-
PDF
- Split View
-
Views
-
Cite
Cite
Michele Murzi, Alfredo G. Cerillo, Stefano Bevilacqua, Danyar Gilmanov, Pierandrea Farneti, Mattia Glauber, Traversing the learning curve in minimally invasive heart valve surgery: a cumulative analysis of an individual surgeon's experience with a right minithoracotomy approach for aortic valve replacement, European Journal of Cardio-Thoracic Surgery, Volume 41, Issue 6, June 2012, Pages 1242–1246, https://doi.org/10.1093/ejcts/ezr230
- Share Icon Share
Abstract
Cumulative sum (CUSUM) analysis, first developed to assess industrial quality control, was then used to monitor cardiac surgery performance more than 10 years ago. This analysis may be more sensitive than the standard statistical tools to analyse surgical results. The aim of this study is to assess a single surgeon's learning curve with right anterior minithoracotomy (RAMT) for aortic valve replacement (AVR) using risk-adjusted CUSUM curves and to compare the short- and medium-term results of these patients with a propensity-matched cohort of patients who had standard AVR (SAVR).
The first 100 patients who underwent RAMT by a single surgeon were analysed, using risk-adjusted CUSUM curves. Predicted risks of failure for individual patients were derived from our institutional database, using logistic regression modelling. Perioperative death or one or more of 10 adverse events constituted failure. Finally, RAMT patients were matched to 100 SAVR patients operated by the same surgeon in the same period, using a propensity score analysis.
The author's RAMT experience was associated with a low risk of cumulative failures from the outset, and no learning curve effect was observed. A cluster of surgical failure was individuated at the end of the CUSUM curve (between patients 90 and 100). The predicted risk of failure for the study population constantly increased over the time. After propensity score matching, no baseline differences were observed between RAMT and SAVR patients. The mortality rate was similar between groups (P = 0.8). However, the RAMT group had a lower need for mechanical-assisted ventilation (P = 0.02), transfusion requirements (P = 0.001), post-operative atrial fibrillation (P = 0.01) and post-operative intensive care unit and hospital stay (P = 0.001). Three-year survival was similar between groups (RAMT 94.5% vs. SAVR 92.8%).
AVR can be safely performed through an RAMT with results comparable with the standard sternotomy technique. Patients undergoing this technique are not exposed to an increased operative risk also during the surgeon's initial experience. CUSUM analysis is a valuable tool to assess the learning curve of new surgical techniques and to implement continuous performance monitoring.
INTRODUCTION
Since Benetti et al. in 1997 first described the right anterolateral minithoracotomy (RAMT) approach [1], some publications have demonstrated that the short-term results of this technique are at least as good as, if not better than, conventional aortic valve replacement (AVR) performed through the standard median sternotomy (SAVR) [2–4]. The theoretical advantages of this approach include the avoiding of sternotomy and thus a reduced pain and surgical trauma, less bleeding, earlier functional recovery, shorter hospital stay and reduced cost. Despite these potential benefits, many surgeons are still reluctant to perform AVR through a small right thoracotomy. The reasons for this surgical reluctance are multifactorial. It include the lack of established training programmes, the perception that success with the technique is limited to more proficient surgeons and a fear of deleterious patient outcomes, especially during the learning curve.
The purpose of the present study is therefore to evaluate the learning curve of a single cardiac surgeon who started to use RAMT for AVR, using cumulative sum (CUSUM) analysis, and to compare the results of these operations with those performed by the same surgeon through a median sternotomy in the same period. Specifically, we wished to evaluate whether patients who underwent RAMT during the learning curve were harmed or run a higher risk compared with patients who underwent SAVR.
MATERIAL AND METHODS
Patient selection
In 2006, after a period of observation and study, M.G. (our institutional lead consultant) decided to introduce the RAMT approach for AVR at our institution. In effort to assess his surgical learning curve prospectively, we decided to adopt a close performance monitoring using both the standard statistical and the CUSUM failure methods. For the purpose of the study, his first 100 patients who underwent this approach represent the population of this study. A control group of 100 patients who underwent SAVR by the same surgeon in the same period were likewise identified using a propensity score matching. All the data presented in the study were prospectively collected and entered in our institutional database, which includes 10 sections that are filled in consecutively by anaesthetists, surgeons, perfusionists, intensive care unit (ICU) and ward doctors. The study was approved by the clinical audit committee of the G. Pasquinucci Heart Hospital to meet ethical and legal requirements, and individual consent was waived.
