-
PDF
- Split View
-
Views
-
Cite
Cite
Gamith Adasuriya, Andrey Barsky, Ines Kralj-Hans, Siddhartha Mohan, Simrat Gill, Zhong Chen, Julian Jarman, David Jones, Haseeb Valli, Georgios V Gkoutos, Vias Markides, Wajid Hussain, Tom Wong, Dipak Kotecha, Shouvik Haldar, REMOTE-AF and CASA-AF trial groups, the cardAIc team, and the BigData@Heart Consortium, Remote monitoring of atrial fibrillation recurrence using mHealth technology (REMOTE-AF), European Heart Journal - Digital Health, Volume 5, Issue 3, May 2024, Pages 344–355, https://doi.org/10.1093/ehjdh/ztae011
- Share Icon Share
Abstract
This proof-of-concept study sought to evaluate changes in heart rate (HR) obtained from a consumer wearable device and compare against implantable loop recorder (ILR)-detected recurrence of atrial fibrillation (AF) and atrial tachycardia (AT) after AF ablation.
REMOTE-AF (NCT05037136) was a prospectively designed sub-study of the CASA-AF randomized controlled trial (NCT04280042). Participants without a permanent pacemaker had an ILR implanted at their index ablation procedure for longstanding persistent AF. Heart rate and step count were continuously monitored using photoplethysmography (PPG) from a commercially available wrist-worn wearable. Photoplethysmography-recorded HR data were pre-processed with noise filtration and episodes at 1-min interval over 30 min of HR elevations (Z-score = 2) were compared with corresponding ILR data. Thirty-five patients were enrolled, with mean age 70.3 ± 6.8 years and median follow-up 10 months (interquartile range 8–12 months). Implantable loop recorder analysis revealed 17 out of 35 patients (49%) had recurrence of AF/AT. Compared with ILR recurrence, wearable-derived elevations in HR ≥ 110 beats per minute had a sensitivity of 95.3%, specificity 54.1%, positive predictive value (PPV) 15.8%, negative predictive value (NPV) 99.2%, and overall accuracy 57.4%. With PPG-recorded HR elevation spikes (non-exercise related), the sensitivity was 87.5%, specificity 62.2%, PPV 39.2%, NPV 92.3%, and overall accuracy 64.0% in the entire patient cohort. In the AF/AT recurrence only group, sensitivity was 87.6%, specificity 68.3%, PPV 53.6%, NPV 93.0%, and overall accuracy 75.0%.
Consumer wearable devices have the potential to contribute to arrhythmia detection after AF ablation.
ClinicalTrials.gov Identifier: NCT05037136 https://clinicaltrials.gov/ct2/show/NCT05037136
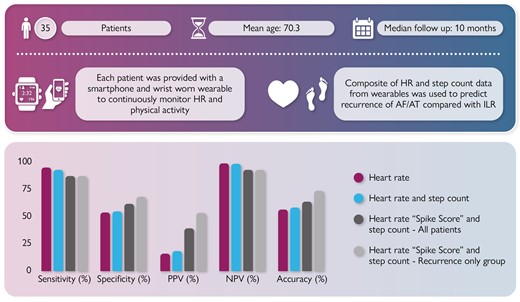
Aims, methods, and findings for REMOTE-AF looking at the detection of AF/AT recurrence using novel composite data from wearables compared with gold standard ILR.
AF, atrial fibrillation; AT, atrial tachycardia; HR, heart rate; ILR, implantable loop recorder; LSPAF, long-standing persistent atrial fibrillation; NPV, negative predictive value; PPV, positive predictive value.
Introduction
Atrial fibrillation (AF) is one of the leading causes of cardiovascular morbidity and mortality, increasing the risk of thromboembolism, heart failure, and confers a five times increased risk of stroke.1 Over 37 million people globally have a diagnosis of AF with its prevalence expected to double by 2060 leading to a significant economic burden.2 Given the anticipated increasing health burden of AF, it is a public heath priority to identify patients with AF and those with atrial arrhythmia recurrence post-intervention.3 The European Society of Cardiology (ESC) AF 2020 guidelines advocate for an integrated care model to be utilized in the diagnosis and management of AF.1 A key component of this model requires the use of digital technology and mobile health (mHealth) solutions, to support both health care professionals (HCPs) and patients in the comprehensive and personalized management of health conditions by offering a patient centred treatment model. This is achieved by empowering patients to engage in shared decision-making through patient education initiatives and encouraging positive lifestyle modifications.4
Modern and technologically advanced mHealth devices, specifically wearables, have emerged as a new-age, ubiquitous, and increasingly accurate solution to monitor cardiovascular biometrics.5 However, there is insufficient evidence to demonstrate their utility in current clinical practice. Many published studies lack quality control and are suboptimal in their design, exposing high risk for both selection and publication bias.6 Nonetheless, this shift from physician to patient-driven monitoring using data from personable wearable devices demonstrates potential and provides a unique opportunity to identify clinically significant arrhythmias in post-intervention patient cohorts. Many of these wearable devices utilize photoplethysmography (PPG) to monitor heart rate (HR) and accelerometers to assist with signal processing to filter noise artefact. Photoplethysmography leverages an optical technique to passively detect pulsatile volumetric changes in blood volume within the peripheral microcirculation to derive a waveform which correlates with the cardiac cycle.7,8 Hence, artificial intelligence (AI) systems have been programmed into wearable devices to accurately predict and detect atrial arrhythmias from PPG waveforms.9
In August 2020, the Heart Rhythm Society in conjunction with the European Heart Rhythm Association (EHRA) recognized the potential in the use of mHealth devices in cardiovascular disease evaluation and designated it as an important frontier in arrhythmia management.10 In addition, the ESC has led the way in incorporating the use of single-lead ECG diagnosis of AF from mHealth devices into their 2020 guidelines for diagnosis and management of AF.1 However, the National Institute of Clinical Excellence in the UK has not yet recommended the use of wearables in their recent AF diagnosis and management guideline (NG196) despite Public Health England sponsoring initiatives to promote the use of wearable technologies.11
A multitude of research studies have assessed the ability of PPG to screen for asymptomatic or index AF; however, there is a paucity of data identifying recurrence of AF post-rhythm control intervention. Our study sought to evaluate the correlation between a novel composite of wearable device recorded data with implantable loop recorder (ILR; Reveal LINQ™) detected AF/atrial tachycardia (AT) recurrence in a cohort of patients who had undergone an AF ablation procedure for long-standing persistent atrial fibrillation (LSPAF).
