-
PDF
- Split View
-
Views
-
Cite
Cite
Rutger Willem Maurice Brouwers, Alberto Brini, Robin Wilhelmus Franciscus Henricus Kuijpers, Jozua Johannes Kraal, Hareld Marijn Clemens Kemps, Predictors of non-participation in a cardiac telerehabilitation programme: a prospective analysis, European Heart Journal - Digital Health, Volume 3, Issue 1, March 2022, Pages 81–89, https://doi.org/10.1093/ehjdh/ztab105
- Share Icon Share
Abstract
Current cardiac telerehabilitation (CTR) interventions are insufficiently tailored to the preferences and competences of individual patients, which raises the question whether their implementation will increase overall participation and adherence to cardiac rehabilitation (CR). However, research on patient-specific factors that influence participation and adoption of CTR interventions is scarce. The aim of this study was to evaluate which patient-related characteristics influence participation in a novel CTR intervention in patients with coronary artery disease.
This prospective observational substudy of the SmartCare-CAD randomized controlled trial evaluated patient characteristics of study participants as proxy for participation in a CTR intervention. We compared demographic, geographic, and health-related characteristics between trial participants and non-participants to determine which characteristics influenced trial participation. A total of 699 patients (300 participants and 399 non-participants; 84% male, mean age 64.3 ± 10.5 years) were included. Most of the non-participants refused participation because of insufficient technical skills or lack of interest in digital health (26%), or preferred centre-based CR (21%). Variables independently associated with non-participation included: higher age, lower educational level, shorter travelling distance, smoking, positive family history for cardiovascular disease, having undergone coronary artery bypass grafting; and a higher blood pressure, worse exercise capacity, and higher risk of depression before the start of CR.
Participation in CTR is strongly influenced by demographic and health-related factors such as age, educational level, smoking status, and both physical and mental functioning. Cardiac telerehabilitation interventions should therefore be redesigned with the involvement of these currently underrepresented patient subgroups.
Introduction
Participation in a cardiac rehabilitation (CR) programme is associated with reduced cardiovascular mortality and hospitalization, increased quality of life (QoL), and a lower residual cardiovascular risk in patients with coronary artery disease (CAD).1–3 Moreover, CR has shown to be cost-effective compared with usual care.4 In the most recent European guidelines on cardiovascular disease (CVD) prevention, exercise-based CR has therefore received a class IA recommendation.5 Unfortunately, less than half of eligible patients participate in CR, and even fewer patients complete CR as dropout throughout the programme is common.6,7 Patient-related factors that are associated with non-participation or dropout from CR include older age, female sex, the presence of comorbidities, and not having undergone coronary revascularization after a cardiovascular event.8,9 In addition, after successfully completing a CR programme, patients often relapse into unhealthy behaviour, increasing their risk for recurrent cardiovascular events.10,11
Cardiac telerehabilitation (CTR) can be defined as ‘cardiac rehabilitation from a distance by using devices that monitor and communicate patient specific information to a caregiver’,12 often involving telephones, videoconferencing, and mobile applications.13 Multidisciplinary CTR has been shown to be (cost-)effective compared with conventional, centre-based CR in patients with CAD,4,14,15 and has recently been endorsed in Dutch multidisciplinary CR guidelines.16 Widespread use of CTR is generally regarded as an intervention that will increase CR participation rates by resolving utilization barriers, and may additionally reduce relapse into unhealthy behaviour after CR completion by incorporating healthier lifestyle behaviour into patients’ daily lives.6,17 These two effects have, however, not been demonstrated yet. In fact, recent CTR trials mainly included participants who were relatively young, predominantly male and had a low to moderate risk of recurrent events.15,18,19 Therefore, it is questionable whether CTR in its current form is suitable and appealing for patients with a higher residual cardiovascular risk, in whom participation in conventional CR programmes is lower and the risk at dropout and non-adherence is higher.7
Tailoring CTR interventions should involve adaptation of programme content and technology to different user profiles, in order to be able to personalize interventions to individual preferences.20 Moreover, involving multiple stakeholders (including healthcare professionals and patients) in this process allows for participatory (re)design of CTR interventions, which is essential for the development of digital health interventions.21 Although from recent CTR trials it is evident that some form of selection bias has occurred (i.e. the inclusion of predominantly male patients with a low to moderate risk),15,18 research on patient-specific factors that influence participation and adoption of CTR interventions is scarce and often evaluated in small patient samples.22 Several trials demonstrated that older age and lower educational levels negatively influenced the acceptance of mobile technology for CR,22,23 which has also been demonstrated for patients with other chronic diseases24,25 and healthy adults.26 Other factors associated with lower acceptance of CTR were lower monthly income and lower levels of physical activity.22 Although it is well known which factors are associated with low participation levels in conventional CR, the literature lacks of a comprehensive evaluation of factors that contribute to non-participation in CTR. Therefore, in this paper, we aim to fill this gap by considering a wide spectrum of explanatory factors among those which have been proposed in the literature, in a large sample of patients with CAD.
Methods
Study design
This study was designed as a single-centre prospective observational study among patients with CAD entering CR and served as a substudy of the SmartCare-CAD trial.15 The study design was approved by the Medical Ethics Review Committee of Máxima Medical Center, Veldhoven, the Netherlands. The SmartCare-CAD trial was a randomized controlled trial that evaluated the effectiveness of a novel CTR intervention with relapse prevention as compared with centre-based CR in 300 patients with CAD in the Netherlands. The study protocol and main results of this trial have been described elsewhere.15,27
Study population and informed consent
Patients entering phase II outpatient CR at Máxima Medical Center were eligible for participation in the SmartCare-CAD trial if they were referred for CR because of stable CAD, an acute coronary syndrome (ACS), and/or after coronary revascularization, and if they were referred for the exercise training module of the CR programme.27,28 Patients were also required to have a personal computer with internet connectivity and a mobile phone with short message service functionality. The complete list of inclusion and exclusion criteria can be found in the study protocol.27 A short description of the selection and inclusion of study participants is given below.
