-
PDF
- Split View
-
Views
-
Cite
Cite
Yang Chen, Steve Harris, Yvonne Rogers, Tariq Ahmad, Folkert W. Asselbergs, Nudging within learning health systems: next generation decision support to improve cardiovascular care, European Heart Journal, Volume 43, Issue 13, 1 April 2022, Pages 1296–1306, https://doi.org/10.1093/eurheartj/ehac030
- Share Icon Share
Abstract
The increasing volume and richness of healthcare data collected during routine clinical practice have not yet translated into significant numbers of actionable insights that have systematically improved patient outcomes. An evidence-practice gap continues to exist in healthcare. We contest that this gap can be reduced by assessing the use of nudge theory as part of clinical decision support systems (CDSS). Deploying nudges to modify clinician behaviour and improve adherence to guideline-directed therapy represents an underused tool in bridging the evidence-practice gap. In conjunction with electronic health records (EHRs) and newer devices including artificial intelligence algorithms that are increasingly integrated within learning health systems, nudges such as CDSS alerts should be iteratively tested for all stakeholders involved in health decision-making: clinicians, researchers, and patients alike. Not only could they improve the implementation of known evidence, but the true value of nudging could lie in areas where traditional randomized controlled trials are lacking, and where clinical equipoise and variation dominate. The opportunity to test CDSS nudge alerts and their ability to standardize behaviour in the face of uncertainty may generate novel insights and improve patient outcomes in areas of clinical practice currently without a robust evidence base.
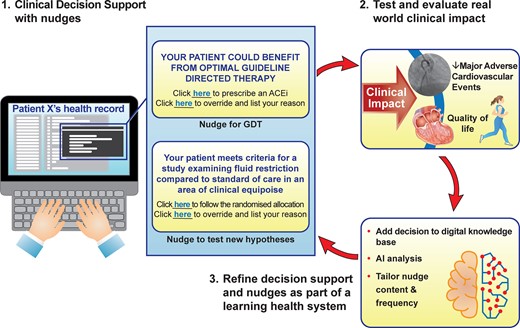
Schematic of a future learning health system with nudge, clinical decision support systems (CDSS), and artificial intelligence-powered analyses forming core parts of the learning cycle. Nudges within the CDSS can be used to either promote better adherence of guideline-directed therapy or test new hypotheses whenever there is clinical equipoise, identified through variation in clinical practice owing to the lack of robust evidence in that context. Although a simple A vs. B randomized controlled trial choice has been presented (fluid restriction vs. standard of care), multiple arms and an adaptive design could be built. Clinical outcomes could include patient-reported outcome measures. The presentation of nudges can be adjusted as new clinical insights are generated either within the local system or externally through data linkage. ACEi, angiotensin-converting enzyme inhibitor; GDT, guideline-directed therapy.
Introduction
Decision-making in healthcare is under increasing scrutiny. As we enter an age of ubiquitous electronic health records (EHRs), clinical decision support systems (CDSS), and large, linked data sets with the potential to accommodate artificial intelligence (AI) algorithms, the need to better understand individual and collective decision-making has reached a critical point. In cardiovascular medicine, we anchor our decision-making to clinical guidelines. However, only a minority of recommendations within guidelines is based on Level A evidence and there remains variation in clinical practice.1 The increasing digitalization of healthcare provides the opportunity to systematically test and evaluate this variation across healthcare professionals within a learning healthcare framework.
In this review, we highlight the role of nudge theory and how better testing and evaluation of nudges as part of a learning health system (LHS)2 can improve decision-making and patient outcomes. Thaler and Sunstein3 defined nudge in 2008 as ‘choice architecture that alters people’s behaviour in a predictable way without forbidding any options or significantly changing their economic incentives’. While prominent examples include a traffic light system for the front of pack nutritional labelling (with healthier food choices in green and unhealthier food choices in red) or the addition of a ‘fly-shaped sticker’ in male urinals at Schiphol airport to reduce cleaning costs,3 nudges in healthcare are less notable—an example is given in Figure 1.
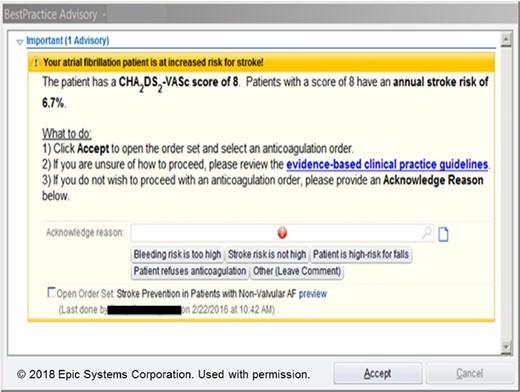
Nudge example in cardiovascular medicine. One recent example is taken from the AF-ALERT trial,4 which used a pop-up clinical decision support systems alert to nudge clinician behaviour whenever trigger conditions were met.
Despite its popularization over a decade ago, the testing and implementation of nudges within cardiovascular research and practice have been scarce compared with traditional topics such as basic sciences, translational medicine, and multicentre trials. Most of the literature has developed alongside the mass digitalization of healthcare.5 We, therefore, focus primarily on nudges as CDSS alerts and associated digital tools such as EHRs as a natural platform for their delivery. A sketch of the relationships between nudge, CDSS, and alerts is given in Figure 2.
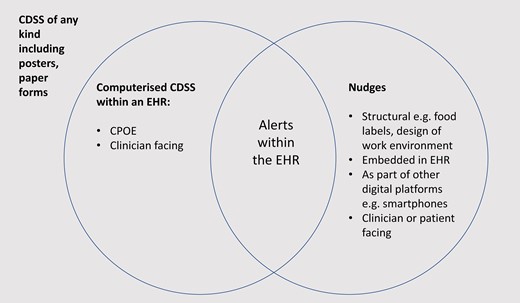
Clinical decision support systems have a broad definition which could include a cumbersome number of entities—for instance, a poster on a wall could represent a form of clinical decision support. Most published literature of clinical decision support system in medical journals refers to either explicitly or implicitly a computerized version embedded within electronic health records, ranging in complexity from a simple reminder alert or highlight of a laboratory result, to default medication listing and dosing.6 CDSS, clinical decision support system; CPOE, computerized provider order entry; EHR, electronic health record.
We draw distinction between the use of CDSS alone and applying nudges as part of their design. For example, while CDSS alerts are a form of nudging, simple default states within EHRs, e.g. an alphabetized list of medications as part of an electronic prescription order, are not explicitly ‘nudge interventions’ unless the design of a default state is deliberate. However, even incidental designs can have consequences—in this case, with effects on prescribing patterns.7 A broad classification of nudge types are included as part of the Supplemental material online, though any aspect of an EHR-based platform can impact on decision-making. The careful and explicit study of their design, including nudges, and their influence on clinical behaviour and outcomes has been largely missing.
Nudging in areas with the known and unknown evidence base
Our central argument is that the routine use of nudges in healthcare can improve decision-making and outcomes through two distinct routes: (i) by reducing the evidence-practice gap through improved adherence to guideline-directed therapy (GDT) and (ii) by generating novel insights.
While ‘bench to bedside’8 has improved patient outcomes through the translation of basic science discovery to clinical trials, this paradigm alone is not enough. By extending the concept ‘from bench to bedside, to patient and population’,9 an additional evidence-practice gap emerges, defined as the suboptimal choices we make, as providers, policymakers, and also as patients. This can affect large groups of individuals depending on the context. For example, in a recent multinational analysis of heart failure prescriptions, fewer than one in five patients achieved target doses of evidence-based medications.10 Nudges theory can play a central role in bridging this gap.11
Nudges can also lead to the generation of new clinical knowledge insights, when deployed in settings of clinical equipoise. Here, the ability of nudges to shape behaviour towards a more uniform course of action may uncover meaningful effects on patient outcomes in areas that lack a robust evidence base and have large variations in current practice. In particular, the testing of clinician-facing CDSS nudge alerts as interventions as part of randomized controlled trials will allow the true potential of nudge impact to be realized.