Methods
Study design
The REMOTE-AF study is a single arm, dual-centre, clinical study (NCT05037136) exploring the validity of PPG-recorded HR data being used in combination with accelerometer derived step count data to predict the recurrence of atrial arrythmias in a post-ablation patient population. We labelled this combination as non-exercise-related elevations in HR, defined as a step count < 500 over a continuous 30-min period. The literature does not define ‘non-exercise’ in relation to step count; however, a previous study accepted 100 steps per minute as ‘moderate intensity’ exercise and 2500 per day as a sedentary lifestyle.12 The study was approved by the UK NRES ethical review board (20/NI/0089). Participants were recruited from the Long-Term Outcomes in CASA-AF study (RfPB NIHR200595, ClinicalTrials.gov: NCT04280042).13 This was an extension of the CASA-AF trial, where 120 adults with symptomatic LSPAF, naïve to previous interventions, EHRA symptom score > 2, and left ventricular ejection fraction ≥40 were recruited between September 2015 and June 2018 and randomized to receive either radiofrequency catheter or thoracoscopic surgical ablation for LSPAF. At the index procedure, patients had an ILR (Reveal LINQ™) implanted which provided continuous cardiac monitoring with CARELINK remote monitoring to assess arrhythmia recurrence over an initial 12-month follow-up period which was subsequently extended to 36 months.14 The ILR had validated detection algorithm for AF/AT.15
Study participants
We remotely recruited patients from the Long-Term Outcomes in CASA-AF study over 2 months between August 2020 and September 2020 which was after the 12-month follow-up period reported in the original CASA-AF study (Figure 1). Patients from this cohort with functioning ILRs or devices with rhythm monitoring capabilities who met the inclusion criteria (Table 1) were asked if they were willing to participate. Each recruited participant was fitted with a wearable device (Fitbit Charge 2) and provided with a smartphone (Samsung A2) at least 21 months after their index procedure. The remote assessment of disease and relapse (RADAR-base) data collection software and Fitbit mobile application was pre-installed onto the smartphone to allow seamless integration with the Fitbit Charge 2 device sensors. The RADAR-base platform streamed data from the wearable, mobile application, and smartphone to a central location.16 This platform was installed on a virtual machine hosted on Amazon Web Services (AWS) in the AWS Europe (London) region, with infrastructure developed for the RATE-AF trial by the BigData@Heart consortium.17,18 Researchers were granted access to the Fitbit intraday developer application which allowed automated data collection for registered participants through the RADAR-base platform.
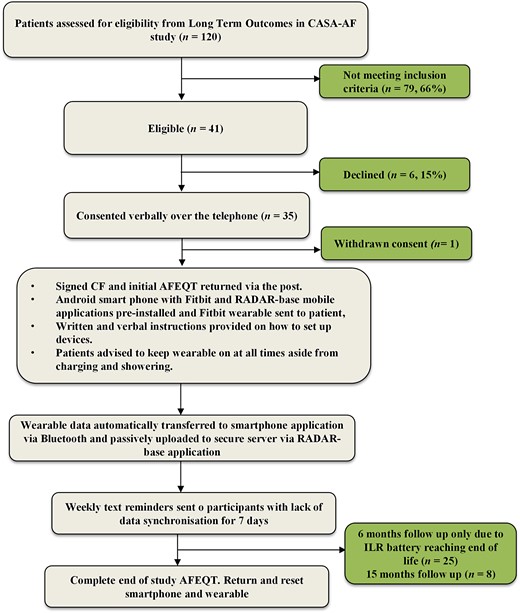
Schematic showing the stepwise process undertaken for patients recruited to REMOTE-AF. LoTO CASA LSAPF, long-term outcomes in long-standing persistent atrial fibrillation; PIS, patient information sheet; CF, consent form; AFEQT, AF quality of life questionnaire; RADAR, remote assessment of diseases and relapses.
Inclusion criteria . | Exclusion criteria . |
---|---|
|
|
Inclusion criteria . | Exclusion criteria . |
---|---|
|
|
Inclusion criteria . | Exclusion criteria . |
---|---|
|
|
Inclusion criteria . | Exclusion criteria . |
---|---|
|
|
The A MEMS three-axis accelerometer sensor enabled tracking of motion patterns and physical activity whilst the optical wrist-based PPG sensor allowed continuous tracking of HR. Participants were encouraged to wear the wearable continuously, removing only during charging and exposure to water, and to connect the Bluetooth-enabled smartphone to the internet at least once a day to allow synchronization of data with the RADAR-base servers. At weekly intervals, participants were reminded via text message to synchronize data if uploads had not occurred. Ad hoc virtual meetings allowed us to continually educate participants on their condition and encourage them to make positive lifestyle interventions in addition to assisting with troubleshooting of technical aspects. The focus of these meetings was primarily reinforcement of ongoing compliance with the wearable, to troubleshoot connectivity and data uploading problems and manage any other wearable-related issues. Participants were kept motivated by empathetic communication and the assurance of ongoing monitoring of their heart rhythm to meet the study objectives as well as timely assessment of heart rhythm disturbances for the duration of the study. In addition, we would take the opportunity presented at each meeting to engage participants in guideline-directed exercise regimes, maintain a healthy balanced mediterranean style diet, and ensure they understood the reasons for any medications they were taking to improve compliance. Participants were asked to report any adverse effects (skin irritation, health anxiety, or stress) related to the use of the wearable. Data were not collected beyond 31 January 2022.
Data analysis
One-minute averaged HR and step count data recorded by the wearable were transmitted to the smartphone mobile application via Bluetooth. Photoplethysmography-recorded HR data underwent signal processing and were filtered for motion artefact using two accelerometer sensors as part of a proprietary algorithm developed by Fitbit. The RADAR-base application was programmed to allow access to the Fitbit developer intraday application and subsequently passively collected data and synchronized directly to a secure, encrypted cloud-based web server. At the conclusion of the study, data were downloaded from the cloud server onto a local server for analysis. The data included HR and step count time series gathered from wearable devices and pooled at 1-min intervals. Mean HR data were calculated for each individual participant at 1-min interval over the duration of follow-up. We developed a method to detect sequences potentially suggestive of atrial arrhythmia recurrence for each patient at 30-min overlapping intervals, where HR was at least 2 SD (Z-score ≥ 2) above their mean for at least 20 min and later excluded those that were exercise related. We extracted all of the available tagged 30-min sequences for each patient (a total of 3208 sequences across our cohort) and associated within these sequences HR data with step data counterparts at the same time points. Sequences were kept only where HR and step count were both available for the duration of the 30-min window. Each of these sequences were then stratified into subcategories where corresponding step count values were denoted at 500 step intervals, with further subcategorization based on HR delta change. We used a simple yet reliable method to identify time points where a participant’s HR spiked. Across each sequence, we searched for the greatest increase in HR across a 2-min interval and recorded the size of that increase (in b.p.m.). We termed this novel composite the ‘spike score’. To compare across patients, we computed the normalized spike score (or spike Z-score), which is this figure divided by the standard deviation of the patient’s HR. We also computed the normalized downward spike score for each sequence, which is the largest 2-min decrease in a patient’s HR that occurred after the upward spike. Normalized spike scores ≥ 0.75 were taken to be indicators of sudden and significant HR elevation. Each data sequence within each subcategory was compared to ILR data to denote if recurrence of an atrial arrythmia had occurred.
For our analysis, a positive finding was recorded when our novel composite method for AF/AT recurrence correlated with ILR-detected electrogram (EGM) episodes showing arrhythmia recurrence. If a text only episode of AF/AT was recorded by the ILR, we sought a confirmatory EGM episode within a 24-h period. Implantable loop recorder-detected recurrences of atrial arrhythmia for at least 30 s were validated by a blinded senior cardiac physiologist as either AF/AT, sinus rhythm, or undetermined. Any events which were labelled as undetermined were sent to two electrophysiology consultants for final assessment. One patient with no ILR-detected AF/AT recurrence provided only 3 min of wearable device data and, therefore, was excluded from our analysis.
