Abstract
Climate change has become a serious issue, and tracing climate events from historical records could be a solution to find a way to deal with it. This study conducted two experiments for classifying metrological text data—one unsupervised method for exploring a solution in the lack of labeled data and another supervised method for achieving high-performance classification. Both experiments took the meteorological text records as material in the early Qing Dynasty (1644 C.E. to 1795 C.E.) from the REACHES database. We also integrated the classification results to develop a Spatio-Temporal research platform with an instant response front-end interface to help humanity researchers access and analyze data according to the three dimensions of time, area, and event categories. With our Spatio-Temporal research platform, we had the ability with ease to analyze the meteorological records during 1650 C.E. to 1700 C.E., the late stage of the Little Ice Age, to investigate the phenomenon of climate change in the Qing Dynasty of China. We will continue to expand the capacity of the database and establish a mature Spatio-Temporal research platform in the future.
1 Introduction
The impacts of climate change have become more and more apparent. Understanding its cause and effect to mitigate its deleterious consequences has become an important research topic. Many clues leading to climate disasters can be traced by observing past meteorological records documented in historical materials. The East Asian Historical Climate Database (Wang et al., 2018) (simplified as REACHES database), categorized chorographies and official histories from A Compendium of Chinese Meteorological Records of the Last 3,000 Years (here we simplified as the Compendium) (Zhang, 2004) into 27 main categories under four domains—Meteorology, Hazard, Unusual phenomena, and Others. The classification system shows interactions or relationships between each category that could be further investigated, making a significant contribution to the analysis of the temporal and spatial characteristics of meteorological phenomena.
Inspired by REACHES, we conducted two experiments on classifying metrological text data–one unsupervised method for exploring a solution in the lack of labeled data, and another supervised method for achieving high-performance classification—with the aim of categorizing a large corpus of data efficiently. Both experiments took the meteorological text records in the early Qing Dynasty (1644 C.E. to 1795 C.E.) from the REACHES database. We then integrated the classification results with a map and a timeline to develop a Spatio-Temporal search interface that facilitates climatologists to analyze data according to time, area, and meteorological categories.
2 Unsupervised Methodology
The first experiment of this study was meteorological text clustering (Chinea-Rios et al., 2015; Hammouda et al., 2004; Kotlerman et al., 2012; Wang et al., 2018). The experiment was composed of three steps: pre-processing, text representation generation, and k-means clustering (MacQueen, 1967). We took 36,123 historical meteorological records from the REACHES database as our input data. As shown in Table 1, each record contains six fields. To decrease the noise of text data which seems not related to meteorological semantic information, we first pre-processed input data, including (1) Replacing characters in descriptions about GanZhi—also called Sexagenary Cycle, a traditional numbering method of time in East Asia, with a character g. Year, Month, and Number expressions were replaced by y, m, and n, respectively. (2) Removing place names and punctuation symbols because they have nothing to do with classification. (3) Some descriptions mentioned multiple categories, and some are long, so we separated each description by periods into sentences to make it possible to distinguish multiple meteorological information from each description, and decrease text information bias.
Table 1The records from the East Asian Historical Climate Database. REACHES has only the record information without meteorological descriptions, these descriptions in Table 1, 4, 5, 6, 8, 11 were all the experts from the Compendium.
ID
. | Year
. | Province
. | County/
. | Meteorological description
. | Source
. |
---|
city
. |
---|
1795-11 | 1663 | Anhui Province | Guichi County | 秋, 池州大水, 城井行舟。【鄉父老云:與明萬曆三十六年水相似。】 | Volume twenty-nine of ‘Chi-zhou Local Gazetteers’, published in Kang-xi period (1711) in Qing Dynasty |
Flood in Chi-zhou in autumn. Villagers said, ‘The flood is similar to the one happened in Wanli period in Ming Dynasty (1608).’ |
1795-12 | 1663 | Anhui Province | Huangshan City | 七月望, 邑令陳恭備建學官, 是日雷雨大作, 千山如注, 田間水數尺, 忽浮飛木於縣東門。 | Volume eight of ‘Tai-ping County record’, published in Chia-ching period in Qing Dynasty |
In mid of July when Chen Gong, the county magistrate, was going to build a school, a thunderstorm happened. Flood was a few feets high, and floodwoods flew at the east gate of the county. |
1795-13 | 1663 | Anhui Province | Shitai County | 秋大水, 父老云:與萬曆三十六年水勢相似。 | Volume two of ‘Shi-di Local Gazetteers’, published in Kang-xi period in Qing Dynasty |
Old man commented about the autumn flood: ‘It was said to be similar to the one happened in the Wanli period in Ming Dynasty (1608).’ |
1795-14 | 1663 | Anhui Province | Dongzhi County | 秋大水, 十一月始退。 | Volume seven of ‘Dong-Liu Local Gazetteers’, published in Qian-long period in Qing Dynasty |
The autumn flood began to recede in November. |
1795-15 | 1663 | Jiangxi Province | Jiujiang | 大水, 潰堤數處, 禾黍盡沒。 | Volume fifty-three of ‘De-Hwa Local Gazetteers’, published in Tong-Zhi period in Qing Dynasty |
Flood broke levees and crops were all be drowned. |
1795-16 | 1663 | Jiangxi Province | Ruichang County | 秋八月, 大水入城。 | Volume one of ‘Rui-Chang Local Gazetteers’, published in Kang-xi period in Qing Dynasty |
Flood crashed the city in August. |
ID
. | Year
. | Province
. | County/
. | Meteorological description
. | Source
. |
---|
city
. |
---|
1795-11 | 1663 | Anhui Province | Guichi County | 秋, 池州大水, 城井行舟。【鄉父老云:與明萬曆三十六年水相似。】 | Volume twenty-nine of ‘Chi-zhou Local Gazetteers’, published in Kang-xi period (1711) in Qing Dynasty |
Flood in Chi-zhou in autumn. Villagers said, ‘The flood is similar to the one happened in Wanli period in Ming Dynasty (1608).’ |
1795-12 | 1663 | Anhui Province | Huangshan City | 七月望, 邑令陳恭備建學官, 是日雷雨大作, 千山如注, 田間水數尺, 忽浮飛木於縣東門。 | Volume eight of ‘Tai-ping County record’, published in Chia-ching period in Qing Dynasty |
In mid of July when Chen Gong, the county magistrate, was going to build a school, a thunderstorm happened. Flood was a few feets high, and floodwoods flew at the east gate of the county. |
1795-13 | 1663 | Anhui Province | Shitai County | 秋大水, 父老云:與萬曆三十六年水勢相似。 | Volume two of ‘Shi-di Local Gazetteers’, published in Kang-xi period in Qing Dynasty |
Old man commented about the autumn flood: ‘It was said to be similar to the one happened in the Wanli period in Ming Dynasty (1608).’ |
1795-14 | 1663 | Anhui Province | Dongzhi County | 秋大水, 十一月始退。 | Volume seven of ‘Dong-Liu Local Gazetteers’, published in Qian-long period in Qing Dynasty |
The autumn flood began to recede in November. |
1795-15 | 1663 | Jiangxi Province | Jiujiang | 大水, 潰堤數處, 禾黍盡沒。 | Volume fifty-three of ‘De-Hwa Local Gazetteers’, published in Tong-Zhi period in Qing Dynasty |
Flood broke levees and crops were all be drowned. |
1795-16 | 1663 | Jiangxi Province | Ruichang County | 秋八月, 大水入城。 | Volume one of ‘Rui-Chang Local Gazetteers’, published in Kang-xi period in Qing Dynasty |
Flood crashed the city in August. |
Table 1The records from the East Asian Historical Climate Database. REACHES has only the record information without meteorological descriptions, these descriptions in Table 1, 4, 5, 6, 8, 11 were all the experts from the Compendium.
