-
PDF
- Split View
-
Views
-
Cite
Cite
Francesca O'Boyle, Neeraj Mediratta, John Chalmers, Richard Warwick, Matthew Shaw, James McShane, Michael Poullis, Long-term survival of non-smokers undergoing coronary artery bypass surgery, European Journal of Cardio-Thoracic Surgery, Volume 45, Issue 3, March 2014, Pages 445–451, https://doi.org/10.1093/ejcts/ezt419
- Share Icon Share
Abstract
We sought to investigate long-term survival of non-smokers undergoing coronary artery bypass surgery (CABG).
A prospective database of consecutive patients was retrospectively analysed and cross correlated with the UK strategic tracking service to evaluate survival after primary CABG. Univariate, multivariate and a propensity analyses were performed.
We analysed 13 337 primary CABG procedures. Median follow-up was 7 years. Kaplan–Meier survival curves demonstrate that non-smokers have a significantly improved long-term survival compared with ex- and current smokers, P < 0.0001. Cox regression analysis identified smoking status, age, diabetes, ejection fraction (EF), body mass index, cerebrovascular disease, dialysis, left internal mammary artery (LIMA) non-usage, postoperative creatinine kinase muscle-brain isoenzyme (CKMB), radial artery usage, preoperative rhythm, forced vital capacity (FVC) and logistic EuroSCORE as significant risk factors determining long-term survival. Propensity matching resulted in 3575 non-smokers being matched 1:1, with ex-smokers. After matching, univariate analysis demonstrated the significantly worse long-term survival of ex-smokers compared with non-smokers, P < 0.0001. Cox regression analysis identified smoking status, age, postoperative CKMB, cerebrovascular disease, dialysis, diabetes, EF, FVC, LIMA non-usage, radial artery used, sinus rhythm and logistic EuroSCORE as significant risk factors determining long-term survival. Survival by smoking status plotted at the mean of the covariates, prepropensity matching, demonstrated that non-smokers had a significantly better long-term survival than ex-smokers, P < 0.0001; however, after propensity matching, non-smokers under 65 years of age had a significantly worse long-term survival compared with ex-smokers, P < 0.0001.
Non-smokers under the age of 65 years of age have significantly worse long-term survival compared with ex-smokers after risk factor adjustment. We speculate that this is because ex-smokers have had the causative factor, smoking, removed, but non-smokers have not.
INTRODUCTION
Smoking status is a risk factor for in-hospital morbidity, but not mortality [1, 2]. The detrimental impact of smoking status on long-term survival post-coronary artery bypass surgery (CABG) is widely accepted [3–6].
Smoking causes cardiovascular and respiratory compromises, which are associated with an increased risk of death post-surgery and reduced long-term survival.
We hypothesized that non-smokers have poorer long-term survival after CABG than ex-smokers. Non-smokers have an intrinsic risk factor for coronary/vascular disease, which does not alter after surgery, unlike ex-smokers whose main risk factor is usually smoking, but as they are ex-smokers this risk factor is no longer present. As non-smokers are less likely to have peripheral vascular disease, cerebrovascular disease or chronic obstructive airway disease, a propensity analysis to allow adjustment for these factors will be needed.
METHODS
Local institutional review board approval was granted for this study.
Database
A prospective single-institutional cardiac surgery database validated by the hospital data analysis department and accredited by the Society of Cardiothoracic Surgeons (SCTS) of Great Britain and Ireland was utilized in conjunction with the national strategic tracing service for long-term follow-up that exists in the UK, as has been described previously [7, 8].
All patients have their forced expiratory volume in 1 s (FEV1), and forced vital capacity (FVC) measured prior to surgery, by a single dedicated respiratory department.
The presence of peripheral vascular disease was based on clinical examination, or prior vascular assessment by ankle-brachial blood pressure index, computer tomography, magnetic resonance imaging, angiography, prior angioplasty/stenting or peripheral bypass surgery.
Smoking status was broken down into three categories: current smoker, ex-smoker and never smoker: current smoker is someone who currently smokes cigarettes regularly, ex-smoker is someone who used to smoke cigarettes regularly until 8 weeks prior to the date of surgery and non-smoker is someone who has never smoked cigarettes regularly [3].
Patients
Patients who had undergone isolated CABG only were included, n = 13 337, between 1 April 1997 and 31 March 2010.
Benchmarking
We benchmarked our in-hospital mortality figures against the UK national results (http://www.scts.org).
Methodology
The characteristics of the patients in this study are given in Table 1.