Study outcomes
Our primary endpoint is the correlation between a composite of mHealth-recorded HR (PPG) and physical activity as denoted by step count and AF/AT recurrence as detected by ILR. We analysed this in a stepwise manner:
PPG-recorded HR elevations (Z-score ≥ 2) correlating with AF/AT recurrence as detected by ILR.
PPG-recorded HR elevations (Z-score ≥ 2) combined with physical activity correlating with AF/AT recurrence as detected by ILR.
PPG-recorded HR elevations (Z-score ≥ 2) ≥110 beats per minute with normalized spike score ≥ 0.75 combined with physical activity correlating with AF/AT recurrence as detected by ILR.
Our secondary endpoint is the correlation between quality-of-life metrics as measured by the AF quality-of-life questionnaire (AFEQT) score and ILR-detected AF/AT recurrence.
Statistical analysis
The sensitivity, specificity, negative predictive value (NPV), positive predictive value (PPV), accuracy, and associated 95% confidence intervals for detection of correlated atrial arrhythmias in individual study participants were calculated using Microsoft Excel (Office 365, Microsoft Corporation, Redmond, Washington, USA) with a significance level of 0.05. Missing data from the wearable, defined as lack of HR and step count data for the entire 30-min duration of identified sequences using the method described above, were excluded from the extraction process and not included in final data analysis.
Results
Thirty-five patients in total were recruited. Thirty-three patients had ILRs in situ; two patients had dual chamber pacemakers instead of ILRs. Six eligible participants who met the inclusion criteria (Table 1) declined to take part. Mean [SD] age of recruited patients was 70.3 [±6.8] years with median 10 months [interquartile range 8–12] follow-up (Table 2). Twenty-five patients completed at least 6 months follow-up with 8 of these patients completing the full 15-month follow-up. In total, 236 871 hr of data were recorded via the wearable which amounts to a mean [SD] of 282 [±3.6] days per participant. On average, participants recorded data during 80.6% of day-time hours (8 a.m. and 8 p.m.) and 71.1% of night-time hours (8 p.m.–8 a.m.). Implantable loop recorder analysis showed 48.6% (17/35 patients) had recurrence of AF/AT. The average AF Quality of Life Questionnaire (AFEQT)19 score for patients in the non-AF/AT recurrence group was 48.9 at the beginning of the study and 32.3 at the end of the follow-up period, an improvement of 20% compared to the AF/AT recurrence group who had scores of 45.0 pre-study and 40.6 post-study.
Characteristics . | All (n = 35) . | Recurrence (n = 17) . | No recurrence (n = 18) . |
---|---|---|---|
Age (years), mean (SD) | 71 (6.8) | 71 (6.31) | 69 (7.2) |
≥75, n (%) | 10 (28.6) | 5 (29.4) | 5 (27.8) |
65–74 | 18 (51.4) | 7 (41.2) | 11 (61.1) |
55–64 | 6 (17.1) | 4 (23.5) | 2 (33.3) |
40–54 | 1 (2.9) | 1 (5.9) | 0 (0) |
Male sex, n (%) | 23 (65.7) | 9 (52.9) | 14 (77.8) |
BMI (kg/m2), median (IQR) | 30.4 (25.3–33.27) | 26.9 (25–33) | 30.9 (28.5–33.3) |
Ethnicity, n (%) | |||
White | 35 (100) | 17 (100) | 18 (100) |
Ejection fraction (%), mean (SD) | 56.9 (8.9) | 55.2 (8.9) | 58.8 (8.7) |
Left atrial diameter (mm), mean (SD) | 44.6 (5.9) | 44.6 (6) | 44.7 (5.8) |
Index procedure, n (%) | |||
Thoracoscopic surgical ablation | 16 (45.7) | 7 (41.2) | 9 (50) |
Radiofrequency catheter ablation | 19 (54.3) | 10 (58.8) | 9 (50) |
Time from index procedure (months), mean (SD) | 34 (7.8) | 33 (5.5) | 37 (6.5) |
AFEQT score, mean (SD) | 46 (21) | 44 (15) | 48 (25) |
Medical history, n (%) | |||
Hypertension | 13 (37.1) | 8 (47.1) | 5 (27.8) |
Diabetes | 3 (8.6) | 1 (5.9) | 2 (11.1) |
Coronary artery disease | 2 (5.7) | 1 (5.9) | 1 (5.6) |
Stroke, TIA, thromboembolism | 3 (8.6) | 2 (11.8) | 1 (5.6) |
Heart failure | 3 (8.6) | 2 (11.8) | 1 (5.6) |
CHA2DS2VASc score, n (%) | |||
0 | 2 (5.7) | 1 (5.9) | 1 (5.6) |
1 | 10 (28.6) | 5 (29.4) | 5 (27.8) |
2 | 11 (31.4) | 5 (29.4) | 6 (33.3) |
≥3 | 12 (34.3) | 6 (35.3) | 6 (33.3) |
HASBLED score, n (%) | |||
0 | 1 (2.9) | 0 (0) | 1 (5.6) |
1 | 9 (25.7) | 6 (35.3) | 3 (16.7) |
2 | 22 (62.9) | 10 (58.9) | 12 (66.7) |
≥3 | 3 (8.6) | 1 (5.9) | 2 (11.1) |
Characteristics . | All (n = 35) . | Recurrence (n = 17) . | No recurrence (n = 18) . |
---|---|---|---|
Age (years), mean (SD) | 71 (6.8) | 71 (6.31) | 69 (7.2) |
≥75, n (%) | 10 (28.6) | 5 (29.4) | 5 (27.8) |
65–74 | 18 (51.4) | 7 (41.2) | 11 (61.1) |
55–64 | 6 (17.1) | 4 (23.5) | 2 (33.3) |
40–54 | 1 (2.9) | 1 (5.9) | 0 (0) |
Male sex, n (%) | 23 (65.7) | 9 (52.9) | 14 (77.8) |
BMI (kg/m2), median (IQR) | 30.4 (25.3–33.27) | 26.9 (25–33) | 30.9 (28.5–33.3) |
Ethnicity, n (%) | |||
White | 35 (100) | 17 (100) | 18 (100) |
Ejection fraction (%), mean (SD) | 56.9 (8.9) | 55.2 (8.9) | 58.8 (8.7) |
Left atrial diameter (mm), mean (SD) | 44.6 (5.9) | 44.6 (6) | 44.7 (5.8) |
Index procedure, n (%) | |||
Thoracoscopic surgical ablation | 16 (45.7) | 7 (41.2) | 9 (50) |
Radiofrequency catheter ablation | 19 (54.3) | 10 (58.8) | 9 (50) |
Time from index procedure (months), mean (SD) | 34 (7.8) | 33 (5.5) | 37 (6.5) |
AFEQT score, mean (SD) | 46 (21) | 44 (15) | 48 (25) |
Medical history, n (%) | |||
Hypertension | 13 (37.1) | 8 (47.1) | 5 (27.8) |
Diabetes | 3 (8.6) | 1 (5.9) | 2 (11.1) |
Coronary artery disease | 2 (5.7) | 1 (5.9) | 1 (5.6) |
Stroke, TIA, thromboembolism | 3 (8.6) | 2 (11.8) | 1 (5.6) |
Heart failure | 3 (8.6) | 2 (11.8) | 1 (5.6) |
CHA2DS2VASc score, n (%) | |||
0 | 2 (5.7) | 1 (5.9) | 1 (5.6) |
1 | 10 (28.6) | 5 (29.4) | 5 (27.8) |
2 | 11 (31.4) | 5 (29.4) | 6 (33.3) |
≥3 | 12 (34.3) | 6 (35.3) | 6 (33.3) |
HASBLED score, n (%) | |||
0 | 1 (2.9) | 0 (0) | 1 (5.6) |
1 | 9 (25.7) | 6 (35.3) | 3 (16.7) |
2 | 22 (62.9) | 10 (58.9) | 12 (66.7) |
≥3 | 3 (8.6) | 1 (5.9) | 2 (11.1) |
AF, atrial fibrillation; AFEQT, AF quality of life questionnaire; BMI, body mass index; CHA2DS2VASc, congestive heart failure, high blood pressure, age 75, diabetes, previous stroke or clot, vascular disease, age 65–74, sex; HASBLED, hypertension; abnormal liver/renal function, stroke history, bleeding history or predisposition, labile INR (international normalized ratio), elderly, drug/alcohol usage; IQR, interquartile range; SD, standard deviation; TIA, transient ischaemic attack.