ID
. | Year
. | Province
. | County/
. | Meteorological description
. | Source
. |
---|
city
. |
---|
1795-11 | 1663 | Anhui Province | Guichi County | 秋, 池州大水, 城井行舟。【鄉父老云:與明萬曆三十六年水相似。】 | Volume twenty-nine of ‘Chi-zhou Local Gazetteers’, published in Kang-xi period (1711) in Qing Dynasty |
Flood in Chi-zhou in autumn. Villagers said, ‘The flood is similar to the one happened in Wanli period in Ming Dynasty (1608).’ |
1795-12 | 1663 | Anhui Province | Huangshan City | 七月望, 邑令陳恭備建學官, 是日雷雨大作, 千山如注, 田間水數尺, 忽浮飛木於縣東門。 | Volume eight of ‘Tai-ping County record’, published in Chia-ching period in Qing Dynasty |
In mid of July when Chen Gong, the county magistrate, was going to build a school, a thunderstorm happened. Flood was a few feets high, and floodwoods flew at the east gate of the county. |
1795-13 | 1663 | Anhui Province | Shitai County | 秋大水, 父老云:與萬曆三十六年水勢相似。 | Volume two of ‘Shi-di Local Gazetteers’, published in Kang-xi period in Qing Dynasty |
Old man commented about the autumn flood: ‘It was said to be similar to the one happened in the Wanli period in Ming Dynasty (1608).’ |
1795-14 | 1663 | Anhui Province | Dongzhi County | 秋大水, 十一月始退。 | Volume seven of ‘Dong-Liu Local Gazetteers’, published in Qian-long period in Qing Dynasty |
The autumn flood began to recede in November. |
1795-15 | 1663 | Jiangxi Province | Jiujiang | 大水, 潰堤數處, 禾黍盡沒。 | Volume fifty-three of ‘De-Hwa Local Gazetteers’, published in Tong-Zhi period in Qing Dynasty |
Flood broke levees and crops were all be drowned. |
1795-16 | 1663 | Jiangxi Province | Ruichang County | 秋八月, 大水入城。 | Volume one of ‘Rui-Chang Local Gazetteers’, published in Kang-xi period in Qing Dynasty |
Flood crashed the city in August. |
ID
. | Year
. | Province
. | County/
. | Meteorological description
. | Source
. |
---|
city
. |
---|
1795-11 | 1663 | Anhui Province | Guichi County | 秋, 池州大水, 城井行舟。【鄉父老云:與明萬曆三十六年水相似。】 | Volume twenty-nine of ‘Chi-zhou Local Gazetteers’, published in Kang-xi period (1711) in Qing Dynasty |
Flood in Chi-zhou in autumn. Villagers said, ‘The flood is similar to the one happened in Wanli period in Ming Dynasty (1608).’ |
1795-12 | 1663 | Anhui Province | Huangshan City | 七月望, 邑令陳恭備建學官, 是日雷雨大作, 千山如注, 田間水數尺, 忽浮飛木於縣東門。 | Volume eight of ‘Tai-ping County record’, published in Chia-ching period in Qing Dynasty |
In mid of July when Chen Gong, the county magistrate, was going to build a school, a thunderstorm happened. Flood was a few feets high, and floodwoods flew at the east gate of the county. |
1795-13 | 1663 | Anhui Province | Shitai County | 秋大水, 父老云:與萬曆三十六年水勢相似。 | Volume two of ‘Shi-di Local Gazetteers’, published in Kang-xi period in Qing Dynasty |
Old man commented about the autumn flood: ‘It was said to be similar to the one happened in the Wanli period in Ming Dynasty (1608).’ |
1795-14 | 1663 | Anhui Province | Dongzhi County | 秋大水, 十一月始退。 | Volume seven of ‘Dong-Liu Local Gazetteers’, published in Qian-long period in Qing Dynasty |
The autumn flood began to recede in November. |
1795-15 | 1663 | Jiangxi Province | Jiujiang | 大水, 潰堤數處, 禾黍盡沒。 | Volume fifty-three of ‘De-Hwa Local Gazetteers’, published in Tong-Zhi period in Qing Dynasty |
Flood broke levees and crops were all be drowned. |
1795-16 | 1663 | Jiangxi Province | Ruichang County | 秋八月, 大水入城。 | Volume one of ‘Rui-Chang Local Gazetteers’, published in Kang-xi period in Qing Dynasty |
Flood crashed the city in August. |
We used these meteorological text data and the Ming Record (明實錄) to train a 200-dimensional word2vec model (Mikolov et al., 2013). We decided to use one Chinese character as a word in the word2vec algorithm, converted each sentence written in Classical Chinese into embedding vectors by averaging their character embeddings, and then used the k-means algorithm to divide all embedded vectors into k groups (Qian et al., 2004; Wang et al., 2008). We constructed the validation set to find the most suitable k value of 300, and evaluated the clustering results with 9,530 labeled records. The true-positive, false-positive, and false-negative numbers of each event classification are calculated to get the total precision, recall, and F1-score, see Table 2.
Table 2The precision, recall, and F1-score of our k-means model
Precision
. | Recall
. | F1-score
. |
---|
0.874 | 0.647 | 0.744 |
Precision
. | Recall
. | F1-score
. |
---|
0.874 | 0.647 | 0.744 |
Table 2The precision, recall, and F1-score of our k-means model
Precision
. | Recall
. | F1-score
. |
---|
0.874 | 0.647 | 0.744 |
Precision
. | Recall
. | F1-score
. |
---|
0.874 | 0.647 | 0.744 |
From Table 3, we could see that many clusters corresponded to the same climatic category, but after careful examination, these groups were still slightly different. Take Clusters 0 (see Table 4), 9 (see Table 5), and 95 (see Table 6) as examples. Even though these three clusters could be roughly classified as flood hazards, it was found that most of the texts of Cluster 95 referred to seasons. The records of Cluster 9 mostly referred to floods killing people and damaging villages, while the records of the other two groups do not.
Table 3The 300 clusters and their semantics. The categorization system of the REACHES database was composed of a hierarchical structure. In order to simplify the evaluating processes, we corresponded each cluster to the most related maincategory of the REACHES categorization system.
Classification
. | Cluster numbers
. | Classification
. | Cluster numbers
. |
---|
-98 Undetermined | 10, 40, 67, 82, 200, 212, 246, 266, 272, 292 | 32 Pests | 25, 32, 50, 94, 121, 124, 129, 141, 156, 169, 188, 190, 216, 222, 251, 269, 294, 299 |
10 Rain | 5, 20, 24, 26, 27, 37, 44, 46, 47, 48, 49, 54, 71, 77, 84, 86, 99, 110, 111, 116, 126, 142, 143, 159, 164, 174, 187, 197, 206, 207, 208, 225, 226, 228, 232, 234, 237, 242, 247, 249, 262, 273, 276, 280, 281, 284, 289, 291 | 33 Crop failure | 8, 16, 18, 29, 33, 34, 55, 56, 65, 79, 87, 88, 92, 103, 105, 115, 120, 123, 132, 147, 153, 163, 172, 178, 179, 194, 201, 202, 223, 231, 243, 256, 258, 268, 271, 278, 279, 282 |
11 Temperature | 113, 118, 146, 193, 220, 224, 233 | 34 Disease | 35, 62, 70, 81, 96, 104, 109, 221, 263 |
12 Visually impaired phenomenon | 57, 184 | 35 Famine | 1, 15, 31, 53, 59, 68, 85, 135, 136, 152, 158, 170, 171, 186, 195, 204, 255, 265, 295 |
13 Thunder | 7, 12, 108, 139, 166, 240 | 50 Geographic phenomenon | 161, 225, 291 |
14 Light | 191 | 56 Falling objects | 168 |
15 Wind | 2, 6, 60, 73, 91, 119, 128, 150, 161, 175, 189, 287, 297 | 62 Abnormal astronomy | 102 |
16 Cloud | 3, 107, 114, 167, 182 | 65 Animals and plants | 180, 203, 274, 283 |
30 Drought | 14, 19, 30, 36, 69, 83, 101, 125, 134, 148, 149, 160, 162, 196, 205, 209, 211, 213, 218, 229, 230, 236, 239 | 71 Social problem | 17, 21, 22, 23, 39. 41, 43, 52, 58, 61, 64, 66, 72, 74,75, 78, 80, 98, 112, 127, 130, 133, 151, 154, 155, 157, 173, 181, 217, 238, 245, 248, 260, 275, 277, 285, 288, 290, 293, 296, 298 |
31 Flood | 0, 4, 9, 11, 13, 23, 28, 38, 42, 45, 51, 63, 76, 90, 93, 95, 117, 122, 131, 137, 138, 176, 177, 183, 185, 192, 198, 199, 210, 214, 215, 219, 227, 235, 241, 250, 252, 253, 254, 259, 264, 267, 270 | 95 Others | 106 |
Classification
. | Cluster numbers
. | Classification
. | Cluster numbers
. |
---|
-98 Undetermined | 10, 40, 67, 82, 200, 212, 246, 266, 272, 292 | 32 Pests | 25, 32, 50, 94, 121, 124, 129, 141, 156, 169, 188, 190, 216, 222, 251, 269, 294, 299 |
10 Rain | 5, 20, 24, 26, 27, 37, 44, 46, 47, 48, 49, 54, 71, 77, 84, 86, 99, 110, 111, 116, 126, 142, 143, 159, 164, 174, 187, 197, 206, 207, 208, 225, 226, 228, 232, 234, 237, 242, 247, 249, 262, 273, 276, 280, 281, 284, 289, 291 | 33 Crop failure | 8, 16, 18, 29, 33, 34, 55, 56, 65, 79, 87, 88, 92, 103, 105, 115, 120, 123, 132, 147, 153, 163, 172, 178, 179, 194, 201, 202, 223, 231, 243, 256, 258, 268, 271, 278, 279, 282 |
11 Temperature | 113, 118, 146, 193, 220, 224, 233 | 34 Disease | 35, 62, 70, 81, 96, 104, 109, 221, 263 |
12 Visually impaired phenomenon | 57, 184 | 35 Famine | 1, 15, 31, 53, 59, 68, 85, 135, 136, 152, 158, 170, 171, 186, 195, 204, 255, 265, 295 |
13 Thunder | 7, 12, 108, 139, 166, 240 | 50 Geographic phenomenon | 161, 225, 291 |
14 Light | 191 | 56 Falling objects | 168 |
15 Wind | 2, 6, 60, 73, 91, 119, 128, 150, 161, 175, 189, 287, 297 | 62 Abnormal astronomy | 102 |
16 Cloud | 3, 107, 114, 167, 182 | 65 Animals and plants | 180, 203, 274, 283 |
30 Drought | 14, 19, 30, 36, 69, 83, 101, 125, 134, 148, 149, 160, 162, 196, 205, 209, 211, 213, 218, 229, 230, 236, 239 | 71 Social problem | 17, 21, 22, 23, 39. 41, 43, 52, 58, 61, 64, 66, 72, 74,75, 78, 80, 98, 112, 127, 130, 133, 151, 154, 155, 157, 173, 181, 217, 238, 245, 248, 260, 275, 277, 285, 288, 290, 293, 296, 298 |
31 Flood | 0, 4, 9, 11, 13, 23, 28, 38, 42, 45, 51, 63, 76, 90, 93, 95, 117, 122, 131, 137, 138, 176, 177, 183, 185, 192, 198, 199, 210, 214, 215, 219, 227, 235, 241, 250, 252, 253, 254, 259, 264, 267, 270 | 95 Others | 106 |
Table 3The 300 clusters and their semantics. The categorization system of the REACHES database was composed of a hierarchical structure. In order to simplify the evaluating processes, we corresponded each cluster to the most related maincategory of the REACHES categorization system.