. | Data, n = 13 337 . |
---|---|
Preoperative | |
Age (years) | 64.911 (65.6), [58.9–71.6] |
Female, % (n) | 19.6 (2614) |
Body mass index (kg/m2) | 28.4, (27.9), [25.4–30.9] |
Heart failure, % (n) | 0.14 (19) |
Critical preoperative state, % (n) | 0.13 (17) |
Previous cerebral vascular accident, % (n) | 1 (133) |
Diabetes, % (n) | 36.7 (4895) |
Preoperative dialysis, % (n) | 0.4 (53) |
Previous myocardial infarction, % (n) | 48.9 (6522) |
Peripheral vascular disease, % (n) | 13.6 (1814) |
Hypertension, % (n) | 60.1 (802) |
Chronic obstructive pulmonary disease, % (n) | |
None | 79.5 (10 603) |
Mild | 7.7 (1027) |
Moderate | 10.9 (1454) |
Severe | 1.9 (253) |
Type of lung disease, % (n) | |
None | 85.0 (11 336) |
Obstructive | 13.2 (1757) |
Restrictive | 1.0 (133) |
Mixed | 0.8 (111) |
FEV1, % | 90, (93.0), [79.2–100] |
Preoperative IABP, % (n) | 1.3 (173) |
Ejection fraction | |
Good, % (n) | 59.7 (7962) |
Moderate, % (n) | 31.4 (4188) |
Poor, % (n) | 8.9 (1187) |
Previous PCI, % (n) | 6.4 (854) |
Status | |
Elective, % (n) | 80.5 (10 736) |
Urgent, % (n) | 18.2 (2428) |
Emergency, % (n) | 1.3 (173) |
Log EuroSCORE | 4.7, (2.6), [1.5–5.0] |
Operative | |
Left internal mammary artery, % (n) | 91.9 (12 257) |
Number of grafts | 3.4, (3.0), [3–4] |
Cardiopulmonary bypass time (min) | 109, (106), [88–127] |
Postoperative | |
Creatinine kinase myocardial isoenzyme | 30.3, (15), [4–34] |
Intensive care length of stay (days) | 2.2, (1), [1–2] |
Hospital length of stay (days) | 9.5 (7.0) [6–9] |
Mortality, % (n) | 2.1 (280) |
Median follow-up (years) | 7.02 |
. | Data, n = 13 337 . |
---|---|
Preoperative | |
Age (years) | 64.911 (65.6), [58.9–71.6] |
Female, % (n) | 19.6 (2614) |
Body mass index (kg/m2) | 28.4, (27.9), [25.4–30.9] |
Heart failure, % (n) | 0.14 (19) |
Critical preoperative state, % (n) | 0.13 (17) |
Previous cerebral vascular accident, % (n) | 1 (133) |
Diabetes, % (n) | 36.7 (4895) |
Preoperative dialysis, % (n) | 0.4 (53) |
Previous myocardial infarction, % (n) | 48.9 (6522) |
Peripheral vascular disease, % (n) | 13.6 (1814) |
Hypertension, % (n) | 60.1 (802) |
Chronic obstructive pulmonary disease, % (n) | |
None | 79.5 (10 603) |
Mild | 7.7 (1027) |
Moderate | 10.9 (1454) |
Severe | 1.9 (253) |
Type of lung disease, % (n) | |
None | 85.0 (11 336) |
Obstructive | 13.2 (1757) |
Restrictive | 1.0 (133) |
Mixed | 0.8 (111) |
FEV1, % | 90, (93.0), [79.2–100] |
Preoperative IABP, % (n) | 1.3 (173) |
Ejection fraction | |
Good, % (n) | 59.7 (7962) |
Moderate, % (n) | 31.4 (4188) |
Poor, % (n) | 8.9 (1187) |
Previous PCI, % (n) | 6.4 (854) |
Status | |
Elective, % (n) | 80.5 (10 736) |
Urgent, % (n) | 18.2 (2428) |
Emergency, % (n) | 1.3 (173) |
Log EuroSCORE | 4.7, (2.6), [1.5–5.0] |
Operative | |
Left internal mammary artery, % (n) | 91.9 (12 257) |
Number of grafts | 3.4, (3.0), [3–4] |
Cardiopulmonary bypass time (min) | 109, (106), [88–127] |
Postoperative | |
Creatinine kinase myocardial isoenzyme | 30.3, (15), [4–34] |
Intensive care length of stay (days) | 2.2, (1), [1–2] |
Hospital length of stay (days) | 9.5 (7.0) [6–9] |
Mortality, % (n) | 2.1 (280) |
Median follow-up (years) | 7.02 |
Continuous variables are shown as mean, (median) [25th–75th interquartile range]. Categorical variables are shown as a percentage (n).
IABP: intra-aortic balloon pump; PCI: percutaneous coronary intervention.