A summary of key considerations in these two scenarios is presented in Table 1, alongside a schematic representation in the Graphical Abstract.
Characteristics of nudges for guideline adherence and for knowledge generation
. | Target audience . | Example . | Aim of nudge . | Pre-requisites . | Adherence . |
---|---|---|---|---|---|
Nudges where evidence is known | Clinicians, patients |
| Deterministic—to reach the recognized standard/best practice, with recognized action to take | Need prior knowledge and data quality to apply | Desirable to be adherent as it moves behaviour (either clinician or patient) towards beneficial outcomes for patients |
Nudges where evidence is not known | Clinicians, researchers |
| Stochastic—to generate evidence through a randomized controlled trial, either with recognized action or with open-ended actions | Need prior equipoise to apply | Non-compliance is an essential component—supports safety of intervention as clinicians can exercise their own judgements, whether to adhere to the randomized allocation, and what actions are required to be taken [either a nudge with closed suggestion (fluid restrict) or with open-ended suggestion (REVeAL-HF)] |
. | Target audience . | Example . | Aim of nudge . | Pre-requisites . | Adherence . |
---|---|---|---|---|---|
Nudges where evidence is known | Clinicians, patients |
| Deterministic—to reach the recognized standard/best practice, with recognized action to take | Need prior knowledge and data quality to apply | Desirable to be adherent as it moves behaviour (either clinician or patient) towards beneficial outcomes for patients |
Nudges where evidence is not known | Clinicians, researchers |
| Stochastic—to generate evidence through a randomized controlled trial, either with recognized action or with open-ended actions | Need prior equipoise to apply | Non-compliance is an essential component—supports safety of intervention as clinicians can exercise their own judgements, whether to adhere to the randomized allocation, and what actions are required to be taken [either a nudge with closed suggestion (fluid restrict) or with open-ended suggestion (REVeAL-HF)] |
Deploying nudges where evidence is known includes in circumstances when they aid guideline-directed therapy. This can be viewed as quality improvement and may form part of multi-faceted interventions including education of staff, audit, and feedback. Deploying nudges where evidence is not known will generate knowledge and requires clinical equipoise and randomization in order to generate novel insights which may be generalizable to multiple contexts.
Characteristics of nudges for guideline adherence and for knowledge generation
. | Target audience . | Example . | Aim of nudge . | Pre-requisites . | Adherence . |
---|---|---|---|---|---|
Nudges where evidence is known | Clinicians, patients |
| Deterministic—to reach the recognized standard/best practice, with recognized action to take | Need prior knowledge and data quality to apply | Desirable to be adherent as it moves behaviour (either clinician or patient) towards beneficial outcomes for patients |
Nudges where evidence is not known | Clinicians, researchers |
| Stochastic—to generate evidence through a randomized controlled trial, either with recognized action or with open-ended actions | Need prior equipoise to apply | Non-compliance is an essential component—supports safety of intervention as clinicians can exercise their own judgements, whether to adhere to the randomized allocation, and what actions are required to be taken [either a nudge with closed suggestion (fluid restrict) or with open-ended suggestion (REVeAL-HF)] |
. | Target audience . | Example . | Aim of nudge . | Pre-requisites . | Adherence . |
---|---|---|---|---|---|
Nudges where evidence is known | Clinicians, patients |
| Deterministic—to reach the recognized standard/best practice, with recognized action to take | Need prior knowledge and data quality to apply | Desirable to be adherent as it moves behaviour (either clinician or patient) towards beneficial outcomes for patients |
Nudges where evidence is not known | Clinicians, researchers |
| Stochastic—to generate evidence through a randomized controlled trial, either with recognized action or with open-ended actions | Need prior equipoise to apply | Non-compliance is an essential component—supports safety of intervention as clinicians can exercise their own judgements, whether to adhere to the randomized allocation, and what actions are required to be taken [either a nudge with closed suggestion (fluid restrict) or with open-ended suggestion (REVeAL-HF)] |
Deploying nudges where evidence is known includes in circumstances when they aid guideline-directed therapy. This can be viewed as quality improvement and may form part of multi-faceted interventions including education of staff, audit, and feedback. Deploying nudges where evidence is not known will generate knowledge and requires clinical equipoise and randomization in order to generate novel insights which may be generalizable to multiple contexts.
The existing evidence for CDSS impact was the subject of a recent meta-analysis—of more than 100 heterogeneous studies analysed, there was a significant 6% improvement in the proportion of patients receiving desired care.12 However, this work only examined CDSS use for improving guideline and process adherence. There was a lack of focus given to descriptions of the human–computer interface, including elements such as degree of clinician involvement and acceptability among different user groups. A future LHS will be one which can routinely deploy nudges within CDSS and better understand why adherence to their recommendations may vary according to clinician and context. Co-design with frontline clinicians as well as clinical leaders will be crucial in order to ensure credibility in their testing and use. In doing so, synchronous progression of our understanding of the ‘user stream of knowledge’ and learning from responses to CDSS nudges,6 can occur alongside increases in knowledge of disease and use of treatments through EHR-based phenotyping and variations in clinical practice.13
As clinicians increasingly spend their working lives directly interacting with EHRs and CDSS as part of patient care,14 studies that examine how to best deploy CDSS alerts and which nudges may modify clinicians’ behaviour in the most effective manner, will be of increasing value.
As of November 2021, there are 41 systematic reviews registered on PROSPERO which include ‘nudge’,15 and only 21 papers returned from a search of ‘nudge’ and ‘cardiology’ on PubMed.16 Of the above results, only one review was directly related to Cardiology and few primary research studies used ‘nudge’ as a term. Current research practice perhaps reflects ‘nudge hesitancy’ within the cardiovascular disease field as well as inconsistency in its definition and reported use. For example, work may not explicitly be labelled by the term nudge and the term alert is often used interchangeably.
This contrasts with their potential efficacy on an aggregate level—in a study of Cochrane systematic reviews, 42 trials of nudge strategies for clinician implementation of guidelines determined an effect in 86% of studies in the hypothesized direction, with more than half of those being statistically significant.17 However, a narrower 2021 scoping review which focused on interventions explicitly identified as nudges found 22 studies of mixed benefit18 with more detailed descriptions of the factors underpinning study designs that had a significant effect on their overall result, including prominence of choices; predisposition to social norms; and clinician workflow impacts. Thus within the limited evidence base, nudge designs have been evaluated using familiar tools such as randomized controlled trials (RCTs), though it is the nudge use for guideline adherence that predominates. Important contextual factors are inconsistently considered during their implementation.
Nudges as blockbuster intervention
Evidence-based medicine through international multicentre randomized clinical trials have guided the practice of cardiovascular medicine and generated blockbuster results, ultimately embodied and communicated in the form of practice-changing guidelines.19–21 However, the lack of attention paid to evaluating the decision-making of clinicians and patients represents a significant asymmetry. As a result, there is an untapped opportunity for our community to make step change improvements in cardiovascular care, through greater testing of nudge interventions and their effect on clinical decision-making. The magnitude of the potential benefits can be profound, as seen when comparing head-to-head the top-end effect size of a successful nudge, with the most prominent recent blockbusters in medicine (Table 2).4,22–24
Author . | Intervention . | Effect size compared with standard of care at time . |
---|---|---|
McMurray et al.22 | Sacubitril/valsartan novel drug in patients with heart failure | 20% relative improvement |
Keeley et al.23 | Primary angioplasty in acute MI | 22% relative improvement |
RECOVERY Collaborative Group24 | Dexamethasone in severe COVID-19 pneumonia | 18–36% relative improvement depending on illness severity |
Piazza et al.4 | CDSS alert to increase anticoagulation in high-risk hospitalized AF patients | 55% relative improvementa |
Author . | Intervention . | Effect size compared with standard of care at time . |
---|---|---|
McMurray et al.22 | Sacubitril/valsartan novel drug in patients with heart failure | 20% relative improvement |
Keeley et al.23 | Primary angioplasty in acute MI | 22% relative improvement |
RECOVERY Collaborative Group24 | Dexamethasone in severe COVID-19 pneumonia | 18–36% relative improvement depending on illness severity |
Piazza et al.4 | CDSS alert to increase anticoagulation in high-risk hospitalized AF patients | 55% relative improvementa |
AF, atrial fibrillation; CDSS, clinical decision support system; COVID-9, coronavirus disease 2019; MI, myocardial infarction.