Definitions of surgical failure
Perioperative death is rare after elective or urgent aortic valve operations (1.0% in our institution during the study period). It is therefore unsuitable for monitoring performance. We thus sought a more sensitive outcome, and in advance of any analyses, we defined surgical failure as the occurrence of one or more of the following events: (i) perioperative death (all deaths within 30 days of operation irrespective of where the death occurred and all hospital deaths after 30 days among patients who had not been discharged after the index operation); (ii) intraoperative conversion to sternotomy; (iii) perioperative myocardial infarction (new Q waves >0.04 ms and/or a reduction in R waves >25% in at least two contiguous leads on electrocardiogram); (iv) ventricular tachycardia or fibrillation; (v) post-operative complete atrioventricular block; (vi) neurological complication (permanent and transient strokes); (vii) acute renal failure (the requirement of haemodialysis or a post-operative elevated creatinine level (>2 mg/dl); (viii) reoperation for bleeding; (ix) mechanical ventilation requirement >48 h; (x) surgical wound infection.
Preoperative planning and surgical technique
Before surgery, all patients underwent imaging with 64-slice computed tomographic scanner (Toshiba Aquilon; Toshiba Medical System, Tokyo, Japan) without contrast enhancement to evaluate aortic calcifications as well as the relationship among the aortic valve, the sternum and the intercostal spaces. Image interpretation was performed from the reconstruction of axial and sagittal source images. Patients were suitable for RAMT if, at the level of main pulmonary artery, more than half of the ascending aorta was located on the right in respect to a vertical line drawn from the right sternal border to the ascending aorta and the distance from the ascending aorta to the sternum did not exceed 10 cm. Contraindication for RAMT are: previous right chest surgery, history of right pleural disease, ascending aorta dilatation and the need of concomitant procedures. Our surgical technique for RAMT has been reported previously [5–7]. Briefly, the surgical access is performed through a 5-cm right minithoracotomy in the second intercostal space. The main working port is created with the use of a soft tissue retractor and rib retractor. Venous cannulation is performed percutaneously with the Seldinger' technique under transoesophageal echocardiographic control through the right femoral vein. Consecutively, the ascending aorta is cannulated using a low-profile aortic cannula (EasyFlow™, San Ramon, CA, USA) and fixed in a traditional manner with two tourniquets. A left ventricular vent is placed in a conventional way. The aorta is cross-clamped directly through the thoracotomy with a flexible angulated cross-clamp (Novare Surgical Systems, Cupertino, CA, USA), antegrade single-shot crystalloid cardioplegia is administered and the patient is cooled to 34°C. When the heart is arrested, the aortic valve is exposed through an oblique/transverse aortotomy, the native valve is removed and the annulus decalcified. Consecutively, valve implantation is performed in supra-annular position using a non-everting technique with 2-0 Ethibond pledgeted sutures (Ethicon, Norderstedt, Germany).
In the SAVR group, aortic valve surgery was performed using a conventional median sternotomy with direct cannulation of the aorta and the right atrium. Myocardial protection was obtained with the direct aortic cross-clamp and combined antegrade and retrograde warm blood cardioplegia administration. The exposure of the valve, decalcification and prosthesis implantation technique are similar to those described earlier.
Post-operatively, no fast track methods or techniques were employed in either group. The time of discharge was determined by conventional surgical judgement and patient threshold for discharge.
