsa |
Sick sinus syndrome | 281 | 30.7 |
3° AV block | 278 | 30.4 |
Atrial arrhythmias | 262 | 28.6 |
2° AV block | 148 | 16.2 |
Brady–Tachy syndrome | 39 | 4.3 |
Bradycardia | 17 | 1.9 |
Other conduction blocks | 13 | 1.4 |
Sinus node diseases | 8 | 0.9 |
Syncope | 8 | 0.9 |
1° AV block | 6 | 0.7 |
Neuro-mediated | 2 | 0.2 |
Others | 18 | 2 |
Unknown | 22 | 2.4 |
Programmed pacing mode | n | % of patients |
DDDR | 321 | 35.1 |
DDD | 274 | 29.9 |
VVIR | 206 | 22.5 |
VVI | 83 | 9.1 |
VDD | 20 | 2.2 |
AAIR | 6 | 0.7 |
DDI | 3 | 0.3 |
DDIR | 2 | 0.2 |
Characteristic . | Measurement . | Result . |
---|---|---|
Age at implant (years) | Mean ± SD | 75.3 ± 10.5 |
Range | 23.0 − 98.0 | |
Gender [n (%)] | Male | 530 (58%) |
Female | 385 (42%) | |
Rhythm disease | n | % of patientsa |
Sick sinus syndrome | 281 | 30.7 |
3° AV block | 278 | 30.4 |
Atrial arrhythmias | 262 | 28.6 |
2° AV block | 148 | 16.2 |
Brady–Tachy syndrome | 39 | 4.3 |
Bradycardia | 17 | 1.9 |
Other conduction blocks | 13 | 1.4 |
Sinus node diseases | 8 | 0.9 |
Syncope | 8 | 0.9 |
1° AV block | 6 | 0.7 |
Neuro-mediated | 2 | 0.2 |
Others | 18 | 2 |
Unknown | 22 | 2.4 |
Programmed pacing mode | n | % of patients |
DDDR | 321 | 35.1 |
DDD | 274 | 29.9 |
VVIR | 206 | 22.5 |
VVI | 83 | 9.1 |
VDD | 20 | 2.2 |
AAIR | 6 | 0.7 |
DDI | 3 | 0.3 |
DDIR | 2 | 0.2 |
Please note that the number of patients programmed to a rate response mode (535) is higher than the number or patients using AutoLifeStyleTM (370): this difference can be explained as some device models did not feature this automatic algorithm.
aPer cents add to more than 100 due to patients possibly having more than one indication.
Patient characteristics and programmed pacing modes for the enrolled patients
Characteristic . | Measurement . | Result . |
---|---|---|
Age at implant (years) | Mean ± SD | 75.3 ± 10.5 |
Range | 23.0 − 98.0 | |
Gender [n (%)] | Male | 530 (58%) |
Female | 385 (42%) | |
Rhythm disease | n | % of patientsa |
Sick sinus syndrome | 281 | 30.7 |
3° AV block | 278 | 30.4 |
Atrial arrhythmias | 262 | 28.6 |
2° AV block | 148 | 16.2 |
Brady–Tachy syndrome | 39 | 4.3 |
Bradycardia | 17 | 1.9 |
Other conduction blocks | 13 | 1.4 |
Sinus node diseases | 8 | 0.9 |
Syncope | 8 | 0.9 |
1° AV block | 6 | 0.7 |
Neuro-mediated | 2 | 0.2 |
Others | 18 | 2 |
Unknown | 22 | 2.4 |
Programmed pacing mode | n | % of patients |
DDDR | 321 | 35.1 |
DDD | 274 | 29.9 |
VVIR | 206 | 22.5 |
VVI | 83 | 9.1 |
VDD | 20 | 2.2 |
AAIR | 6 | 0.7 |
DDI | 3 | 0.3 |
DDIR | 2 | 0.2 |
Characteristic . | Measurement . | Result . |
---|---|---|
Age at implant (years) | Mean ± SD | 75.3 ± 10.5 |
Range | 23.0 − 98.0 | |
Gender [n (%)] | Male | 530 (58%) |
Female | 385 (42%) | |
Rhythm disease | n | % of patientsa |
Sick sinus syndrome | 281 | 30.7 |
3° AV block | 278 | 30.4 |
Atrial arrhythmias | 262 | 28.6 |
2° AV block | 148 | 16.2 |
Brady–Tachy syndrome | 39 | 4.3 |
Bradycardia | 17 | 1.9 |
Other conduction blocks | 13 | 1.4 |
Sinus node diseases | 8 | 0.9 |
Syncope | 8 | 0.9 |
1° AV block | 6 | 0.7 |
Neuro-mediated | 2 | 0.2 |
Others | 18 | 2 |
Unknown | 22 | 2.4 |
Programmed pacing mode | n | % of patients |
DDDR | 321 | 35.1 |
DDD | 274 | 29.9 |
VVIR | 206 | 22.5 |
VVI | 83 | 9.1 |
VDD | 20 | 2.2 |
AAIR | 6 | 0.7 |
DDI | 3 | 0.3 |
DDIR | 2 | 0.2 |
Please note that the number of patients programmed to a rate response mode (535) is higher than the number or patients using AutoLifeStyleTM (370): this difference can be explained as some device models did not feature this automatic algorithm.
