-
PDF
- Split View
-
Views
-
Cite
Cite
R Cherukupalli, A Achanta, A Cherukupalli, S Potukuchi, Machine learning based diagnosis of heart failure with preserved ejection fraction among South Asian patients, European Heart Journal, Volume 43, Issue Supplement_1, February 2022, ehab849.061, https://doi.org/10.1093/eurheartj/ehab849.061
- Share Icon Share
Abstract
Type of funding sources: None.
Diagnosis of Heart Failure with preserved Ejection Fraction (HFpEF) remains challenging even for an astute clinician with the current diagnostic tests, algorithms and scoring systems. The use of Machine Learning (ML) in cardiovascular research has expanded exponentially in recent years.
In this study we studied the predictive accuracy of a machine-learning model for the diagnosis of HFpEF in South Asian patients.
A single centre retrospective data of 1068 admitted patients with a clinical diagnosis of HF were obtained. From this data outliers were removed based on box-and-whisker plot study. This data was then segregated into three: without HF, HF with reduced ejection fraction and HFpEF. Finally, we arrived at 530 patients’ data with left ventricular ejection fraction (LVEF) values of >45%. This data set was randomly divided into two parts: 80% as Training dataset and 20% as Test dataset. Two ML techniques such as Logistic Regression and Neural Networks were chosen to build the prediction models. Orange Data Mining Software version 3.28 was used to build these models. The overall performance of the ML methods has been reported using Precision, Recall and F1 scores of precision and recall; measure that combines precision and recall.
The table summarizes precision, recall and F1 score of both models. The predicted results from the ML models are compared with HFA PEFF score.
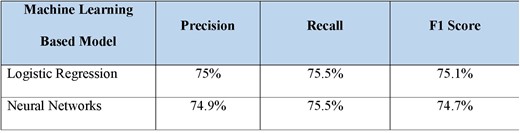
Abstract Figure.