Classification
. | Cluster numbers
. | Classification
. | Cluster numbers
. |
---|
-98 Undetermined | 10, 40, 67, 82, 200, 212, 246, 266, 272, 292 | 32 Pests | 25, 32, 50, 94, 121, 124, 129, 141, 156, 169, 188, 190, 216, 222, 251, 269, 294, 299 |
10 Rain | 5, 20, 24, 26, 27, 37, 44, 46, 47, 48, 49, 54, 71, 77, 84, 86, 99, 110, 111, 116, 126, 142, 143, 159, 164, 174, 187, 197, 206, 207, 208, 225, 226, 228, 232, 234, 237, 242, 247, 249, 262, 273, 276, 280, 281, 284, 289, 291 | 33 Crop failure | 8, 16, 18, 29, 33, 34, 55, 56, 65, 79, 87, 88, 92, 103, 105, 115, 120, 123, 132, 147, 153, 163, 172, 178, 179, 194, 201, 202, 223, 231, 243, 256, 258, 268, 271, 278, 279, 282 |
11 Temperature | 113, 118, 146, 193, 220, 224, 233 | 34 Disease | 35, 62, 70, 81, 96, 104, 109, 221, 263 |
12 Visually impaired phenomenon | 57, 184 | 35 Famine | 1, 15, 31, 53, 59, 68, 85, 135, 136, 152, 158, 170, 171, 186, 195, 204, 255, 265, 295 |
13 Thunder | 7, 12, 108, 139, 166, 240 | 50 Geographic phenomenon | 161, 225, 291 |
14 Light | 191 | 56 Falling objects | 168 |
15 Wind | 2, 6, 60, 73, 91, 119, 128, 150, 161, 175, 189, 287, 297 | 62 Abnormal astronomy | 102 |
16 Cloud | 3, 107, 114, 167, 182 | 65 Animals and plants | 180, 203, 274, 283 |
30 Drought | 14, 19, 30, 36, 69, 83, 101, 125, 134, 148, 149, 160, 162, 196, 205, 209, 211, 213, 218, 229, 230, 236, 239 | 71 Social problem | 17, 21, 22, 23, 39. 41, 43, 52, 58, 61, 64, 66, 72, 74,75, 78, 80, 98, 112, 127, 130, 133, 151, 154, 155, 157, 173, 181, 217, 238, 245, 248, 260, 275, 277, 285, 288, 290, 293, 296, 298 |
31 Flood | 0, 4, 9, 11, 13, 23, 28, 38, 42, 45, 51, 63, 76, 90, 93, 95, 117, 122, 131, 137, 138, 176, 177, 183, 185, 192, 198, 199, 210, 214, 215, 219, 227, 235, 241, 250, 252, 253, 254, 259, 264, 267, 270 | 95 Others | 106 |
Classification
. | Cluster numbers
. | Classification
. | Cluster numbers
. |
---|
-98 Undetermined | 10, 40, 67, 82, 200, 212, 246, 266, 272, 292 | 32 Pests | 25, 32, 50, 94, 121, 124, 129, 141, 156, 169, 188, 190, 216, 222, 251, 269, 294, 299 |
10 Rain | 5, 20, 24, 26, 27, 37, 44, 46, 47, 48, 49, 54, 71, 77, 84, 86, 99, 110, 111, 116, 126, 142, 143, 159, 164, 174, 187, 197, 206, 207, 208, 225, 226, 228, 232, 234, 237, 242, 247, 249, 262, 273, 276, 280, 281, 284, 289, 291 | 33 Crop failure | 8, 16, 18, 29, 33, 34, 55, 56, 65, 79, 87, 88, 92, 103, 105, 115, 120, 123, 132, 147, 153, 163, 172, 178, 179, 194, 201, 202, 223, 231, 243, 256, 258, 268, 271, 278, 279, 282 |
11 Temperature | 113, 118, 146, 193, 220, 224, 233 | 34 Disease | 35, 62, 70, 81, 96, 104, 109, 221, 263 |
12 Visually impaired phenomenon | 57, 184 | 35 Famine | 1, 15, 31, 53, 59, 68, 85, 135, 136, 152, 158, 170, 171, 186, 195, 204, 255, 265, 295 |
13 Thunder | 7, 12, 108, 139, 166, 240 | 50 Geographic phenomenon | 161, 225, 291 |
14 Light | 191 | 56 Falling objects | 168 |
15 Wind | 2, 6, 60, 73, 91, 119, 128, 150, 161, 175, 189, 287, 297 | 62 Abnormal astronomy | 102 |
16 Cloud | 3, 107, 114, 167, 182 | 65 Animals and plants | 180, 203, 274, 283 |
30 Drought | 14, 19, 30, 36, 69, 83, 101, 125, 134, 148, 149, 160, 162, 196, 205, 209, 211, 213, 218, 229, 230, 236, 239 | 71 Social problem | 17, 21, 22, 23, 39. 41, 43, 52, 58, 61, 64, 66, 72, 74,75, 78, 80, 98, 112, 127, 130, 133, 151, 154, 155, 157, 173, 181, 217, 238, 245, 248, 260, 275, 277, 285, 288, 290, 293, 296, 298 |
31 Flood | 0, 4, 9, 11, 13, 23, 28, 38, 42, 45, 51, 63, 76, 90, 93, 95, 117, 122, 131, 137, 138, 176, 177, 183, 185, 192, 198, 199, 210, 214, 215, 219, 227, 235, 241, 250, 252, 253, 254, 259, 264, 267, 270 | 95 Others | 106 |
Table 4Examples of sentences in Cluster 0
免八分水災 |
Tax exemption for areas with level 8 floods. |
河水為災 |
Disasters caused by river flooding. |
秋, 濁漳橫溢, 南宮被水災 |
The turbid Zhang water flooded in autumn, and Nangong suffered floods. |
八月初五日, (莆田)水災, 漳、泉更甚 |
On the fifth day of August, floods occurred in the Putian area, and the floods in Zhangzhou and Quanzhou were more serious. |
是年直隸水、旱、雹災, 免賦有差 |
Floods, droughts, and hail (hail disasters) occurred in Zhili (province) that year, so different taxation policies on individual districts were exempted. |
先被雹災, 後被水災 |
The local area was hit by hailstorms first, and then by floods. |
五月十三日, 洪水為災 |
May 13th, the flood became a disaster. |
秋, 大水成災 |
A massive flood in autumn led to a disaster. |
秋大水, 圩田災 |
Flooding in autumn led to a disaster, destroying lowland farmland. |
免八分水災 |
Tax exemption for areas with level 8 floods. |
河水為災 |
Disasters caused by river flooding. |
秋, 濁漳橫溢, 南宮被水災 |
The turbid Zhang water flooded in autumn, and Nangong suffered floods. |
八月初五日, (莆田)水災, 漳、泉更甚 |
On the fifth day of August, floods occurred in the Putian area, and the floods in Zhangzhou and Quanzhou were more serious. |
是年直隸水、旱、雹災, 免賦有差 |
Floods, droughts, and hail (hail disasters) occurred in Zhili (province) that year, so different taxation policies on individual districts were exempted. |
先被雹災, 後被水災 |
The local area was hit by hailstorms first, and then by floods. |
五月十三日, 洪水為災 |
May 13th, the flood became a disaster. |
秋, 大水成災 |
A massive flood in autumn led to a disaster. |
秋大水, 圩田災 |
Flooding in autumn led to a disaster, destroying lowland farmland. |
Table 4Examples of sentences in Cluster 0
免八分水災 |
Tax exemption for areas with level 8 floods. |
河水為災 |
Disasters caused by river flooding. |
秋, 濁漳橫溢, 南宮被水災 |
The turbid Zhang water flooded in autumn, and Nangong suffered floods. |
八月初五日, (莆田)水災, 漳、泉更甚 |
On the fifth day of August, floods occurred in the Putian area, and the floods in Zhangzhou and Quanzhou were more serious. |
是年直隸水、旱、雹災, 免賦有差 |
Floods, droughts, and hail (hail disasters) occurred in Zhili (province) that year, so different taxation policies on individual districts were exempted. |
先被雹災, 後被水災 |
The local area was hit by hailstorms first, and then by floods. |
五月十三日, 洪水為災 |
May 13th, the flood became a disaster. |
秋, 大水成災 |
A massive flood in autumn led to a disaster. |
秋大水, 圩田災 |
Flooding in autumn led to a disaster, destroying lowland farmland. |
免八分水災 |
Tax exemption for areas with level 8 floods. |
河水為災 |
Disasters caused by river flooding. |
秋, 濁漳橫溢, 南宮被水災 |
The turbid Zhang water flooded in autumn, and Nangong suffered floods. |
八月初五日, (莆田)水災, 漳、泉更甚 |
On the fifth day of August, floods occurred in the Putian area, and the floods in Zhangzhou and Quanzhou were more serious. |
是年直隸水、旱、雹災, 免賦有差 |
Floods, droughts, and hail (hail disasters) occurred in Zhili (province) that year, so different taxation policies on individual districts were exempted. |
先被雹災, 後被水災 |
The local area was hit by hailstorms first, and then by floods. |
五月十三日, 洪水為災 |
May 13th, the flood became a disaster. |