SmartCare-CAD trial participation
Patients eligible for participation in SmartCare-CAD were verbally informed about the SmartCare-CAD study procedures by their CR co-ordinator (nurse specialist) during the CR intake procedure and received written information when interested in trial participation. After a brief reflection period, they were seen by the co-ordinating investigator of the SmartCare-CAD trial to obtain written informed consent for trial participation. If patients refused to participate in the SmartCare-CAD trial, they entered the regular, centre-based CR programme. They received verbal and written information about the substudy and were asked to provide written informed consent for the registration of their reason for refusal (for the SmartCare-CAD trial) and the use of their demographic, geographic, and health-related characteristics for the substudy (Figure 1). Both participants (in the SmartCare-CAD trial) and non-participants were included in the substudy.
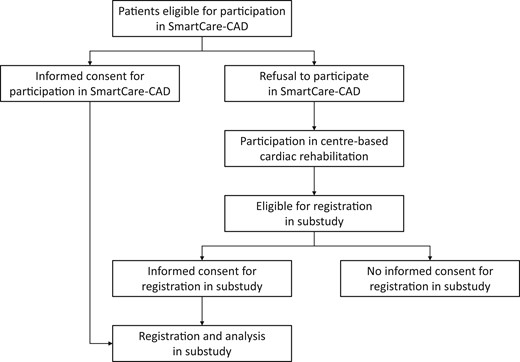
Measured variables
The main outcome measure was participation in the SmartCare-CAD trial, which served as proxy for participation in a CTR programme. Furthermore, we compared demographic, geographic, and health-related characteristics between trial participants and non-participants to determine which characteristics influenced trial participation. Education was also included as additional patient information and was categorized as highly educated (defined as having a college or university degree) vs. not highly educated. Measurements obtained before the start of CR (including questionnaires on QoL, anxiety, and depression) were part of the individual needs assessment in the CR intake procedure28,29 and were included in our dataset.
Health-related QoL was assessed by the KvL-H (Kwaliteit van Leven bij Hartpatiënten; QoL in cardiac patients) questionnaire, a validated Dutch translation of the MacNew heart disease health-related QoL questionnaire.30 Anxiety and depression were assessed by the GAD-7 (Generalized Anxiety Disorder) and PHQ-9 (Patient Health Questionnaire) questionnaires, respectively.31,32
Statistical analysis
Each patient was labelled as participant (in the SmartCare-CAD trial) or non-participant. The Shapiro–Wilk test was used to test for normality among the collected patient characteristics. Based on their distribution, continuous variables are reported as mean ± standard deviation (SD) or median and interquartile range (IQR); categorical data are reported as numbers and percentages. We analysed differences in variables between participants and non-participants using the χ2 test for categorical variables and the independent samples T-test (or Mann–Whitney U-test) for continuous variables. These statistical tests were two-tailed and P-values (‘P’) were considered statistically significant when P < 0.05.
The dataset contained a substantial amount of missing data (range 0–18%). We performed multiple imputation using predictive mean matching.33 For each of the 20 imputed datasets, a saturated logistic regression model was performed. The probability of non-participating to CTR was modelled with a logit link function. Variables with a P-value of <0.10 were considered statistically significant for each imputed dataset. A majority vote (50%) determined the set of final predictors to be used in each imputed model, i.e. if an explanatory variable is significant in more than 50% of the cases (10/20), then it is retained as final predictor for the logistic regression in each imputed dataset. We used Rubin’s rule34 to pool the results over the imputations. All statistical analyses were carried out using SAS 9.4 software.
Results
Between May 2016 and July 2018, 300 patients were included in the SmartCare-CAD trial, and 399 declined participation in SmartCare-CAD but provided informed consent to be registered in the substudy, resulting in a total of 699 patients included in the substudy.
Patients were mostly male (84%) and had a mean age of 64.3 ± 10.5 years. Most patients were referred for CR because of an ACS (71%), and 91% had undergone coronary revascularization before the start of CR. The most frequently reported reasons for non-participation in SmartCare-CAD were insufficient technical skills or lack of interest in digital health (26%), preference for centre-based CR (21%), (trial) participation being too burdensome (13%), not being convinced of the added value of CTR (7%), and lack of time (6%; Figure 2).
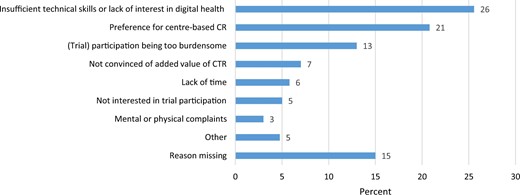
Most frequently reported reasons for non-participation in the SmartCare-CAD trial. CR, cardiac rehabilitation; CTR, cardiac telerehabilitation.
Non-participants were significantly older (67.0 vs. 60.7 years, P < 0.001), more often female (20.3% vs. 11.3%, P = 0.002), less often employed (28.0% vs. 60.0%, P < 0.001), and were less often highly educated (24.2% vs. 50.3%, P < 0.001) than trial participants (Table 1). Furthermore, non-participants more often smoked before hospitalization (25.3% vs. 6.4%, P < 0.001), more often had previously diagnosed diabetes (17.3% vs. 11.7%, P = 0.039), had higher systolic blood pressure at the start of CR (134 vs. 125 mmHg, P < 0.001), and more often had a positive family history for CVD (67.9% vs. 57.9%, P = 0.010). Finally, they had significantly lower exercise capacity (82.9% vs. 91.6% of expected workload), more comorbidities (37.9% vs. 18.7% with Charlson Comorbidity Index ≥2), lower QoL (total score), and higher risk of depression at the start of CR (P-values of <0.001, 0.030, and 0.001, respectively).