Event rates and outcomes measured are omitted. These papers have been discussed at length in the literature and the focus of this comparison is to highlight the possibilities of nudges being equal in relative effect to any blockbuster, depending on the research context.
Author . | Intervention . | Effect size compared with standard of care at time . |
---|---|---|
McMurray et al.22 | Sacubitril/valsartan novel drug in patients with heart failure | 20% relative improvement |
Keeley et al.23 | Primary angioplasty in acute MI | 22% relative improvement |
RECOVERY Collaborative Group24 | Dexamethasone in severe COVID-19 pneumonia | 18–36% relative improvement depending on illness severity |
Piazza et al.4 | CDSS alert to increase anticoagulation in high-risk hospitalized AF patients | 55% relative improvementa |
Author . | Intervention . | Effect size compared with standard of care at time . |
---|---|---|
McMurray et al.22 | Sacubitril/valsartan novel drug in patients with heart failure | 20% relative improvement |
Keeley et al.23 | Primary angioplasty in acute MI | 22% relative improvement |
RECOVERY Collaborative Group24 | Dexamethasone in severe COVID-19 pneumonia | 18–36% relative improvement depending on illness severity |
Piazza et al.4 | CDSS alert to increase anticoagulation in high-risk hospitalized AF patients | 55% relative improvementa |
AF, atrial fibrillation; CDSS, clinical decision support system; COVID-9, coronavirus disease 2019; MI, myocardial infarction.
Event rates and outcomes measured are omitted. These papers have been discussed at length in the literature and the focus of this comparison is to highlight the possibilities of nudges being equal in relative effect to any blockbuster, depending on the research context.
A key caveat to the above comparison is that most nudge studies report process measures instead of hard clinical outcomes, although the reference for the AF-ALERT study4 included in Table 2 is deliberate. While currently an outlier, it represents a precedent for future nudge studies to aim for impact on clinical outcomes. A missing element from the table is that of financial costs and cost-effectiveness—an area where nudges deployed within EHRs will out-compete traditional RCTs many times over.2
Wielding the right tools to evaluate nudge
In terms of widespread testing, previous economic and technical barriers to studying and deploying nudge on a large scale are now surmountable. Over 90% of US hospitals had computerized health records by the mid-2010s25 and globally, lower and middle-income countries are continuing their progress towards digitalization.26 The increasing availability and sophistry of EHRs mean that it is now possible for many more healthcare systems to not only accurately codify clinical details, but also elements of human factors that have traditionally been unattended to, such as quantifying the confidence of decision-making in real time, and how this may be linked to the selection of a particular diagnosis or treatment.27 The implications of subsequently using nudges to steer clinicians towards certain decisions in the face of uncertainty and to modify them in real time as evidence accumulates of clinician preferences and clinical outcomes represent novelties that should form the crux of the future LHS.
Thus, while human factors research in medicine has primarily focused on safety in the form of checklists28 or shift patterns,29 the ability to test other hypotheses as part of CDSS nudges embedded within the EHR can now be realized. The challenge will be one of prioritization, and in which clinical settings CDSS nudges should be tested first. Just as with the checklist literature, accepting that effects of local context can predominate over replicability of action would enable us to move beyond the moot ‘nudges do/don’t work’ commentaries seen within the checklist literature.30
Examples of nudge in the cardiovascular field
There are several nudge trials within cardiovascular medicine, though they remain rare. A scoping search for relevant RCTs was conducted (see Supplementary material online, Appendix S2). We focus on three selected examples to illustrate key principles relevant for all current and future studies and list additional relevant studies in Table 3. The REVeAL-HF trial31 represents the latest addition to the field. Having recruited over 3000 patients within 1 year at a single healthcare system, the REVeAL-HF team demonstrated that such pragmatic trials are feasible. The nudge intervention consisted of changing the choice architecture of the EHR to display a 1-year mortality risk for patients admitted with heart failure (modelled from data generated within the EHR). The hypothesis tested was whether the display of this novel information affected downstream clinical decision-making such as the use of heart failure pharmacotherapies, onwards referrals to palliative care, and clinical outcomes including rehospitalizations and 1-year mortality. Although the trial demonstrated no overall effect for the CDSS nudge,41 several aspects of the study are worth noting for future endeavours: the description of CDSS testing to preserve computing speeds of the EHR platform via ‘run in’ periods; the detail of the alert design, including features such as font colour and data visualization; and engagement with clinical teams including pre-trial education at departmental meetings and opportunities for feedback to optimize the alert design. The ambition to generate novel insights rather than targeting the more traditional goal of improved guideline adherence represents a novel contribution in spite of the neutral result. The study authors have speculated that a nudge intervention more closely coupled to clinical actions could have made a difference to outcomes, rather than the supply of additional prognostic information alone. One missed opportunity was the discussion of whether clinicians accepted the accuracy of the prognostic algorithm within the CDSS alert. Would clinicians have been more likely to change their behaviour if they demonstrably trusted the accuracy of the model? Could trust be improved through a more explainable algorithm?42 And how can issues of algorithm aversion be planned for in future studies and practice, where professional ‘gut instinct’ may dominate an algorithm’s (superior) recommendation.43
List of selected studies and registered randomized controlled trials relating to nudge in cardiovascular medicine
Trial . | Study context . | Nudge . | Result . |
---|---|---|---|
REVeAL-HF NCT03845660 Knowledge generating | RCT conducted in hospitalized heart failure patients to determine the effect of alert on downstream clinical decision-making (N = 3124) | CDSS nudge alert presented to clinicians of an estimated 1-year mortality for admitted patients | No difference in primary clinical endpoint (composite of all-cause mortality or rehospitalization) |
AF-ALERT NCT02339493 Guideline directed | RCT conducted in patients with AF who were hospitalized but not prescribed anticoagulant therapy at the time of recruitment (N = 458) | CDSS nudge alert presented to clinicians which calculated the ChadsVasc score and recommended prescription of anticoagulant | Patients in alert group had 16.3% absolute increase in anticoagulation prescription during hospitalization and reduced the 90-day composite endpoint of death, MI, CVA, and systemic embolic event by 10.6% |
Active Choice in the EHR to Promote Statin Therapy NCT03271931 Guideline directed | Cluster RCT of alerts within the EHR for guideline-directed statin prescriptions in high-risk CVD patients using a three-arm design. (N = 11 693) | CDSS nudge alert presented to clinicians whenever a patient met eligibility criteria, according to ASCVD risk score or structured clinical diagnosis. An appropriate dose of atorvastatin was pre-selected | Neither active nor passive alerts increased optimum dose statin prescription rates compared with a baseline of 40.3% |
Electronic Warning System for Atrial Fibrillation NCT02455102 Guideline directed | RCT of alerts within the EHR to improve anticoagulation prescription among hospitalized patients with AF (N = 889) | CDSS nudge alert presented to clinicians which identified AF in structured and free-text entries, advised clinician to confirm the presence of AF, calculate the CHA2DS2-VASc, and to prescribe anticoagulation in indicated | Relative increase in adequate prescription rates by 38% compared with the control group |
PRESCRIBE NCT03021759 Guideline directed | Cluster RCT of a clinician-facing automated patient dashboard intervention on guideline-directed prescription of statin therapy (N = 4774) | Email nudge to primary care physicians with a link to a dashboard that listed patients eligible for stain prescription. Included reminders with peer review performance in the second intervention arm | Significant increase in statin prescription in the intervention arm, by between 4 and 6% depending on nudge alone vs. nudge with peer comparison |
ACTIVE-REWARD NCT02531022 Guideline directed | RCT in patients with ischaemic heart disease. Four-month intervention aimed at increasing physical activity (N = 105) | Wearable tracker, with daily nudge and feedback and small financial incentives if targets met | Compared with the standard of care, patients in the intervention arm had significant increase in daily steps taken during trial and follow-up (more than 1000 steps vs. control) |
ENCOURAGE NCT02490423 Guideline directed | RCT in patients seen within Cardiology clinics with an indication for statin therapy. Over a 12-month period (N = 182) | Multiple nudges sent across email, text, and telephone calls using AI to adapt their messaging and integration to individual home circumstances | Compared with the standard of care, adherence to statins increased by significant amount, more than 10% absolute increase in days covered |