秋, 大水成災 |
A massive flood in autumn led to a disaster. |
秋大水, 圩田災 |
Flooding in autumn led to a disaster, destroying lowland farmland. |
Table 5Examples of sentences in Cluster 9
群蛟湧出, 平地水深數尺, 民畜飄蕩, 三十九都、四十都舉宅沉溺者不計其處, 所過山崩地陷, 石積沙壅, 高下翻悉成水國 |
Flash floods caused water to accumulate on the ground by several feet, and villagers and livestock were washed away by the water. There are countless families in the Thirty-Nine Capital and Forty Capital areas where the whose houses were flooded. In the area where the flood flowed, the rocks collapsed, the land subsided, and the loess gravel piled up, turning it into a water town. |
大水, 平地深丈餘, 禽獸死者無算 |
Flooding caused water to accumulate on the ground by several meters (a unit of height), and countless animals died. |
河決荊隆口, 清河以西平地水深丈餘, 村落漂沒無遺, 至十一年乃息 |
The Yellow River burst the dyke at Jinglongkou. The flat land on the west side of the Qinghe River was covered with water several meters height, and the villages were flooded. It was until the eleventh year that the disaster eased. |
大水, 北城幾陷, 壞田廬無數, 民溺死者眾 |
The flood covered most of the North City, damaging countless farmland and drowned many civilians. |
河決朱源塞, 毀民田廬幾盡 |
The Yellow River burst the dyke at Zhuyuan Pass, almost completely destroying the houses and farmland. |
六月宣政鄉蛟出, 淹死居民 |
A flash flood broke out in Xuanzheng Township in June, drowning many residents. |
秋大水, 決西城而過, 人多淹溺 |
A massive flood in autumn broke the dike in Xicheng, flooding many villagers. |
秋月有蛟出, 壞民田舍 |
A flash flood broke out in autumn, damaging farmland and houses. |
七月, 大水, 四壩盡淹, 居民徙入山避之 |
Floods occurred in July, and the four dams were submerged. Therefore, residents migrated to the mountains to avoid the floods. |
群蛟湧出, 平地水深數尺, 民畜飄蕩, 三十九都、四十都舉宅沉溺者不計其處, 所過山崩地陷, 石積沙壅, 高下翻悉成水國 |
Flash floods caused water to accumulate on the ground by several feet, and villagers and livestock were washed away by the water. There are countless families in the Thirty-Nine Capital and Forty Capital areas where the whose houses were flooded. In the area where the flood flowed, the rocks collapsed, the land subsided, and the loess gravel piled up, turning it into a water town. |
大水, 平地深丈餘, 禽獸死者無算 |
Flooding caused water to accumulate on the ground by several meters (a unit of height), and countless animals died. |
河決荊隆口, 清河以西平地水深丈餘, 村落漂沒無遺, 至十一年乃息 |
The Yellow River burst the dyke at Jinglongkou. The flat land on the west side of the Qinghe River was covered with water several meters height, and the villages were flooded. It was until the eleventh year that the disaster eased. |
大水, 北城幾陷, 壞田廬無數, 民溺死者眾 |
The flood covered most of the North City, damaging countless farmland and drowned many civilians. |
河決朱源塞, 毀民田廬幾盡 |
The Yellow River burst the dyke at Zhuyuan Pass, almost completely destroying the houses and farmland. |
六月宣政鄉蛟出, 淹死居民 |
A flash flood broke out in Xuanzheng Township in June, drowning many residents. |
秋大水, 決西城而過, 人多淹溺 |
A massive flood in autumn broke the dike in Xicheng, flooding many villagers. |
秋月有蛟出, 壞民田舍 |
A flash flood broke out in autumn, damaging farmland and houses. |
七月, 大水, 四壩盡淹, 居民徙入山避之 |
Floods occurred in July, and the four dams were submerged. Therefore, residents migrated to the mountains to avoid the floods. |
Table 5Examples of sentences in Cluster 9
群蛟湧出, 平地水深數尺, 民畜飄蕩, 三十九都、四十都舉宅沉溺者不計其處, 所過山崩地陷, 石積沙壅, 高下翻悉成水國 |
Flash floods caused water to accumulate on the ground by several feet, and villagers and livestock were washed away by the water. There are countless families in the Thirty-Nine Capital and Forty Capital areas where the whose houses were flooded. In the area where the flood flowed, the rocks collapsed, the land subsided, and the loess gravel piled up, turning it into a water town. |
大水, 平地深丈餘, 禽獸死者無算 |
Flooding caused water to accumulate on the ground by several meters (a unit of height), and countless animals died. |
河決荊隆口, 清河以西平地水深丈餘, 村落漂沒無遺, 至十一年乃息 |
The Yellow River burst the dyke at Jinglongkou. The flat land on the west side of the Qinghe River was covered with water several meters height, and the villages were flooded. It was until the eleventh year that the disaster eased. |
大水, 北城幾陷, 壞田廬無數, 民溺死者眾 |
The flood covered most of the North City, damaging countless farmland and drowned many civilians. |
河決朱源塞, 毀民田廬幾盡 |
The Yellow River burst the dyke at Zhuyuan Pass, almost completely destroying the houses and farmland. |
六月宣政鄉蛟出, 淹死居民 |
A flash flood broke out in Xuanzheng Township in June, drowning many residents. |
秋大水, 決西城而過, 人多淹溺 |
A massive flood in autumn broke the dike in Xicheng, flooding many villagers. |
秋月有蛟出, 壞民田舍 |
A flash flood broke out in autumn, damaging farmland and houses. |
七月, 大水, 四壩盡淹, 居民徙入山避之 |
Floods occurred in July, and the four dams were submerged. Therefore, residents migrated to the mountains to avoid the floods. |
群蛟湧出, 平地水深數尺, 民畜飄蕩, 三十九都、四十都舉宅沉溺者不計其處, 所過山崩地陷, 石積沙壅, 高下翻悉成水國 |
Flash floods caused water to accumulate on the ground by several feet, and villagers and livestock were washed away by the water. There are countless families in the Thirty-Nine Capital and Forty Capital areas where the whose houses were flooded. In the area where the flood flowed, the rocks collapsed, the land subsided, and the loess gravel piled up, turning it into a water town. |
大水, 平地深丈餘, 禽獸死者無算 |
Flooding caused water to accumulate on the ground by several meters (a unit of height), and countless animals died. |
河決荊隆口, 清河以西平地水深丈餘, 村落漂沒無遺, 至十一年乃息 |
The Yellow River burst the dyke at Jinglongkou. The flat land on the west side of the Qinghe River was covered with water several meters height, and the villages were flooded. It was until the eleventh year that the disaster eased. |
大水, 北城幾陷, 壞田廬無數, 民溺死者眾 |
The flood covered most of the North City, damaging countless farmland and drowned many civilians. |
河決朱源塞, 毀民田廬幾盡 |
The Yellow River burst the dyke at Zhuyuan Pass, almost completely destroying the houses and farmland. |
六月宣政鄉蛟出, 淹死居民 |
A flash flood broke out in Xuanzheng Township in June, drowning many residents. |
秋大水, 決西城而過, 人多淹溺 |
A massive flood in autumn broke the dike in Xicheng, flooding many villagers. |
秋月有蛟出, 壞民田舍 |
A flash flood broke out in autumn, damaging farmland and houses. |
七月, 大水, 四壩盡淹, 居民徙入山避之 |
Floods occurred in July, and the four dams were submerged. Therefore, residents migrated to the mountains to avoid the floods. |
Table 6Examples of sentences in Cluster 95
春大水 |
Floods happened in spring. |
秋水, 大風 |
Floods came in autumn with strong winds. |
秋大水, 儉收 |
In autumn, floods happened, causing taxes to be reduced. |
夏大水, 秋復大水 |
Flooding occurred in summer, and again in autumn. |
夏秋大水 |
Floods occurred in summer and autumn. |
