-
PDF
- Split View
-
Views
-
Cite
Cite
Abhirup Banerjee, Paul Leeson, Scoring systems developed by machine learning: intelligent but simple to use?, European Heart Journal, Volume 45, Issue 11, 14 March 2024, Pages 937–939, https://doi.org/10.1093/eurheartj/ehae053
- Share Icon Share
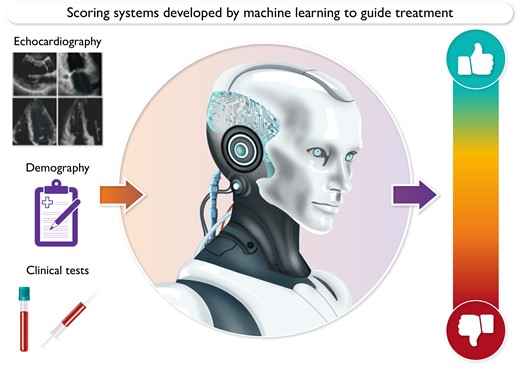
Interpretation of multiple pieces of information including imaging, blood tests and demographic information (left panel) is required for clinical decision making. Risk scores are an existing, familiar way to summarise this information and guide clinical decision making (right panel). A new generation of more accurate risk scores could be developed by machine learning (central panel) to summarise complex clinical information in scores that allow easy interpretation.
This editorial refers to ‘Artificial intelligence-derived risk score for mortality in secondary mitral regurgitation treated by transcatheter edge-to-edge repair: the EuroSMR risk score’, by J. Hausleiter et al., https://doi.org/10.1093/eurheartj/ehad871.
Mitral valve transcatheter edge-to-edge repair (M-TEER) is a minimally invasive procedure designed to correct mitral regurgitation due to problems with valve leaflet coaptation. M-TEER, performed via catheter, offers a less invasive alternative to open-heart surgery, particularly beneficial for patients unsuitable for traditional surgical interventions. However, recent randomized trials indicate a high mid-term mortality rate following M-TEER, and there is interest in developing better, objective characterization and stratification of patients who may benefit from M-TEER.
Published in the current issue of the European Heart Journal, Hausleiter et al.1 present a new risk score, the EuroSMR score, which is deigned to predict 1-year outcomes in patients with secondary mitral regurgitation undergoing M-TEER. A major strength of the work is that they have used real-world patient datasets from multiple centres contributing to registries. As a result, the score could be developed based on information from 3449 patients treated with M-TEER and validated over a further 428 patients. The derivation cohort included an impressive number of 12 cardiac centres from the initial EuroSMR registry across Europe, the Italian Society of Interventional Cardiology (GIse) registry of transcatheter treatment of mitral valve regurgitation (GIOTTO) registry, the Spanish MitraScore registry, and the German MitraPro registry. Furthermore, in order to avoid data contamination and make the approach generalizable, the authors used patients from two additional study sites, which recently joined the initial EuroSMR registry, exclusively as the validation cohort.
Based on 18 clinical, echocardiographic, laboratory, and medication parameters, it was possible to improve discrimination of surviving and non-surviving patients with a hazard ratio (HR) of 4.5 and a 95% confidence interval (CI) of 2.8–7.1 in the independent validation cohort. Potentially most clinically interesting, the authors used the EuroSMR score to identify a secondary mitral regurgitation patient subgroup at extreme risk for mortality with a HR of 6.5 (95% CI 3.0–14.0), for which the procedure may be considered futile. The proposed EuroSMR score demonstrates significant improvement over its previous counterparts of EuroScore II,2 MitraScore,3 and Cardiovascular Outcomes Assessment of the MitraClip Percutaneous Therapy for Heart Failure Patients with Functional Mitral Regurgitation (COAPT) score.4 Notably, the EuroScore II was developed for in-hospital mortality prediction after a range of cardiac surgical procedures and not just mitral interventions. Therefore the comparison may not be entirely fair. The MitraScore is specific for patients with mitral valve problems but does not differentiate between primary and secondary mitral regurgitation, and the COAPT score was developed from seven features over only 614 highly selected secondary mitral regurgitation patients with only 302 patients treated by M-TEER.