Characteristics . | All (n = 35) . | Recurrence (n = 17) . | No recurrence (n = 18) . |
---|---|---|---|
Age (years), mean (SD) | 71 (6.8) | 71 (6.31) | 69 (7.2) |
≥75, n (%) | 10 (28.6) | 5 (29.4) | 5 (27.8) |
65–74 | 18 (51.4) | 7 (41.2) | 11 (61.1) |
55–64 | 6 (17.1) | 4 (23.5) | 2 (33.3) |
40–54 | 1 (2.9) | 1 (5.9) | 0 (0) |
Male sex, n (%) | 23 (65.7) | 9 (52.9) | 14 (77.8) |
BMI (kg/m2), median (IQR) | 30.4 (25.3–33.27) | 26.9 (25–33) | 30.9 (28.5–33.3) |
Ethnicity, n (%) | |||
White | 35 (100) | 17 (100) | 18 (100) |
Ejection fraction (%), mean (SD) | 56.9 (8.9) | 55.2 (8.9) | 58.8 (8.7) |
Left atrial diameter (mm), mean (SD) | 44.6 (5.9) | 44.6 (6) | 44.7 (5.8) |
Index procedure, n (%) | |||
Thoracoscopic surgical ablation | 16 (45.7) | 7 (41.2) | 9 (50) |
Radiofrequency catheter ablation | 19 (54.3) | 10 (58.8) | 9 (50) |
Time from index procedure (months), mean (SD) | 34 (7.8) | 33 (5.5) | 37 (6.5) |
AFEQT score, mean (SD) | 46 (21) | 44 (15) | 48 (25) |
Medical history, n (%) | |||
Hypertension | 13 (37.1) | 8 (47.1) | 5 (27.8) |
Diabetes | 3 (8.6) | 1 (5.9) | 2 (11.1) |
Coronary artery disease | 2 (5.7) | 1 (5.9) | 1 (5.6) |
Stroke, TIA, thromboembolism | 3 (8.6) | 2 (11.8) | 1 (5.6) |
Heart failure | 3 (8.6) | 2 (11.8) | 1 (5.6) |
CHA2DS2VASc score, n (%) | |||
0 | 2 (5.7) | 1 (5.9) | 1 (5.6) |
1 | 10 (28.6) | 5 (29.4) | 5 (27.8) |
2 | 11 (31.4) | 5 (29.4) | 6 (33.3) |
≥3 | 12 (34.3) | 6 (35.3) | 6 (33.3) |
HASBLED score, n (%) | |||
0 | 1 (2.9) | 0 (0) | 1 (5.6) |
1 | 9 (25.7) | 6 (35.3) | 3 (16.7) |
2 | 22 (62.9) | 10 (58.9) | 12 (66.7) |
≥3 | 3 (8.6) | 1 (5.9) | 2 (11.1) |
Characteristics . | All (n = 35) . | Recurrence (n = 17) . | No recurrence (n = 18) . |
---|---|---|---|
Age (years), mean (SD) | 71 (6.8) | 71 (6.31) | 69 (7.2) |
≥75, n (%) | 10 (28.6) | 5 (29.4) | 5 (27.8) |
65–74 | 18 (51.4) | 7 (41.2) | 11 (61.1) |
55–64 | 6 (17.1) | 4 (23.5) | 2 (33.3) |
40–54 | 1 (2.9) | 1 (5.9) | 0 (0) |
Male sex, n (%) | 23 (65.7) | 9 (52.9) | 14 (77.8) |
BMI (kg/m2), median (IQR) | 30.4 (25.3–33.27) | 26.9 (25–33) | 30.9 (28.5–33.3) |
Ethnicity, n (%) | |||
White | 35 (100) | 17 (100) | 18 (100) |
Ejection fraction (%), mean (SD) | 56.9 (8.9) | 55.2 (8.9) | 58.8 (8.7) |
Left atrial diameter (mm), mean (SD) | 44.6 (5.9) | 44.6 (6) | 44.7 (5.8) |
Index procedure, n (%) | |||
Thoracoscopic surgical ablation | 16 (45.7) | 7 (41.2) | 9 (50) |
Radiofrequency catheter ablation | 19 (54.3) | 10 (58.8) | 9 (50) |
Time from index procedure (months), mean (SD) | 34 (7.8) | 33 (5.5) | 37 (6.5) |
AFEQT score, mean (SD) | 46 (21) | 44 (15) | 48 (25) |
Medical history, n (%) | |||
Hypertension | 13 (37.1) | 8 (47.1) | 5 (27.8) |
Diabetes | 3 (8.6) | 1 (5.9) | 2 (11.1) |
Coronary artery disease | 2 (5.7) | 1 (5.9) | 1 (5.6) |
Stroke, TIA, thromboembolism | 3 (8.6) | 2 (11.8) | 1 (5.6) |
Heart failure | 3 (8.6) | 2 (11.8) | 1 (5.6) |
CHA2DS2VASc score, n (%) | |||
0 | 2 (5.7) | 1 (5.9) | 1 (5.6) |
1 | 10 (28.6) | 5 (29.4) | 5 (27.8) |
2 | 11 (31.4) | 5 (29.4) | 6 (33.3) |
≥3 | 12 (34.3) | 6 (35.3) | 6 (33.3) |
HASBLED score, n (%) | |||
0 | 1 (2.9) | 0 (0) | 1 (5.6) |
1 | 9 (25.7) | 6 (35.3) | 3 (16.7) |
2 | 22 (62.9) | 10 (58.9) | 12 (66.7) |
≥3 | 3 (8.6) | 1 (5.9) | 2 (11.1) |
AF, atrial fibrillation; AFEQT, AF quality of life questionnaire; BMI, body mass index; CHA2DS2VASc, congestive heart failure, high blood pressure, age 75, diabetes, previous stroke or clot, vascular disease, age 65–74, sex; HASBLED, hypertension; abnormal liver/renal function, stroke history, bleeding history or predisposition, labile INR (international normalized ratio), elderly, drug/alcohol usage; IQR, interquartile range; SD, standard deviation; TIA, transient ischaemic attack.