秋漲大發 |
Floods in autumn skyrocketed. |
秋大水, 賑 |
Flooding occurred in autumn, so disaster relief was carried out. |
秋, 金鄉、魚臺大水 |
In autumn, floods occurred in Jinxiang and Yutai areas. |
春大水 |
Floods happened in spring. |
秋水, 大風 |
Floods came in autumn with strong winds. |
秋大水, 儉收 |
In autumn, floods happened, causing taxes to be reduced. |
夏大水, 秋復大水 |
Flooding occurred in summer, and again in autumn. |
夏秋大水 |
Floods occurred in summer and autumn. |
秋漲大發 |
Floods in autumn skyrocketed. |
秋大水, 賑 |
Flooding occurred in autumn, so disaster relief was carried out. |
秋, 金鄉、魚臺大水 |
In autumn, floods occurred in Jinxiang and Yutai areas. |
Table 6Examples of sentences in Cluster 95
春大水 |
Floods happened in spring. |
秋水, 大風 |
Floods came in autumn with strong winds. |
秋大水, 儉收 |
In autumn, floods happened, causing taxes to be reduced. |
夏大水, 秋復大水 |
Flooding occurred in summer, and again in autumn. |
夏秋大水 |
Floods occurred in summer and autumn. |
秋漲大發 |
Floods in autumn skyrocketed. |
秋大水, 賑 |
Flooding occurred in autumn, so disaster relief was carried out. |
秋, 金鄉、魚臺大水 |
In autumn, floods occurred in Jinxiang and Yutai areas. |
春大水 |
Floods happened in spring. |
秋水, 大風 |
Floods came in autumn with strong winds. |
秋大水, 儉收 |
In autumn, floods happened, causing taxes to be reduced. |
夏大水, 秋復大水 |
Flooding occurred in summer, and again in autumn. |
夏秋大水 |
Floods occurred in summer and autumn. |
秋漲大發 |
Floods in autumn skyrocketed. |
秋大水, 賑 |
Flooding occurred in autumn, so disaster relief was carried out. |
秋, 金鄉、魚臺大水 |
In autumn, floods occurred in Jinxiang and Yutai areas. |
3 Supervised Methodology
In the first experiment, we found that it was possible to help researchers generalize the data or even collect training instances through the above demonstrated unsupervised machine learning approach. In the second experiment, we changed our target to build a high-performance classifier by training a multi-label classifier based on a supervised method using BERT–Bidirectional Encoder Representation from Transformers (Devlin et al., 2018). BERT is a two-step deep learning framework composed of pre-training and fine-tuning procedures. Since we designed the model with multi-label outputs, separating each description into sentences in advance for better clustering results became unnecessary. We utilized 9,530 labeled records without splitting each description into sentences, evenly extracted 80% from each category for training and 20% for evaluation. Because some of the main categories lacked training instances, we merged similar ones and turned them into twenty-four categories (see Table 7).
Table 7Twenty-four category labels after merging some categories derived from REACHES
Code
. | Category
. | Amount
. | Code
. | Category
. | Amount
. |
---|
10 | Precipitation | 2,203 | 34 | Disease | 176 |
11 | Temperature | 412 | 35 | Famine | 1,083 |
12 | Visibility | 91 | 50 | Geophysical abnormities | 454 |
13 | Thunder, Lighting | 236 | 53_56_57_58 | Precipitation abnormities | 42 |
(color, plants, animal, metal) |
14 | Optical | 43 | 59 | Acoustical abnormities | 43 |
15 | Wind | 686 | 62 | Sun-related phenomena | 167 |
16 | Cloud | 52 | 63_64 | Moon-and-Astral-related phenomena | 19 |
17 | Gas, Air | 26 | 62_63_64 | Astronomical phenomena | 185 |
30 | Drought | 1,924 | 65 | Plant abnormities | 68 |
31 | Flood | 2,224 | 68 | Animal abnormities | 194 |
32 | Pest/Vermin | 653 | 71 | Socioeconomic turmoil | 2,754 |
33 | Crops | 3,032 | −99_-98_95 | Others, unrecognized vocabularies and unclear descriptions | 155 |
Code
. | Category
. | Amount
. | Code
. | Category
. | Amount
. |
---|
10 | Precipitation | 2,203 | 34 | Disease | 176 |
11 | Temperature | 412 | 35 | Famine | 1,083 |
12 | Visibility | 91 | 50 | Geophysical abnormities | 454 |
13 | Thunder, Lighting | 236 | 53_56_57_58 | Precipitation abnormities | 42 |
(color, plants, animal, metal) |
14 | Optical | 43 | 59 | Acoustical abnormities | 43 |
15 | Wind | 686 | 62 | Sun-related phenomena | 167 |
16 | Cloud | 52 | 63_64 | Moon-and-Astral-related phenomena | 19 |
17 | Gas, Air | 26 | 62_63_64 | Astronomical phenomena | 185 |
30 | Drought | 1,924 | 65 | Plant abnormities | 68 |
31 | Flood | 2,224 | 68 | Animal abnormities | 194 |
32 | Pest/Vermin | 653 | 71 | Socioeconomic turmoil | 2,754 |
33 | Crops | 3,032 | −99_-98_95 | Others, unrecognized vocabularies and unclear descriptions | 155 |
Table 7Twenty-four category labels after merging some categories derived from REACHES
Code
. | Category
. | Amount
. | Code
. | Category
. | Amount
. |
---|
10 | Precipitation | 2,203 | 34 | Disease | 176 |
11 | Temperature | 412 | 35 | Famine | 1,083 |
12 | Visibility | 91 | 50 | Geophysical abnormities | 454 |
13 | Thunder, Lighting | 236 | 53_56_57_58 | Precipitation abnormities | 42 |
(color, plants, animal, metal) |
14 | Optical | 43 | 59 | Acoustical abnormities | 43 |
15 | Wind | 686 | 62 | Sun-related phenomena | 167 |
16 | Cloud | 52 | 63_64 | Moon-and-Astral-related phenomena | 19 |
17 | Gas, Air | 26 | 62_63_64 | Astronomical phenomena | 185 |
30 | Drought | 1,924 | 65 | Plant abnormities | 68 |
31 | Flood | 2,224 | 68 | Animal abnormities | 194 |
32 | Pest/Vermin | 653 | 71 | Socioeconomic turmoil | 2,754 |
33 | Crops | 3,032 | −99_-98_95 | Others, unrecognized vocabularies and unclear descriptions | 155 |
Code
. | Category
. | Amount
. | Code
. | Category
. | Amount
. |
---|
10 | Precipitation | 2,203 | 34 | Disease | 176 |
11 | Temperature | 412 | 35 | Famine | 1,083 |
12 | Visibility | 91 | 50 | Geophysical abnormities | 454 |
13 | Thunder, Lighting | 236 | 53_56_57_58 | Precipitation abnormities | 42 |
(color, plants, animal, metal) |
14 | Optical | 43 | 59 | Acoustical abnormities | 43 |
15 | Wind | 686 | 62 | Sun-related phenomena | 167 |
16 | Cloud | 52 | 63_64 | Moon-and-Astral-related phenomena | 19 |
17 | Gas, Air | 26 | 62_63_64 | Astronomical phenomena | 185 |
30 | Drought | 1,924 | 65 | Plant abnormities | 68 |
31 | Flood | 2,224 | 68 | Animal abnormities | 194 |
32 | Pest/Vermin | 653 | 71 | Socioeconomic turmoil | 2,754 |
33 | Crops | 3,032 | −99_-98_95 | Others, unrecognized vocabularies and unclear descriptions | 155 |
We adopted the pre-trained Chinese BERT language model to encode the text descriptions and formed the labels in training instances into a series of binary-decision problems as in Table 8. Set the batch size to thirty-two, the learning rate to 1e-5, and the max length of each sentence no longer than 255 characters with character-based segmentation.
Table 8The data format of training and evaluating sets. Each paragraph may containmultiple climate-relative events.