EPIC-HF NCT03334188 Guideline directed | RCT of patients with HFREF seen at a scheduled cardiology clinic who were not on optimal doses of guideline-directed therapy (N = 306) | Patient-facing nudge which was an activation tool that included video content and a one-page checklist | Compared with the standard of care, 20% absolute increase in number of patients experiencing initiation or intensification of GDMT |
Effectiveness of Financial Incentives and Text Messages to Improve Health Care in Population With Moderate and High Cardiovascular Risk NCT03300154 Guideline directed | Cluster RCT conducted in primary care clinics in Argentina, three-arm trial comparing usual care with financial incentives or framing text messages to increase clinic attendance of patients at high risk of cardiovascular disease (>10% in 10 years). N = 917 | Patient-facing $10 food voucher to attend first clinic and enrolment into a lottery for subsequent clinic visit; OR nudges via text messages with standard message highlighting the benefit of healthcare sent weekly | Financial incentives group 25% absolute increase in attendance for first follow-up and 18% increase for second. Framing text messages, no significant difference |
PROMPT-HF NCT04514458 Guideline directed | Cluster RCT of tailored alerts within the EHR to improve guideline-directed medical therapy for HFREF patients | Nudge CDSS alert aimed at clinicians, to prescribe or intensify doses of beta-blockers, ACEi/ARB/ARNI, MRA, and SGLT2i | In recruitment |
NUDGE NCT03973931 Guideline directed | RCT of a patient-facing nudge, to encourage medication adherence across multitude of cardiovascular conditions | Text message-based generic or tailored nudges | Recruiting |
NCT03834155 Guideline directed | RCT of a clinician and patient-facing nudge, to encourage enrolment into cardiac rehabilitation | Nudge via one-page decision aid tool, text messages, and app | Recruiting |
Trial . | Study context . | Nudge . | Result . |
---|---|---|---|
REVeAL-HF NCT03845660 Knowledge generating | RCT conducted in hospitalized heart failure patients to determine the effect of alert on downstream clinical decision-making (N = 3124) | CDSS nudge alert presented to clinicians of an estimated 1-year mortality for admitted patients | No difference in primary clinical endpoint (composite of all-cause mortality or rehospitalization) |
AF-ALERT NCT02339493 Guideline directed | RCT conducted in patients with AF who were hospitalized but not prescribed anticoagulant therapy at the time of recruitment (N = 458) | CDSS nudge alert presented to clinicians which calculated the ChadsVasc score and recommended prescription of anticoagulant | Patients in alert group had 16.3% absolute increase in anticoagulation prescription during hospitalization and reduced the 90-day composite endpoint of death, MI, CVA, and systemic embolic event by 10.6% |
Active Choice in the EHR to Promote Statin Therapy NCT03271931 Guideline directed | Cluster RCT of alerts within the EHR for guideline-directed statin prescriptions in high-risk CVD patients using a three-arm design. (N = 11 693) | CDSS nudge alert presented to clinicians whenever a patient met eligibility criteria, according to ASCVD risk score or structured clinical diagnosis. An appropriate dose of atorvastatin was pre-selected | Neither active nor passive alerts increased optimum dose statin prescription rates compared with a baseline of 40.3% |
Electronic Warning System for Atrial Fibrillation NCT02455102 Guideline directed | RCT of alerts within the EHR to improve anticoagulation prescription among hospitalized patients with AF (N = 889) | CDSS nudge alert presented to clinicians which identified AF in structured and free-text entries, advised clinician to confirm the presence of AF, calculate the CHA2DS2-VASc, and to prescribe anticoagulation in indicated | Relative increase in adequate prescription rates by 38% compared with the control group |
PRESCRIBE NCT03021759 Guideline directed | Cluster RCT of a clinician-facing automated patient dashboard intervention on guideline-directed prescription of statin therapy (N = 4774) | Email nudge to primary care physicians with a link to a dashboard that listed patients eligible for stain prescription. Included reminders with peer review performance in the second intervention arm | Significant increase in statin prescription in the intervention arm, by between 4 and 6% depending on nudge alone vs. nudge with peer comparison |
ACTIVE-REWARD NCT02531022 Guideline directed | RCT in patients with ischaemic heart disease. Four-month intervention aimed at increasing physical activity (N = 105) | Wearable tracker, with daily nudge and feedback and small financial incentives if targets met | Compared with the standard of care, patients in the intervention arm had significant increase in daily steps taken during trial and follow-up (more than 1000 steps vs. control) |
ENCOURAGE NCT02490423 Guideline directed | RCT in patients seen within Cardiology clinics with an indication for statin therapy. Over a 12-month period (N = 182) | Multiple nudges sent across email, text, and telephone calls using AI to adapt their messaging and integration to individual home circumstances | Compared with the standard of care, adherence to statins increased by significant amount, more than 10% absolute increase in days covered |
EPIC-HF NCT03334188 Guideline directed | RCT of patients with HFREF seen at a scheduled cardiology clinic who were not on optimal doses of guideline-directed therapy (N = 306) | Patient-facing nudge which was an activation tool that included video content and a one-page checklist | Compared with the standard of care, 20% absolute increase in number of patients experiencing initiation or intensification of GDMT |
Effectiveness of Financial Incentives and Text Messages to Improve Health Care in Population With Moderate and High Cardiovascular Risk NCT03300154 Guideline directed | Cluster RCT conducted in primary care clinics in Argentina, three-arm trial comparing usual care with financial incentives or framing text messages to increase clinic attendance of patients at high risk of cardiovascular disease (>10% in 10 years). N = 917 | Patient-facing $10 food voucher to attend first clinic and enrolment into a lottery for subsequent clinic visit; OR nudges via text messages with standard message highlighting the benefit of healthcare sent weekly | Financial incentives group 25% absolute increase in attendance for first follow-up and 18% increase for second. Framing text messages, no significant difference |
PROMPT-HF NCT04514458 Guideline directed | Cluster RCT of tailored alerts within the EHR to improve guideline-directed medical therapy for HFREF patients | Nudge CDSS alert aimed at clinicians, to prescribe or intensify doses of beta-blockers, ACEi/ARB/ARNI, MRA, and SGLT2i | In recruitment |
NUDGE NCT03973931 Guideline directed | RCT of a patient-facing nudge, to encourage medication adherence across multitude of cardiovascular conditions | Text message-based generic or tailored nudges | Recruiting |
NCT03834155 Guideline directed | RCT of a clinician and patient-facing nudge, to encourage enrolment into cardiac rehabilitation | Nudge via one-page decision aid tool, text messages, and app | Recruiting |
List of selected studies and registered randomized controlled trials relating to nudge in cardiovascular medicine
Trial . | Study context . | Nudge . | Result . |
---|---|---|---|
REVeAL-HF NCT03845660 Knowledge generating | RCT conducted in hospitalized heart failure patients to determine the effect of alert on downstream clinical decision-making (N = 3124) | CDSS nudge alert presented to clinicians of an estimated 1-year mortality for admitted patients | No difference in primary clinical endpoint (composite of all-cause mortality or rehospitalization) |
AF-ALERT NCT02339493 Guideline directed | RCT conducted in patients with AF who were hospitalized but not prescribed anticoagulant therapy at the time of recruitment (N = 458) | CDSS nudge alert presented to clinicians which calculated the ChadsVasc score and recommended prescription of anticoagulant | Patients in alert group had 16.3% absolute increase in anticoagulation prescription during hospitalization and reduced the 90-day composite endpoint of death, MI, CVA, and systemic embolic event by 10.6% |
Active Choice in the EHR to Promote Statin Therapy NCT03271931 Guideline directed | Cluster RCT of alerts within the EHR for guideline-directed statin prescriptions in high-risk CVD patients using a three-arm design. (N = 11 693) | CDSS nudge alert presented to clinicians whenever a patient met eligibility criteria, according to ASCVD risk score or structured clinical diagnosis. An appropriate dose of atorvastatin was pre-selected | Neither active nor passive alerts increased optimum dose statin prescription rates compared with a baseline of 40.3% |
Electronic Warning System for Atrial Fibrillation NCT02455102 Guideline directed | RCT of alerts within the EHR to improve anticoagulation prescription among hospitalized patients with AF (N = 889) | CDSS nudge alert presented to clinicians which identified AF in structured and free-text entries, advised clinician to confirm the presence of AF, calculate the CHA2DS2-VASc, and to prescribe anticoagulation in indicated | Relative increase in adequate prescription rates by 38% compared with the control group |