Statistical analysis
The learning curve associated with RAMT was evaluated using a risk-adjusted cumulative failure chart. The statistical principles were adapted from the comprehensive tutorial by Rogers et al. [8]. CUSUM is defined as Sn = (Xi − p0i), where Xi = 0 for success and 1 for failure, and p0i denotes the predicted probability of failure for operation i. The graph starts at zero, but is incremented by 1 − p0i for a failure and decremented by p0i for a success. This graph is very intuitive because it moves upwards if the failure rate increases above that predicted by the risk model, moves downwards if the rate decreases and oscillates around zero if performance is consistent with predicted risks, which is acceptable. Published risk indices are mainly calibrated for surgical mortality. Risk adjustment models for specific post-operative morbidities and for a different combined end point have recently been published [9], but none of these were appropriate for the combined end point we defined. Therefore, predictors of failure were investigated empirically in the whole data set (626 patients who had isolated primary AVR from 2003 to 2010) by logistic regression modelling (Table 1). All variables listed in Table 2 were considered as possible predictors of failure, but interactions between these variables were not. Multiple regression models were fitted interactively, first by identifying significant predictors separately, second by including significant predictors in a multivariable model and finally by removing variables that did not reach statistical significance at P < 0.2.
Variable . | OR/HR . | 95% CI for OR . | P-value . | |
---|---|---|---|---|
. | . | Lower . | Upper . | . |
Long-term mortality | ||||
Age > 70 | 1.23 | 0.970 | 4.134 | 0.003 |
NYHA III or IV | 1.102 | 0.991 | 3.635 | 0.05 |
COPD | 1.478 | 1.018 | 4.658 | 0.03 |
Vascular disease | 1.332 | 1.143 | 5.562 | 0.03 |
Chronic kidney disease | 2.426 | 0.951 | 4.158 | 0.005 |
Variable . | OR/HR . | 95% CI for OR . | P-value . | |
---|---|---|---|---|
. | . | Lower . | Upper . | . |
Long-term mortality | ||||
Age > 70 | 1.23 | 0.970 | 4.134 | 0.003 |
NYHA III or IV | 1.102 | 0.991 | 3.635 | 0.05 |
COPD | 1.478 | 1.018 | 4.658 | 0.03 |
Vascular disease | 1.332 | 1.143 | 5.562 | 0.03 |
Chronic kidney disease | 2.426 | 0.951 | 4.158 | 0.005 |
OR: odds ratio; HR: hazard ratio; CI: confidence interval; NYHA: New York Heart Association; COPD: chronic obstructive lung disease. Model statistics: area under the receiver operating characteristic curve = 0.76; Hosmer–Lemeshow goodness-of-fit test, not significant for lack of fit P = 0.42.
Variable . | OR/HR . | 95% CI for OR . | P-value . | |
---|---|---|---|---|
. | . | Lower . | Upper . | . |
Long-term mortality | ||||
Age > 70 | 1.23 | 0.970 | 4.134 | 0.003 |
NYHA III or IV | 1.102 | 0.991 | 3.635 | 0.05 |
COPD | 1.478 | 1.018 | 4.658 | 0.03 |
Vascular disease | 1.332 | 1.143 | 5.562 | 0.03 |
Chronic kidney disease | 2.426 | 0.951 | 4.158 | 0.005 |
Variable . | OR/HR . | 95% CI for OR . | P-value . | |
---|---|---|---|---|
. | . | Lower . | Upper . | . |
Long-term mortality | ||||
Age > 70 | 1.23 | 0.970 | 4.134 | 0.003 |
NYHA III or IV | 1.102 | 0.991 | 3.635 | 0.05 |
COPD | 1.478 | 1.018 | 4.658 | 0.03 |
Vascular disease | 1.332 | 1.143 | 5.562 | 0.03 |
Chronic kidney disease | 2.426 | 0.951 | 4.158 | 0.005 |
OR: odds ratio; HR: hazard ratio; CI: confidence interval; NYHA: New York Heart Association; COPD: chronic obstructive lung disease. Model statistics: area under the receiver operating characteristic curve = 0.76; Hosmer–Lemeshow goodness-of-fit test, not significant for lack of fit P = 0.42.