. | Data, n = 13 337 . |
---|---|
Preoperative | |
Age (years) | 64.911 (65.6), [58.9–71.6] |
Female, % (n) | 19.6 (2614) |
Body mass index (kg/m2) | 28.4, (27.9), [25.4–30.9] |
Heart failure, % (n) | 0.14 (19) |
Critical preoperative state, % (n) | 0.13 (17) |
Previous cerebral vascular accident, % (n) | 1 (133) |
Diabetes, % (n) | 36.7 (4895) |
Preoperative dialysis, % (n) | 0.4 (53) |
Previous myocardial infarction, % (n) | 48.9 (6522) |
Peripheral vascular disease, % (n) | 13.6 (1814) |
Hypertension, % (n) | 60.1 (802) |
Chronic obstructive pulmonary disease, % (n) | |
None | 79.5 (10 603) |
Mild | 7.7 (1027) |
Moderate | 10.9 (1454) |
Severe | 1.9 (253) |
Type of lung disease, % (n) | |
None | 85.0 (11 336) |
Obstructive | 13.2 (1757) |
Restrictive | 1.0 (133) |
Mixed | 0.8 (111) |
FEV1, % | 90, (93.0), [79.2–100] |
Preoperative IABP, % (n) | 1.3 (173) |
Ejection fraction | |
Good, % (n) | 59.7 (7962) |
Moderate, % (n) | 31.4 (4188) |
Poor, % (n) | 8.9 (1187) |
Previous PCI, % (n) | 6.4 (854) |
Status | |
Elective, % (n) | 80.5 (10 736) |
Urgent, % (n) | 18.2 (2428) |
Emergency, % (n) | 1.3 (173) |
Log EuroSCORE | 4.7, (2.6), [1.5–5.0] |
Operative | |
Left internal mammary artery, % (n) | 91.9 (12 257) |
Number of grafts | 3.4, (3.0), [3–4] |
Cardiopulmonary bypass time (min) | 109, (106), [88–127] |
Postoperative | |
Creatinine kinase myocardial isoenzyme | 30.3, (15), [4–34] |
Intensive care length of stay (days) | 2.2, (1), [1–2] |
Hospital length of stay (days) | 9.5 (7.0) [6–9] |
Mortality, % (n) | 2.1 (280) |
Median follow-up (years) | 7.02 |
. | Data, n = 13 337 . |
---|---|
Preoperative | |
Age (years) | 64.911 (65.6), [58.9–71.6] |
Female, % (n) | 19.6 (2614) |
Body mass index (kg/m2) | 28.4, (27.9), [25.4–30.9] |
Heart failure, % (n) | 0.14 (19) |
Critical preoperative state, % (n) | 0.13 (17) |
Previous cerebral vascular accident, % (n) | 1 (133) |
Diabetes, % (n) | 36.7 (4895) |
Preoperative dialysis, % (n) | 0.4 (53) |
Previous myocardial infarction, % (n) | 48.9 (6522) |
Peripheral vascular disease, % (n) | 13.6 (1814) |
Hypertension, % (n) | 60.1 (802) |
Chronic obstructive pulmonary disease, % (n) | |
None | 79.5 (10 603) |
Mild | 7.7 (1027) |
Moderate | 10.9 (1454) |
Severe | 1.9 (253) |
Type of lung disease, % (n) | |
None | 85.0 (11 336) |
Obstructive | 13.2 (1757) |
Restrictive | 1.0 (133) |
Mixed | 0.8 (111) |
FEV1, % | 90, (93.0), [79.2–100] |
Preoperative IABP, % (n) | 1.3 (173) |
Ejection fraction | |
Good, % (n) | 59.7 (7962) |
Moderate, % (n) | 31.4 (4188) |
Poor, % (n) | 8.9 (1187) |
Previous PCI, % (n) | 6.4 (854) |
Status | |
Elective, % (n) | 80.5 (10 736) |
Urgent, % (n) | 18.2 (2428) |
Emergency, % (n) | 1.3 (173) |
Log EuroSCORE | 4.7, (2.6), [1.5–5.0] |
Operative | |
Left internal mammary artery, % (n) | 91.9 (12 257) |
Number of grafts | 3.4, (3.0), [3–4] |
Cardiopulmonary bypass time (min) | 109, (106), [88–127] |
Postoperative | |
Creatinine kinase myocardial isoenzyme | 30.3, (15), [4–34] |
Intensive care length of stay (days) | 2.2, (1), [1–2] |
Hospital length of stay (days) | 9.5 (7.0) [6–9] |
Mortality, % (n) | 2.1 (280) |
Median follow-up (years) | 7.02 |
Continuous variables are shown as mean, (median) [25th–75th interquartile range]. Categorical variables are shown as a percentage (n).
IABP: intra-aortic balloon pump; PCI: percutaneous coronary intervention.
In-hospital mortality
Stepwise logistic regression was utilized to identify potential significant determining factors with regard to in-hospital mortality. The entry criterion was P < 0.05, and the removal criterion was P > 0.1 (Table 2).
Variable . | OR . | 95% CI (OR) . | P-value . |
---|---|---|---|
Non-smoker | 0.70 | 0.51–0.96 | 0.03 |
EuroSCORE | 1.05 | 1.04–1.06 | <0.0001 |
CKMB | 1.01 | 1.01–1.01 | <0.0001 |
Diabetes | 2.08 | 1.38–3.14 | 0.001 |
Age | 1.06 | 1.04–1.08 | <0.0001 |
Ejection fraction | |||
Moderate | 1.43 | 1.06–1.93 | 0.02 |
Poor | 1.97 | 1.33–2.92 | 0.001 |
Cerebral vascular disease | 0.63 | 0.41–0.98 | 0.04 |
Dialysis | 5.10 | 2.00–13.0 | 0.001 |
Forced vital capacity | 0.40 | 0.22–0.73 | 0.003 |
Variable . | OR . | 95% CI (OR) . | P-value . |
---|---|---|---|
Non-smoker | 0.70 | 0.51–0.96 | 0.03 |
EuroSCORE | 1.05 | 1.04–1.06 | <0.0001 |
CKMB | 1.01 | 1.01–1.01 | <0.0001 |
Diabetes | 2.08 | 1.38–3.14 | 0.001 |
Age | 1.06 | 1.04–1.08 | <0.0001 |
Ejection fraction | |||
Moderate | 1.43 | 1.06–1.93 | 0.02 |
Poor | 1.97 | 1.33–2.92 | 0.001 |
Cerebral vascular disease | 0.63 | 0.41–0.98 | 0.04 |
Dialysis | 5.10 | 2.00–13.0 | 0.001 |
Forced vital capacity | 0.40 | 0.22–0.73 | 0.003 |
CKMB: creatinine kinase muscle-brain isoenzyme.
