-
PDF
- Split View
-
Views
-
Cite
Cite
J Zheng, G Fu, D Struppa, I Abudayyeh, M Yacoub, H El-Askary, X Du, C Rakovski, High precision machine learning-enabled ECG algorithm for predicting sites of idiopathic ventricular arrhythmia origin, European Heart Journal, Volume 42, Issue Supplement_1, October 2021, ehab724.0303, https://doi.org/10.1093/eurheartj/ehab724.0303
- Share Icon Share
Abstract
Radiofrequency catheter ablation (CA) is an efficient antiarrhythmic treatment with a class I indication for idiopathic ventricular arrhythmia (IVA). The accurate prediction of the origins of IVA can significantly increase the procedure success rate, reduce operation duration and decrease the risk of complications. The present work proposes an ECG analysis algorithm to estimate 21 possible origins of idiopathic ventricular arrhythmia at a clinical-grade level accuracy, which include left coronary cusp (LCC), right coronary cusp (RCC), aortomitral continuity (AMC), summit, LCC-RCC commissure, left His bundle, mitral valve (MV), left septal including left anterior fascicle (LAF), left posterior fascicle (LPF), left anterior papillary muscle (LAPM), left posterior papillary muscle (LPPM), anterior cusp (AC), left cusp (LC), right cusp (RC), RVOT septal, free wall, right His bundle, tricuspid valve (TV), and right anterior papillary muscle (RAPM).
A total of 18,612 ECG recordings extracted from 545 patients who underwent successful CA to treat IVA were proportionally sampled into training, validation and testing cohorts. We designed four classification schemes responding to different hierarchical levels of the possible IVA origins. The first scheme will help the operators to figure out the origin from epicardium of left ventricular summit, right, and left ventricle. The second one can separate origins from left/right outflow tract and left/right non-out flow tract, respectively. The third one is able to predict 18 anatomical locations, and the fourth scheme can distinguish 21 possible sites. For every classification scheme, we compared 98 distinct machine learning models with optimized hyperparameter values obtained through extensive grid search and reported an optimal algorithm with the highest accuracy scores attained on the validation cohorts.
In the first classification scheme used to predict right ventricular endocardium, left ventricular endocardium, and epicardium of left ventricular summit, the model achieved an accuracy of 99.79 (99.41–99.89) and a F1-score of 99.84 (99.6–99.96). For scheme 2, the proposed method reached an accuracy of 99.62 (99.09–99.78) and a F1-score of 99.42 (98.79–99.75). For scheme 3, the model achieved an accuracy of 97.78 (96.76–98.41), a F1-score of 97.74 (94.15–99.73), and an adjusted accuracy of 98.53 (98.33–99.15). For scheme 4 that can distinguish 21 origin sites, the proposed model attained an accuracy of 98.24 (97.36–98.71), a F1-score of 98.56 (97.88–99.12) and an adjusted accuracy of 98.75 (98.35–99.38).
The proposed machine learning model can be immediately and effortlessly deployed to electrophysiology labs allowing cardiologists to predict the exact origins of arrhythmia and provide an optimum treatment plan both before and during the CA procedure. This approach will significantly reduce the CA procedure duration and the risk of complications.
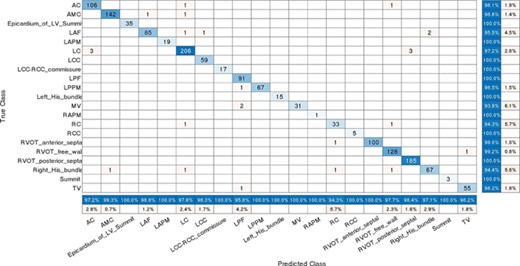
Confusion matrix for classification schemes
- anti-arrhythmia agents
- cardiac arrhythmia
- papillary muscle
- electrocardiogram
- cardiologists
- bundle of his
- radiofrequency catheter ablation
- mitral valve
- tricuspid valve
- endocardium
- left ventricle
- electrophysiology
- heart ventricle
- laboratory techniques and procedures
- natural sciences
- ventricular arrhythmia
- care plan
- epicardium
- laboratory test finding
- right ventricular outflow tract
- precision
- fluid flow
- machine learning