Variable . | RAMT (100 patients) . | SAVR (100 patients) . | P-value . |
---|---|---|---|
Age (years) | 65.4 ± 9 | 64.6 ± 9 | 0.8 |
BMI | 27.8 ± 5.1 | 27.9 ± 4.8 | 0.8 |
Female gender | 70 | 72 | 0.9 |
NYHA functional class III or IV | 20 | 21 | 0.9 |
Diabetes mellitus | 16 | 18 | 0.7 |
Hypertension | 67 | 71 | 0.5 |
Dyslipidemia | 60 | 60 | 0.9 |
Cerebrovascular disease | 14 | 16 | 0.7 |
Vascular disease | 10 | 8 | 0.6 |
COPD | 14 | 20 | 0.2 |
History of AF | 20 | 14 | 0.2 |
Renal failure | 4 | 5 | 0.9 |
Dialysis | 2 | 1 | 0.5 |
Ejection fraction <30% | 6 | 5 | 0.7 |
Urgent operation | 7 | 11 | 0.3 |
Previous MI | 4 | 6 | 0.5 |
Previous PCI | 3 | 3 | 0.9 |
Logistic EuroSCORE | 4.6 (2–14) | 4.6 (2–18) | 0.9 |
Valve pathology | |||
Stenosis | 52 | 50 | |
Regurgitation | 24 | 21 | |
Mixed lesion | 24 | 29 | 0.7 |
Variable . | RAMT (100 patients) . | SAVR (100 patients) . | P-value . |
---|---|---|---|
Age (years) | 65.4 ± 9 | 64.6 ± 9 | 0.8 |
BMI | 27.8 ± 5.1 | 27.9 ± 4.8 | 0.8 |
Female gender | 70 | 72 | 0.9 |
NYHA functional class III or IV | 20 | 21 | 0.9 |
Diabetes mellitus | 16 | 18 | 0.7 |
Hypertension | 67 | 71 | 0.5 |
Dyslipidemia | 60 | 60 | 0.9 |
Cerebrovascular disease | 14 | 16 | 0.7 |
Vascular disease | 10 | 8 | 0.6 |
COPD | 14 | 20 | 0.2 |
History of AF | 20 | 14 | 0.2 |
Renal failure | 4 | 5 | 0.9 |
Dialysis | 2 | 1 | 0.5 |
Ejection fraction <30% | 6 | 5 | 0.7 |
Urgent operation | 7 | 11 | 0.3 |
Previous MI | 4 | 6 | 0.5 |
Previous PCI | 3 | 3 | 0.9 |
Logistic EuroSCORE | 4.6 (2–14) | 4.6 (2–18) | 0.9 |
Valve pathology | |||
Stenosis | 52 | 50 | |
Regurgitation | 24 | 21 | |
Mixed lesion | 24 | 29 | 0.7 |
BMI: body mass index; NYHA: New York Heart Association; COPD: chronic obstructive pulmonary disease; AF: atrial fibrillation; MI: myocardial infarction; PCI: percutaneous coronary intervention.
Variable . | RAMT (100 patients) . | SAVR (100 patients) . | P-value . |
---|---|---|---|
Age (years) | 65.4 ± 9 | 64.6 ± 9 | 0.8 |
BMI | 27.8 ± 5.1 | 27.9 ± 4.8 | 0.8 |
Female gender | 70 | 72 | 0.9 |
NYHA functional class III or IV | 20 | 21 | 0.9 |
Diabetes mellitus | 16 | 18 | 0.7 |
Hypertension | 67 | 71 | 0.5 |
Dyslipidemia | 60 | 60 | 0.9 |
Cerebrovascular disease | 14 | 16 | 0.7 |
Vascular disease | 10 | 8 | 0.6 |
COPD | 14 | 20 | 0.2 |
History of AF | 20 | 14 | 0.2 |
Renal failure | 4 | 5 | 0.9 |
Dialysis | 2 | 1 | 0.5 |
Ejection fraction <30% | 6 | 5 | 0.7 |
Urgent operation | 7 | 11 | 0.3 |
Previous MI | 4 | 6 | 0.5 |
Previous PCI | 3 | 3 | 0.9 |
Logistic EuroSCORE | 4.6 (2–14) | 4.6 (2–18) | 0.9 |
Valve pathology | |||
Stenosis | 52 | 50 | |
Regurgitation | 24 | 21 | |
Mixed lesion | 24 | 29 | 0.7 |
Variable . | RAMT (100 patients) . | SAVR (100 patients) . | P-value . |
---|---|---|---|
Age (years) | 65.4 ± 9 | 64.6 ± 9 | 0.8 |
BMI | 27.8 ± 5.1 | 27.9 ± 4.8 | 0.8 |
Female gender | 70 | 72 | 0.9 |
NYHA functional class III or IV | 20 | 21 | 0.9 |
Diabetes mellitus | 16 | 18 | 0.7 |
Hypertension | 67 | 71 | 0.5 |
Dyslipidemia | 60 | 60 | 0.9 |
Cerebrovascular disease | 14 | 16 | 0.7 |
Vascular disease | 10 | 8 | 0.6 |
COPD | 14 | 20 | 0.2 |
History of AF | 20 | 14 | 0.2 |
Renal failure | 4 | 5 | 0.9 |
Dialysis | 2 | 1 | 0.5 |
Ejection fraction <30% | 6 | 5 | 0.7 |
Urgent operation | 7 | 11 | 0.3 |
Previous MI | 4 | 6 | 0.5 |
Previous PCI | 3 | 3 | 0.9 |
Logistic EuroSCORE | 4.6 (2–14) | 4.6 (2–18) | 0.9 |
Valve pathology | |||
Stenosis | 52 | 50 | |
Regurgitation | 24 | 21 | |
Mixed lesion | 24 | 29 | 0.7 |
BMI: body mass index; NYHA: New York Heart Association; COPD: chronic obstructive pulmonary disease; AF: atrial fibrillation; MI: myocardial infarction; PCI: percutaneous coronary intervention.
