-
PDF
- Split View
-
Views
-
Cite
Cite
Kai Ninomiya, Shigetaka Kageyama, Scot Garg, Shinichiro Masuda, Nozomi Kotoku, Pruthvi C Revaiah, Neil O’leary, Yoshinobu Onuma, Patrick W Serruys, for the SYNTAX Extended Survival Investigators, Can machine learning unravel unsuspected, clinically important factors predictive of long-term mortality in complex coronary artery disease? A call for ‘big data’, European Heart Journal - Digital Health, Volume 4, Issue 3, May 2023, Pages 275–278, https://doi.org/10.1093/ehjdh/ztad014
- Share Icon Share
Abstract
Risk stratification and individual risk prediction play a key role in making treatment decisions in patients with complex coronary artery disease (CAD). The aim of this study was to assess whether machine learning (ML) algorithms can improve discriminative ability and identify unsuspected, but potentially important, factors in the prediction of long-term mortality following percutaneous coronary intervention or coronary artery bypass grafting in patients with complex CAD.
To predict long-term mortality, the ML algorisms were applied to the SYNTAXES database with 75 pre-procedural variables including demographic and clinical factors, blood sampling, imaging, and patient-reported outcomes. The discriminative ability and feature importance of the ML model was assessed in the derivation cohort of the SYNTAXES trial using a 10-fold cross-validation approach. The ML model showed an acceptable discrimination (area under the curve = 0.76) in cross-validation. C-reactive protein, patient-reported pre-procedural mental status, gamma-glutamyl transferase, and HbA1c were identified as important variables predicting 10-year mortality.
The ML algorithms disclosed unsuspected, but potentially important prognostic factors of very long-term mortality among patients with CAD. A ‘mega-analysis’ based on large randomized or non-randomized data, the so-called ‘big data’, may be warranted to confirm these findings.
SYNTAXES ClinicalTrials.gov reference: NCT03417050, SYNTAX ClinicalTrials.gov reference: NCT00114972
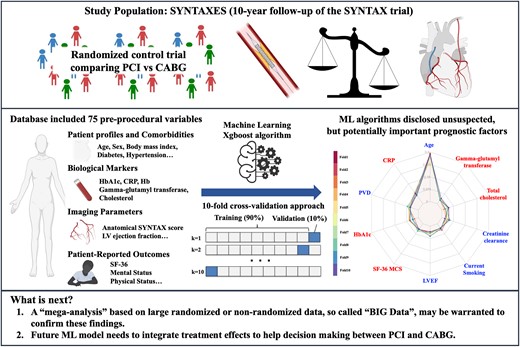
Introduction
Risk stratification and individual risk prediction play a key role in making treatment decisions in patients with complex coronary artery disease (CAD).1 Guidelines for revascularization on both sides of the Atlantic recommend using the anatomical SYNTAX score (aSS) to stratify the risk between percutaneous coronary intervention (PCI) and coronary artery bypass grafting (CABG). The SYNTAX score II 2020 (SS2020) was subsequently developed to predict 10-year mortality in patients with three-vessel disease (3VD) or left main CAD (LMCAD) following PCI or CABG by combining aSS with seven clinical prognostic determinants identified in Cox regression analysis.2
Recently, machine learning (ML) has emerged as a novel approach for developing risk models predictive of clinical outcomes.3 Several studies have shown that advanced ML algorithms achieved better risk prediction and stratification and that ML could potentially unravel variables previously unrecognized as being associated with clinical events.
The aim of this short report was to use ML algorithms in the SYNTAX trial data set to determine whether unsuspected, but potentially important prognostic factors predictive of long-term mortality following PCI or CABG could be identified.
Methods
The SYNTAX trial is a randomized controlled trial comparing PCI with CABG in 1800 patients with de novo 3VD and/or LMCAD (NCT00114972). The SYNTAXES study (NCT03417050) is an investigator-driven extended 10-year follow-up [median 11.2 years (interquartile range: 7.7–12.1) overall and 11.9 years in survivors] of the SYNTAX trial, which reported vital status up to 10 years.3
The database included 75 pre-procedural variables of clinical factors, blood sampling, imaging parameters, and patient-reported outcomes. An eXtreme gradient boosting (Xgb) algorithm was used to predict long-term mortality following PCI or CABG. For the model development, a 10-fold cross-validation approach, training the model in 90% of the patients and validating the model in the remaining 10%, was used to utilize our entire data set.
To determine the major predictors of all-cause death, we assessed each feature’s importance in the Xgb model within each fold using the gain from the varImp function of the Caret R package. The feature importance of each variable was ranked by taking average of the feature importance in each fold.