Analysis of our data in a stepwise manner showed PPG-recorded HR correlating with AF/AT recurrence had a sensitivity of 95.3% (95% CI, 71–99); specificity 54.1% (95% CI, 50–59); PPV 15.8% (95% CI, 4–27); NPV 99.2% (95% CI, 99–100); and accuracy 57.4% (95% CI, 52–63) (Table 3). Combining PPG-recorded HR and step count with AF/AT recurrence yielded a sensitivity of 93.2% (95% CI, 68–99%); specificity 54.9% (95% CI, 49–60%); PPV 19.1% (95% CI, 6–18%); NPV 98.6% (95% CI, 97–99%); and accuracy 58.7% (95% CI, 52–65%) (Table 3) (Figures 2, 3, and 4). Photoplethysmography-recorded HR sequences identified by a normalized spike score of 0.75 or above over 2 min for both onset of HR ≥ 110 and cessation < 110 over the same time period produced a sensitivity of 87.5% (95% CI, 63–99%); specificity 62.2% (95% CI, 56–69%); PPV 39.2% (95% CI, 24–54%); NPV 92.3% (95% CI, 89–96%); and accuracy 64.0% (95% CI, 56–71%) (Table 3) (Figure 5). Focused assessment of the latter in the AF/AT recurrence group as defined by ILR led to a sensitivity of 87.6 (95% CI, 65–99%); specificity 68.3% (95% CI, 60–76%); PPV 53.6% (95% CI, 38–69%); NPV 93.0% (95% CI, 88–98%); and accuracy 74.0% (95% CI, 66–82%) (Table 3).
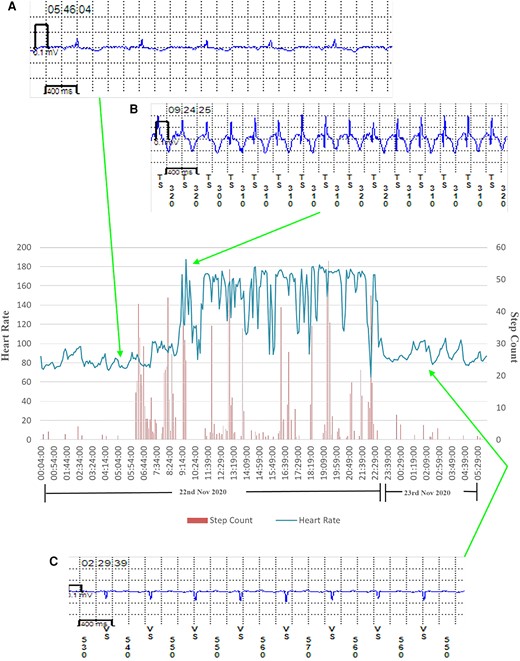
Transition from normal sinus rhythm to atrial tachycardia in a patient with atrial tachycardia recurrence. Heart rate and step count as recorded by a photoplethysmography and accelerometer-enabled wrist-worn device demonstrate a period of fixed maximum rate tachycardia and increased beat-to-beat variability, which correlates with implantable loop recorder-confirmed paroxysmal atrial tachycardia. (A) Implantable loop recorder electrogram showing normal sinus rhythm, (B) implantable loop recorder electrogram showing atrial tachycardia, and (C) implantable loop recorder electrogram showing cardioversion back to normal sinus rhythm.
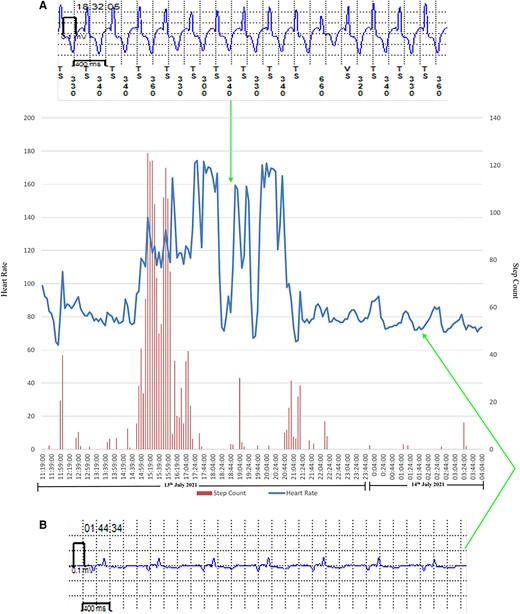
Transition from normal sinus rhythm to atrial fibrillation in a patient with atrial fibrillation recurrence. Heart rate and step count as recorded by a photoplethysmography and accelerometer-enabled wrist-worn device demonstrate a period of variable rate tachycardia and increased beat-to-beat variability, which correlates with implantable loop recorder-confirmed paroxysmal atrial fibrillation. (A) Implantable loop recorder electrogram showing atrial fibrillation and (B) implantable loop recorder electrogram showing normal sinus rhythm.
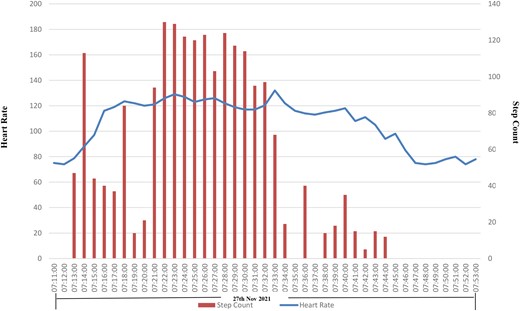
Demonstration of a gradual increase over time in photoplethysmography-recorded heart rate with an increase in accelerometer-derived step count suggestive of sinus tachycardia.