aPer cents add to more than 100 due to patients possibly having more than one indication.
RA leads (n = 640) . | RV leads (n = 889) . | ||||
---|---|---|---|---|---|
Manufacturer | n | % | Manufacturer | n | % |
Guidant | 304 | 47.5 | Guidant | 446 | 50.2 |
Medtronic | 148 | 23.1 | Medtronic | 157 | 17.7 |
Pacesetter-St Jude | 108 | 16.9 | Pacesetter-St Jude | 136 | 15.3 |
Vitatron | 29 | 4.5 | Biotronik | 87 | 9.8 |
Biotronik | 26 | 4.1 | ELA/Sorin | 29 | 3.3 |
ELA/Sorin | 14 | 2.2 | Vitatron | 15 | 1.7 |
Intermedics | 8 | 1.3 | Intermedics | 13 | 1.5 |
Medico | 1 | 0.2 | Telectronics | 4 | 0.4 |
Osypka | 1 | 0.2 | Medico | 1 | 0.1 |
Unknown | 1 | 0.2 | unknown | 1 | 0.1 |
RA leads—chronic | 142 | 22.0 | RV leads—chronic | 243 | 27.0 |
Age of chronic RA leads (mean±SD) | 7.5 ± 2.8 years | Age of chronic RV leads (mean ±SD) | 7.5 ± 2.8 years |
RA leads (n = 640) . | RV leads (n = 889) . | ||||
---|---|---|---|---|---|
Manufacturer | n | % | Manufacturer | n | % |
Guidant | 304 | 47.5 | Guidant | 446 | 50.2 |
Medtronic | 148 | 23.1 | Medtronic | 157 | 17.7 |
Pacesetter-St Jude | 108 | 16.9 | Pacesetter-St Jude | 136 | 15.3 |
Vitatron | 29 | 4.5 | Biotronik | 87 | 9.8 |
Biotronik | 26 | 4.1 | ELA/Sorin | 29 | 3.3 |
ELA/Sorin | 14 | 2.2 | Vitatron | 15 | 1.7 |
Intermedics | 8 | 1.3 | Intermedics | 13 | 1.5 |
Medico | 1 | 0.2 | Telectronics | 4 | 0.4 |
Osypka | 1 | 0.2 | Medico | 1 | 0.1 |
Unknown | 1 | 0.2 | unknown | 1 | 0.1 |
RA leads—chronic | 142 | 22.0 | RV leads—chronic | 243 | 27.0 |
Age of chronic RA leads (mean±SD) | 7.5 ± 2.8 years | Age of chronic RV leads (mean ±SD) | 7.5 ± 2.8 years |
RA leads (n = 640) . | RV leads (n = 889) . | ||||
---|---|---|---|---|---|
Manufacturer | n | % | Manufacturer | n | % |
Guidant | 304 | 47.5 | Guidant | 446 | 50.2 |
Medtronic | 148 | 23.1 | Medtronic | 157 | 17.7 |
Pacesetter-St Jude | 108 | 16.9 | Pacesetter-St Jude | 136 | 15.3 |
Vitatron | 29 | 4.5 | Biotronik | 87 | 9.8 |
Biotronik | 26 | 4.1 | ELA/Sorin | 29 | 3.3 |
ELA/Sorin | 14 | 2.2 | Vitatron | 15 | 1.7 |
Intermedics | 8 | 1.3 | Intermedics | 13 | 1.5 |
Medico | 1 | 0.2 | Telectronics | 4 | 0.4 |
Osypka | 1 | 0.2 | Medico | 1 | 0.1 |
Unknown | 1 | 0.2 | unknown | 1 | 0.1 |
RA leads—chronic | 142 | 22.0 | RV leads—chronic | 243 | 27.0 |
Age of chronic RA leads (mean±SD) | 7.5 ± 2.8 years | Age of chronic RV leads (mean ±SD) | 7.5 ± 2.8 years |
RA leads (n = 640) . | RV leads (n = 889) . | ||||