Demographic, geographic, and health-related characteristics of study participants and non-participants before the start of cardiac rehabilitation
. | Non-participants . | Participants . | P-value . |
---|---|---|---|
Demographic characteristics | |||
Age, yearsa | 67.0 ± 10.4 | 60.7 ± 9.5 | <0.001 |
Female, n (%) | 81 (20.3) | 34 (11.3) | 0.002 |
Highly educated, n (%) | 67 (24.2) | 149 (50.3) | <0.001 |
Employed, n (%) | 100 (28.0) | 180 (60.0) | <0.001 |
Living together, n (%) | 314 (86.5) | 261 (87.3) | 0.765 |
Geographic and logistic characteristics | |||
Distance to hospital, kmb | 6.6 (9.3) | 8.0 (11.7) | 0.018 |
Waiting time for CR intake, daysb | 13 (10) | 13 (12) | 0.389 |
Health-related characteristics | |||
Diagnosis, n (%) | 0.704 | ||
Stable angina | 113 (28.4) | 79 (26.3) | |
Unstable angina | 53 (13.3) | 35 (11.7) | |
NSTEMI | 93 (23.4) | 82 (27.3) | |
STEMI | 131 (32.9) | 100 (33.3) | |
Other | 8 (2.0) | 4 (1.3) | |
Treatment, n (%) | <0.001 | ||
Medication | 35 (8.8) | 25 (8.3) | |
PCI | 190 (47.6) | 189 (63.0) | |
CABG | 152 (38.1) | 78 (26.0) | |
CABG + valve surgery | 16 (4.0) | 8 (2.7) | |
Other | 6 (1.5) | 0 (0) | |
Duration of hospitalization, daysb | 5 (5) | 4 (4) | 0.045 |
Measurements | |||
Charlson Comorbidity Indexb | 1 (1) | 1 (1) | <0.001 |
Body mass index, kg/m2a | 26.7 ± 3.9 | 27.3 ± 3.5 | 0.058 |
Waist circumference, cma | 101 ± 11 | 102 ± 10 | 0.476 |
Systolic blood pressure, mmHga | 134 ± 21 | 125 ± 17 | <0.001 |
Diastolic blood pressure, mmHga | 75 ± 13 | 74 ± 10 | 0.249 |
Exercise capacity, % of expected workloada | 82.9 ± 21.2 | 91.6 ± 18.9 | <0.001 |
Adherence to physical activity guidelines, days/weekb | 7 (2) | 7 (2) | 0.397 |
Adherence to exercise guidelines, days/weekb | 1 (3) | 2 (3) | 0.195 |
Questionnaires | |||
Quality of life, KVL-H total scoreb | 5.0 (1.6) | 5.3 (1.5) | 0.030 |
Quality of life, KVL-H emotional domainb | 5.2 (1.8) | 5.5 (1.7) | 0.004 |
Quality of life, KVL-H physical domainb | 4.5 (2.0) | 4.9 (1.7) | 0.018 |
Quality of life, KVL-H social domainb | 5.4 (1.8) | 5.6 (1.4) | 0.609 |
Anxiety, GAD-7 scoreb | 2 (0) | 2 (0) | 0.533 |
Depression, PHQ-2 scoreb | 1 (2) | 0 (1) | 0.001 |
Depression, PHQ-9 scoreb | 4 (2) | 3 (1) | 0.001 |
Risk factors for cardiovascular disease, n (%) | |||
(Previously diagnosed) hypercholesterolemia | 168 (42.1) | 117 (39.3) | 0.450 |
(Previously diagnosed) hypertension | 148 (37.1) | 122 (40.7) | 0.337 |
(Previously diagnosed) diabetes mellitus | 69 (17.3) | 35 (11.7) | 0.039 |
Smoking at hospitalization | 98 (25.3) | 19 (6.4) | <0.001 |
Family predisposition for CVD (patient reported) | 226 (67.9) | 172 (57.9) | 0.010 |
. | Non-participants . | Participants . | P-value . |
---|---|---|---|
Demographic characteristics | |||
Age, yearsa | 67.0 ± 10.4 | 60.7 ± 9.5 | <0.001 |
Female, n (%) | 81 (20.3) | 34 (11.3) | 0.002 |
Highly educated, n (%) | 67 (24.2) | 149 (50.3) | <0.001 |
Employed, n (%) | 100 (28.0) | 180 (60.0) | <0.001 |
Living together, n (%) | 314 (86.5) | 261 (87.3) | 0.765 |
Geographic and logistic characteristics | |||
Distance to hospital, kmb | 6.6 (9.3) | 8.0 (11.7) | 0.018 |
Waiting time for CR intake, daysb | 13 (10) | 13 (12) | 0.389 |
Health-related characteristics | |||
Diagnosis, n (%) | 0.704 | ||
Stable angina | 113 (28.4) | 79 (26.3) | |
Unstable angina | 53 (13.3) | 35 (11.7) | |
NSTEMI | 93 (23.4) | 82 (27.3) | |
STEMI | 131 (32.9) | 100 (33.3) | |
Other | 8 (2.0) | 4 (1.3) | |
Treatment, n (%) | <0.001 | ||
Medication | 35 (8.8) | 25 (8.3) | |
PCI | 190 (47.6) | 189 (63.0) | |
CABG | 152 (38.1) | 78 (26.0) | |
CABG + valve surgery | 16 (4.0) | 8 (2.7) | |
Other | 6 (1.5) | 0 (0) | |
Duration of hospitalization, daysb | 5 (5) | 4 (4) | 0.045 |
Measurements | |||
Charlson Comorbidity Indexb | 1 (1) | 1 (1) | <0.001 |
Body mass index, kg/m2a | 26.7 ± 3.9 | 27.3 ± 3.5 | 0.058 |