The real novelty of this work, over and above other risk scores, comes from the fact that the EuroSMR score relies on application of a machine learning model, the extreme gradient boosting (XGBoost) algorithm, rather than using conventional statistical approaches. The work implies that use of XGBoost may provide significant improvements over other statistical approaches more commonly used to develop risk models, such as logistic regression. However, in this particular application, the limited number of input feature variables (28) may not be fully utilizing the effectiveness of the XGBoost. In fact, over a limited features set, traditional methods can often offer comparable performance, with added explainability in the models. The current study includes a large and heterogenous population from four European registries and therefore it would be interesting to know whether the performance improvements in EuroSMR primarily relate to the size of the dataset or the application of the XGBoost algorithm.
Another drawback of the use of XGBoost is that it could not perform the variable selection or extraction on its own. The model initially included all 28 variables, which were refined by recursive feature elimination, i.e. from the initially trained XGBoost algorithm, then the variables were ranked according to their feature importance for prediction of 1-year all-cause mortality using SHapley Additive exPlanations (SHAP) values. The least important features were discarded and the model was retrained, thus iteratively optimizing until a specified number of input variables (18) remained. The regularized logistic regression or random forest approach has the ability to measure the importance of the variables during training and discarding them from the model, which makes their comparisons with XGBoost more important in this study.
We are living in an era where advances in artificial intelligence (AI) technologies have made it possible to process and learn from large amounts of heterogeneous, multi-modal data very efficiently. As a result, complex characterization of disease and outcomes has become possible. However, at the same time, keeping information ‘simple’ remains essential in many clinical scenarios to allow robust and fast decision-making (see Graphical Abstract). This is demonstrated by the enthusiasm in the current study for rapid clinical translation of the simple EuroSMR score, which is already being provided on a website. Risk scores, or binary outcomes, have always been popular as a means to provide simple summaries of available information in clinical practice. These scores do not necessarily need to be limited to risk prediction but can also be developed to provide scoring of, for example, a disease severity. With this in mind, it has recently5 been demonstrated that disease characteristics such as complex patterns of cardiac remodelling assessed by echocardiography in hypertensive patients can be effectively summarized in a single normalized score between 0 and 1. Using a similar semi-supervised contrastive machine learning method, Alkhodari et al.6 expanded this work to demonstrate feasibility of developing a simple score from large datasets of tens of thousands of participants with multi-organ and multi-modality clinical measurements. Furthermore the data we use do not have to be traditional clinical parameters. In another recent study, Beetz et al.7 developed a geometric deep learning approach for explainable myocardial infarction prediction scores based on 3D cardiac anatomical shape.
Machine learning has proved itself a powerful approach to identify and learn patterns in large clinical datasets. However, examples of real-world clinical application remain limited. The reasons for this are multi-factorial and include behavioural factors such as trust in the ‘black box’ of machine learning, as well as lack of real-world validity and testing of some developed algorithms. Another factor is that outcomes developed by AI can often be complex or need to be caveated by levels of uncertainty within the machine learning itself. Scoring systems have always provided a familiar and simple way of bringing together complex information, which we already expect to have some degree of uncertainty. Most scores in clinical use have been developed based on traditional statistical approaches, but it is likely that these scores will now start to be replaced, or updated, with AI-derived scores. Whether AI-derived scores start to replace other ways in which we assess disease remains to be seen, but the ability to describe complex disease patterns with simple explanations has always been a powerful means to transfer and interpret clinical information.
Declarations
Disclosure of Interest
P.L. is supported by the Oxford NIHR Biomedical Research Centre and has research funding related to AI-derived scores from the UKRI MRC (MR/W003686/1). A.B. is a Royal Society University Research Fellow and is supported by the Royal Society grant no. URF\R1\221314. P.L. is a stockholder and founder of Ultromics: a medical AI company and inventor on a patent (UK 2113322.8) related to AI-derived scores.
Funding
P.L. is supported by the Oxford NIHR Biomedical Research Centre. A.B. is a Royal Society University Research Fellow and is supported by the Royal Society grant no. URF\R1\221314.
References
Author notes
The opinions expressed in this article are not necessarily those of the Editors of the European Heart Journal or of the European Society of Cardiology.