---|---|---|---|---|---|
Manufacturer | n | % | Manufacturer | n | % |
Guidant | 304 | 47.5 | Guidant | 446 | 50.2 |
Medtronic | 148 | 23.1 | Medtronic | 157 | 17.7 |
Pacesetter-St Jude | 108 | 16.9 | Pacesetter-St Jude | 136 | 15.3 |
Vitatron | 29 | 4.5 | Biotronik | 87 | 9.8 |
Biotronik | 26 | 4.1 | ELA/Sorin | 29 | 3.3 |
ELA/Sorin | 14 | 2.2 | Vitatron | 15 | 1.7 |
Intermedics | 8 | 1.3 | Intermedics | 13 | 1.5 |
Medico | 1 | 0.2 | Telectronics | 4 | 0.4 |
Osypka | 1 | 0.2 | Medico | 1 | 0.1 |
Unknown | 1 | 0.2 | unknown | 1 | 0.1 |
RA leads—chronic | 142 | 22.0 | RV leads—chronic | 243 | 27.0 |
Age of chronic RA leads (mean±SD) | 7.5 ± 2.8 years | Age of chronic RV leads (mean ±SD) | 7.5 ± 2.8 years |
Globally, a total of 2736 automatic algorithms were programmed ON/AUTO at hospital discharge in all the study patients.
Reprogramming-free rate
Ninety-eight patients were removed from the overall analysis for having at least one algorithm not active at the time of discharge, leaving 817 patients for the final overall analysis. At 12 months, a total of 156 algorithms (5.7%) had been reprogrammed for any reason in 115 patients. The proportion of patients free from any algorithm reprogramming at 12 months was 86.1% with a lower 95% confidence limit of 84.1% (Figure 1). The 12-month reprogramming-free rates for the individual algorithms were (Figure 2): 94.3% for ventricular AutoSense (93.0% lower confidence bound); 93.3% for atrial AutoSense (91.6% lower confidence bound); 93.9% for AutoLifeStyleTM (91.8% lower confidence bound); and 95.9% for Automatic Capture (94.8% lower confidence bound).
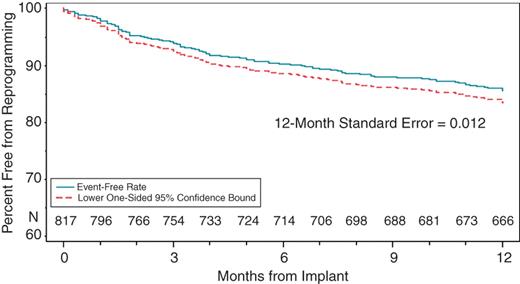
Kaplan–Meier analysis showing the percentage of patients that were free from any algorithm reprogramming. At 12 months the percentage is 86.1% with a lower 95% confidence limit of 84.1%.
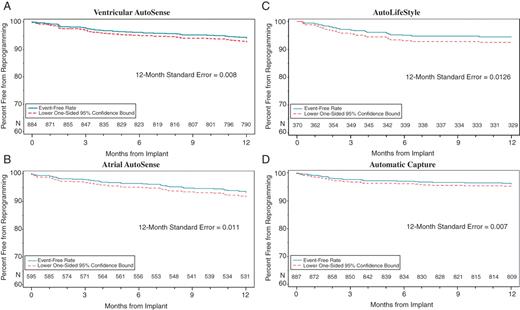
Kaplan–Meier analyses showing the percentage of patients that were free from individual algorithm reprogramming. The 12-month reprogramming-free rate for the individual algorithms were: 94.3% for ventricular AutoSense (93.0% lower confidence bound), 93.3% for atrial AutoSense (91.6% lower confidence bound), 93.9% for AutoLifeStyle (91.8% lower confidence bound), and 95.9% for Automatic Capture (94.8% lower confidence bound). (A) ventricular AutoSense, (B) atrial AutoSense, (C) AutoLifeStyle, and (D) Automatic Capture.