ID
. | meteorological description
. | -99_-98_95
. | 10
. | 11
. | 12
. | 13
. | 14
. | 15
. | 16
. | 17
. | 30
. | 31
. | 32
. | 33
. | 34
. | 35
. | 50
. | 53_56_57_58
. | 59
. | 62
. | 63_64
. | 62_63_64
. | 65
. | 68
. | 71
. |
---|
2545-15-0 | 秋被旱, 勘不成災, 蠲免丁米一次。 | 1 | 0 | 0 | 0 | 0 | 0 | 0 | 0 | 0 | 1 | 0 | 0 | 0 | 0 | 0 | 0 | 0 | 0 | 0 | 0 | 0 | 0 | 0 | 1 |
1851-20-2 | 其大堤決時, 眾見大火如球, 旋轉堤上, 火焰蓬勃, 少頃雨雹疾擊, 人畜號聲震野。 | 1 | 1 | 0 | 0 | 0 | 0 | 0 | 0 | 0 | 0 | 0 | 0 | 0 | 0 | 0 | 0 | 0 | 0 | 0 | 0 | 0 | 0 | 1 | 1 |
1825-33-2 | 十一月, 有四龍見於西南。 | 1 | 0 | 0 | 0 | 0 | 0 | 0 | 0 | 0 | 0 | 0 | 0 | 0 | 0 | 0 | 0 | 0 | 0 | 0 | 0 | 0 | 0 | 0 | 0 |
2371-26-3 | 十月上諭:山西大同、朔平二府上年偶被旱災, 將上年成災之大同、懷仁、渾源、應州、山陰、廣靈、陽高、靈丘、天丘、朔州、馬邑, 並未成災而收成亦歉之右玉、左雲、平魯等十四州縣 | 1 | 0 | 0 | 0 | 0 | 0 | 0 | 0 | 0 | 1 | 0 | 0 | 1 | 0 | 0 | 0 | 0 | 0 | 0 | 0 | 0 | 0 | 0 | 0 |
2374-04-1 | 覆准上、下兩江地方被災銀米兼賑, 災輕州縣每米一石折銀一兩, 其被災較重之上江宿州等六安縣加賑月分, 折價散給每米一石加增二錢。 | 1 | 0 | 0 | 0 | 0 | 0 | 0 | 0 | 0 | 0 | 0 | 0 | 0 | 0 | 0 | 0 | 0 | 0 | 0 | 0 | 0 | 0 | 0 | 1 |
ID
. | meteorological description
. | -99_-98_95
. | 10
. | 11
. | 12
. | 13
. | 14
. | 15
. | 16
. | 17
. | 30
. | 31
. | 32
. | 33
. | 34
. | 35
. | 50
. | 53_56_57_58
. | 59
. | 62
. | 63_64
. | 62_63_64
. | 65
. | 68
. | 71
. |
---|
2545-15-0 | 秋被旱, 勘不成災, 蠲免丁米一次。 | 1 | 0 | 0 | 0 | 0 | 0 | 0 | 0 | 0 | 1 | 0 | 0 | 0 | 0 | 0 | 0 | 0 | 0 | 0 | 0 | 0 | 0 | 0 | 1 |
1851-20-2 | 其大堤決時, 眾見大火如球, 旋轉堤上, 火焰蓬勃, 少頃雨雹疾擊, 人畜號聲震野。 | 1 | 1 | 0 | 0 | 0 | 0 | 0 | 0 | 0 | 0 | 0 | 0 | 0 | 0 | 0 | 0 | 0 | 0 | 0 | 0 | 0 | 0 | 1 | 1 |
1825-33-2 | 十一月, 有四龍見於西南。 | 1 | 0 | 0 | 0 | 0 | 0 | 0 | 0 | 0 | 0 | 0 | 0 | 0 | 0 | 0 | 0 | 0 | 0 | 0 | 0 | 0 | 0 | 0 | 0 |
2371-26-3 | 十月上諭:山西大同、朔平二府上年偶被旱災, 將上年成災之大同、懷仁、渾源、應州、山陰、廣靈、陽高、靈丘、天丘、朔州、馬邑, 並未成災而收成亦歉之右玉、左雲、平魯等十四州縣 | 1 | 0 | 0 | 0 | 0 | 0 | 0 | 0 | 0 | 1 | 0 | 0 | 1 | 0 | 0 | 0 | 0 | 0 | 0 | 0 | 0 | 0 | 0 | 0 |
2374-04-1 | 覆准上、下兩江地方被災銀米兼賑, 災輕州縣每米一石折銀一兩, 其被災較重之上江宿州等六安縣加賑月分, 折價散給每米一石加增二錢。 | 1 | 0 | 0 | 0 | 0 | 0 | 0 | 0 | 0 | 0 | 0 | 0 | 0 | 0 | 0 | 0 | 0 | 0 | 0 | 0 | 0 | 0 | 0 | 1 |
Table 8The data format of training and evaluating sets. Each paragraph may containmultiple climate-relative events.
ID
. | meteorological description
. | -99_-98_95
. | 10
. | 11
. | 12
. | 13
. | 14
. | 15
. | 16
. | 17
. | 30
. | 31
. | 32
. | 33
. | 34
. | 35
. | 50
. | 53_56_57_58
. | 59
. | 62
. | 63_64
. | 62_63_64
. | 65
. | 68
. | 71
. |
---|
2545-15-0 | 秋被旱, 勘不成災, 蠲免丁米一次。 | 1 | 0 | 0 | 0 | 0 | 0 | 0 | 0 | 0 | 1 | 0 | 0 | 0 | 0 | 0 | 0 | 0 | 0 | 0 | 0 | 0 | 0 | 0 | 1 |
1851-20-2 | 其大堤決時, 眾見大火如球, 旋轉堤上, 火焰蓬勃, 少頃雨雹疾擊, 人畜號聲震野。 | 1 | 1 | 0 | 0 | 0 | 0 | 0 | 0 | 0 | 0 | 0 | 0 | 0 | 0 | 0 | 0 | 0 | 0 | 0 | 0 | 0 | 0 | 1 | 1 |
1825-33-2 | 十一月, 有四龍見於西南。 | 1 | 0 | 0 | 0 | 0 | 0 | 0 | 0 | 0 | 0 | 0 | 0 | 0 | 0 | 0 | 0 | 0 | 0 | 0 | 0 | 0 | 0 | 0 | 0 |
2371-26-3 | 十月上諭:山西大同、朔平二府上年偶被旱災, 將上年成災之大同、懷仁、渾源、應州、山陰、廣靈、陽高、靈丘、天丘、朔州、馬邑, 並未成災而收成亦歉之右玉、左雲、平魯等十四州縣 | 1 | 0 | 0 | 0 | 0 | 0 | 0 | 0 | 0 | 1 | 0 | 0 | 1 | 0 | 0 | 0 | 0 | 0 | 0 | 0 | 0 | 0 | 0 | 0 |
2374-04-1 | 覆准上、下兩江地方被災銀米兼賑, 災輕州縣每米一石折銀一兩, 其被災較重之上江宿州等六安縣加賑月分, 折價散給每米一石加增二錢。 | 1 | 0 | 0 | 0 | 0 | 0 | 0 | 0 | 0 | 0 | 0 | 0 | 0 | 0 | 0 | 0 | 0 | 0 | 0 | 0 | 0 | 0 | 0 | 1 |
ID
. | meteorological description
. | -99_-98_95
. | 10
. | 11
. | 12
. | 13
. | 14
. | 15
. | 16
. | 17
. | 30
. | 31
. | 32
. | 33
. | 34
. | 35
. | 50
. | 53_56_57_58
. | 59
. | 62
. | 63_64
. | 62_63_64
. | 65
. | 68
. | 71
. |
---|
2545-15-0 | 秋被旱, 勘不成災, 蠲免丁米一次。 | 1 | 0 | 0 | 0 | 0 | 0 | 0 | 0 | 0 | 1 | 0 | 0 | 0 | 0 | 0 | 0 | 0 | 0 | 0 | 0 | 0 | 0 | 0 | 1 |
1851-20-2 | 其大堤決時, 眾見大火如球, 旋轉堤上, 火焰蓬勃, 少頃雨雹疾擊, 人畜號聲震野。 | 1 | 1 | 0 | 0 | 0 | 0 | 0 | 0 | 0 | 0 | 0 | 0 | 0 | 0 | 0 | 0 | 0 | 0 | 0 | 0 | 0 | 0 | 1 | 1 |
1825-33-2 | 十一月, 有四龍見於西南。 | 1 | 0 | 0 | 0 | 0 | 0 | 0 | 0 | 0 | 0 | 0 | 0 | 0 | 0 | 0 | 0 | 0 | 0 | 0 | 0 | 0 | 0 | 0 | 0 |
2371-26-3 | 十月上諭:山西大同、朔平二府上年偶被旱災, 將上年成災之大同、懷仁、渾源、應州、山陰、廣靈、陽高、靈丘、天丘、朔州、馬邑, 並未成災而收成亦歉之右玉、左雲、平魯等十四州縣 | 1 | 0 | 0 | 0 | 0 | 0 | 0 | 0 | 0 | 1 | 0 | 0 | 1 | 0 | 0 | 0 | 0 | 0 | 0 | 0 | 0 | 0 | 0 | 0 |
2374-04-1 | 覆准上、下兩江地方被災銀米兼賑, 災輕州縣每米一石折銀一兩, 其被災較重之上江宿州等六安縣加賑月分, 折價散給每米一石加增二錢。 | 1 | 0 | 0 | 0 | 0 | 0 | 0 | 0 | 0 | 0 | 0 | 0 | 0 | 0 | 0 | 0 | 0 | 0 | 0 | 0 | 0 | 0 | 0 | 1 |
We evaluated the classification model in every twenty epochs to see the results in Table 9, calculated both the micro and macro metrics for every event label, using precision, recall, and F1-measure. Finally received a high performance of 96.7% micro F1-score.