Waist circumference, cma | 101 ± 11 | 102 ± 10 | 0.476 |
Systolic blood pressure, mmHga | 134 ± 21 | 125 ± 17 | <0.001 |
Diastolic blood pressure, mmHga | 75 ± 13 | 74 ± 10 | 0.249 |
Exercise capacity, % of expected workloada | 82.9 ± 21.2 | 91.6 ± 18.9 | <0.001 |
Adherence to physical activity guidelines, days/weekb | 7 (2) | 7 (2) | 0.397 |
Adherence to exercise guidelines, days/weekb | 1 (3) | 2 (3) | 0.195 |
Questionnaires | |||
Quality of life, KVL-H total scoreb | 5.0 (1.6) | 5.3 (1.5) | 0.030 |
Quality of life, KVL-H emotional domainb | 5.2 (1.8) | 5.5 (1.7) | 0.004 |
Quality of life, KVL-H physical domainb | 4.5 (2.0) | 4.9 (1.7) | 0.018 |
Quality of life, KVL-H social domainb | 5.4 (1.8) | 5.6 (1.4) | 0.609 |
Anxiety, GAD-7 scoreb | 2 (0) | 2 (0) | 0.533 |
Depression, PHQ-2 scoreb | 1 (2) | 0 (1) | 0.001 |
Depression, PHQ-9 scoreb | 4 (2) | 3 (1) | 0.001 |
Risk factors for cardiovascular disease, n (%) | |||
(Previously diagnosed) hypercholesterolemia | 168 (42.1) | 117 (39.3) | 0.450 |
(Previously diagnosed) hypertension | 148 (37.1) | 122 (40.7) | 0.337 |
(Previously diagnosed) diabetes mellitus | 69 (17.3) | 35 (11.7) | 0.039 |
Smoking at hospitalization | 98 (25.3) | 19 (6.4) | <0.001 |
Family predisposition for CVD (patient reported) | 226 (67.9) | 172 (57.9) | 0.010 |
CABG, coronary artery bypass grafting; CR, cardiac rehabilitation; CVD, cardiovascular disease; GAD-7, Generalised Anxiety Disorder-7 (higher scores indicate higher risk of anxiety disorder); KVL-H Kwaliteit van Leven bij Hartpatiënten (quality of life in cardiac patients; higher scores indicate better quality of life); NSTEMI, non-ST segment elevation myocardial infarction; PCI, percutaneous coronary intervention; PHQ, patient health questionnaire (higher scores indicate higher risk of depression); STEMI, ST-segment elevation myocardial infarction.
Values reported as mean ± standard deviation.
Values reported as median (interquartile range).
Demographic, geographic, and health-related characteristics of study participants and non-participants before the start of cardiac rehabilitation
. | Non-participants . | Participants . | P-value . |
---|---|---|---|
Demographic characteristics | |||
Age, yearsa | 67.0 ± 10.4 | 60.7 ± 9.5 | <0.001 |
Female, n (%) | 81 (20.3) | 34 (11.3) | 0.002 |
Highly educated, n (%) | 67 (24.2) | 149 (50.3) | <0.001 |
Employed, n (%) | 100 (28.0) | 180 (60.0) | <0.001 |
Living together, n (%) | 314 (86.5) | 261 (87.3) | 0.765 |
Geographic and logistic characteristics | |||
Distance to hospital, kmb | 6.6 (9.3) | 8.0 (11.7) | 0.018 |
Waiting time for CR intake, daysb | 13 (10) | 13 (12) | 0.389 |
Health-related characteristics | |||
Diagnosis, n (%) | 0.704 | ||
Stable angina | 113 (28.4) | 79 (26.3) | |
Unstable angina | 53 (13.3) | 35 (11.7) | |
NSTEMI | 93 (23.4) | 82 (27.3) | |
STEMI | 131 (32.9) | 100 (33.3) | |
Other | 8 (2.0) | 4 (1.3) | |
Treatment, n (%) | <0.001 | ||
Medication | 35 (8.8) | 25 (8.3) | |
PCI | 190 (47.6) | 189 (63.0) | |
CABG | 152 (38.1) | 78 (26.0) | |
CABG + valve surgery | 16 (4.0) | 8 (2.7) | |
Other | 6 (1.5) | 0 (0) | |
Duration of hospitalization, daysb | 5 (5) | 4 (4) | 0.045 |
Measurements | |||
Charlson Comorbidity Indexb | 1 (1) | 1 (1) | <0.001 |
Body mass index, kg/m2a | 26.7 ± 3.9 | 27.3 ± 3.5 | 0.058 |
Waist circumference, cma | 101 ± 11 | 102 ± 10 | 0.476 |
Systolic blood pressure, mmHga | 134 ± 21 | 125 ± 17 | <0.001 |
Diastolic blood pressure, mmHga | 75 ± 13 | 74 ± 10 | 0.249 |
Exercise capacity, % of expected workloada | 82.9 ± 21.2 | 91.6 ± 18.9 | <0.001 |
Adherence to physical activity guidelines, days/weekb | 7 (2) | 7 (2) | 0.397 |
Adherence to exercise guidelines, days/weekb | 1 (3) | 2 (3) | 0.195 |
Questionnaires | |||
Quality of life, KVL-H total scoreb | 5.0 (1.6) | 5.3 (1.5) | 0.030 |
Quality of life, KVL-H emotional domainb | 5.2 (1.8) | 5.5 (1.7) | 0.004 |