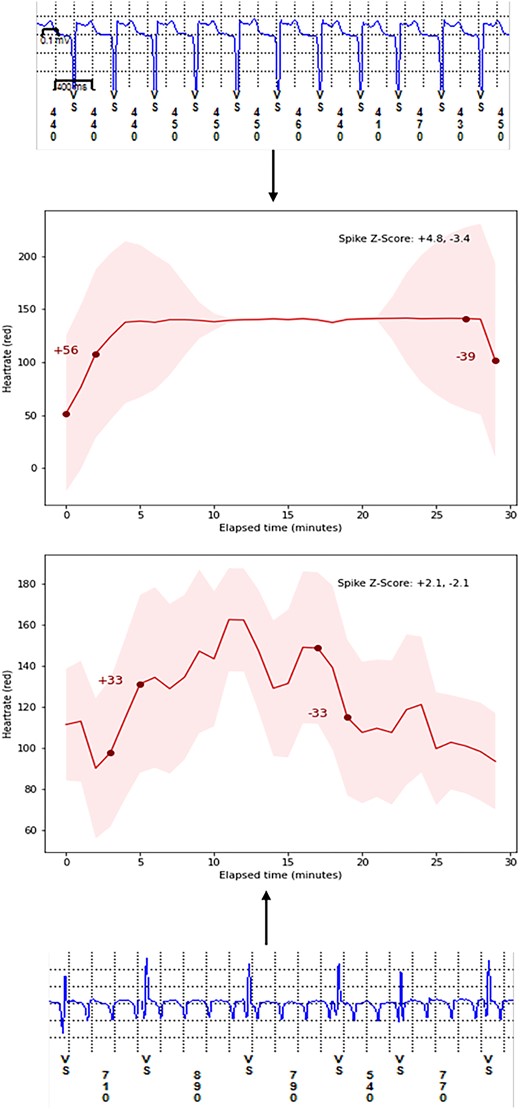
Demonstration of our underlying composite method to detect atrial fibrillation/atrial tachycardia recurrence by utilizing matching onset and offset for heart rate delta change (spike score) and subsequent arrhythmia recurrence as detected by implantable loop recorder. A normalized spike score ≥ 0.75 for both onset and offset of tachycardia is more likely to represent an episode of atrial arrhythmia recurrence due to a sudden increase in heart rate rather than exercise induced tachycardia with a gradual increase in heart rate. LoTO in CASA LSPAF, long-term outcomes in long-standing persistent atrial fibrillation RCT (ClinicalTrials.gov Identifier: NCT04280042). ILR, implantable loop recorder.
Results table showing sensitivity, specificity, positive predictive value, negative predictive value, and accuracy for each stepwise combination of wearable device data
. | Sensitivity (%) . | Specificity (%) . | PPV (%) . | NPV (%) . | Accuracy (%) . |
---|---|---|---|---|---|
Heart rate ≥ 110 beats per minute | 95.3 | 54.1 | 15.8 | 99.2 | 57.4 |
Heart rate ≥ 110 beats per minute and step count | 93.2 | 54.9 | 19.1 | 98.6 | 58.7 |
Heart rate spike score and step count | 87.5 | 62.2 | 39.2 | 92.3 | 64.0 |
HR spike score and step count in recurrence group | 87.6 | 68.3 | 53.6 | 93.0 | 74.0 |
. | Sensitivity (%) . | Specificity (%) . | PPV (%) . | NPV (%) . | Accuracy (%) . |
---|---|---|---|---|---|
Heart rate ≥ 110 beats per minute | 95.3 | 54.1 | 15.8 | 99.2 | 57.4 |
Heart rate ≥ 110 beats per minute and step count | 93.2 | 54.9 | 19.1 | 98.6 | 58.7 |
Heart rate spike score and step count | 87.5 | 62.2 | 39.2 | 92.3 | 64.0 |
HR spike score and step count in recurrence group | 87.6 | 68.3 | 53.6 | 93.0 | 74.0 |
PPV, positive predictive value; NPV, negative predictive value.
Results table showing sensitivity, specificity, positive predictive value, negative predictive value, and accuracy for each stepwise combination of wearable device data
. | Sensitivity (%) . | Specificity (%) . | PPV (%) . | NPV (%) . | Accuracy (%) . |
---|---|---|---|---|---|
Heart rate ≥ 110 beats per minute | 95.3 | 54.1 | 15.8 | 99.2 | 57.4 |
Heart rate ≥ 110 beats per minute and step count | 93.2 | 54.9 | 19.1 | 98.6 | 58.7 |
Heart rate spike score and step count | 87.5 | 62.2 | 39.2 | 92.3 | 64.0 |
HR spike score and step count in recurrence group | 87.6 | 68.3 | 53.6 | 93.0 | 74.0 |
. | Sensitivity (%) . | Specificity (%) . | PPV (%) . | NPV (%) . | Accuracy (%) . |
---|---|---|---|---|---|
Heart rate ≥ 110 beats per minute | 95.3 | 54.1 | 15.8 | 99.2 | 57.4 |
Heart rate ≥ 110 beats per minute and step count | 93.2 | 54.9 | 19.1 | 98.6 | 58.7 |
Heart rate spike score and step count | 87.5 | 62.2 | 39.2 | 92.3 | 64.0 |
HR spike score and step count in recurrence group | 87.6 | 68.3 | 53.6 | 93.0 | 74.0 |
PPV, positive predictive value; NPV, negative predictive value.
Virtual meetings with each participant occurred at monthly intervals for the entirety of the study with an overall average of 10 meetings per participant at a duration of 15 min per meeting. Compliance with these sessions were recorded at 100%. No adverse events such as skin irritation from wearable devices, health anxiety, or stress were reported by any of the 35 participants. Three wearable devices required strap replacement and resulted in nine follow-up days lost.
Discussion
REMOTE-AF evaluated the correlation between wearable device-derived HR and AF/AT recurrence. Current physician prescribed non-continuous rhythm monitoring tools (ambulatory Holter monitors) which assess for arrhythmias at set time intervals are known to have low detection yields.20 In symptomatic patients with suspected arrhythmias, a 24-h ambulatory Holter monitor has a diagnostic yield of 7% compared with 47% with a 7-day monitor.21 At present, the gold standard for detection of atrial arrhythmias is continuous monitoring via the ILR. mHealth wearable devices can bridge the gap between intermittent time-limited Holter monitoring and invasive and costly ILR monitoring.
To analyse our data, we adopted a stepwise approach to sequentially combine parameters from the wearable device. We saw an increase in PPV from 15.8 to 39.2% and improvement in accuracy from 57.4 to 64.0% when using our novel composite HR spike score method compared to using PPG-recorded HR alone to detect AF/AT recurrence. This is because the spike score is more likely to represent true arrhythmia rather than physiological HR increase and hence more accurately differentiate between sinus tachycardia and pathological recurrences of AF/AT. In patients with known AF/AT recurrence as detected by ILR, our novel composite methodology utilizing spike score resulted in the highest accuracy in identifying AF/AT recurrence in a post-ablation cohort when compared with ILR. Irrespective of sequential improvements in accuracy, using this method results in 5 of 10 episodes being misclassified as AF/AT. Further work with larger patient populations is needed to improve the diagnostic accuracy of our method. Our results yielded a high NPV and low PPV, likely due to our small sample size with low prevalence of AF/AT recurrence. In clinical practice, this supports the use of wearables in high-risk patient populations with known diagnoses of atrial arrhythmias rather than for use in screening in low-risk patient populations (global AF prevalence of 0.51%).2 Our results also show that our novel composite method in its current form, appropriately classifies non-recurrence of AF/AT but occasionally misclassifies recurrence. Within the current consumer market, the diversity in the accuracy and quality of PPG embedded in wearable devices limits the potential of integrating these data safely into the clinician’s decision-making process.5,22 Extensive research and funding has been dedicated to assessing the accuracy of PPG-enabled wearables in detecting atrial arrhythmias, predominantly AF screening but there remains a lack of data in post-AF intervention populations. Products developed by multi-billion-dollar global technology giants have shown promise that wearable devices could become a viable alternative in diagnosing and monitoring arrhythmias. The Apple Watch (Apple Inc, Cupertino, CA, USA), Fitbit (Google LLC, Mountain View, CA, USA), and Huawei Smartwatch (Huawei Technologies Co Ltd, Shenzhen, China) have been assessed in non-randomized but large-scale prospective clinical trials, reporting accuracy of 95–97% in detecting AF and modest PPVs.23–25
Our statistical analysis revealed a PPV significantly lower than figures reported by these landmark PPG-detected arrhythmia studies; however, these large-scale studies were based on AF screening and not recurrence as in our cohort. Based on a recent meta-analysis, these figures are likely to be unrealistically elevated; therefore, our results are likely to be more representative of the accuracy of PPG in detecting AF/AT recurrence.6 Given that there is limited published data, predominantly case reports26–29 documenting wearable use in rhythm monitoring after catheter ablation, we are unable to make a direct comparison of PPG-enabled wearables in this patient cohort. The reported clinical metric in the landmark studies only analysed PPG HR waveforms intermittently whilst stationary, with the majority at night. We reported results for all data both during active day-time hours and whilst ambulatory, where clinically significant arrhythmias are more likely. Overall, our data provide greater real-world analysis and highlight the issue of lower diagnostic yields and challenge related to sensitivity and specificity of data from wearable devices during periods of activity. Conducting this study during a global pandemic also produced its own challenges but further advanced the necessity and accessibility for remote monitoring strategies, not just for western populations but worldwide, to ensure health equity.