Variable . | OR . | 95% CI (OR) . | P-value . |
---|---|---|---|
Non-smoker | 0.70 | 0.51–0.96 | 0.03 |
EuroSCORE | 1.05 | 1.04–1.06 | <0.0001 |
CKMB | 1.01 | 1.01–1.01 | <0.0001 |
Diabetes | 2.08 | 1.38–3.14 | 0.001 |
Age | 1.06 | 1.04–1.08 | <0.0001 |
Ejection fraction | |||
Moderate | 1.43 | 1.06–1.93 | 0.02 |
Poor | 1.97 | 1.33–2.92 | 0.001 |
Cerebral vascular disease | 0.63 | 0.41–0.98 | 0.04 |
Dialysis | 5.10 | 2.00–13.0 | 0.001 |
Forced vital capacity | 0.40 | 0.22–0.73 | 0.003 |
Variable . | OR . | 95% CI (OR) . | P-value . |
---|---|---|---|
Non-smoker | 0.70 | 0.51–0.96 | 0.03 |
EuroSCORE | 1.05 | 1.04–1.06 | <0.0001 |
CKMB | 1.01 | 1.01–1.01 | <0.0001 |
Diabetes | 2.08 | 1.38–3.14 | 0.001 |
Age | 1.06 | 1.04–1.08 | <0.0001 |
Ejection fraction | |||
Moderate | 1.43 | 1.06–1.93 | 0.02 |
Poor | 1.97 | 1.33–2.92 | 0.001 |
Cerebral vascular disease | 0.63 | 0.41–0.98 | 0.04 |
Dialysis | 5.10 | 2.00–13.0 | 0.001 |
Forced vital capacity | 0.40 | 0.22–0.73 | 0.003 |
CKMB: creatinine kinase muscle-brain isoenzyme.
Long-term survival
Univariate analysis
Kaplan–Meier survival curves were constructed, for patients classified as non-, ex- or current smokers (Fig. 1).
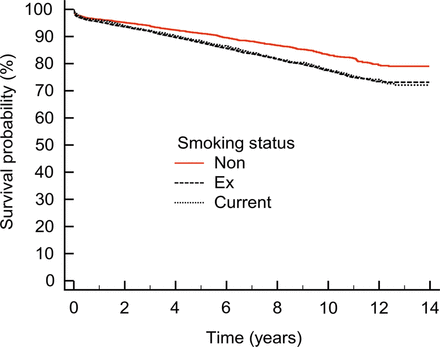
Kaplan–Meier survival curves for non-, ex-, and current smokers, P < 0.0001.
Multivariate analysis
Stepwise Cox proportional hazards regression analysis was utilized to identify potential significant determining factors with regard to long-term survival. The entry criterion was P < 0.05, and the removal criterion was P > 0.1 (Table 3). The effect of smoking status was plotted at the mean of the covariates (Fig. 2).
Covariate . | HR . | 95% CI (HR) . | P-value . |
---|---|---|---|
Study group | |||
Non-smoker | 0.74 | 0.66–0.83 | <0.0001 |
Smoker | 1.34 | 1.18–1.52 | <0.0001 |
Age | 1.06 | 1.05–1.07 | <0.0001 |
Diabetes oral | 1.47 | 1.30–1.67 | <0.0001 |
Diabetes insulin | 1.77 | 1.50–2.08 | <0.0001 |
Ejection fraction | |||
Moderate | 1.34 | 1.22–1.48 | <0.0001 |
Poor | 2.17 | 1.90–2.49 | <0.0001 |
Body mass index | 0.99 | 0.98–1.00 | 0.02 |
Cerebrovascular disease | 1.20 | 1.04–1.38 | 0.01 |
Dialysis | 5.81 | 3.95–8.54 | <0.0001 |
LIMA non-usage | 1.33 | 1.17–1.51 | <0.0001 |
EuroSCORE | 1.02 | 1.02–1.03 | <0.0001 |
CKMB | 1.00 | 1.00–1.00 | <0.0001 |
Radial usage | 0.88 | 0.79–0.98 | 0.02 |
Atrial fibrillation | 1.55 | 1.29–1.85 | <0.0001 |
Forced vital capacity | 0.45 | 0.36–0.55 | <0.0001 |
After propensity matching | |||
Non-smoker | 0.71 | 0.63–0.81 | <0.0001 |
Age | 1.07 | 1.06–1.08 | <0.0001 |
CKMB | 1.00 | 1.00–1.00 | <0.0001 |
Cerebrovascular disease | 1.26 | 1.03–1.54 | 0.03 |
Dialysis | 7.13 | 4.64–10.9 | <0.0001 |
Diabetes | 1.56 | 1.36–1.80 | <0.0001 |
Ejection fraction | |||
Moderate | 1.38 | 1.21–1.58 | <0.0001 |
Poor | 2.14 | 1.73–2.67 | <0.0001 |
EuroSCORE | 1.02 | 1.01–1.02 | 0.0002 |
Forced vital capacity | 0.47 | 0.35–0.64 | <0.0001 |
LIMA non-usage | 1.47 | 1.23–1.77 | <0.0001 |
Radial usage | 0.83 | 0.71–0.97 | 0.02 |
Atrial fibrillation | 1.49 | 1.15–1.94 | 0.003 |
Covariate . | HR . | 95% CI (HR) . | P-value . |
---|---|---|---|
Study group | |||
Non-smoker | 0.74 | 0.66–0.83 | <0.0001 |
Smoker | 1.34 | 1.18–1.52 | <0.0001 |
Age | 1.06 | 1.05–1.07 | <0.0001 |
Diabetes oral | 1.47 | 1.30–1.67 | <0.0001 |
Diabetes insulin | 1.77 | 1.50–2.08 | <0.0001 |
Ejection fraction | |||
Moderate | 1.34 | 1.22–1.48 | <0.0001 |
Poor | 2.17 | 1.90–2.49 | <0.0001 |
Body mass index | 0.99 | 0.98–1.00 | 0.02 |
