-
PDF
- Split View
-
Views
-
Cite
Cite
M Gurnani, A Sau, K Patlatzoglou, J Barker, L Pastika, N S Peters, D B Kramer, J W Waks, F S Ng, Utilizing unsupervised machine learning to uncover novel phenogroups within the broad QRS complex, European Heart Journal, Volume 45, Issue Supplement_1, October 2024, ehae666.333, https://doi.org/10.1093/eurheartj/ehae666.333
- Share Icon Share
Abstract
Conduction disease can manifest as a broad QRS complex on the ECG. Broad QRS is conventionally categorised as left bundle branch block (LBBB) and right bundle branch block (RBBB) or non-specific intraventricular conduction delay (IVCD), based on QRS morphology. These subgroups were coined over a century ago and have been relevant for heart failure with reduced ejection fraction and cardiac resynchronization therapy (CRT). However, there may be more precise phenogroups underlying broad QRS complexes.
Using unsupervised machine learning to define novel broad QRS phenogroups and characterise them using clinical variables and disease outcomes.
We extracted 51 relevant features using a Variational Autoencoder from 47,456 median-beat ECGs with broad QRS intervals (QRS ≥ 130ms) from a secondary care cohort. These were input into Discriminative Dimensionality Reduction via Learning a Tree (DDRTree), a clustering method that models the continuum of heterogeneity by projecting the underlying distribution as a tree structure in a low-dimensional space, while assigning distinct branch/cluster labels.
Six distinct phenogroups were identified within broad QRS morphologies (Figure-1). Two branches were RBBB dominant (Branch 1: more males, high-comorbidity; Branch 6: low-comorbidity/healthy-RBBB). Two branches were LBBB dominant (Branch 4: mixed IVCD with CHB burden; Branch 5: more females, CRT responders). Two branches were IVCD/mixed-phenotype (Branch 2: IVCD-dominant, high risk of future cardiovascular events; Branch 3: average/core branch). Within LBBB, Branch 4 and 5 showed similar trends with improving echocardiography profiles and CRT response when adjusted for covariates, each compared against the core branch. Branch 5 was distinct due its high risk of future HF (HR 1.19 [1.07-1.33] p < 0.01), cardiovascular death (HR 1.35 [1.20-1.50] p < 0.0001), all-cause mortality (HR 1.08 [1.01-1.16] p < 0.05) and CHB (HR 1.35 [1.16-1.56] p < 0.0001) (Figure-2). Conversely within RBBB, Branch 1 and 6 showed stark differences across various traits. Branch 1 captured a high-risk profile associated to higher risk of cardiovascular death (HR 1.37 [1.24-1.52] p < 0.0001), all-cause mortality (HR 1.20 [1.12-1.29] p < 0.0001) and CHB (HR 1.15 [1.01-1.32] p < 0.05) (Figure-2), while Branch 6 retained a low-risk phenotype. The tree dimensions also revealed relevant trends in cardiac anatomy and valvular dysfunction; the top-left region in Figure-1 associated with higher left ventricular ejection fraction and lower left ventricular end-diastolic dimension, lower left ventricular end-systolic dimension and a greater CRT response.
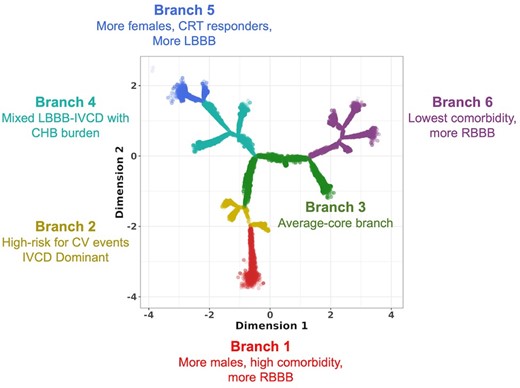
Figure-1
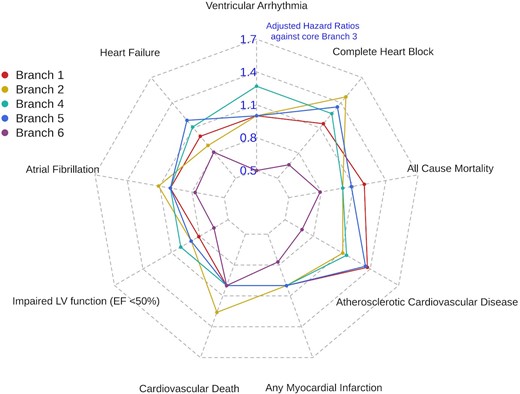
Figure-2
Author notes
Funding Acknowledgements: Type of funding sources: Public grant(s) – National budget only. Main funding source(s): British Heart Foundation
- phenotype
- left ventricular ejection fraction
- echocardiography
- qrs complex
- right bundle-branch block
- heart failure
- left ventricle
- heterogeneity
- comorbidity
- diastole
- systole
- trees (plant)
- incomplete bundle branch block
- heart
- mortality
- left bundle-branch block
- cardiac resynchronization therapy
- cardiovascular event
- cardiovascular death
- secondary care
- precision
- heart failure with reduced ejection fraction
- unsupervised machine learning