Finally, we compared the results of RAMT patients with a control group of patients who underwent SAVR by the same surgeon. To reduce the effect of selection bias and potential confounding in this study, we developed a propensity score analysis [10]. The propensity for RAMT surgery was determined without regard to outcomes by the use of a non-parsimonious multiple logistic-regression analysis. All the variables listed in Table 2 were included in the analysis. Groups were also matched on the type (bioprosthetic or mechanical) of valve used to ensure that there was no bias introduced from the differing post-operative regimens for the valve types. Matching was carried out in one RAMT patient with one SAVR patient. Specifically, we matched each RAMT patient with one SAVR patient who had a five-digit propensity score. If this could not be done, we then proceeded to the next highest digit match (four, three, two and one digit) to make the best matches, in a hierarchical sequence until no more matches could be made.
All 100 RAMT patients were fully matched. Continuous data were expressed as the mean ± SD and categorical data as percentages. The Kolmogorov–Smirnov test was used to check for normality of data before further analysis. Differences between the groups were compared with the use of the Student's t-test for continuous variables and with the χ2 test or the Fisher's test for categorical variables. Non-parametric methods were used for variables that were not normally distributed. All reported P-values are two-sided, and P-values of 0.05 were considered to indicate statistical significance. All statistical analysis was performed with SPSS version 15.0 (SPSS Inc., Chicago, IL, USA).
RESULTS
Learning curve analysis and performance monitoring
The risk-adjusted CUSUM failure curve for the entire cohort is illustrated in Fig. 1. The CUSUM curve presents a small initial sharp slope that was quickly replaced by a negative downward inflection, indicating satisfactory results with respect to the predicted failure rates. Interestingly, we observed just three surgical failures in the first 40 patients, indicating the lack of any major learning curve effect. For the next 10 patients (patients 40–50 approximately), the plot display returns to run horizontally and two consecutive failures were observed at operations 55 and 56. Consecutively, the curve had a negative inflection from operations 65–85. Specifically, we observed four failure between operations 86 and 93. This cluster of failure prompted a review. We found that these patients were sick and fragile than the other as evidenced by the higher predicted probability of failure. Indeed, when observing the moving average of probability of failure (Fig. 2), a trend is evident, suggesting an increase in the predicted surgical risk of the patients over time. This trend was constant for all the study period, reflecting a more liberal patient selection process.
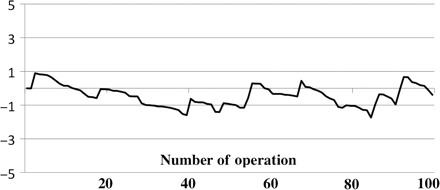
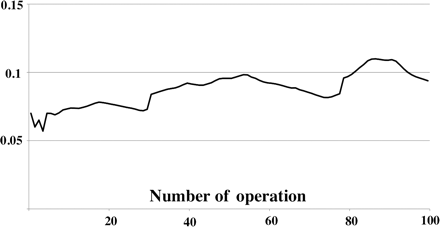
Graph reporting the moving average of predicted failure. The moving average was the average of predicted risks for previous three patients, current patient and next three patients.