Cerebrovascular disease | 1.20 | 1.04–1.38 | 0.01 |
Dialysis | 5.81 | 3.95–8.54 | <0.0001 |
LIMA non-usage | 1.33 | 1.17–1.51 | <0.0001 |
EuroSCORE | 1.02 | 1.02–1.03 | <0.0001 |
CKMB | 1.00 | 1.00–1.00 | <0.0001 |
Radial usage | 0.88 | 0.79–0.98 | 0.02 |
Atrial fibrillation | 1.55 | 1.29–1.85 | <0.0001 |
Forced vital capacity | 0.45 | 0.36–0.55 | <0.0001 |
After propensity matching | |||
Non-smoker | 0.71 | 0.63–0.81 | <0.0001 |
Age | 1.07 | 1.06–1.08 | <0.0001 |
CKMB | 1.00 | 1.00–1.00 | <0.0001 |
Cerebrovascular disease | 1.26 | 1.03–1.54 | 0.03 |
Dialysis | 7.13 | 4.64–10.9 | <0.0001 |
Diabetes | 1.56 | 1.36–1.80 | <0.0001 |
Ejection fraction | |||
Moderate | 1.38 | 1.21–1.58 | <0.0001 |
Poor | 2.14 | 1.73–2.67 | <0.0001 |
EuroSCORE | 1.02 | 1.01–1.02 | 0.0002 |
Forced vital capacity | 0.47 | 0.35–0.64 | <0.0001 |
LIMA non-usage | 1.47 | 1.23–1.77 | <0.0001 |
Radial usage | 0.83 | 0.71–0.97 | 0.02 |
Atrial fibrillation | 1.49 | 1.15–1.94 | 0.003 |
LIMA: left internal mammary artery; CKMB: creatinine kinase muscle-brain isoenzyme.
Covariate . | HR . | 95% CI (HR) . | P-value . |
---|---|---|---|
Study group | |||
Non-smoker | 0.74 | 0.66–0.83 | <0.0001 |
Smoker | 1.34 | 1.18–1.52 | <0.0001 |
Age | 1.06 | 1.05–1.07 | <0.0001 |
Diabetes oral | 1.47 | 1.30–1.67 | <0.0001 |
Diabetes insulin | 1.77 | 1.50–2.08 | <0.0001 |
Ejection fraction | |||
Moderate | 1.34 | 1.22–1.48 | <0.0001 |
Poor | 2.17 | 1.90–2.49 | <0.0001 |
Body mass index | 0.99 | 0.98–1.00 | 0.02 |
Cerebrovascular disease | 1.20 | 1.04–1.38 | 0.01 |
Dialysis | 5.81 | 3.95–8.54 | <0.0001 |
LIMA non-usage | 1.33 | 1.17–1.51 | <0.0001 |
EuroSCORE | 1.02 | 1.02–1.03 | <0.0001 |
CKMB | 1.00 | 1.00–1.00 | <0.0001 |
Radial usage | 0.88 | 0.79–0.98 | 0.02 |
Atrial fibrillation | 1.55 | 1.29–1.85 | <0.0001 |
Forced vital capacity | 0.45 | 0.36–0.55 | <0.0001 |
After propensity matching | |||
Non-smoker | 0.71 | 0.63–0.81 | <0.0001 |
Age | 1.07 | 1.06–1.08 | <0.0001 |
CKMB | 1.00 | 1.00–1.00 | <0.0001 |
Cerebrovascular disease | 1.26 | 1.03–1.54 | 0.03 |
Dialysis | 7.13 | 4.64–10.9 | <0.0001 |
Diabetes | 1.56 | 1.36–1.80 | <0.0001 |
Ejection fraction | |||
Moderate | 1.38 | 1.21–1.58 | <0.0001 |
Poor | 2.14 | 1.73–2.67 | <0.0001 |
EuroSCORE | 1.02 | 1.01–1.02 | 0.0002 |
Forced vital capacity | 0.47 | 0.35–0.64 | <0.0001 |
LIMA non-usage | 1.47 | 1.23–1.77 | <0.0001 |
Radial usage | 0.83 | 0.71–0.97 | 0.02 |
Atrial fibrillation | 1.49 | 1.15–1.94 | 0.003 |
Covariate . | HR . | 95% CI (HR) . | P-value . |
---|---|---|---|
Study group | |||
Non-smoker | 0.74 | 0.66–0.83 | <0.0001 |
Smoker | 1.34 | 1.18–1.52 | <0.0001 |
Age | 1.06 | 1.05–1.07 | <0.0001 |
Diabetes oral | 1.47 | 1.30–1.67 | <0.0001 |
Diabetes insulin | 1.77 | 1.50–2.08 | <0.0001 |
Ejection fraction | |||
Moderate | 1.34 | 1.22–1.48 | <0.0001 |
Poor | 2.17 | 1.90–2.49 | <0.0001 |
Body mass index | 0.99 | 0.98–1.00 | 0.02 |
Cerebrovascular disease | 1.20 | 1.04–1.38 | 0.01 |
Dialysis | 5.81 | 3.95–8.54 | <0.0001 |
LIMA non-usage | 1.33 | 1.17–1.51 | <0.0001 |
EuroSCORE | 1.02 | 1.02–1.03 | <0.0001 |
CKMB | 1.00 | 1.00–1.00 | <0.0001 |
Radial usage | 0.88 | 0.79–0.98 | 0.02 |
Atrial fibrillation | 1.55 | 1.29–1.85 | <0.0001 |
Forced vital capacity | 0.45 | 0.36–0.55 | <0.0001 |
After propensity matching | |||
Non-smoker | 0.71 | 0.63–0.81 | <0.0001 |
Age | 1.07 | 1.06–1.08 | <0.0001 |
CKMB | 1.00 | 1.00–1.00 | <0.0001 |
Cerebrovascular disease | 1.26 | 1.03–1.54 | 0.03 |