Quality of life, KVL-H physical domainb | 4.5 (2.0) | 4.9 (1.7) | 0.018 |
Quality of life, KVL-H social domainb | 5.4 (1.8) | 5.6 (1.4) | 0.609 |
Anxiety, GAD-7 scoreb | 2 (0) | 2 (0) | 0.533 |
Depression, PHQ-2 scoreb | 1 (2) | 0 (1) | 0.001 |
Depression, PHQ-9 scoreb | 4 (2) | 3 (1) | 0.001 |
Risk factors for cardiovascular disease, n (%) | |||
(Previously diagnosed) hypercholesterolemia | 168 (42.1) | 117 (39.3) | 0.450 |
(Previously diagnosed) hypertension | 148 (37.1) | 122 (40.7) | 0.337 |
(Previously diagnosed) diabetes mellitus | 69 (17.3) | 35 (11.7) | 0.039 |
Smoking at hospitalization | 98 (25.3) | 19 (6.4) | <0.001 |
Family predisposition for CVD (patient reported) | 226 (67.9) | 172 (57.9) | 0.010 |
. | Non-participants . | Participants . | P-value . |
---|---|---|---|
Demographic characteristics | |||
Age, yearsa | 67.0 ± 10.4 | 60.7 ± 9.5 | <0.001 |
Female, n (%) | 81 (20.3) | 34 (11.3) | 0.002 |
Highly educated, n (%) | 67 (24.2) | 149 (50.3) | <0.001 |
Employed, n (%) | 100 (28.0) | 180 (60.0) | <0.001 |
Living together, n (%) | 314 (86.5) | 261 (87.3) | 0.765 |
Geographic and logistic characteristics | |||
Distance to hospital, kmb | 6.6 (9.3) | 8.0 (11.7) | 0.018 |
Waiting time for CR intake, daysb | 13 (10) | 13 (12) | 0.389 |
Health-related characteristics | |||
Diagnosis, n (%) | 0.704 | ||
Stable angina | 113 (28.4) | 79 (26.3) | |
Unstable angina | 53 (13.3) | 35 (11.7) | |
NSTEMI | 93 (23.4) | 82 (27.3) | |
STEMI | 131 (32.9) | 100 (33.3) | |
Other | 8 (2.0) | 4 (1.3) | |
Treatment, n (%) | <0.001 | ||
Medication | 35 (8.8) | 25 (8.3) | |
PCI | 190 (47.6) | 189 (63.0) | |
CABG | 152 (38.1) | 78 (26.0) | |
CABG + valve surgery | 16 (4.0) | 8 (2.7) | |
Other | 6 (1.5) | 0 (0) | |
Duration of hospitalization, daysb | 5 (5) | 4 (4) | 0.045 |
Measurements | |||
Charlson Comorbidity Indexb | 1 (1) | 1 (1) | <0.001 |
Body mass index, kg/m2a | 26.7 ± 3.9 | 27.3 ± 3.5 | 0.058 |
Waist circumference, cma | 101 ± 11 | 102 ± 10 | 0.476 |
Systolic blood pressure, mmHga | 134 ± 21 | 125 ± 17 | <0.001 |
Diastolic blood pressure, mmHga | 75 ± 13 | 74 ± 10 | 0.249 |
Exercise capacity, % of expected workloada | 82.9 ± 21.2 | 91.6 ± 18.9 | <0.001 |
Adherence to physical activity guidelines, days/weekb | 7 (2) | 7 (2) | 0.397 |
Adherence to exercise guidelines, days/weekb | 1 (3) | 2 (3) | 0.195 |
Questionnaires | |||
Quality of life, KVL-H total scoreb | 5.0 (1.6) | 5.3 (1.5) | 0.030 |
Quality of life, KVL-H emotional domainb | 5.2 (1.8) | 5.5 (1.7) | 0.004 |
Quality of life, KVL-H physical domainb | 4.5 (2.0) | 4.9 (1.7) | 0.018 |
Quality of life, KVL-H social domainb | 5.4 (1.8) | 5.6 (1.4) | 0.609 |
Anxiety, GAD-7 scoreb | 2 (0) | 2 (0) | 0.533 |
Depression, PHQ-2 scoreb | 1 (2) | 0 (1) | 0.001 |
Depression, PHQ-9 scoreb | 4 (2) | 3 (1) | 0.001 |
Risk factors for cardiovascular disease, n (%) | |||
(Previously diagnosed) hypercholesterolemia | 168 (42.1) | 117 (39.3) | 0.450 |
(Previously diagnosed) hypertension | 148 (37.1) | 122 (40.7) | 0.337 |
(Previously diagnosed) diabetes mellitus | 69 (17.3) | 35 (11.7) | 0.039 |
Smoking at hospitalization | 98 (25.3) | 19 (6.4) | <0.001 |
Family predisposition for CVD (patient reported) | 226 (67.9) | 172 (57.9) | 0.010 |
CABG, coronary artery bypass grafting; CR, cardiac rehabilitation; CVD, cardiovascular disease; GAD-7, Generalised Anxiety Disorder-7 (higher scores indicate higher risk of anxiety disorder); KVL-H Kwaliteit van Leven bij Hartpatiënten (quality of life in cardiac patients; higher scores indicate better quality of life); NSTEMI, non-ST segment elevation myocardial infarction; PCI, percutaneous coronary intervention; PHQ, patient health questionnaire (higher scores indicate higher risk of depression); STEMI, ST-segment elevation myocardial infarction.
Values reported as mean ± standard deviation.
Values reported as median (interquartile range).
