-
PDF
- Split View
-
Views
-
Cite
Cite
A Garcia Olea, I Valdelvira Vazquez, I Diez Gonzalez, A Atutxa Salazar, K Gojenola Galletebeitia, J M Ormaetxe Merodio, Prediction of new onset atrial fibrillation recurrence or persistence with artificial intelligence: first insights of the PRAFAI study, European Heart Journal - Digital Health, Volume 3, Issue 4, December 2022, ztac076.2773, https://doi.org/10.1093/ehjdh/ztac076.2773
- Share Icon Share
Abstract
Atrial fibrillation (AF) is the most prevalent arrhythmia in the world and it is associated with a high rate of cardiovascular morbidity and mortality. A higher burden of atrial fibrillation is related to more adverse cardiovascular events and the rhythm control strategy (maintenance or recovery of sinus rhythm) is particularly indicated in patients with paroxysmal symptomatic atrial fibrillation. All things considered, in order to select the optimal therapeutic strategy, it might be useful to accurately predict the probability of recurrence or permanence of AF after its onset. The predictive models implemented so far, show a limited predictive power or were built on samples of patients undergoing specific therapies. Thus, developing an specific model based on features derived from electronic health records (EHR) of patients with a first AF episode seems essential.
To develop an artificial intelligence powered predictive model based on EHR variables in order to predict the AF recurrence after its onset.
The methodological design of the research is based on the analysis of secondary data from a retrospective cohort obtained from the EHR of a tertiary hospital. All new onset AF from 2015 to 2018 were identified by ICD codes, and a 2 years EHR driven follow-up was made to determine the AF recurrence or permanence of the arrhythmia in those patients. A systematic review was pursued to determine which features exhibited a greater predictive power for AF recurrence, and they were inquired to the system for the mentioned participants. After the standardization of the variables and a feature selection process, several missing value imputation methods and around 12 different Machine Learning algorithms were tested to predict the AF recurrence in a subset of patients who developed AF in the first semester of 2015, in order to select the algorithms with the highest prediction accuracy for further tests.
523 patients had an AF onset in the first semester of 2015. Among them, 310 (59.3%) had AF recurrence in a 2 years period. A support vector machine (SVM) algorithm with a median imputation method to handle missing values performed the best and had an 0.77 AUC in the training set and 0.71 in the test set. Hyperthyroidism, vasodilator drug use and obstructive sleep apnea were notoriously related to AF recurrence, while Ic antiarrhythmic drugs, AF ablation, cognitive impairment and anxiety were the most influential variables for the absence of recurrence. Classical AF recurrence factors such as age or left atrium diameter ratify their role in this setting.
A SVM Machine Learning algorithm discloses an accurate AF recurrence probability based on EHR features. Pharmacologic and epidemiological variables, added to classically assessed ones, seem to be specially related to this arrhythmic recurrence. Further research including the whole cohort features will probably improve the accuracy of this selected SVM algorithm.
Type of funding sources: None.
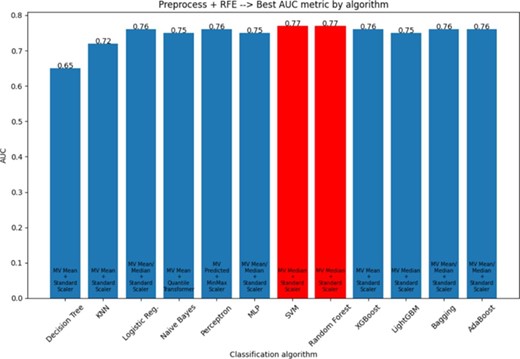
Figure 1. Machine Learning algorithm selection
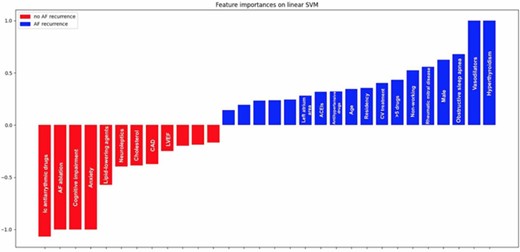
Figure 2. Features related to AF recurrence
- anti-arrhythmia agents
- anxiety
- cardiac arrhythmia
- atrial fibrillation
- hyperthyroidism
- artificial intelligence
- left atrium
- obstructive sleep apnea
- vasodilators
- cardiovascular system
- follow-up
- morbidity
- mortality
- pharmacology
- sinus rhythm
- cognitive impairment
- electronic medical records
- cardiovascular event
- ablation
- rhythm
- imputation
- machine learning