Recurrence of paroxysmal and persistent atrial arrhythmias which are rate controlled are likely to be misclassified as non-recurrence by our novel composite method. However, our NPV consistently being above 90% for all analysed groups shows that this had a minimal effect on our results. Our secondary endpoint showed that in patients with recurrence of atrial arrhythmia, patients described a poorer quality of life as evidenced by a higher AFEQT score when compared with the non-recurrence group. Consequently, our results demonstrate promise in identifying atrial arrythmia recurrence by combining PPG-recorded, non-exercise related elevations in HR with quality-of-life data. As a result, there is potential to avoid the invasive nature of ILR insertion as well as labour and cost intensive follow-up to achieve long-term cost-effective monitoring. With further improvement in diagnostic accuracy, this could lead to an electronic point-of-care notification being sent via a smart device to assist pill in the pocket arrhythmia and anticoagulation management strategies as well as timely interventions (direct current cardioversion or redo catheter ablation) in patients experiencing recurrence of atrial arrythmias.30,31 Lastly, due to our recruitment resulting in a homogeneous population, more heterogeneity with regard to ethnicity and gender is required to draw more specific conclusions.
To our knowledge, this study is the first to utilize a novel PPG-derived HR spike score methodology to detect AF/AT recurrence using gold-standard ILR technology as a comparator. The ILR (Reveal LINQ™) used in this study was found to have an AF detection sensitivity for episodes ≥2 min of 97.4%; specificity 97.0%; PPV 92.5%; and NPV 99.0% and was able to detect AF burden with a sensitivity of 98.4%.15 Therefore, the ILR serves as an excellent comparator.
The use of mHealth wearables to detect atrial arrhythmia recurrence is gaining traction and to our knowledge REMOTE-AF is one of the two studies, to report results for atrial arrythmia recurrence in patients with a known history of AF, utilizing PPG-enabled wearable technology. The other study utilized a smart ring to detect atrial arrythmia recurrence in a patient cohort post direct current cardioversion (DCCV).32 Currently active is the SMART-ALERT clinical trial which will also use the ILR as a comparator for PPG-detected AF from a wearable device, both a smartwatch and smart ring. Data from this study can be used to further support or refute the use of PPG-based wearables to detect atrial arrhythmias.33
Another study in progress is the SAFER (ISRCTN72104369)34 trial which will evaluate evidence to support the tolerability and feasibility of screening patients >65 years old using PPG-enabled wearables. Our REMOTE-AF cohort with a mean age of 70.3 years and median follow-up at 10 months has already clearly demonstrated acceptability and tolerability in an older patient cohort. We recorded good compliance with device use and a roughly equal proportion of use during day-time and night-time hours. Alongside results reported by the eBRAVE-AF trial (NCT04250220),31 which screened for AF using smartphone PPG sensors, lead to greater confidence that digital wearable technology can safely be used for not just screening but monitoring of known arrhythmia patients in the cohort at greatest risk of AF and its complications. Limited economic analyses demonstrate affordability and cost-savings associated with AF detection using wearables, specifically in over 65 s, further highlighting their potential to improve health outcomes as measured by quality-adjusted life year by enhancing primary and primordial prevention.35,36
Incorporating heart rate variability (HRV) into our novel composite method would likely have further improved our PPV as significant changes to HRV have been shown to correlate with AF.37 The Fitbit application programming interface integrating HRV data was not open to researchers at the commencement of the study; therefore, REMOTE-AF study team did not have access to this data.
Future work requires more extensive investigation of PPG-enabled wearable devices and arrhythmia detection tools in real-world clinical settings with the design of larger trials including randomized clinical studies to report major adverse cardiovascular event outcomes. A reduction in time to detection of AF/AT recurrence would allow for more responsive implementation of treatment strategies and lifestyle modifications to reduce disease burden and improve quality of life. Consumer mHealth devices can also act to further health literacy by facilitating patient understanding of disease progression and encourage positive behaviour change to modify their risk profile. With the ESC AF 2020 guidelines advocating an integrated care model for patients with arrythmias, the use of remote monitoring technologies and integrated smartphone applications can assist clinicians in fulfilling this aim by providing real-time patient monitoring outside traditional healthcare environments, facilitate patient education and provide encouragement to empower patients as they are able to track their own health in real time and thus provide a visual stimulus to engage in positive behaviours to improve their underlying cardiovascular status. As PPG technology continues to evolve and used in combination with integrated single-lead ECG, we can cautiously begin to consider this technology as an adjunct to improve arrhythmia outcomes and guide treatment decisions.
We believe this proof-of-concept study can provide a foundation for further research to develop our method in conjunction with sophisticated algorithmic developmental tools to detect atrial arrhythmias with precision.
Limitations
PPG technology itself has many limitations with the most prominent being motion artefact and noise interference, which can disrupt arrhythmia detection algorithms and signal quality, affecting the accuracy of HR measurements.7,38 The devices used in the study were 2 years old with manufacturer-recommended battery life of 4–5 days; however, due to the lithium polymer battery life, gradual deterioration resulted in patients’ charging devices every 2–3 days which inevitably resulted in reduced compliance and more frequent interruptions to monitoring. The Fitbit Charge 2 is also only able to store data for 7 days before overwriting. To minimize data loss, we sent weekly text reminders to participants whose data did not synchronize in the past 7 days; however, an automatic bi-weekly in-app notification would likely to have increased adherence. A similar data upload issue was noted with the ILR. The Reveal LINQ™ device can store EGM data for 27 min of patient-activated episodes and 30 min of automatic-detected episodes,39 hence, if multiple episodes are recorded over this time period without being downloaded to the base device, data are overwritten.
A further major limitation of the study was the small sample size and that all participants were Caucasian, potentially limiting the generalizability of our results. Sample size limitations prevented us from being able to power our study to derive statistical significance or draw wider conclusions applicable to a more diverse population. We were also unable to complete more than 6-month follow-up for many of our patients as the ILRs which were implanted at their index ablation during the original CASA-AF study reached end of life and were incapable of ongoing rhythm monitoring. Given the exploratory nature of our study, our pilot data show promise for further work to be undertaken with a sufficiently powered population size.