Multivariable logistic regression analysis demonstrated that the following variables were associated with non-participation in the SmartCare-CAD trial: higher age, lower educational level, shorter travelling distance to the nearest hospital, smoking, no previously diagnosed hypertension, positive family history for CVD, having undergone coronary artery bypass grafting (CABG; as compared with PCI), higher blood pressure before start of CR, worse exercise capacity before start of CR, higher risk of depression, and better QoL (social subscale). The estimates of significant variables in the logistic regression analysis are provided in Table 2. During the analysis, it was investigated whether higher-order interactions (among the entire set of predictors) could have improved the model fit. However, the higher-order model showed very similar goodness-of-fit measures that did not indicate a substantial improvement of the model fit. Furthermore, for the sake of the interpretability and inferential purposes, the linear mean model discussed here was preferred.
Covariates . | Estimate . | SE . | 95% CI . |
---|---|---|---|
Intercept | −7.87 | 1.24 | [−10.30; −5.45] |
Age (per year) | 0.09 | 0.011 | [0.064; 0.11] |
Being highly educated | −1.09 | 0.21 | [−1.51; −0.68] |
Distance to hospital (per kilometre) | −0.02 | 0.010 | [−0.0424; −0.0028] |
Smoking | 2.34 | 0.11 | [1.68; 2.99] |
Previously diagnosed hypertension | −0.42 | 0.19 | [−0.799; −0.037] |
Positive family history for CVD | 0.56 | 0.20 | [0.16; 0.96] |
CABG (as compared with PCI) | 0.48 | 0.23 | [0.031; 0.922] |
Systolic blood pressure (per mmHg) | 0.02 | 0.005 | [0.009; 0.029] |
Workload (per % of expected maximal workload) | −0.02 | 0.006 | [−0.026; −0.005] |
Risk of depression (PHQ-2 score, per point increase) | 0.30 | 0.10 | [0.11; 0.50] |
Quality of life (KVL-H social score, per point increase) | 0.24 | 0.12 | [0.0048; 0.4728] |
Covariates . | Estimate . | SE . | 95% CI . |
---|---|---|---|
Intercept | −7.87 | 1.24 | [−10.30; −5.45] |
Age (per year) | 0.09 | 0.011 | [0.064; 0.11] |
Being highly educated | −1.09 | 0.21 | [−1.51; −0.68] |
Distance to hospital (per kilometre) | −0.02 | 0.010 | [−0.0424; −0.0028] |
Smoking | 2.34 | 0.11 | [1.68; 2.99] |
Previously diagnosed hypertension | −0.42 | 0.19 | [−0.799; −0.037] |
Positive family history for CVD | 0.56 | 0.20 | [0.16; 0.96] |
CABG (as compared with PCI) | 0.48 | 0.23 | [0.031; 0.922] |
Systolic blood pressure (per mmHg) | 0.02 | 0.005 | [0.009; 0.029] |
Workload (per % of expected maximal workload) | −0.02 | 0.006 | [−0.026; −0.005] |
Risk of depression (PHQ-2 score, per point increase) | 0.30 | 0.10 | [0.11; 0.50] |
Quality of life (KVL-H social score, per point increase) | 0.24 | 0.12 | [0.0048; 0.4728] |
The displayed effects (in the logit scale) were all statistically significant (P < 0.05 after pooling the 20 imputations) in the Multivariable Logistic Regression analysis. The backward elimination excluded the following variables from the final analysis: gender, employment, civil state (living together), waiting time for CR intake, diagnosis, duration of hospitalization, Charlson Comorbidity Index, body mass index, waist circumference, adherence to physical activity and exercise guidelines, quality of life (total, emotional, and physical subscales), GAD-7 score, PHQ-9 score, previously diagnosed hypercholesterolaemia, and previously diagnosed diabetes mellitus. The intercept effect is modelling the probability that a non-highly educated, non-smoking patient, with no family history of CVD, and no previously diagnosed hypertension, who underwent PCI, did not participate in the CTR programme.
CABG, coronary artery bypass grafting; CTR, cardiac telerehabilitation; CVD, cardiovascular disease; KVL-H, Kwaliteit van Leven bij Hartpatiënten (quality of life in cardiac patients; higher scores indicate better quality of life); PCI, percutaneous coronary intervention; PHQ, patient health questionnaire (higher scores indicate higher risk of depression).