Right anterior minithoracotomy vs. standard aortic valve replacement
After matching the 100 RAMT patients with an equivalent group of 100 SAVR patients, the preoperative risk factors were similar in the two groups (Table 2). The 30-day mortality and morbidity are listed in Table 3. There was only one death in the SAVR group. The RAMT group had a significantly lower need for post-operative mechanical-assisted ventilation (P = 0.02), blood transfusion requirements and were less likely to develop post-operative atrial fibrillation (P = 0.01). In addition, RAMT patients had a lower ICU stay (P = 0.01) and post-operative hospital stay (P = 0.001). However, cardiopulmonary bypass (P = 0.03) and aortic cross-clamp (P = 0.01) time were significantly higher in the RAMT groups.
Variable . | RAMT (100 patients) . | Sternotomy (100 patients) . | P-value . |
---|---|---|---|
Operative data | |||
CPB time | 119 ± 30 | 106 ± 32 | 0.03 |
Cross-clamp time | 83 ± 20 | 74 ± 28 | 0.01 |
Mechanical prosthesis | 8 | 8 | 0.9 |
Biological prosthesis | 92 | 92 | 0.9 |
Failures | |||
In-hospital mortality | 0 | 1 | 0.9 |
Reoperation for bleeding | 2 | 4 | 0.4 |
Perioperative MI | 1 | 1 | 0.9 |
Stroke | 1 | 1 | 0.9 |
TIA | 3 | 2 | 0.6 |
MAV > 48 h | 3 | 4 | 0.7 |
Renal complications | 3 | 2 | 0.6 |
Ventricular fibrillation | 1 | 0 | 0.9 |
Post-AVB | 0 | 1 | 0.9 |
Wound infection | 0 | 3 | 0.08 |
Reoperation for early prosthesis dysfunction | 0 | 1 | 0.9 |
Other complications | |||
Post-operative PMK insertion | 3 | 3 | 0.9 |
Post-operative AF | 24 | 41 | 0.01 |
Blood transfusions (packed red cell) | 0.7 ± 1.1 | 2 ± 3 | 0.001 |
Fresh Frozen Plasma | 1 ± 1.7 | 1 ± 1.4 | 0.8 |
MAV (h) | 6 (3–78) | 9 (3–86) | 0.02 |
ICU stay (days) | 1.2 (1–6) | 1.9 (1–10) | 0.05 |
Post-operative stay (days) | 5.4 (4–17) | 6.2 (4–22) | 0.001 |
Variable . | RAMT (100 patients) . | Sternotomy (100 patients) . | P-value . |
---|---|---|---|
Operative data | |||
CPB time | 119 ± 30 | 106 ± 32 | 0.03 |
Cross-clamp time | 83 ± 20 | 74 ± 28 | 0.01 |
Mechanical prosthesis | 8 | 8 | 0.9 |
Biological prosthesis | 92 | 92 | 0.9 |
Failures | |||
In-hospital mortality | 0 | 1 | 0.9 |
Reoperation for bleeding | 2 | 4 | 0.4 |
Perioperative MI | 1 | 1 | 0.9 |
Stroke | 1 | 1 | 0.9 |
TIA | 3 | 2 | 0.6 |
MAV > 48 h | 3 | 4 | 0.7 |
Renal complications | 3 | 2 | 0.6 |
Ventricular fibrillation | 1 | 0 | 0.9 |
Post-AVB | 0 | 1 | 0.9 |
Wound infection | 0 | 3 | 0.08 |
Reoperation for early prosthesis dysfunction | 0 | 1 | 0.9 |
Other complications | |||
Post-operative PMK insertion | 3 | 3 | 0.9 |
Post-operative AF | 24 | 41 | 0.01 |
Blood transfusions (packed red cell) | 0.7 ± 1.1 | 2 ± 3 | 0.001 |
Fresh Frozen Plasma | 1 ± 1.7 | 1 ± 1.4 | 0.8 |
MAV (h) | 6 (3–78) | 9 (3–86) | 0.02 |
ICU stay (days) | 1.2 (1–6) | 1.9 (1–10) | 0.05 |
Post-operative stay (days) | 5.4 (4–17) | 6.2 (4–22) | 0.001 |
CPB: cardiopulmonary bypass; MI: myocardial infarction; TIA: transient ischaemic attack; MAV: assisted mechanical ventilation; AVB: atrioventricular block; PMK: pacemaker; AF: atrial fibrillation; ICU: intensive care unit.