Interruptions to ILR data upload via the CARELINK remote monitoring software is also acknowledged as a major limitation. Depending on the frequency of arrhythmia recurrence, a more intensive download of ILR data would have been required to capture all EGM episodes of detected AF/AT recurrence (that are then able to be validated) rather than text only episodes. Our method of using text only episodes only if a confirmatory ILR EGM was available within 24 h to confirm AF/AT recurrence is likely to have affected the sensitivity of our results and is acknowledged as a significant limitation. In the two patients where we used pacemaker rhythm recording capabilities instead of ILR, we may have missed several episodes of AF/AT recurrence as episodes not documented on pacing reports at three monthly device interrogations had to be excluded. Furthermore, assessment of ILR EGMs was undertaken by a single reviewer; therefore, we recognize possible misclassifications may have occurred. Finally, the research team did not have access to raw PPG waveform or HRV data which possibly led to a lower PPV. Applying the normalized spike score to raw PPG waveforms or incorporating HRV data into our novel composite method may have led to improvements in PPV.
Lastly, we acknowledge ESC guidelines for the management of AF changed from when patients were recruited to the original CASA-AF study (2014 guidelines) to their subsequent recruitment to REMOTE-AF (2020 guidelines) and a change in management may have occurred as ablation technology evolved. However, despite this, the goals of treatment for long-standing persistent AF were similar, and this pilot study was not designed to assess difference in types of intervention but to look at the accuracy of wearables compared to ILR in detecting atrial arrhythmia recurrence.
Future studies that use a large variety of data modalities, similar to those recorded in REMOTE-AF, will need specific expertise in data handling and advanced data science due to the complexity of data acquired from wearables. With appropriate training and education for HCPs, it will enable a stepwise framework to incorporate high-value AI into routine cardiovascular care.18
Conclusion
Our novel composite of wearable device data (PPG-recorded HR data, HR spike score, and step count) demonstrates potential to predict AF/AT recurrence, in a post-ablation cohort compared with the gold standard ILR however improvements in accuracy are still needed. Further work is required to determine whether consumer wearables integrating HR and step count with advanced algorithmic detection tools can improve AF/AT detection and guide treatment strategies.
Lead author biography
Dr Gamith Adasuriya graduated from Barts and the London, School of Medicine and Dentistry, University of London and is currently training as a cardiology registrar in the UK. Dr Adasuriya has a special research interest in the use of AI, wearables, and remote monitoring in cardiovascular patients and has written review articles in this field. Dr Adasuriya is passionate about medical education as well as digital clinical safety and has a role as a clinical safety officer, ensuring appropriate risk management of digital health technologies prior to implementation. Outside of medicine, Dr Adasuriya is an enthusiastic cricketer.
Acknowledgements
We would like to thank the CASA-AF research team at the Royal Brompton and Harefield Hospitals and the cardAIc team at the University of Birmingham/University Hospitals Birmingham NHS Foundation Trust for set up of the study, as well as Grace Augustine (senior cardiac physiologist at Kettering General Hospital NHS Foundation Trust) for helping with the validation using ILR. We would also like to thank Julia Kurps, Joris Borgdorff, and Nivethika Mahasivam at the Hyve (Utrecht, The Netherlands) for providing technology support, the RADAR-base team (Richard Dobson, Amos Folarin, Yatharth Ranjan, Pauline Conde, Callum Stewart, and Zulqarnain Rashid at King’s College London, UK) and the BigData@Heart Consortium (Lead: Diederick E Grobbee, University Medical Centre Utrecht, The Netherlands; Scientific Coordinator: Folkert W Asselbergs, University of Amsterdam, The Netherlands).
Funding
REMOTE-AF study did not receive formal funding. Smart devices and the technology used in the trial were provided by the University of Birmingham as part of funding from an Innovative Medicines Initiative and European Union Horizon 2020 Framework grant under agreement number 116074 (BigData@Heart; https://www.bigdata-heart.eu/), supported by the European Union’s Horizon 2020 Framework research and innovation programme and European Federation of Pharmaceutical Industries and Associations. The cardAIc team at the University of Birmingham/University Hospitals Birmingham NHS Foundation Trust have received funding from the National Institute for Health Research (NIHR) Birmingham Biomedical Research Centre (NIHR203326), Health Data Research UK (HDRUK/CFC/01), NHS Data for R&D Subnational Secure Data Environment programme, and a British Heart Foundation Accelerator Award (AA/18/2/34218). The funders had no role in considering the study design or in the collection, analysis, interpretation of data, writing of the report, or decision to submit the article for publication. The opinions expressed in this study are those of the authors and do not represent the EU, NIHR, UK Department of Health and Social Care, or any of the listed institutions.
Data availability
Data supporting the findings of this study are available within the paper. Unanalysed data are available from the first author (G.A.) on reasonable request.
References
St. Jude Medical. The AF effect on quality of life questionnaire (AFEQT). St. Jude Medical, Inc.; 2023. https://www.england.nhs.uk/wp-content/uploads/2022/12/Atrial-Fibrillation-Effect-on-Quality-of-life-Questionnaire.pdf (13 January 2023).
Betts T. Continuous rhythm monitoring with implantable cardiac monitors and wearable devices with real-time smartphone alerts during AF episodes (SMART-ALERT). ClinicalTrials.gov.; 2023. https://www.clinicaltrials.gov/ct2/show/NCT05207150 (11 January 2023).
Author notes
Conflict of interest: S.G. reports funding through the BigData@Heart Innovative Medicines Initiative [grant no. 116074]. A.B. reports funding from the BigData@Heart Innovative Medicines Initiative [grant no. 116074] during the conduct of the study. D.K. reports grants from the National Institute for Health Research (NIHR CDF-2015-08-074 RATE-AF; NIHR130280 DaRe2THINK; NIHR132974 D2T-NeuroVascular; and NIHR203326 BRC), the British Heart Foundation (PG/17/55/33087, AA/18/2/34218, and FS/CDRF/21/21032), the EU/EFPIA Innovative Medicines Initiative (BigData@Heart 116074), EU Horizon (HYPERMARKER 101095480), UK National Health Service—Data for R&D—Subnational Secure Data Environment programme, and the ESC supported by educational grants from Boehringer Ingelheim/BMS-Pfizer Alliance/Bayer/Daiichi Sankyo/Boston Scientific, the NIHR/University of Oxford Biomedical Research Centre, and British Heart Foundation/University of Birmingham Accelerator Award (STEEER-AF). In addition, he has received research grants and advisory board fees from Bayer, Amomed, and Protherics Medicines Development; all outside the submitted work. G.G. reports support from the NIHR Birmingham Experimental Cancer Medicine Centre, NIHR Birmingham Surgical Reconstruction and Microbiology Research Centre, Nanocommons H2020-EU (731032), and the MRC Heath Data Research UK (HDRUK/CFC/01). S.H. reports speaker fees from Alivercor, consultancy fees from BMS, a research grant from Abbott, and the NIHR grants (CASA and LOTO). G.A., I.K.H., Z.C., J.J., D.J., V.M., W.H., and T.W. have no relevant disclosures.