Covariates . | Estimate . | SE . | 95% CI . |
---|---|---|---|
Intercept | −7.87 | 1.24 | [−10.30; −5.45] |
Age (per year) | 0.09 | 0.011 | [0.064; 0.11] |
Being highly educated | −1.09 | 0.21 | [−1.51; −0.68] |
Distance to hospital (per kilometre) | −0.02 | 0.010 | [−0.0424; −0.0028] |
Smoking | 2.34 | 0.11 | [1.68; 2.99] |
Previously diagnosed hypertension | −0.42 | 0.19 | [−0.799; −0.037] |
Positive family history for CVD | 0.56 | 0.20 | [0.16; 0.96] |
CABG (as compared with PCI) | 0.48 | 0.23 | [0.031; 0.922] |
Systolic blood pressure (per mmHg) | 0.02 | 0.005 | [0.009; 0.029] |
Workload (per % of expected maximal workload) | −0.02 | 0.006 | [−0.026; −0.005] |
Risk of depression (PHQ-2 score, per point increase) | 0.30 | 0.10 | [0.11; 0.50] |
Quality of life (KVL-H social score, per point increase) | 0.24 | 0.12 | [0.0048; 0.4728] |
Covariates . | Estimate . | SE . | 95% CI . |
---|---|---|---|
Intercept | −7.87 | 1.24 | [−10.30; −5.45] |
Age (per year) | 0.09 | 0.011 | [0.064; 0.11] |
Being highly educated | −1.09 | 0.21 | [−1.51; −0.68] |
Distance to hospital (per kilometre) | −0.02 | 0.010 | [−0.0424; −0.0028] |
Smoking | 2.34 | 0.11 | [1.68; 2.99] |
Previously diagnosed hypertension | −0.42 | 0.19 | [−0.799; −0.037] |
Positive family history for CVD | 0.56 | 0.20 | [0.16; 0.96] |
CABG (as compared with PCI) | 0.48 | 0.23 | [0.031; 0.922] |
Systolic blood pressure (per mmHg) | 0.02 | 0.005 | [0.009; 0.029] |
Workload (per % of expected maximal workload) | −0.02 | 0.006 | [−0.026; −0.005] |
Risk of depression (PHQ-2 score, per point increase) | 0.30 | 0.10 | [0.11; 0.50] |
Quality of life (KVL-H social score, per point increase) | 0.24 | 0.12 | [0.0048; 0.4728] |
The displayed effects (in the logit scale) were all statistically significant (P < 0.05 after pooling the 20 imputations) in the Multivariable Logistic Regression analysis. The backward elimination excluded the following variables from the final analysis: gender, employment, civil state (living together), waiting time for CR intake, diagnosis, duration of hospitalization, Charlson Comorbidity Index, body mass index, waist circumference, adherence to physical activity and exercise guidelines, quality of life (total, emotional, and physical subscales), GAD-7 score, PHQ-9 score, previously diagnosed hypercholesterolaemia, and previously diagnosed diabetes mellitus. The intercept effect is modelling the probability that a non-highly educated, non-smoking patient, with no family history of CVD, and no previously diagnosed hypertension, who underwent PCI, did not participate in the CTR programme.
CABG, coronary artery bypass grafting; CTR, cardiac telerehabilitation; CVD, cardiovascular disease; KVL-H, Kwaliteit van Leven bij Hartpatiënten (quality of life in cardiac patients; higher scores indicate better quality of life); PCI, percutaneous coronary intervention; PHQ, patient health questionnaire (higher scores indicate higher risk of depression).
Discussion
The aim of this study was to evaluate which patient-related characteristics influenced participation in the SmartCare-CAD trial, which served as proxy for participation in a CTR programme. We observed that higher age, lower educational level, shorter travelling distance to the nearest hospital, smoking, absence of previously diagnosed hypertension, positive family history for CVD, having undergone CABG (as compared with PCI), and higher blood pressure, worse exercise capacity, higher risk of depression, and better QoL (social subscale) before the start of CR were independently associated with non-participation in the SmartCare-CAD trial. The most frequently reported reasons for non-participation in the trial were insufficient technical skills or lack of interest in digital health, preference for centre-based CR, (trial) participation being too burdensome, and not being convinced of the added value of CTR.
Factors influencing trial participation
The results of this study are comparable with previous studies that evaluated factors predicting participation in CTR, telemedicine, or use of eHealth. Although involving different patient populations, these studies also showed a lower tendency towards usage of CTR or other eHealth interventions in patients who had higher age, lower educational levels, and lower monthly incomes.22–25,35–37 Higher age and lower educational levels are both associated with lower digital health literacy,26,38 which likely affects acceptance and use of eHealth interventions,38 as was apparent from the reasons for non-participation in our study. It is, however, highly important that CTR programmes appeal to elderly patients as they are underrepresented in centre-based CR,7,8 and CTR could resolve utilization barriers of centre-based CR that are important for elderly patients, such as transportation issues. Moreover, results from the Eu-CaRE trial39 demonstrated that a CTR intervention in elderly patients, who declined participation in centre-based CR, resulted in improved peak oxygen uptake as compared with no CR. Lower educational levels are associated with worse prognosis, even after participating in a CR programme.40 As not participating in CR further worsens their risk of mortality and morbidity, the participation of elderly patients and those with lower educational levels in CR or CTR is essential.
We observed that smoking, higher blood pressure, worse exercise capacity, and higher risk of depression before the start of CR negatively affected trial participation. This indicates that non-participants generally had worse cardiovascular risk profiles as compared with participants. We could not find similar associations in previous studies, although Fang et al.22 reported lower daily physical activity in CR patients who did not accept CTR. Possibly, lower exercise capacity and increased risk of depression in non-participants in our study were related to higher comorbidity rates (as indicated by a statistically significant difference in the distribution of the Charlson Comorbidity Index), which may have also influenced trial participation. Although Torrent-Sellens et al.37 reported that internet users with chronic diseases were more likely to use eHealth,lower exercise capacity, and the presence of cardiovascular risk factors and comorbidities (both physical and mental) in our study population may have led to an increased demand for supervised, centre-based exercise sessions and the involvement of other professionals such as psychologists and dieticians (who could only provide centre-based counselling). This increased demand for supervised therapy may have also caused CABG (as compared with PCI) to be an independent predictor of non-participation. However, the amount of time spent on direct supervision of patients did not differ between CTR and centre-based CR in SmartCare-CAD,15 indicating that the amount of supervision is not reduced when participating in CTR. Moreover, as CTR has also been proven to be effective in higher-risk patients with more comorbidities, such as patients with heart failure,6,14 tailoring of CTR interventions to these populations should be pursued.