Dialysis | 7.13 | 4.64–10.9 | <0.0001 |
Diabetes | 1.56 | 1.36–1.80 | <0.0001 |
Ejection fraction | |||
Moderate | 1.38 | 1.21–1.58 | <0.0001 |
Poor | 2.14 | 1.73–2.67 | <0.0001 |
EuroSCORE | 1.02 | 1.01–1.02 | 0.0002 |
Forced vital capacity | 0.47 | 0.35–0.64 | <0.0001 |
LIMA non-usage | 1.47 | 1.23–1.77 | <0.0001 |
Radial usage | 0.83 | 0.71–0.97 | 0.02 |
Atrial fibrillation | 1.49 | 1.15–1.94 | 0.003 |
LIMA: left internal mammary artery; CKMB: creatinine kinase muscle-brain isoenzyme.
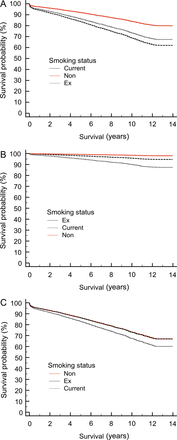
The effect of smoking status on long-term survival plotted at the mean of the covariates. (A) Whole study cohort, (B) patients <65 years old and (C) patients over 65 years old.
Propensity analysis
Logistic regression for group membership of smoking status was used to calculate the propensity score for 1:1 matching. Nearest neighbour matching without replacement with a caliper of 0.2 was utilized. Current smokers were excluded from analysis.
Variables used in the propensity match included: age, ejection fraction (EF), dialysis, EuroSCORE, postoperative creatinine kinase muscle-brain isoenzyme (CKMB), left internal mammary artery (LIMA) usage, radial artery usage, previous cerebrovascular disease, sinus rhythm, diabetes, preoperative FEV1 and FVC.
A dotplot of standardized mean differences (Cohen's d) for all covariates before and after matching was produced for patients who only received a statin. Kaplan–Meier and Cox survival curves post-propensity matching were created. Full propensity analysis data are supplied in Supplementary data.
Statistical software
All statistical analyses other than the propensity matching were performed with MedCalc for Windows (version 12.1.4, MedCalc Software, Mariakerke, Belgium). The propensity matching was performed with SPSS (version 20.0 for Windows, SPSS, Inc., Chicago, IL, USA), SPSS Statistics Integration Plug-In for R, and R 2.12.2.
RESULTS
100% long-term follow-up via the national strategic tracing service was achieved. Benchmarking of our institutional mortality rates compared with the UK did not reveal any differences (part of the continuous UK cardiac surgery quality assessment programme by the SCTS). The logistic EuroSCORE ranged from 0.881 to 80.411. The long-term follow-up was from 6 months to 12 years, mean 6.6 years and median 7 years.
In-hospital mortality
Logistic regression (ROC 0.83, Hosmer–Lemeshow 0.13), identified logistic EuroSCORE (odds ratio [OR] 1.05, 95% confidence interval [CI] 1.04–1.06), P < 0.0001, age (OR 1.06, 95% CI 1.04–1.08), P < 0.0001, dialysis (OR 5.1, 95% CI 2.0–13.0), P = 0.001, FVC (OR 0.40, 95% CI 0.22–0.73), P = 0.003, cerebrovascular disease (OR 0.64, 95% CI 0.41–0.98), P = 0.04, diabetes (OR 2.1, 95% CI 1.4–3.1), P = 0.001, moderate left ventricular EF (OR 1.4, 95% CI 1.1–1.9), P = 0.02, poor EF (OR 2.0, 95% CI 1.3–2.9), P = 0.001, CKMB release (OR 1.01, 95% CI 1.01–1.01), P < 0.0001 and non-smoker (OR 0.70, 95% CI 0.51–0.96), P = 0.03, as significant risk factors determining in-hospital mortality, Table 2. LIMA usage and FEV1 were excluded as significant risk factors.
Long-term survival
Univariate
Kaplan–Meier survival curves demonstrate that non-smokers have a significantly improved long-term survival compared with ex- and current smokers, P < 0.0001 (Fig. 1). With regard to survival, 433 events were recorded in non-smokers, 1331 events were recorded in ex-smokers and 332 events were recorded in current smokers.