Time to first reprogramming
The analysis of the time of first reprogramming showed that most reprogramming occurred during the first 6 months post-implant, with a remarkable decrease in the second 6 months of the follow-up period. Figure 3 shows the distribution of reprogramming per month of follow-up. Of all reprogrammings, 67.8% occurred in the first 6 months of follow-up, with an average of 13 patients/month experiencing reprogramming, whereas in the second half of the follow-up time only 5.3 patients/month experienced reprogramming.
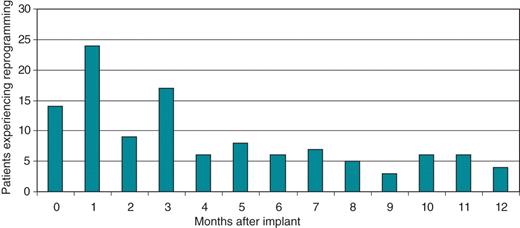
Distribution of reprogrammings per month of follow-up. Of all reprogrammings, 67.8% occurred in the first 6 months of follow-up, with an average of 13 patients/month experiencing reprogramming, whereas in the second half of the follow-up time only 5.3 patients/month experienced reprogramming.
Individual algorithm analyses showed similar results: the majority of the reprogrammings occurred within the first 6 months of follow-up: 77.5% for Automatic Capture; 54.8% for atrial AutoSense; 69.2 % for ventricular AutoSense; and 95.5% for AutoLifeStyleTM.
Reasons for reprogramming
Table 3 shows a list of reasons for reprogramming and their occurrences. One-third of the programming changes (49 of 156) were due to unintentional reprogramming, programming mistakes, or changes in device settings, such that the algorithm was no longer available (e.g. dual-chamber devices programmed to VVI: atrial AutoSense was ‘reprogrammed’ as it was no longer available).
Reasons for reprogramming . | n . | % . |
---|---|---|
Unintentional or algorithm N/A | 49 | 1.8 |
Others—not AE/algorithm related | 42 | 1.5 |
Lead repositioning procedures | 7 | 0.3 |
Other surgical procedures | 3 | 0.1 |
Ventricular oversensing | 17 | 0.6 |
Unsatisfactory threshold test | 12 | 0.4 |
Unsatisfactory rate response | 10 | 0.4 |
Atrial oversensing | 8 | 0.3 |
Atrial undersensing | 8 | 0.3 |
Ventricular undersensing | 0 | 0.0 |
Overall | 156 | 5.7 |
Reasons for reprogramming . | n . | % . |
---|---|---|
Unintentional or algorithm N/A | 49 | 1.8 |
Others—not AE/algorithm related | 42 | 1.5 |
Lead repositioning procedures | 7 | 0.3 |
Other surgical procedures | 3 | 0.1 |
Ventricular oversensing | 17 | 0.6 |
Unsatisfactory threshold test | 12 | 0.4 |
Unsatisfactory rate response | 10 | 0.4 |
Atrial oversensing | 8 | 0.3 |
Atrial undersensing | 8 | 0.3 |
Ventricular undersensing | 0 | 0.0 |
Overall | 156 | 5.7 |
Reprogramming reasons which might have a connection with the automatic algorithms performance are highlighted in bold and represent 2% of all algorithms.