PRESCRIBE NCT03021759 Guideline directed | Cluster RCT of a clinician-facing automated patient dashboard intervention on guideline-directed prescription of statin therapy (N = 4774) | Email nudge to primary care physicians with a link to a dashboard that listed patients eligible for stain prescription. Included reminders with peer review performance in the second intervention arm | Significant increase in statin prescription in the intervention arm, by between 4 and 6% depending on nudge alone vs. nudge with peer comparison |
ACTIVE-REWARD NCT02531022 Guideline directed | RCT in patients with ischaemic heart disease. Four-month intervention aimed at increasing physical activity (N = 105) | Wearable tracker, with daily nudge and feedback and small financial incentives if targets met | Compared with the standard of care, patients in the intervention arm had significant increase in daily steps taken during trial and follow-up (more than 1000 steps vs. control) |
ENCOURAGE NCT02490423 Guideline directed | RCT in patients seen within Cardiology clinics with an indication for statin therapy. Over a 12-month period (N = 182) | Multiple nudges sent across email, text, and telephone calls using AI to adapt their messaging and integration to individual home circumstances | Compared with the standard of care, adherence to statins increased by significant amount, more than 10% absolute increase in days covered |
EPIC-HF NCT03334188 Guideline directed | RCT of patients with HFREF seen at a scheduled cardiology clinic who were not on optimal doses of guideline-directed therapy (N = 306) | Patient-facing nudge which was an activation tool that included video content and a one-page checklist | Compared with the standard of care, 20% absolute increase in number of patients experiencing initiation or intensification of GDMT |
Effectiveness of Financial Incentives and Text Messages to Improve Health Care in Population With Moderate and High Cardiovascular Risk NCT03300154 Guideline directed | Cluster RCT conducted in primary care clinics in Argentina, three-arm trial comparing usual care with financial incentives or framing text messages to increase clinic attendance of patients at high risk of cardiovascular disease (>10% in 10 years). N = 917 | Patient-facing $10 food voucher to attend first clinic and enrolment into a lottery for subsequent clinic visit; OR nudges via text messages with standard message highlighting the benefit of healthcare sent weekly | Financial incentives group 25% absolute increase in attendance for first follow-up and 18% increase for second. Framing text messages, no significant difference |
PROMPT-HF NCT04514458 Guideline directed | Cluster RCT of tailored alerts within the EHR to improve guideline-directed medical therapy for HFREF patients | Nudge CDSS alert aimed at clinicians, to prescribe or intensify doses of beta-blockers, ACEi/ARB/ARNI, MRA, and SGLT2i | In recruitment |
NUDGE NCT03973931 Guideline directed | RCT of a patient-facing nudge, to encourage medication adherence across multitude of cardiovascular conditions | Text message-based generic or tailored nudges | Recruiting |
NCT03834155 Guideline directed | RCT of a clinician and patient-facing nudge, to encourage enrolment into cardiac rehabilitation | Nudge via one-page decision aid tool, text messages, and app | Recruiting |
Trial . | Study context . | Nudge . | Result . |
---|---|---|---|
REVeAL-HF NCT03845660 Knowledge generating | RCT conducted in hospitalized heart failure patients to determine the effect of alert on downstream clinical decision-making (N = 3124) | CDSS nudge alert presented to clinicians of an estimated 1-year mortality for admitted patients | No difference in primary clinical endpoint (composite of all-cause mortality or rehospitalization) |
AF-ALERT NCT02339493 Guideline directed | RCT conducted in patients with AF who were hospitalized but not prescribed anticoagulant therapy at the time of recruitment (N = 458) | CDSS nudge alert presented to clinicians which calculated the ChadsVasc score and recommended prescription of anticoagulant | Patients in alert group had 16.3% absolute increase in anticoagulation prescription during hospitalization and reduced the 90-day composite endpoint of death, MI, CVA, and systemic embolic event by 10.6% |
Active Choice in the EHR to Promote Statin Therapy NCT03271931 Guideline directed | Cluster RCT of alerts within the EHR for guideline-directed statin prescriptions in high-risk CVD patients using a three-arm design. (N = 11 693) | CDSS nudge alert presented to clinicians whenever a patient met eligibility criteria, according to ASCVD risk score or structured clinical diagnosis. An appropriate dose of atorvastatin was pre-selected | Neither active nor passive alerts increased optimum dose statin prescription rates compared with a baseline of 40.3% |
Electronic Warning System for Atrial Fibrillation NCT02455102 Guideline directed | RCT of alerts within the EHR to improve anticoagulation prescription among hospitalized patients with AF (N = 889) | CDSS nudge alert presented to clinicians which identified AF in structured and free-text entries, advised clinician to confirm the presence of AF, calculate the CHA2DS2-VASc, and to prescribe anticoagulation in indicated | Relative increase in adequate prescription rates by 38% compared with the control group |
PRESCRIBE NCT03021759 Guideline directed | Cluster RCT of a clinician-facing automated patient dashboard intervention on guideline-directed prescription of statin therapy (N = 4774) | Email nudge to primary care physicians with a link to a dashboard that listed patients eligible for stain prescription. Included reminders with peer review performance in the second intervention arm | Significant increase in statin prescription in the intervention arm, by between 4 and 6% depending on nudge alone vs. nudge with peer comparison |
ACTIVE-REWARD NCT02531022 Guideline directed | RCT in patients with ischaemic heart disease. Four-month intervention aimed at increasing physical activity (N = 105) | Wearable tracker, with daily nudge and feedback and small financial incentives if targets met | Compared with the standard of care, patients in the intervention arm had significant increase in daily steps taken during trial and follow-up (more than 1000 steps vs. control) |
ENCOURAGE NCT02490423 Guideline directed | RCT in patients seen within Cardiology clinics with an indication for statin therapy. Over a 12-month period (N = 182) | Multiple nudges sent across email, text, and telephone calls using AI to adapt their messaging and integration to individual home circumstances | Compared with the standard of care, adherence to statins increased by significant amount, more than 10% absolute increase in days covered |
EPIC-HF NCT03334188 Guideline directed | RCT of patients with HFREF seen at a scheduled cardiology clinic who were not on optimal doses of guideline-directed therapy (N = 306) | Patient-facing nudge which was an activation tool that included video content and a one-page checklist | Compared with the standard of care, 20% absolute increase in number of patients experiencing initiation or intensification of GDMT |
Effectiveness of Financial Incentives and Text Messages to Improve Health Care in Population With Moderate and High Cardiovascular Risk NCT03300154 Guideline directed | Cluster RCT conducted in primary care clinics in Argentina, three-arm trial comparing usual care with financial incentives or framing text messages to increase clinic attendance of patients at high risk of cardiovascular disease (>10% in 10 years). N = 917 | Patient-facing $10 food voucher to attend first clinic and enrolment into a lottery for subsequent clinic visit; OR nudges via text messages with standard message highlighting the benefit of healthcare sent weekly | Financial incentives group 25% absolute increase in attendance for first follow-up and 18% increase for second. Framing text messages, no significant difference |
PROMPT-HF NCT04514458 Guideline directed | Cluster RCT of tailored alerts within the EHR to improve guideline-directed medical therapy for HFREF patients | Nudge CDSS alert aimed at clinicians, to prescribe or intensify doses of beta-blockers, ACEi/ARB/ARNI, MRA, and SGLT2i | In recruitment |
NUDGE NCT03973931 Guideline directed | RCT of a patient-facing nudge, to encourage medication adherence across multitude of cardiovascular conditions | Text message-based generic or tailored nudges | Recruiting |
NCT03834155 Guideline directed | RCT of a clinician and patient-facing nudge, to encourage enrolment into cardiac rehabilitation | Nudge via one-page decision aid tool, text messages, and app | Recruiting |
The issue of trust and understanding of CDSS recommendations were additionally highlighted by a recent study of nudge alerts on cardiologists’ prescription rates of statins.44 Eighty-two attending cardiologists were recruited as part of a cluster RCT design. Regardless of either passive alert (which required manual access) or an active interruptive alert to accept or decline guideline-directed statin therapy, there were no significant changes in statin prescriptions during the 1-year study. The authors commented that clinicians in the study were unsure regarding the clinical justification behind the CDSS recommendation, emphasizing the influence of clinical teams’ cognitive heuristics and baseline models of working on nudge deployment. Contrastingly AF-ALERT,4 which also evaluated a CDSS nudge for adherence to GDT demonstrated a significant difference in anticoagulation prescribing behaviour, and crucially, clinical outcomes, as highlighted in Table 2. Once more, clinical context is a key to understand the different trial results—perhaps the patient benefit of anticoagulation and stroke risk reduction were more salient to clinicians than the benefits of a statin prescription. Of note, all three highlighted trials were conducted in the USA and received ethical approval for waiver of consent.