Cox regression analysis
Study group
Cox regression analysis (c-index 0.75) identified smoking status, age, diabetes, EF, body mass index (BMI), cerebrovascular disease, dialysis, LIMA non-usage, postoperative CKMB, radial artery usage, preoperative rhythm, FVC and logistic EuroSCORE as significant risk factors determining long-term survival, Table 3 (study group). Female sex, hypertension, graft number, off-pump surgery and FEV1 were excluded by the model as being significant factors.
Cox regression analysis demonstrated that non-smokers had a hazard ratio (HR) <1, indicating that they have better survival than ex-smokers, as demonstrated when survival by smoking status was plotted at the mean of the covariates (Fig. 2A). When survival was broken down by age of surgery, a break point of 65 years old was found. Below 65 years of age non-smokers had significantly improved long-term survival compared with ex-smokers, Fig. 2B. However, above 65 years of age, non-smokers and ex-smokers had identical long-term survival (Fig. 2C).
Propensity-matched group
Propensity matching resulted in 3575 non-smokers being matched 1:1, with ex-smokers. Current smokers were excluded from propensity matching due to the methodological nature of matching. With regard to survival, 433 events were recorded in non-smokers (identical to prepropensity matching as 1:1 matching occurred), and 593 events were recorded in ex-smokers.
The mean logistic EuroSCORE before matching was 4.7 (25th–75th percentile 1.5–5.0) compared with a mean risk of 2.6 (25th–75th percentile of 1.4–4.8) post-matching, P = 0.01 (Mann–Whitney U-test—independent samples).
A dotplot of standardized mean differences (Cohen's d) for all covariates before and after matching for statin therapy is shown in Fig. 3, and overall χ2 balance test was not significant, χ2 (12) = 5.0, P = 0.96. Full details of the propensity match are enclosed in the Supplementary data.
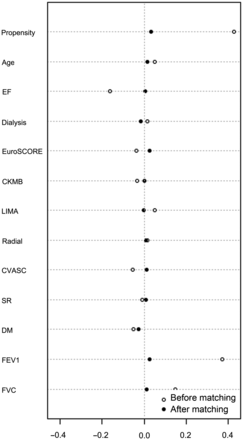
Dotplot of standardized mean differences (Cohen's d—x axis) for all covariates before and after matching for statin therapy. Overall χ2 balance test was not significant, χ2 (12) = 5.0, P = 0.96. EF: ejection fraction, CKMB: postoperative creatinine kinase muscle-brain isoenzyme, LIMA: left internal mammary artery, CVASC: previous cerebrovascular event, SR: sinus rhythm preoperatively, DM: diabetes (oral, medication or insulin controlled).
Univariate analysis demonstrated the significantly worse long-term survival of ex-smokers compared with non-smokers, Fig. 4A, P < 0.0001.
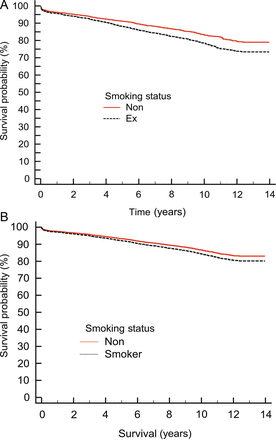
Post-propensity matching, n = 3575. (A) Kaplan–Meier survival curves for non- and ex-smokers, P < 0.0001 and (B) the effect of smoking status on long-term survival plotted at the mean of the covariates.
Cox regression analysis (c-index 0.73) identified smoking status, age, postoperative CKMB, cerebrovascular disease, dialysis, diabetes, EF, FVC, LIMA non-usage, radial artery used, sinus rhythm and logistic EuroSCORE as significant risk factors determining long-term survival, Table 3 (after propensity matching). FEV1 was excluded by the model as being significant factors.
Non-smokers had a HR of <1, indicating that they have better survival than ex-smokers. Survival post propensity matching identified smoking status when plotted at the mean of the covariates, had minimal clinical effect on survival, Fig. 4B. When survival was broken down by age group, patients <65 years old undergoing CABG had a significantly worse long-term survival, Fig. 5A, compared with those >65 years old, Fig. 5B.
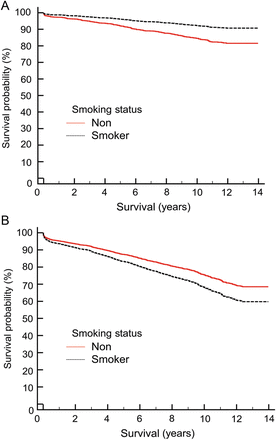
The effect of smoking status post-propensity matching on long-term survival plotted at the mean of the covariates. (A) Patients <65 years old and (B) patients >65 years old.
Two additional propensity matches were performed in patients below and above 65 years of age to eliminate any bias due to subgroup analysis. The same conclusions with regard to age, smoking status and long-term survival were found (data not shown).
DISCUSSION
Non-smokers as a group have significantly improved long-term survival compared with ex- and current smokers. However, after risk adjustment, non-smokers under 65 years of age actually have a worse long-term survival compared with ex-smokers. As neither of these groups now smoke, we postulate that the difference is secondary to the removal of the inciting risk factor, smoking, in the ex-smokers.