Reasons for reprogramming . | n . | % . |
---|---|---|
Unintentional or algorithm N/A | 49 | 1.8 |
Others—not AE/algorithm related | 42 | 1.5 |
Lead repositioning procedures | 7 | 0.3 |
Other surgical procedures | 3 | 0.1 |
Ventricular oversensing | 17 | 0.6 |
Unsatisfactory threshold test | 12 | 0.4 |
Unsatisfactory rate response | 10 | 0.4 |
Atrial oversensing | 8 | 0.3 |
Atrial undersensing | 8 | 0.3 |
Ventricular undersensing | 0 | 0.0 |
Overall | 156 | 5.7 |
Reasons for reprogramming . | n . | % . |
---|---|---|
Unintentional or algorithm N/A | 49 | 1.8 |
Others—not AE/algorithm related | 42 | 1.5 |
Lead repositioning procedures | 7 | 0.3 |
Other surgical procedures | 3 | 0.1 |
Ventricular oversensing | 17 | 0.6 |
Unsatisfactory threshold test | 12 | 0.4 |
Unsatisfactory rate response | 10 | 0.4 |
Atrial oversensing | 8 | 0.3 |
Atrial undersensing | 8 | 0.3 |
Ventricular undersensing | 0 | 0.0 |
Overall | 156 | 5.7 |
Reprogramming reasons which might have a connection with the automatic algorithms performance are highlighted in bold and represent 2% of all algorithms.
Reprogrammings for which the investigator suspected a connection with the algorithm performance occurred in 2% of all active algorithms (55 of 2736), although this association was confirmed in only 13 cases (0.48%).
Atrial AutoSense was turned to Off/Manual in 16 cases (0.6%) due to suspected sensing issues (eight oversensing and eight undersensing), which were confirmed in only six cases (three undersensing and three oversensing). Ventricular AutoSense was turned to Off/Manual in 17 cases (0.6%) due to suspected oversensing, which was confirmed in only two cases. No ventricular undersensing was reported. A Kaplan–Meier log-rank test was performed for both atrial and ventricular algorithms to check if certain lead locations were associated with a higher incidence of sensing issues. There was no statistical significance that any atrial or ventricular lead location was associated with a higher incidence of sensing issues. Compared with other lead locations, even VDD systems showed comparable reprogramming rates of the atrial AutoSense algorithm, however, the number of reprogrammed algorithms is low for most lead locations, so that the registry is probably underpowered to detect a significant difference.
Automatic Capture algorithm was reprogrammed in 12 cases (0.4%) due to threshold tests that were judged unsatisfactory by the investigators, regardless of the fact that the algorithm was performing according to its specifications (e.g. threshold not detected due to RV threshold too high; evoked response signal too low; and noise) and that no harm was posed to the patients. Out of these 12 cases, there was a single confirmation of underperformance of the algorithm (0.04%).
AutoLifeStyleTM was reprogrammed in 10 cases (0.4%), either triggered by patient's symptoms (complaints for palpitations—nine cases) or by physician's judgment (heart rate was considered too low without patient's symptoms—one case). Association of palpitations with excessive paced rate was confirmed in four cases. We evaluated whether performing the initialization of the AutoLifeStyleTM algorithm (which was the case in 50% of these patients) was associated with a lower incidence of unsatisfactory rate response, but the results showed an even distribution of reprogrammings regardless of the initial calibration.
Of the remaining reprogrammed algorithms, few (10 of 2736 or 0.4%) occurred because of temporary programming settings employed during other electrophysiological/surgical procedures. Among these latter, 7 of 2736 (0.3%) were due to poor lead performance, with the reprogramming occurring prior to the lead repositioning procedure. The remaining reprogrammed algorithms (42 of 2736 or 0.9%) were turned Off/Manual due to other reasons, and were neither related to adverse events, nor to the algorithm performance.
Relationship to adverse events
A total of 111 of 156 reprogrammings (71%) were not related to any clinical events. Additionally, 23 algorithms were turned off in association with adverse events that were unrelated to the performance of the automatic algorithms: lead repositioning, non-cardiac surgical procedures, ablations, etc.
Serious adverse events related to automatic algorithms were infrequent. Out of the 55 reprogrammings that were potentially related to the automatic algorithms, only 10 were associated with serious adverse events: There were 27 reports of patient deaths (2.9%). There was no evidence of correlation between the patient deaths and the PM's algorithms or function.
four cases of palpitations leading to reprogramming of AutoLifeStyleTM because of pacing rate that was considered excessively high;
one case of palpitations possibly due to atrial undersensing leading to reprogramming of atrial AutoSense (atrial lead suspected for subclavian crush);
four episodes of dizziness leading to reprogramming of Automatic Capture (two cases), ventricular AutoSense (one case), or both algorithms (one case); however, only in a single case the Automatic Capture algorithm was underperforming but no harm was posed to the patient, thanks to the automatic reversion into a higher safe pacing output;
one episode of syncope leading to reprogramming of Automatic Capture, regardless that the physician declared that the episode was not linked to the automatic algorithm.