The feasibility of trial conduct within routine workflows and end-user acceptability are of particular salience given the trend of decision-making by clinicians that are increasingly abdicated to diagnostic and therapeutic pathways and protocols. Research questions such as ‘what is the rate of clinician adherence to CDSS and which factors influence these’ will become key hypotheses to test and answer. And while the eye-catching AF-ALERT trial4 demonstrated a 55% reduction in composite clinical outcomes, no details on clinician demographics or local clinical context were reported, tempering the generalizability of such a result.
Overall, nudge remains unfamiliar to the cardiovascular community despite its widespread use in other scientific disciplines and popular science parlance since 2008. Of all ESC professional guidelines, nudge is mentioned only in passing in the previous 2016 ESC prevention guideline:32 ‘Strategies such as “nudging” (to push mildly) and “default” have been proposed as tools. By changing the context to make healthy decisions the default, the individual is nudged in the healthy direction’.
Evidence from other contexts
Nudges have influenced clinician behaviour in a variety of other contexts and several studies stand out45–47 in terms of their generalizability to a cardiology audience (Table 4).
Author . | Study context . | Result . | Lessons . |
---|---|---|---|
Montoy et al.45 | Randomization of clinicians to different prepopulated quantities of discharge prescriptions for opioids in emergency departments | A lower default quantity was associated with fewer prescriptions | To minimize possible bias, the study intervention was made without announcement, and prescribers were not informed of the study itself |
Manz et al.46 | A stepped-wedge cluster RCT, with behavioural nudges directed at oncologists, combined with machine learning mortality predictions positively influenced clinician behaviour through initiating more care planning conversations | Absolute increase of 4% among all patients and 11% in those with high predicted mortality | Integrating behavioural economic principles into the machine learning-based nudge, as a series of co-interventions including peer comparison, feedback, and opt-out reminders |
Sacarny et al.47 | RCT comparing peer comparison letter vs. placebo letter, across 5055 highest Medicare prescribers of the antipsychotic quetiapine | Over 9 months, the treatment arm supplied 11.1% fewer quetiapine days per prescriber vs. the control arm | Effects were larger than those observed in existing large-scale behavioural interventions, potentially because of the content of the peer comparison letter |
Author . | Study context . | Result . | Lessons . |
---|---|---|---|
Montoy et al.45 | Randomization of clinicians to different prepopulated quantities of discharge prescriptions for opioids in emergency departments | A lower default quantity was associated with fewer prescriptions | To minimize possible bias, the study intervention was made without announcement, and prescribers were not informed of the study itself |
Manz et al.46 | A stepped-wedge cluster RCT, with behavioural nudges directed at oncologists, combined with machine learning mortality predictions positively influenced clinician behaviour through initiating more care planning conversations | Absolute increase of 4% among all patients and 11% in those with high predicted mortality | Integrating behavioural economic principles into the machine learning-based nudge, as a series of co-interventions including peer comparison, feedback, and opt-out reminders |
Sacarny et al.47 | RCT comparing peer comparison letter vs. placebo letter, across 5055 highest Medicare prescribers of the antipsychotic quetiapine | Over 9 months, the treatment arm supplied 11.1% fewer quetiapine days per prescriber vs. the control arm | Effects were larger than those observed in existing large-scale behavioural interventions, potentially because of the content of the peer comparison letter |
Studies stretch across medical contexts and also include non-digital platforms but with a generalizable lesson to all EHR-based nudges.
Author . | Study context . | Result . | Lessons . |
---|---|---|---|
Montoy et al.45 | Randomization of clinicians to different prepopulated quantities of discharge prescriptions for opioids in emergency departments | A lower default quantity was associated with fewer prescriptions | To minimize possible bias, the study intervention was made without announcement, and prescribers were not informed of the study itself |
Manz et al.46 | A stepped-wedge cluster RCT, with behavioural nudges directed at oncologists, combined with machine learning mortality predictions positively influenced clinician behaviour through initiating more care planning conversations | Absolute increase of 4% among all patients and 11% in those with high predicted mortality | Integrating behavioural economic principles into the machine learning-based nudge, as a series of co-interventions including peer comparison, feedback, and opt-out reminders |
Sacarny et al.47 | RCT comparing peer comparison letter vs. placebo letter, across 5055 highest Medicare prescribers of the antipsychotic quetiapine | Over 9 months, the treatment arm supplied 11.1% fewer quetiapine days per prescriber vs. the control arm | Effects were larger than those observed in existing large-scale behavioural interventions, potentially because of the content of the peer comparison letter |
Author . | Study context . | Result . | Lessons . |
---|---|---|---|
Montoy et al.45 | Randomization of clinicians to different prepopulated quantities of discharge prescriptions for opioids in emergency departments | A lower default quantity was associated with fewer prescriptions | To minimize possible bias, the study intervention was made without announcement, and prescribers were not informed of the study itself |
Manz et al.46 | A stepped-wedge cluster RCT, with behavioural nudges directed at oncologists, combined with machine learning mortality predictions positively influenced clinician behaviour through initiating more care planning conversations | Absolute increase of 4% among all patients and 11% in those with high predicted mortality | Integrating behavioural economic principles into the machine learning-based nudge, as a series of co-interventions including peer comparison, feedback, and opt-out reminders |
Sacarny et al.47 | RCT comparing peer comparison letter vs. placebo letter, across 5055 highest Medicare prescribers of the antipsychotic quetiapine | Over 9 months, the treatment arm supplied 11.1% fewer quetiapine days per prescriber vs. the control arm | Effects were larger than those observed in existing large-scale behavioural interventions, potentially because of the content of the peer comparison letter |
Studies stretch across medical contexts and also include non-digital platforms but with a generalizable lesson to all EHR-based nudges.