Reasons for non-participation
Among the most frequently reported reasons for non-participation in the SmartCare-CAD trial were insufficient technical skills, a preference for centre-based CR, and not being convinced of the added value of CTR, together accounting for more than half of the refusals. It should be noted here that the amount of patients that did not participate in CTR due to insufficient technical skills is probably underestimated due to the upfront exclusion of patients without an internet connection or mobile phone. A recent survey on the use of technology in CR during the COVID-19 pandemic similarly reported that a lack of patient confidence with the technology used was a major barrier for its use.41 Patients may believe that by participating in CTR they receive a treatment inferior to centre-based CR, which may also be influenced by a (lack of) endorsement by their clinicians and relatives.12 These results indicate that current CTR interventions do not comply with patients’ individual needs, expectations and (digital) competences. To improve the acceptance and use of CTR interventions, these interventions should be adapted to patients’ experiences with digital health interventions, combined with adequate (human) support and training of patients with limited digital competences. This is particularly important because if interventions such as CTR are not tailored to a broad spectrum of patients, their implementation may worsen instead of improve (digital) health equity, as patients underrepresented in conventional CR (e.g. elderly, those with low socioeconomic status) often have limited access to digital healthcare.42,43
Strengths and limitations
To our knowledge, this is the first study evaluating which patient-related characteristics influenced participation in a CTR trial. We performed a comprehensive analysis on a large and representative sample of patients eligible for participation in CTR. In our analysis, we did not focus on a pre-specified set of potential factors but instead investigated a wide spectrum of explanatory factors including—but not limited to—those which have been proposed in the literature. Apart from similarities with comparable trials, we found new factors to be associated with non-participation in CTR, demonstrating the added value of a comprehensive analysis.
This study has two important limitations. First, participation in a clinical trial evaluating the effectiveness of CTR is different from participation in a CTR intervention that is part of regular care. As many factors (e.g. demographic factors, costs, altruism, and perceived personal gain/risk), most of which were not taken into account in our study, influence the decision to participate in a clinical trial,44 this may have influenced patients’ decisions on participation in our study. Still, as only 13% of non-participants refused to participate because they found (trial) participation too burdensome, we believe the patient sample is representative and our results provide important insights in reasons for CTR non-participation. Second, we did not register which patients were not eligible for participation in the SmartCare-CAD trial and/or were not informed about the trial. In the recruitment period for SmartCare-CAD, ∼1500 patients were referred for CR because of CAD and participated in the exercise training module of the CR programme. Given the inclusion of 699 patients in the substudy, this leaves a gap of ∼800 patients who were either not eligible for participation in SmartCare-CAD, were not informed about the trial, or of whom it was not registered that they refused to participate in the trial. Although we believe that we have included a representative patient population in the substudy, the underestimated number of non-participants might have affected the results of our analyses.
Implications for practice
As stated before, increasing the overall use of CR programmes is a major challenge. In order to exploit CTR’s potential to increase overall CR participation rates, current CTR interventions should be tailored to appeal to elderly patients and patients with lower educational levels, lower digital literacy, lower exercise capacity, and patients who underwent CABG. In order to increase the acceptance and use of novel CTR interventions, their redesign should include several aspects. First and foremost, patients and healthcare professionals should be actively involved in order to build and evaluate new interventions based on their needs, expectations, and (digital) competences. Joensson et al.45 used such participatory design to develop a web application for the delivery of CTR in heart failure patients. In this study, however, patients who evaluated the design and usability of the application were relatively young and 80% of patients were highly educated; to account for a wide spectrum of potential users, patients of different age groups, educational levels, and with a variation of comorbidities should be included in this process. Second, current CTR interventions are relatively one-dimensional as they solely focus on home-based exercise training. Although exercise training is a crucial part of CR, patients in whom other components of CR (e.g. dietary counselling, smoking cessation) are at least as important as exercise training may not be interested in current CTR programmes. Redesigned CTR interventions should encompass all CR core components in order to appeal to a broad spectrum of patients. Third, as patients often report that in CTR they missed the social aspects and support of centre-based training,41 new CTR interventions should enable participants to interact with other participants. This could be achieved not only by facilitating direct communication (e.g. by e-mail, messaging, or videoconferencing) but also by including elements of gamification, in which competition elements and rewards for healthy behaviour do not only encourage the adoption of a healthy lifestyle but also enable social interaction with other patients. These social interactions may also provide highly needed (technical) support for patients with limited digital competences.
Conclusion
Participation in CTR is strongly influenced by demographic and health-related factors such as age, educational level, smoking status, and both physical and mental functioning. As these factors reflect the factors that contribute to non-participation in conventional CR, the implementation of current CTR interventions may only result in limited increases in overall participation and adherence to CR. In order to increase CTR use in daily practice and to improve health equity through digital innovations, CTR interventions should be redesigned taking into account the needs and competences of a wide spectrum of cardiovascular patients, especially subgroups that are underrepresented in current centre-based CR and CTR interventions.
Lead author biography
Rutger Brouwers is a cardiologist in training at Catharina Hospital Eindhoven and Máxima Medical Center Eindhoven/Veldhoven, and PhD candidate at Eindhoven University of Technology under the supervision of Dr Hareld Kemps. His research focuses on the effectiveness and cost-effectiveness of cardiac telerehabilitation in patients with coronary artery disease.
Acknowledgements
We would like to thank all patients who participated in the SmartCare-CAD trial and its substudy, and all cardiac rehabilitation professionals and students at Máxima Medical Center who contributed to the realization of the trial.
Funding
This study was part of the SmartCare project, which was partially funded under the ICT Policy Support Programme (ICT PSP), grant agreement number 325158.
Conflict of interest: The Authors declare that there is no conflict of interest.
Data availability
The data underlying this article will be shared on reasonable request to the corresponding author.
References
British Heart Foundation. National Audit of Cardiac Rehabilitation (NACR) Quality and Outcomes Report 2019. Birmingham: British Heart Foundation;
Committee for Cardiovascular Prevention and Cardiac Rehabilitation of the Netherlands Society of Cardiology and Landelijk Multidisciplinair Overleg Hartrevalidatie.
Committee for Cardiovascular Prevention and Cardiac Rehabilitation of the Netherlands Society of Cardiology and Landelijk Multidisciplinair Overleg Hartrevalidatie.