None of the algorithm reprogrammings and none of the clinical adverse events were attributable to device malfunctioning.
Discussion
This large, prospective, multicentre registry observed the real-life clinical utilization of automaticity algorithms in a large cohort of patients over a period of 12 months following device implant.
The capability of the implanted PM to deliver therapy as expected is crucial because PM patients rely on their implanted device to reduce symptoms, treat bradycardia, sustain hemodynamic function, and improve their quality of life. This must remain true, especially when certain device adjustments and optimizations are delegated to the device itself.
Four main algorithms were evaluated in this study: ventricular Automatic Capture, atrial and ventricular AutoSense, and AutoLifeStyleTM.
Automatic Capture
One key feature related to device automaticity is the capability of an implanted device to measure the stimulation threshold and consequently adjust the pacing output.
The reprogramming-free rate of ventricular Automatic Capture in the AUTOMATICITY registry was 95.9%, indicating an incidence of reprogramming for any reason of 4.1%.
Only a limited number of algorithms (0.4%) were reprogrammed due to potential issues with the algorithm. Moreover, in >90% of these cases, the issues were only suspected and not confirmed, and they were not associated with patient symptoms or with adverse events.
The issue of sudden variations in ventricular threshold is one of the challenges for this type of technology. Sudden unexpected threshold increases are reported to occur in 13–25% of patients15–18 even though improvements in lead manufacturing should ensure lower threshold variations over time. Pacing systems which feature algorithms with beat-to-beat automatic management of pacing capture, including backup high-voltage pacing, may manage elevated thresholds and sudden variations without endangering patient safety.
The results of this registry support the safe utilization of the Automatic Capture algorithm in a broad population, irrespective of the lead manufacturing technology and including patients with very old pacing leads.
The capability of the algorithm to react to changing patient conditions may further reduce the need for physician intervention and manual reprogramming of the automatic feature.
AutoSense
Atrial and ventricular amplitudes are changing parameters because the endocardial signals are influenced by several physiologic factors, characteristics of lead design, and lead maturation.19–21 The intracardiac atrial electrogram may decrease to less than half its previously measured value, especially during atrial fibrillation (AF) episodes. On the other hand, the consistent use of high sensitivity increases the risk of oversensing.
The reprogramming-free rate of AutoSense feature in the AUTOMATICITY registry was 94.3% for ventricular AutoSense and 93.3% for atrial AutoSense, indicating an incidence of reprogramming for any reasons of 5.7% for the ventricular algorithm and 6.7% for the atrial algorithm.
Only a limited number of reprogrammings (1.2% of all programmed algorithms) were attributable to potential sensing issues, equally distributed between atrial and ventricular (0.6% each). Moreover, in >75% of those rare cases the sensing issues were only suspected and they were not linked to evidence of any patient symptoms or adverse events.
Reliable detection of the intrinsic signals prevents competition between pacing and spontaneous rhythm, thereby minimizing the risk of arrhythmias. Proper sensing of intracardiac P waves enables a physiologic sequence of atrial and ventricular contractions.22 Finally, maintenance of appropriate atrio-ventricular synchrony can also result in a significantly lower incidence of atrial fibrillation.23
AutoLifeStyleTM
The AutoLifeStyleTM algorithm that was evaluated in the AUTOMATICITY registry provides continuous automatic optimization of both minute ventilation response and accelerometer response.
The reprogramming-free rate of the AutoLifeStyleTM feature in the AUTOMATICITY registry was 93.9%, indicating an incidence of reprogramming for any reasons of 6.1%.
Only a limited number of reprogrammings (0.4%) were potentially related to the performance of the algorithm, and only 0.15% were associated with clinical adverse events.
The low incidence of reprogramming suggests that this combination of accelerometer and dual-sensor minute ventilation ensures a physiological alternative that mimics the natural rhythm of the heart.