The explanation of the finding of an age cut-off of 65 years with regard to the effect of smoking status and survival is speculative. We postulate that non-smoking individuals that develop coronary artery disease prior to 65 years of age are genetically susceptible and are thus different to their smoking counterparts; however we provide no evidence to support this.
Smoking causes cardiovascular and respiratory diseases, which are associated with reduced long-term survival. Cessation of smoking removes the causative risk factor but the risk secondary to previous organ damage remains. After cessation of smoking, the long-term survival slowly reduces back to baseline. In non-smokers the underlying risk factor(s) if not hypertension, hypercholesterolaemia, thyroid dysfunction, renal failure or diabetes remain unchanged. The above risk factors are treated in the same manner regardless of smoking status. We hypothesized that non-smokers will have a worse long-term survival compared with ex-smokers as they have something genetically different in their constitution. The incidence of this ‘genetic difference’ will be the same as the general population in the ex-smoker cohort. We thus speculate that non-smokers are an enriched cohort of ‘bad genetic risk’ individuals.
COPD has been shown to be a significant factor determining long-term survival in patients with ischaemic heart disease patients and post-CABG [3, 9]. Numerous publications frequently do not include pulmonary disease as a potential risk factor for long term survival, or use qualitative criteria, such as clinical opinion. We recommend that FEV1, FVC and preferably if available pulmonary diffusion should be utilized to classify patients pulmonary disease by the Global Initiative for Chronic Obstructive Lung Disease criteria, and restrictive or obstructive [10]. Failure to quantify pulmonary dysfunction makes comparison between different publications difficult. Obstructive and restive lung diseases represent different pathological processes. Analysis of the restrictive and obstructive components separately identified that restrictive lung disease implies a worse long-term survival due to a higher HR on Cox analysis, confirming previous work [3].
Arterial grafting is beneficial to long-term survival [11–13]. The use of the internal mammary artery is known to be associated with increased survival post-CABG; however, it has not been included in a number of previous long-term prediction studies. Concerns about LIMA usage in patients who smoke with COPD exist due to the concerns about pulmonary dysfunction, but are unfounded [3]. Multivariate analysis revealed that LIMA usage is not a risk factor with regard to in-hospital mortality, but is a significant factor determining long-term survival regardless of pulmonary function. The number of patients with bilateral internal mammary arteries was too small in our dataset to analyse. We feel that our data demonstrating radial artery superiority with regard to long-term survival are subject to bias as this conduit is only used selectively in ‘fitter’ patients in our institution. This finding is supported by the observation that uncontrolled studies demonstrate a superiority of the radial artery, but randomized trails do not [14, 15].
Intraoperative myocardial damage is the main cause of cardiac dysfunction post-cardiac surgery, be it myocardial stunning, inadequate cardioplegia or coronary graft dysfunction. The finding that CKMB was a significant factor affecting long-term outcome demonstrates the importance of intraoperative myocardial protection and coronary anastomosis technique and has been previously identified as a significant factor effecting long-term survival [8, 16]. None of the previous studies of smoking status have adjusted for this intraoperative risk factor.
Debate continues with regard to the use of off-pump techniques. Multivariate analysis demonstrated that off-pump is not associated with a significant difference in hospital mortality, or long-term survival in our cohort. Identifying BMI and the absence of sinus rhythm as significant factors determining long-term survival confirms previous findings [17–19].
The value of multivariate analysis and propensity matching was demonstrated by the difference in outcomes by smoking status. Univariate analysis pre- and post-propensity matching demonstrated the superior survival of non-smokers; however, after risk factor adjustment (propensity matching), non-smokers under 65 years of age actually had a significantly worse long-term survival, a finding missed by Cox regression of the raw dataset. Performing a multivariate analysis after a propensity match is a previously described methodology [20]. The concept of survival plotted at the mean of the covariates, a well-described Cox output, is key to understanding the results of this manuscript [21].
Non-smokers anecdotally rarely start smoking after cardiac surgery; however ex-smokers frequently restart or have occasional cigarettes. As the ex-smoker group had better long-term survival than the non-smokers after risk factor adjustment, this potential confounding error will not have influenced our results.
Non-smokers under the age of 65 years have significantly worse long-term survival compared with ex-smokers after risk factor adjustment. We speculate that this is because ex-smokers have had the causative factor, smoking, removed, but non-smokers have not.
Limitations
We do not have cause of death of the patients, a frequent limitation of retrospective analysis; however, our series is large, prospective and consecutive and features 100% long-term follow-up. Very few patients received bilateral internal mammary grafts (BIMA), so this factor could not be analysed without introducing a statistical error to a lack of power. We are unable to account for patient selection bias, a limitation of all retrospective analyses. We made no attempt to breakdown the follow-up period into time intervals as have been done previously. The 14-year period that the patients in this study were recruited from may introduce a time factor error. This is a common confounding factor of any large study over a prolonged period. Cox modelling is a mathematical approximation; however, a c-index above 0.7, for both analyses, indicates that our model is reasonably accurate.
Smoking status was not recorded postoperatively; however, we speculate that non-smokers are unlikely to start smoking after undergoing CABG. In addition, the number of ex-smokers (defined as having stopped completely for a period of 6 weeks prior to surgery) who restart smoking after undergoing CABG is likely to be small.
SUPPLEMENTARY MATERIAL
Supplementary material is available at EJCTS online.
Funding
None.
Conflict of interest: none declared.