Table 9Evaluation results of each round of training
Epochs
. | Micro precision
. | Micro recall
. | Micro F1
. | Macro precision
. | Macro recall
. | Macro F1
. |
---|
20 | 0.938 | 0.904 | 0.938 | 0.736 | 0.519 | 0.557 |
40 | 0.970 | 0.957 | 0.964 | 0.948 | 0.856 | 0.896 |
60 | 0.967 | 0.967 | 0.967 | 0.928 | 0.905 | 0.907 |
80 | 0.968 | 0.966 | 0.967 | 0.929 | 0.902 | 0.906 |
Epochs
. | Micro precision
. | Micro recall
. | Micro F1
. | Macro precision
. | Macro recall
. | Macro F1
. |
---|
20 | 0.938 | 0.904 | 0.938 | 0.736 | 0.519 | 0.557 |
40 | 0.970 | 0.957 | 0.964 | 0.948 | 0.856 | 0.896 |
60 | 0.967 | 0.967 | 0.967 | 0.928 | 0.905 | 0.907 |
80 | 0.968 | 0.966 | 0.967 | 0.929 | 0.902 | 0.906 |
Table 9Evaluation results of each round of training
Epochs
. | Micro precision
. | Micro recall
. | Micro F1
. | Macro precision
. | Macro recall
. | Macro F1
. |
---|
20 | 0.938 | 0.904 | 0.938 | 0.736 | 0.519 | 0.557 |
40 | 0.970 | 0.957 | 0.964 | 0.948 | 0.856 | 0.896 |
60 | 0.967 | 0.967 | 0.967 | 0.928 | 0.905 | 0.907 |
80 | 0.968 | 0.966 | 0.967 | 0.929 | 0.902 | 0.906 |
Epochs
. | Micro precision
. | Micro recall
. | Micro F1
. | Macro precision
. | Macro recall
. | Macro F1
. |
---|
20 | 0.938 | 0.904 | 0.938 | 0.736 | 0.519 | 0.557 |
40 | 0.970 | 0.957 | 0.964 | 0.948 | 0.856 | 0.896 |
60 | 0.967 | 0.967 | 0.967 | 0.928 | 0.905 | 0.907 |
80 | 0.968 | 0.966 | 0.967 | 0.929 | 0.902 | 0.906 |
4 Front-End Interface
We presented meteorological events on a map interface based on the year and location of the climate events in the historical meteorological records, providing a platform for researchers to easily find desired data (see Fig. 1).1 The main features of the interface include a scrolling timeline, a pop-up condition selection window, and an instant response map. When a user selects the conditions, the map will correspondingly display the records satisfying the conditions. If the cursor hovers over a location tag on the map, the map on the page below will show all meteorological records of the location within the timeline interval.
5 Case Study
To show the usage of our platform, we looked into meteorological records from 1650 C.E. to 1700 C.E. in our database, which is the late stage of the Little Ice Age, to investigate the phenomenon of climate change in the Qing Dynasty of China (Chu, 1973; Solomon et al., 2007). First, we chose the Temperature event category. Among these records, there are sixty-eight extreme cold climate records. We could see that this kind of phenomenon was located from the tropical zone to the tepid zone in China (see Fig. 2). Therefore, we can conclude that extreme cold records appear in the middle- to high-latitude areas and lower latitude areas.
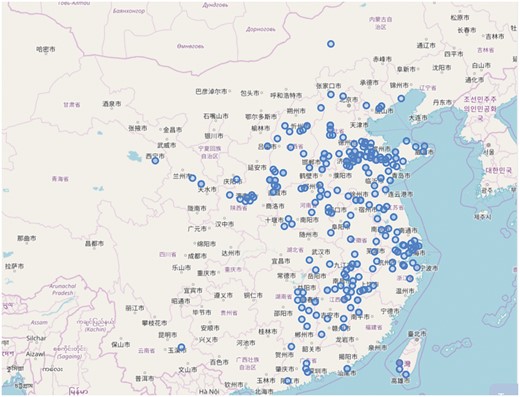
Fig. 2
Areas with low-temperature record from 1650 C.E. to 1700 C.E.
Extreme cold weather usually comes with disasters. We included the Rain category as our variables in Step 2. Among these records, there were 379 records related to Snowfall. To deeply look into the records, besides directly collecting the data of meteorological phenomena of Snowfall, we further collected the indirect meteorological phenomena data, such as ‘three days in a row’, ‘frost-damaged trees’, etc. (see Table 10). From Table 10, we can see that during the Little Ice Age (1650 C.E. to 1700 C.E.), the freezing climate led to disasters more frequently than that during the Non-Little Ice Age (1745 C.E. to 1795 C.E.).
Table 10Numbers of data related to snow during Little Ice Age and Non-Little Ice Age in the rain category
Meteorological phenomena data
. | Little Ice Age
. | Non-Little Ice Age(1745–1795)
. |
---|
(1650–1700)
. |
---|
Rain | 3,014 | 1,618 |
Snow | 379 | 119 |
Snow, more than 10 days/month/10 days in a row three days in a row or more | 46 | 5 |
Snow, frost damaged trees | 8 | 2 |
Snow, birds, animals, and human beings freeze to death | 17 | 6 |
Meteorological phenomena data
. | Little Ice Age
. | Non-Little Ice Age(1745–1795)
. |
---|
(1650–1700)
. |
---|
Rain | 3,014 | 1,618 |
Snow | 379 | 119 |
Snow, more than 10 days/month/10 days in a row three days in a row or more | 46 | 5 |
Snow, frost damaged trees | 8 | 2 |
Snow, birds, animals, and human beings freeze to death | 17 | 6 |
Table 10Numbers of data related to snow during Little Ice Age and Non-Little Ice Age in the rain category
Meteorological phenomena data
. | Little Ice Age
. | Non-Little Ice Age(1745–1795)
. |
---|
(1650–1700)
. |
---|
Rain | 3,014 | 1,618 |
Snow | 379 | 119 |
Snow, more than 10 days/month/10 days in a row three days in a row or more | 46 | 5 |
Snow, frost damaged trees | 8 | 2 |
Snow, birds, animals, and human beings freeze to death | 17 | 6 |
Meteorological phenomena data
. | Little Ice Age
. | Non-Little Ice Age(1745–1795)
. |
---|
(1650–1700)
. |
---|
Rain | 3,014 | 1,618 |
Snow | 379 | 119 |
Snow, more than 10 days/month/10 days in a row three days in a row or more | 46 | 5 |
Snow, frost damaged trees | 8 | 2 |
Snow, birds, animals, and human beings freeze to death | 17 | 6 |
When investigating the meteorological phenomena during the Little Ice Age, we found uncommon snowfall records in Taiwan. As shown in Table 11, these records were in terrain areas, such as Chia-Yi county and Tainan City, which could be seen as solid evidence of the extreme climate during the Little Ice Age.