The specific design of the AutoLifeStyleTM optimization process,14 in combination with the fact that 21 of 22 reprogrammings (95.5%) occurred before the first 6 months of follow-up, suggest that, in the absence of an early need for reprogramming, the benefit of using this automatic algorithm may extend beyond the 12 months observed in this registry.
Distribution of reprogrammings between patients who performed a manual calibration of the algorithm and those who followed the algorithm's standard adjustment steps was identical.
Automatic follow-up
According to the recent American College of Cardiology/American Heart Association/Heart Rhythm Society guidelines24 patients with a PM should be followed every 3–12 months with more frequent follow-up visits as the battery approaches elective replacement time. The impact of recent PM and ICD advisories caused a further increase in follow-up visits25 in order to accurately monitor the integrity of the implantable systems. Growing awareness of expanded device indications, together with an aging trend in the Western European population, are causing the average patient to be older and to have more severe baseline cardiac diseases.
Remote monitoring of implantable cardiac devices introduces relevant changes in the follow-up of patients with PM.
For remote patient management and follow-up to become a successful standard of care, accurate, and reliable automatic measurements of basic device parameters are essential. Implantable cardiac devices which allow automatic monitoring of basic functions may allow significant facilitation of patient follow-up. The results of the AUTOMATICITY registry show that device measurements of key settings related to sensing, voltage threshold, and rate response could be automatically adjusted to optimal settings for the patient minimizing the need for physician's intervention.
Most commonly, inappropriate algorithms performance may simply indicate changes in clinical characteristics of the patient (i.e. AF) and/or changes in device or lead functioning in the early post-implant period.
Remote device monitoring allows following physicians the possibility to immediately be aware of changes in patient status and/or device functionality and to take appropriate actions when needed, thus representing a safe, effective, and cost-saving alternative to conventional in-clinic follow-up programs.
The distribution of reprogramming in the AUTOMATICITY registry, with most of the changes occurring within the first six months of follow-up, also encourages the shift towards remote device follow-up. In fact, pacing parameters appear to be optimized relatively soon after implantation and maintained unmodified thereafter. This same finding is confirmed in a report from the InSync ICD Italian Registry,26 where there was a marked reduction in number of interrogations requiring reprogramming between the first 6 months of follow-up and subsequent periods. Such distribution suggests that, in the absence of an early need for reprogramming, automatic algorithms may maintain their benefits beyond the 12-month timeline.
Study limitations
Only devices with automatic algorithms ON/AUTO could be included in this study. The study does not provide information about the current clinical practice of activating the algorithms at implant, but it provides useful insight on the management of patients who are treated by means of such algorithms during their follow-up.
The AUTOMATICITY registry has been conducted with pacing devices from a single manufacturer (Boston Scientific) in order to evaluate a subset of automatic algorithms as a ‘package’. A general assessment of clinical practice regarding the use and acceptance of automatic algorithms cannot be performed with the results of this registry. However, the results of the registry provide important feedback regarding a physician's level of confidence, understanding and satisfaction when they choose to rely on advanced automatic features for the management of their patients.
Conclusions
The results of the AUTOMATICITY registry suggest that automatic measurement of key parameters and automatic management of pacing settings, in response to changing patient needs, is feasible and safe. Algorithm performance over the long term proved highly reliable, whereas reprogrammings may sometimes result from physician mistrust in the event of symptoms, rather than prove algorithm failures.
These features allow a significant reduction in time spent on device inspection, without a trade-off for patient safety.
This would also set the background for remote PM follow-up, which represents a further improvement on cost reduction.
Conflict of interest: M.A. has consulting agreements with Bayer, Boehringer Ingelheim, Sanofi-aventis, and MSD, and received unrestricted institutional grants from Boston Scientific, Medtronic, and St Jude Medical; C.N. was conducting research sponsored by Biotronik, Medtronic, St Jude Medical, Vitatron, and Guidant; E.V. and C.D. are both employees of Boston Scientific.
Acknowledgements
The authors would like to thank Jan Mark Vorstenbosch (Boston Scientific, Nieuwegein, Netherlands) and Helen Reeve (Boston Scientific, Diegem, Belgium) for their assistance and technical review in designing and conducting the study.