Variable . | RAMT (100 patients) . | Sternotomy (100 patients) . | P-value . |
---|---|---|---|
Operative data | |||
CPB time | 119 ± 30 | 106 ± 32 | 0.03 |
Cross-clamp time | 83 ± 20 | 74 ± 28 | 0.01 |
Mechanical prosthesis | 8 | 8 | 0.9 |
Biological prosthesis | 92 | 92 | 0.9 |
Failures | |||
In-hospital mortality | 0 | 1 | 0.9 |
Reoperation for bleeding | 2 | 4 | 0.4 |
Perioperative MI | 1 | 1 | 0.9 |
Stroke | 1 | 1 | 0.9 |
TIA | 3 | 2 | 0.6 |
MAV > 48 h | 3 | 4 | 0.7 |
Renal complications | 3 | 2 | 0.6 |
Ventricular fibrillation | 1 | 0 | 0.9 |
Post-AVB | 0 | 1 | 0.9 |
Wound infection | 0 | 3 | 0.08 |
Reoperation for early prosthesis dysfunction | 0 | 1 | 0.9 |
Other complications | |||
Post-operative PMK insertion | 3 | 3 | 0.9 |
Post-operative AF | 24 | 41 | 0.01 |
Blood transfusions (packed red cell) | 0.7 ± 1.1 | 2 ± 3 | 0.001 |
Fresh Frozen Plasma | 1 ± 1.7 | 1 ± 1.4 | 0.8 |
MAV (h) | 6 (3–78) | 9 (3–86) | 0.02 |
ICU stay (days) | 1.2 (1–6) | 1.9 (1–10) | 0.05 |
Post-operative stay (days) | 5.4 (4–17) | 6.2 (4–22) | 0.001 |
Variable . | RAMT (100 patients) . | Sternotomy (100 patients) . | P-value . |
---|---|---|---|
Operative data | |||
CPB time | 119 ± 30 | 106 ± 32 | 0.03 |
Cross-clamp time | 83 ± 20 | 74 ± 28 | 0.01 |
Mechanical prosthesis | 8 | 8 | 0.9 |
Biological prosthesis | 92 | 92 | 0.9 |
Failures | |||
In-hospital mortality | 0 | 1 | 0.9 |
Reoperation for bleeding | 2 | 4 | 0.4 |
Perioperative MI | 1 | 1 | 0.9 |
Stroke | 1 | 1 | 0.9 |
TIA | 3 | 2 | 0.6 |
MAV > 48 h | 3 | 4 | 0.7 |
Renal complications | 3 | 2 | 0.6 |
Ventricular fibrillation | 1 | 0 | 0.9 |
Post-AVB | 0 | 1 | 0.9 |
Wound infection | 0 | 3 | 0.08 |
Reoperation for early prosthesis dysfunction | 0 | 1 | 0.9 |
Other complications | |||
Post-operative PMK insertion | 3 | 3 | 0.9 |
Post-operative AF | 24 | 41 | 0.01 |
Blood transfusions (packed red cell) | 0.7 ± 1.1 | 2 ± 3 | 0.001 |
Fresh Frozen Plasma | 1 ± 1.7 | 1 ± 1.4 | 0.8 |
MAV (h) | 6 (3–78) | 9 (3–86) | 0.02 |
ICU stay (days) | 1.2 (1–6) | 1.9 (1–10) | 0.05 |
Post-operative stay (days) | 5.4 (4–17) | 6.2 (4–22) | 0.001 |
CPB: cardiopulmonary bypass; MI: myocardial infarction; TIA: transient ischaemic attack; MAV: assisted mechanical ventilation; AVB: atrioventricular block; PMK: pacemaker; AF: atrial fibrillation; ICU: intensive care unit.
