-
PDF
- Split View
-
Views
-
Cite
Cite
Wouter B van Dijk, Ewoud Schuit, Replacing physical with virtual genetic tests: The importance of conscious methodological decisions, European Journal of Preventive Cardiology, Volume 27, Issue 15, 1 October 2020, Pages 1637–1638, https://doi.org/10.1177/2047487320904525
- Share Icon Share
The “virtual genetic tests” to identify patients with causative familial hypercholesterolemia (FH) mutations presented by Pina et al. are essentially three prediction models based on supervised machine learning classifiers, developed in one and externally validated in another independent cohort.1 By describing these as virtual genetic tests, Pina et al. show how prediction models could potentially be employed as alternatives for existing in vitro tests.
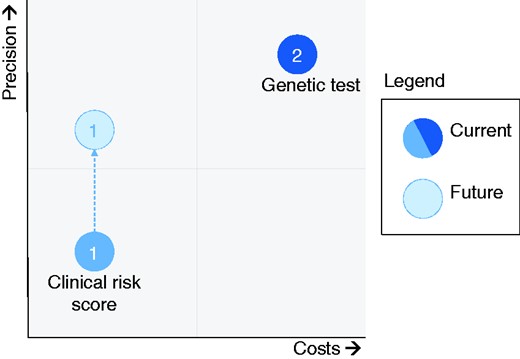
Potential of machine learning for improving precision of tests without raising costs.
Xenia (hospitality) towards technology advancements from other fields, in this case machine learning, can help to achieve this inexpensively. By using machine learning, Pina et al. show how to leverage machine learning to digitise these early stages of disease diagnostics.
Moulding (machine learning) prediction models to serve as full virtual genetic tests, however, requires a high specificity and/or negative predictive value (NPV) to lower the number of needless referrals. The specificity and NPV as presented in Table 1 by Pina et al. still seem to leave room for improvement in that regard.
Aptly, the authors show how their prediction models, comprising a classification tree (CT), gradient boosting machine (GBM) and neural network (NN), outperform the current DLS in the detection of patients with these FH mutations in terms of discriminative performance (respective Area Under the Receiver Operating Curves (AUROC) at external validation: 0.70 (CT), 0.78 (GBM), 0.76 (NN), 0.64 (DLS)). Similar to more traditional models, machine learning models need to confine to certain modelling and reporting standards to be able to assess their true potential.
Next, we would like to highlight a methodological issue of the models developed by Pina et al. and suggest reporting improvements that would help to better assess the potential of the models.
First, machine learning models have been found to need a number of events per variable (EPV) of 50 to 200 for reliable predictor–outcome association estimation, compared to an EPV of 10 to 20 with traditional modelling techniques.5 When the EPV is lower than this identified standard, models will be overfitted to their development data and are likely to substantially underperform when applied to new patients. With a total number of 111 events in the total development cohort, the models presented by Pina et al. are most likely overfitted, and would qualify as high risk of bias when evaluated by common risk of bias tools – for example, the Prediction model Risk Of Bias ASsessment Tool (PROBAST).6 On a similar note, the number of events in the external validation cohort, or, here, actually the number of non-events (n = 57), is lower than the recommended 100 events (or non-events; whichever group of patients is smaller) for reliable model assessment at external validation.7 So, the external validation of the current study would be also assessed as high risk of bias according to PROBAST.6
Second, according to the Transparent Reporting of a multivariable prediction model for Individual Prognosis Or Diagnosis (TRIPOD), model performance should at least be presented in terms of discrimination (e.g. AUROC) and calibration (i.e. agreement between predicted risk and observed probability) to be able to assess a model’s value.8 Pina et al. did present both in the form of the AUROC (discrimination) and Hosmer–Lemeshow test (calibration). According to the authors, all developed models showed miscalibration at external validation based on a Hosmer–Lemeshow test at a 0.05 p-value cut-off. As this test in itself is known to be sample-size dependent (p-values increase with decreasing sample sizes; Pina et al. used a relatively low sample size) and lacks information about the extent and direction of miscalibration, it seems premature to draw the conclusion that the models truly outperform the DLS based on the AUROC only. The low EPV used in the models’ development and external validation stage and the miscalibration at external validation seem to indicate that further research, ideally with more data, is needed before these models can be recommended to be used for clinical decision-making.
Machine learning models developed on larger amounts of data have the potential, both in traditional healthcare systems and in upcoming learning healthcare systems, to create more accurate and efficient diagnostic and prognostic tests. Yet, these models should be held to the same standards as traditional prediction models (or higher when it comes to EPV) in terms of methodology and reporting to allow these new techniques to bring future improvements.
Declaration of conflicting interests
The author(s) declared no potential conflicts of interest with respect to the research, authorship, and/or publication of this article.
Funding
The author(s) disclosed receipt of the following financial support for the research, authorship, and/or publication of this article: this work was supported by the Netherlands Organisation for Health Research and Development (ZonMW) (grant number 91217027).
Comments