The following investigators and institutions participated in the AUTOMATICITY registry. Investigators are listed after centres in alphabetical order: Amphia Ziekenhuis: Marco Alings—Amtssygehuset Gentofte (KAS Gentofte): Poul Erik Bloch-Thomsen—Bata Hospital: Cestmir Cihalik—Bergmannsheil Universitätsklinik: Thomas Lawo—Centre Hospitalier Général: Benaissa Agraou—CH Broussais: Philippe Deutsch—CH René Pleven: Patrick Bazin—CH Saint Philibert: Yves Guyomar—CHU Bourg en Bresse: Marc Bobillier—CHU Grenoble—Hopital Michallon: Pascal Defaye—CHU La Miletrie: Alain Amiel—Clinique Bizet: Arnaud Lazarus—Clinique Bouchard: Maxime Guenoun—Clinique St Hilaire: Pierre Le Franc—Fransiscus Ziekenhuis: Fanny L. Oei—Gelre Ziekenhuis: D. Nicastia—Gemeinschafstpraxis Hoenen/Franke: Stefan Hoenen—Gemini Ziekenhuis: A.E. de Porto—GZO Spital Wetzikon: Heiner Vontobel—Haga Ziekenhuis locatie Leyweg: Ramon Robles de Medina—Hanusch Krankenhaus Wien: Walter Kainz—Hopital Ambroise Paré: Philippe Brunner—Hospital Clinico Universitario Malaga: Javier Alzueta—Hospital Infante D. Pedro de Aveiro: Jose Antonio Santos—Hospital General de Castellón: Alejandro Bellver Navarro—Ijsselmeer Ziekenhuizen: C. Hie—IKEM—Institut klinické a experimentální medicíny: Josef Kautzner—Kantonsspital St Gallen: Peter Ammann-Kardiol— Gemeinschaftspraxis Dr Kaltofen: Gert Kaltofen—Kennemer Gasthuis: Raymond Tukkie—KH der Barmherzigen Schwestern Linz: Eduard Zeindlhofer—KH der Barmherzigen Schwestern Ried im Innkreis: Christopher Nimeth—KH der Barmherzigen Schwestern Wien: Martin Frömmel—Klinikum Coburg: Johannes Brachmann—Klinikum Nürnberg Süd: Konrad Göhl—Krankenhaus Hietzing: Susanne Trinks—Lange Land Ziekenhuis: Peter van der Meer—Landes Krankenhaus Bruck an der Mur: Gerald Zenker—Landes Krankenhaus Steyr: Franz Gebetsberger—Medigreif Bördekrankenhaus: Tilmann Unger—Medisch Centrum Alkmaar: Jacob H. Ruiter—Medisch Centrum Haaglanden-Westeinde: Hemanth Ramanna—Polyclinique Louis Pasteur: K.B. Hadj—Praxis Lang/Bischoff: Andreas Lang—Praxis Predel/Heinrich: Diethard Predel—Praxisgemeinschaft Kardiologie Drs. Straube, Schnabel: Andreas Schnabel—Public Hospital Elisabethinen: Martin Martinek—Queen Elizabeth hospital: Cheung-Wah Cheng—Queen Elizabeth the Queen Mother: Kevin Ward—Roskilde Sygehus: Gunnar Jensen—Royal Sussex County Hospital: Sean O'Nunain—Second aff. Hospital of Zhejiang: Shan Jiang—Slingeland Ziekenhuis: W.F. Terpstra—SMZ Süd-Kaiser Franz Josef Spital: Erich Budschedl—Spaarne Ziekenhuis Hoofddorp: D.J. van Doorn—St Nikolaus Hospital: Frédéric Marenne—Uniklinik, HTC: Thorsten Wahlers—Universitätklinikum, Klinik für Kardiol., Pneumo. und Angiol.: Tobias Zeus—Universitätsspital Basel: Stefan Osswald—Vejle Sygehus: Birger Engby—Viborg Sygehus: Per Dahl Christensen—Zhongshan Hospital, Fudan University: Ge Junbo—Ziekenhuis Gelderse Vallei: Maurits van der Veen.
Funding
This work was supported by Guidant Europe/Boston Scientific NV/SA, International Clinical Research Department, Diegem, Belgium.
References
Author notes
M.A. involved in study design, drafting, and revision of manuscript. E.V. involved in drafting of manuscript and its revisions.