Results
At 10-year follow-up, there were 460 deaths with 212 (24%) and 248 (28%) in the CABG and PCI groups, respectively [hazard ratio 1.19 (95% confidence interval 0.99–1.43), log-rank P = 0.066].3
Figure 1A shows the 10 most important variables to predict 10-year mortality using a 10-fold cross-validation approach. Age has the largest feature importance, followed in order by C-reactive protein, peripheral vascular disease (PVD), HbA1c, 36-Item Short Form Survey Mental Component (SF36-MCS), left ventricular ejection fraction (LVEF), current smoking, creatinine clearance (CrCl), total cholesterol (T-Chol), and gamma-glutamyl transferase (GGT), whilst treatment mode (PCI or CABG) was ranked only 19th.
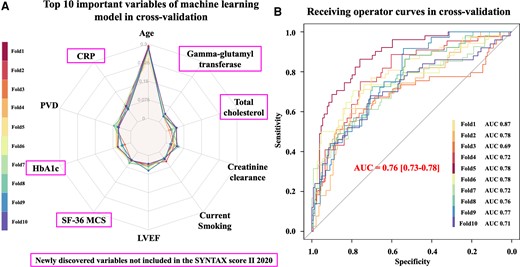
Top 10 important prognostic factors to predict 10-year death in the SYNTAX trial. (A) Top 10 important variables disclosed by the machine learning model using a 10-fold cross-validation approach. CRP, C-reactive protein; PVD, peripheral vascular disease; SF-36 MCS, 36-Item Short Form Survey Mental Component Summary; LVEF, left ventricular ejection fraction. (B) Discriminative ability for the prediction of 10-year mortality of the machine learning model using a 10-fold cross-validation approach.
The Xgb model achieved higher discriminative ability with area under the curve (AUC) of 0.76 in 10-fold cross-validation (Figure 1B) compared to the original SS2020 with AUC of 0.73.
Discussion
In the construction of SS2020, the Cox proportional hazards model was used to identify the anatomical and clinical factors to predict individual mortality following PCI or CABG. In order to avoid overfitting, unconventional prognostic factors, such as biological markers and patient-reported outcomes, were however excluded, and only 11 variables were actually tested from an array of factors of known prognostic importance.
At variance from the conventional statistical approach, ML can handle large numbers of variables and diverse parameters. Our ML model, which was built using clinical factors, blood sampling, imaging parameters, and patient-reported outcomes, demonstrated that those unconventional heterogeneous variables may have similar importance to conventional variables in predicting 10-year mortality.
Whilst most of the factors highlighted in this ML model are expected to be impactful over 10-year follow-up, findings on SF36-MCS and GGT were remarkable. Our group reported that pre-procedural biomarkers and patient-reported pre-procedural physical and mental health status (SF36-MCS) were associated with long-term mortality post revascularization, regardless of modality.4,5 Although GGT is a poorly acknowledged prognostic factor, several epidemiological studies demonstrated an association with cardiovascular disease and mortality.6
The present findings were derived from a single data set and need further confirmation using an external validation data set. However, limited data on 10-year outcomes following PCI or CABG are available and variables such as mental/physical status, GGT, or C-reactive protein are not always collected in the clinical trials; hence, external validation of our model might be not feasible in the context of a clinical trial.
The ultimate goal is to help select the modality of revascularization; therefore, future ML models need to integrate treatment effects and interaction with treatment arm (PCI or CABG).
Conclusions
The ML algorithms disclosed unsuspected, but potentially important prognostic factors of very long-term mortality among patients with CAD. A ‘mega-analysis’ based on large randomized or non-randomized data, the so-called ‘big data’, may be warranted to confirm these findings.
Acknowledgements
None.
Funding
The SYNTAX Extended Survival study was supported by the German Foundation of Heart Research (Frankfurt am Main, Germany). The SYNTAX trial, during 0–5-year follow-up, was funded by Boston Scientific Corporation (Marlborough, MA, USA). Both sponsors had no role in the study design, data collection, data analyses, and interpretation of the study data, nor were involved in the decision to publish the final manuscript. The principal investigators and authors had complete scientific freedom.
Data availability
All data are included in the submission/manuscript file.
References
Author notes
Kai Ninomiya and Shigetaka Kageyama contributed equally to the study.
Conflict of interest: P.W.S. reports institutional grants from Sinomedical Sciences Technology, SMT (Sahajanand Medical Technological), Philips/Volcano, Xeltis, GE Healthcare, Novartis, and HeartFlow, outside the submitted work.
- percutaneous coronary intervention
- coronary artery bypass surgery
- coronary arteriosclerosis
- area under curve
- hemoglobin a, glycosylated
- phlebotomy
- c-reactive protein
- diagnostic imaging
- gamma-glutamyl transferase
- mortality
- treatment outcome
- prognostic factors
- stratification
- patient self-report
- syntax
- machine learning