The recurring theme for successful nudges appears to be a greater focus on understanding the nuances of how physicians make decisions and how factors such as the Hawthorne effect48 need to be navigated and depending on the context, leveraged, in order to drive improvements. For cardiologists, comparison with peers49 may be one strategy to frame nudges to help drive behaviour change. Presently, the additional factors that can have an impact on the likelihood of nudge success, such as time constraints and the organizational structure of teams tasked to deliver nudge interventions, have only a paucity of data to describe their effects in specific clinical contexts.50
Nudges for patients
Nudges for patients have mainly focused on the intent–behaviour gap51 and whether communication of healthcare information results in healthier choices. A 2016 meta-analysis examined the effect of presenting individual risk to patients52 and found no significant effects on smoking cessation, diet or physical activity, or even motivation to change behaviour. More optimistically, a 2021 pilot study of nudges in the form of text message reminders for medication refills demonstrated early signals of possible benefit,53 further highlighting the importance of choosing the right context. Follow-up work as part of a larger study which includes measuring clinical outcomes is now underway.54
Prospective research of patient-facing CDSS nudges will need to match the growth of health technology companies who offer services to patients, often in a primary prevention cohort.55 Therefore, while current evidence for broad nudge interventions at a population level is mixed,56 this may soon change over time. For patients with existing conditions, examples of commitment contracts have shown success—e.g. in adherence to optimal secondary prevention in the context of heart failure,57 though a systematic review of nudges for patients with chronic disease emphasized the heterogeneity of study designs, results, and types of nudges.58 While the platforms that deliver patient-facing nudges can vary considerably, one recent example of a multi-faceted intervention stems from the EPIC-HF trial40—there was patient choice in receiving a video and checklist nudge by email, text, or both. The framing of the intervention encouraged patients to ‘promote a conversation about changing at least one thing to optimize medication management’ and 49% in the intervention group received initiation or intensification compared with 30% in the control group.
Therefore, while there are many opportunities, the risk of leaving patient-facing nudge interventions understudied and without formal regulation will manifest in the unintended consequences they may have on healthcare systems, with either downstream under or over-medicalization of problems.13
Limitations of nudge
Greater adoption and testing of nudges will require groups to deal with the following considerations.
Misuse
A key criticism of nudge is misinterpreting the breadth of its use in addressing areas reserved for wider policy and governmental action. For example, nudge was erroneously framed in the UK as a panacea for all the challenges set forth in the Marmot review59 of health inequalities in 2011. The over-reliance on simply changing an individuals’ choice architecture ahead of using other policy tools drew firm criticism from Sundar:60 ‘…effective sewage systems, clean running water, workplace safety laws, and smoking bans … public health needs to go back to basics and deal with inequity at a structural level rather than relying on nudge theory to change individual behaviour’.
A similar critique applies to CDSS-based nudges aimed at clinicians—we must not forgo the fundamentals of clinician education, audit and feedback, and other helpful tools that improve clinical knowledge and patient care. There are also other prominent examples of nudge misuse. In January 2020, an EHR vendor admitted colluding with an opioid manufacturer to create a pain alert CDSS which encouraged physicians to prescribe more extended-release opioid, thus selling more of the manufacturer’s product.61 The true prevalence of political and commercial influences on nudge design is beyond the scope of this review but these are important to consider in any context.
Lack of reproducibility of effect size and direction
Changes in behaviour are not entirely predictable—the ‘dose’ or ‘route’ of a nudge represent areas of considerable variation whenever they are evaluated and local context in modifying effects can be key. For instance, there are examples of nudge use that have resulted in heterogeneous effects. In the setting of alerts for acute kidney injury, a multicentre RCT which was negative contained signals of harm in some of the study sites that could not be attributed to a specific process.62 Many have suggested that nudges should be thought of as inductive rules of thumb which require careful testing and evaluation, rather than medicinal products with a predictable mechanism of action and side effect profile.63 Therefore, similar to when ‘health policy reversals’ occur, the rapid testing of nudges as part of RCTs and other experimental designs, tailored to the local context, will be the only way to effectively prescribe and ‘deprescribe’ CDSS nudge alerts.64
Lack of standardized nudging and dataset quality
Clinical decision support systems nudges may be required to work across multiple different EHR platforms—their recommendations and delivery will only be as effective as the datasets in which they are embedded within. Encouragingly, there is progress in moving towards increased data quality and interoperability, for both data and CDSS use. For example, Substitutable Medical Apps, Reusable Technology, Fast Healthcare Interoperability Resources, the Observational Medical Outcomes Partnership common data model, and Guideline Definition Language v2.65–67 Rather than a uniform, homogeneous nudge across different EHR platforms, the process and function of nudges could be standardized, rather than their precise form.68 Thus for computerized nudges, instead of designing a single set of CDSS code, adaptations to different EHR platforms to retain a standardized set of functions may be required. This review has deliberately focused on the technological frontier. However, a pre-requisite for deploying effective CDSS nudges is the digital maturity of the healthcare system, including its EHR platform as well as broader connectivity—digital maturity frameworks have been proposed before and can help policymakers identify gaps to work on.69,70 Co-funding of EHR adoption in combined research and clinical budgets may accelerate system maturity.
Nudge fatigue and automation bias
Alert or nudge fatigue has been described as a multi-faceted concept that can cause high override rates of CDSS recommendations, through either clinician de-sensitization or cognitive overload (where too much information is presented).71 In spite of these risks, in the comprehensive meta-analysis of CDSS by Kwan et al.,12 only 14% of the trials considered alert fatigue. While the effect of alert fatigue was non-significant in these studies, the point remains regarding its unknown effect in the majority of CDSS trials. A better understanding of how and when alert fatigue becomes relevant, and in whom, e.g. senior clinicians vs. trainees, represent gaps in knowledge which require further research.
A starting place will be more studies such which can quantify the phenomenon through relevant surrogate measures—for example, a recent pseudorandomized trial of a CDSS alert to improve prescription of GDT medications in heart failure patients reported the number of alerts needed to change the quality of care—an estimate comparable to the number needed to treat in clinical trial.72 Their results were consistent with a high alert burden—alerts were triggered an average of over 25 times per hospitalization with a response rate of <2%. In the trial, 405 interruptive alerts were needed to be triggered for one additional patient to be discharged on an angiotensin-converting enzyme inhibitor or angiotensin receptor blocker. Echoing the lessons from previous trials about context, the study group introduced a nudge when prescribing performance was already high (∼80% prescription rates) and in all hospitalizations, not just those related to decompensation of heart failure.
The mitigation of alert fatigue through tailoring the frequency and design of nudge alerts may eventually become a growing use case for AI in healthcare. This contrasts with AI and CDSS alert deployment more generally, where a common vulnerability for clinicians harnessing their power is automation bias. Here, reflexive adherence to recommendations may also lead to errors and patient harm. As clinicians, preserving the virtues of independent problem-solving and vigilance in continually asking whether a recommendation from a CDSS should be overridden will become an essential skill.