Follow-up (100% complete) was similar between the two groups (RAMT 45 ± 1.6 vs. SAVR 43 ± 20 months; P = 0.5). The Kaplan–Meier curve analysis showed no difference among groups at 1- and 3-year survival (RAMT 99 and 94.5% vs. SAVR 98 and 92.8%). At the follow-up, just one SAVR patient required a reintervention on the aortic valve for prosthetic valve endocarditis.
DISCUSSION
The rapid evolution of less invasive heart valve surgery in recent years has resulted in its widespread popularization as an alternative to conventional AVR [1–4]. Cardiothoracic surgeons have therefore been confronted with a need to gain experience in these novel approaches to aortic valve surgery while maintaining high-quality results. When a novel technique is introduced, the surgeon finds himself/herself gaining proficiency and experience on suitable patients. Hence, the surgeon embarks on a ‘learning curve’, and unfortunately, his/her patients may possibly be at a higher risk. The traditional way of surgical results audit with the retrospective analysis of outcome data, and statistical testing is an appropriate method to assess the learning curve when the difference has reached a magnitude of statistical significance. However, when this is not the case, the CUSUM curve is more suitable for this kind of analysis. Indeed, CUSUM charts have the advantage of taking into account the importance of time as a ‘hidden variable’ in clinical studies while also avoiding the problem of repeated significance testing. de Leval et al. [11] pioneered the use of CUSUM analysis to monitor surgical performance in paediatric cardiac surgery. From that moment, several publications have used the CUSUM method to assess surgical results in cardiac surgery [12–15]. In this study, the CUSUM curve presented an initial negative shift demonstrating that the complication rate was below the accepted failure. It is extremely interesting to note that, apart from a very small upward inflection, no major learning curve effect was observed. These positive results may possibly reflect a careful patient selection at the beginning of the surgeon's experience. Subsequently, when the surgeon felt confident with this new technique, he started to consider also the sicker patients suitable for RAMT and this may have resulted in a small assessment of the curve, which begun to run horizontally. This is reflected also in the moving average of the probability of the failure graph (Fig. 2), which clearly shows the constant increase in the surgical risk profile of the study population over the time. Finally, from patients 90 to 100, the curve presented a short steep slope, indicating a cluster of surgical failure. This positive sloped curve in the final portion of the graph prompted a review of these patients, and we found that these patients were sicker and fragile than the other, as evidenced by a higher predicted risk of failure (Fig. 2). Our data here show that AVR through a small thoracotomy is safe and reproducible. Moreover, the patients having AVR using RAMT procedures had less need for post-operative mechanical-assisted ventilation, heamotransfusion requirement and had a lower rate of post-operative atrial fibrillation. We speculate that all these benefits may have resulted in a shorter ICU and post-operative hospital stay in comparison with patients undergoing conventional sternotomy. However, the aortic cross-clamp and cardiopulmonary bypass times were higher in the RAMT groups. This has been a consistent finding in most minimally invasive valve surgery studies [2, 3, 16, 17]. The increase in these times is modest and, to our knowledge, has not resulted in adverse consequences. Interestingly, also the medium-term results confirmed that RAMT can be performed, and results similar to those of SAVR can be obtained. The study has several limitations that are important to consider when interpreting the main findings. Propensity score analysis is simply a method for reducing bias in observational studies and the matching was limited by available variables. Therefore, it is possible that the way in which cases were selected for RAMT is unlikely to be adequately characterized by our data, despite the large number of clinical variables being available. Second, the first author started to perform RAMT after 3 years of experience with video-assisted mitral valve surgery performed through right thoracotomy. Third, the study compares only a single surgeon's patient outcomes. Finally, the primary outcome variable used represented the composite occurrence of mortality and/or any of 10 predefined major complications. Some of these complications are less consequential than others. In conclusion, new cardiac surgical procedures such as RAMT demand a close outcome analysis, especially during the initial phase of application, and the CUSUM technique, which alerts the surgical team to suboptimal results, represents the ideal statistical tool. Indeed, this method is sufficiently sensitive and simple. Moreover, our analysis confirmed previous studies remarking that RAMT can be performed safely, and early- and medium-term results comparable with those of SAVR can be obtained also during the surgeon's initial experience.
Conflict of interest: none declared.