Testing nudges
The above limitations could be better accounted for collectively through the formation of ‘nudge units’, with a range of roles previously highlighted (Table 5).73
The role of dedicated units to help deploy nudges within healthcare. Adapted from Patel et al.73
Deploying nudges in healthcare . | Roles of a nudge unit . |
---|---|
Identify opportunities | Identify suboptimal care and map decision-making processes. Target a specific change that could most effectively shift behaviour. Evaluate feasibility of a nudge-based intervention in the local context, e.g. ICT infrastructure and staff buy-in |
Type of research question answered |
|
Measure outcomes | Including process measures (e.g. prescribing patterns, referral rates) and patient outcomes (e.g. length of stay, readmissions, mortality) |
Pragmatically implement | Assess the effects on clinician workflow. Consider unintended consequences, their mitigations, and scalability |
Align stakeholders | Obtain buy-in from system and clinical leadership as well as support and feedback from frontline clinicians |
Compare effectiveness and iterate design | Design interventions in a testable manner and evaluate changes from the intervention (fidelity) including findings to further optimize nudge design |
Deploying nudges in healthcare . | Roles of a nudge unit . |
---|---|
Identify opportunities | Identify suboptimal care and map decision-making processes. Target a specific change that could most effectively shift behaviour. Evaluate feasibility of a nudge-based intervention in the local context, e.g. ICT infrastructure and staff buy-in |
Type of research question answered |
|
Measure outcomes | Including process measures (e.g. prescribing patterns, referral rates) and patient outcomes (e.g. length of stay, readmissions, mortality) |
Pragmatically implement | Assess the effects on clinician workflow. Consider unintended consequences, their mitigations, and scalability |
Align stakeholders | Obtain buy-in from system and clinical leadership as well as support and feedback from frontline clinicians |
Compare effectiveness and iterate design | Design interventions in a testable manner and evaluate changes from the intervention (fidelity) including findings to further optimize nudge design |
The role of dedicated units to help deploy nudges within healthcare. Adapted from Patel et al.73
Deploying nudges in healthcare . | Roles of a nudge unit . |
---|---|
Identify opportunities | Identify suboptimal care and map decision-making processes. Target a specific change that could most effectively shift behaviour. Evaluate feasibility of a nudge-based intervention in the local context, e.g. ICT infrastructure and staff buy-in |
Type of research question answered |
|
Measure outcomes | Including process measures (e.g. prescribing patterns, referral rates) and patient outcomes (e.g. length of stay, readmissions, mortality) |
Pragmatically implement | Assess the effects on clinician workflow. Consider unintended consequences, their mitigations, and scalability |
Align stakeholders | Obtain buy-in from system and clinical leadership as well as support and feedback from frontline clinicians |
Compare effectiveness and iterate design | Design interventions in a testable manner and evaluate changes from the intervention (fidelity) including findings to further optimize nudge design |
Deploying nudges in healthcare . | Roles of a nudge unit . |
---|---|
Identify opportunities | Identify suboptimal care and map decision-making processes. Target a specific change that could most effectively shift behaviour. Evaluate feasibility of a nudge-based intervention in the local context, e.g. ICT infrastructure and staff buy-in |
Type of research question answered |
|
Measure outcomes | Including process measures (e.g. prescribing patterns, referral rates) and patient outcomes (e.g. length of stay, readmissions, mortality) |
Pragmatically implement | Assess the effects on clinician workflow. Consider unintended consequences, their mitigations, and scalability |
Align stakeholders | Obtain buy-in from system and clinical leadership as well as support and feedback from frontline clinicians |
Compare effectiveness and iterate design | Design interventions in a testable manner and evaluate changes from the intervention (fidelity) including findings to further optimize nudge design |
An overarching review of work from nudge units, comparing published meta-analyses as well as trials run by two of the largest nudge units in the USA, demonstrated that in 126 studies covering more than 20 million patients, the average nudge effect size was between 8 and 34% depending on the context.74 Importantly, the ability to use different types of nudge designs within a CDSS—such as harnessing saliency features or modifying default choices18—as well as using nudges alongside co-interventions ranging from education to audit and feedback, represent opportunities that require the experience and expertise of multidisciplinary teams, grouped as nudge units, to fully exploit.
Greater collaboration between practising clinicians, academics, and nudge units could rationalize the application of nudge strategies, stratified according to acute and chronic settings within cardiovascular medicine. Here the experience of clinicians may offer fundamental principles for good nudge design and appropriate choice contexts. For example, in an urgent clinical scenario, a CDSS alert may be ignored and multiple choices of action may be distracting.
Meanwhile, in a chronic setting, greater nuance and weighting towards patient preference may predominate and clinician bandwidth could stretch to considering multiple alerts if appropriate to their workflow. Further use cases of nudge could emerge to bridge notable gaps in traditional cardiovascular research—for instance, helping to promote better documentation of patient-reported outcome measures, an area that EHR vendors are already facilitating.75
The evolving science of measuring EHR use, and exploiting automated data log capture at scale, means that additional and parallel research questions may be answered. This could include assessing how tailored nudges could be used to reduce unwarranted variation in EHR use among clinicians. For example, in one study, female ambulatory physicians across specialty disciplines spent 33 more minutes in the EHR per day than male physicians (102.2 vs. 68.8 min).76
Nudges in areas of practice with clinical equipoise
Perhaps the most impactful areas for the testing of future nudges rests with identifying scenarios where there is significant variation in practice among clinicians. Such variation may either be warranted or unwarranted. The latter is the target for quality improvement efforts and where most nudges currently operate, while the former represents the signature of equipoise being present. A lack of robust evidence base to inform best practice may represent the ideal opportunity context needed for pragmatic trials of nudge interventions. The use of CDSS nudges to systematically test one set of actions, as part of an RCT compared with the heterogeneous standard of care, may generate novel insights. One example could be the threshold at which potassium or magnesium is supplemented in the context of acute arrhythmia and low-normal values.77 For cardiologists, the scale of the opportunity may be significant, given that only a minority of recommendations in the current AHA or ESC guidelines is informed by Level A evidence.1,78 Further, if and when insights are generated, there could be the virtuous cycle of nudge use in studying the adoption of such knowledge generation and by wider extension, implementation of professional guidelines. In a recent meta-analysis of studies which embedded appropriate use criteria specified by the American College of Cardiology into routine clinical workflows, none used nudges.79
As an extension, nudge testing in the context of clinical equipoise should not necessarily default to traditional two-arm RCT designs—an adaptive trial design should be explored by every learning hospital and health system of the future.80 One example is NUDGE-EHR, which initially includes 16 treatment arms and seeks to determine which form of nudge is superior at reducing the prescription of high-risk medications (benzodiazepines, Z drugs, and anticholinergics) among older adults.81
Ensuring an open dialogue with patients will be key to studies of the future—increasing awareness of the degree of variation of clinician decision-making alongside the benefits and risks of being involved in pragmatic research, and randomization at the point of routine care, will help to safeguard against situations where there are unclear explanations of how decisions have been made. The broader issues of consent, shared decision-making, and how the black box of expert clinicians ‘arrive’ at decisions fall outside the scope of this review but represent areas for future work, particularly at the human–computer interface with future AI (AI-CDSS).82
Personalized nudging and risk tolerance
As partnerships between cardiovascular science and computer science grow, ambitious visions of digital twins83 and AI-powered informatics consults84 may one day be realized. There will be fundamental changes to working in medicine as well as how medicine will work. Although the application of AI to healthcare is still in its infancy,85 the increased testing of AI algorithms as part of AI-CDSS and the role of nudge to maximize their intended ‘dose’ will represent forthcoming research priorities.
Extensions to personalized nudging for both clinicians and patients alike may occur, with individualized communication of risk an area that could change the current consent landscape as applied to medical procedures.86
Alongside these research questions and the formation of nudge units, the requirement for interdisciplinary training programmes for clinical researchers and more ambitious funding programmes from policy makers, such as the ARPA programme in the UK, will be key.87 Fostering collaborations throughout the careers of practicing cardiologists and domain experts responsible for nudge design and human–computer interfaces will avoid artificial gaps being created between nudge theory and nudge practice.7,88
While our review is not intended to make a forecast about how successful nudges could change the practice of cardiology in the years to come, by adopting rapid cycle testing,89 their ‘blockbuster’ potential may not look like the traditional ‘one size fits all’ tablet or procedure that we are used to. Instead, nudges applied universally would be shaped by local practice patterns and idiosyncrasies so that a ‘thousand flowers bloom’ model,90,91 with precise nudge effects varying by local context materializes. As more data become curated, linked, and ingested into (AI)-CDSS models,82 nudges will become increasingly sophisticated through their (automated) deployment by ‘electronic health engines’ rather than EHRs—integrating factors including social and economic drivers of health, behavioural risks, genomic, and citizen-generated data.92
Conclusion
In the age of LHSs and progressive advancements in data science, the testing of nudge-based interventions for clinicians and patients alike represents a rich opportunity to systematically reduce evidence-practice gaps as well as generate new evidence that could improve care in many areas of cardiovascular medicine and beyond. Increasing collaborations and opportunities for doctors and researchers to work across professional boundaries will help facilitate collective, bespoke nudge trials that could deliver blockbuster results, an outcome that is increasingly hard to observe in traditional RCT designs.
Supplementary material
Supplementary material is available at European Heart Journal online.
Acknowledgements
The authors thank F. Perry Wilson, MD MSCE, who helped to review an earlier version of this manuscript.
Funding
No specific funding is relevant to this work.
Conflict of interest: none declared.
References