Table 11Uncommon snow records in Taiwan
Year
. | Province
. | County/city
. | Cluster
. | Meteorological description
. | Data sources
. |
---|
1683 | Taiwan Province | – | Temperature (including frost and dew), and rain | 冬十一月, 雨雪。是夜冰堅厚寸餘。從來臺灣無雪無冰, 此異事也。 | Volume ten of ‘Taiwan Local Gazetteers’, published in Kang-xi period (1685) in Qing Dynasty |
In November, winter, it snows and rains. The ice is more than an inch thick that night. It's strange because there has not snowed since ancient times. |
1683 | Taiwan Province | -- | Rain, crop failure, temperature (including frost and dew) | 五月, 大雨。霪雨連月, 鄭氏之土田阡陌多被沖陷, 有“高岸為谷”之歎。冬始雨雪, 冰堅厚寸餘。臺土氣熱, 從無霜雪。 | Volume nine of ‘Rebuilt Taiwan Local Gazetteers’, published in Kang-xi period in Qing Dynasty |
In May, it rained heavily. After a long period of rain, the fields of Zheng's family were crashed, and the high bank became valley. It began to snow in winter, and the ice was more than an inch thick. Normally the field was hot, and there was no frost and snow in Taiwan. |
1683 | Taiwan Province | Chia-yi County | Flood, rain, crop failure, temperature (including frost and dew) | 夏五月, 大雨水, 時霪雨連月, 鄭氏土田多沖陷, 有“高岸為谷”之歎。冬十一月, 始雨雪, 冰堅厚寸餘。諸羅有霜無雪, 是歲甫入版圖, 地氣自北而南, 信有矣。 | Volume twelve of ‘Tsu-lo Local Gazetteers’, published in Kang-xi period in Qing Dynasty |
In May, it rained heavily. After a long period of rain, the fields of Zheng's family were crashed, and the high bank became valley. In November, Winter, it snowed and iced over. Normally the field was hot, and there was no frost and snow in Tsu-lo (ancient name of Taiwan). It was believed that the climate was from Northern area, starting from the year Tso-lo became Qing’s territory. |
1683 | Taiwan Province | Tainan City | Flood, rain, flood, rain, crop failure, temperature (frost and dew), temperature (frost and dew), temperature (frost and dew), drought, rain | 春, 鯽魚潭涸。夏五月, 大雨水, 田園多沖陷。六月, 澎湖潮水漲四尺。秋八月壬子, 鹿耳門潮水漲。冬十有一月, 雨雪冰。是臺地氣暖, 從無霜雪, 是歲八月甫入版圖, 冬遂雨雪, 冰堅寸許, 地氣自北而南, 運屬一統故也。 | ‘Rebuilt Taiwan Local Gazetteers’, published in Qian-long period in Qing Dynasty |
In Spring, crucian pond dried up. In May, Summer, it rained a lot, and fields are mostly crashed. In June, the tide at Penghu rose four feet high. On August 18th, the tide at Luermen rose. In November, Winter, it snowed and iced over. Normally the field was hot, and there was no frost and snow in Taiwan. However, started from August when Taiwan was under Qing’s authority, it rained and snowed in Winter, and the ice was more than an inch thick. The climate was from the Northern Area. It is because of territorial unity. |
Year
. | Province
. | County/city
. | Cluster
. | Meteorological description
. | Data sources
. |
---|
1683 | Taiwan Province | – | Temperature (including frost and dew), and rain | 冬十一月, 雨雪。是夜冰堅厚寸餘。從來臺灣無雪無冰, 此異事也。 | Volume ten of ‘Taiwan Local Gazetteers’, published in Kang-xi period (1685) in Qing Dynasty |
In November, winter, it snows and rains. The ice is more than an inch thick that night. It's strange because there has not snowed since ancient times. |
1683 | Taiwan Province | -- | Rain, crop failure, temperature (including frost and dew) | 五月, 大雨。霪雨連月, 鄭氏之土田阡陌多被沖陷, 有“高岸為谷”之歎。冬始雨雪, 冰堅厚寸餘。臺土氣熱, 從無霜雪。 | Volume nine of ‘Rebuilt Taiwan Local Gazetteers’, published in Kang-xi period in Qing Dynasty |
In May, it rained heavily. After a long period of rain, the fields of Zheng's family were crashed, and the high bank became valley. It began to snow in winter, and the ice was more than an inch thick. Normally the field was hot, and there was no frost and snow in Taiwan. |
1683 | Taiwan Province | Chia-yi County | Flood, rain, crop failure, temperature (including frost and dew) | 夏五月, 大雨水, 時霪雨連月, 鄭氏土田多沖陷, 有“高岸為谷”之歎。冬十一月, 始雨雪, 冰堅厚寸餘。諸羅有霜無雪, 是歲甫入版圖, 地氣自北而南, 信有矣。 | Volume twelve of ‘Tsu-lo Local Gazetteers’, published in Kang-xi period in Qing Dynasty |
In May, it rained heavily. After a long period of rain, the fields of Zheng's family were crashed, and the high bank became valley. In November, Winter, it snowed and iced over. Normally the field was hot, and there was no frost and snow in Tsu-lo (ancient name of Taiwan). It was believed that the climate was from Northern area, starting from the year Tso-lo became Qing’s territory. |
1683 | Taiwan Province | Tainan City | Flood, rain, flood, rain, crop failure, temperature (frost and dew), temperature (frost and dew), temperature (frost and dew), drought, rain | 春, 鯽魚潭涸。夏五月, 大雨水, 田園多沖陷。六月, 澎湖潮水漲四尺。秋八月壬子, 鹿耳門潮水漲。冬十有一月, 雨雪冰。是臺地氣暖, 從無霜雪, 是歲八月甫入版圖, 冬遂雨雪, 冰堅寸許, 地氣自北而南, 運屬一統故也。 | ‘Rebuilt Taiwan Local Gazetteers’, published in Qian-long period in Qing Dynasty |
In Spring, crucian pond dried up. In May, Summer, it rained a lot, and fields are mostly crashed. In June, the tide at Penghu rose four feet high. On August 18th, the tide at Luermen rose. In November, Winter, it snowed and iced over. Normally the field was hot, and there was no frost and snow in Taiwan. However, started from August when Taiwan was under Qing’s authority, it rained and snowed in Winter, and the ice was more than an inch thick. The climate was from the Northern Area. It is because of territorial unity. |
Table 11Uncommon snow records in Taiwan
Year
. | Province
. | County/city
. | Cluster
. | Meteorological description
. | Data sources
. |
---|
1683 | Taiwan Province | – | Temperature (including frost and dew), and rain | 冬十一月, 雨雪。是夜冰堅厚寸餘。從來臺灣無雪無冰, 此異事也。 | Volume ten of ‘Taiwan Local Gazetteers’, published in Kang-xi period (1685) in Qing Dynasty |
In November, winter, it snows and rains. The ice is more than an inch thick that night. It's strange because there has not snowed since ancient times. |
1683 | Taiwan Province | -- | Rain, crop failure, temperature (including frost and dew) | 五月, 大雨。霪雨連月, 鄭氏之土田阡陌多被沖陷, 有“高岸為谷”之歎。冬始雨雪, 冰堅厚寸餘。臺土氣熱, 從無霜雪。 | Volume nine of ‘Rebuilt Taiwan Local Gazetteers’, published in Kang-xi period in Qing Dynasty |
In May, it rained heavily. After a long period of rain, the fields of Zheng's family were crashed, and the high bank became valley. It began to snow in winter, and the ice was more than an inch thick. Normally the field was hot, and there was no frost and snow in Taiwan. |
1683 | Taiwan Province | Chia-yi County | Flood, rain, crop failure, temperature (including frost and dew) | 夏五月, 大雨水, 時霪雨連月, 鄭氏土田多沖陷, 有“高岸為谷”之歎。冬十一月, 始雨雪, 冰堅厚寸餘。諸羅有霜無雪, 是歲甫入版圖, 地氣自北而南, 信有矣。 | Volume twelve of ‘Tsu-lo Local Gazetteers’, published in Kang-xi period in Qing Dynasty |
In May, it rained heavily. After a long period of rain, the fields of Zheng's family were crashed, and the high bank became valley. In November, Winter, it snowed and iced over. Normally the field was hot, and there was no frost and snow in Tsu-lo (ancient name of Taiwan). It was believed that the climate was from Northern area, starting from the year Tso-lo became Qing’s territory. |
1683 | Taiwan Province | Tainan City | Flood, rain, flood, rain, crop failure, temperature (frost and dew), temperature (frost and dew), temperature (frost and dew), drought, rain | 春, 鯽魚潭涸。夏五月, 大雨水, 田園多沖陷。六月, 澎湖潮水漲四尺。秋八月壬子, 鹿耳門潮水漲。冬十有一月, 雨雪冰。是臺地氣暖, 從無霜雪, 是歲八月甫入版圖, 冬遂雨雪, 冰堅寸許, 地氣自北而南, 運屬一統故也。 | ‘Rebuilt Taiwan Local Gazetteers’, published in Qian-long period in Qing Dynasty |
In Spring, crucian pond dried up. In May, Summer, it rained a lot, and fields are mostly crashed. In June, the tide at Penghu rose four feet high. On August 18th, the tide at Luermen rose. In November, Winter, it snowed and iced over. Normally the field was hot, and there was no frost and snow in Taiwan. However, started from August when Taiwan was under Qing’s authority, it rained and snowed in Winter, and the ice was more than an inch thick. The climate was from the Northern Area. It is because of territorial unity. |
Year
. | Province
. | County/city
. | Cluster
. | Meteorological description
. | Data sources
. |
---|
1683 | Taiwan Province | – | Temperature (including frost and dew), and rain | 冬十一月, 雨雪。是夜冰堅厚寸餘。從來臺灣無雪無冰, 此異事也。 | Volume ten of ‘Taiwan Local Gazetteers’, published in Kang-xi period (1685) in Qing Dynasty |
In November, winter, it snows and rains. The ice is more than an inch thick that night. It's strange because there has not snowed since ancient times. |
1683 | Taiwan Province | -- | Rain, crop failure, temperature (including frost and dew) | 五月, 大雨。霪雨連月, 鄭氏之土田阡陌多被沖陷, 有“高岸為谷”之歎。冬始雨雪, 冰堅厚寸餘。臺土氣熱, 從無霜雪。 | Volume nine of ‘Rebuilt Taiwan Local Gazetteers’, published in Kang-xi period in Qing Dynasty |
In May, it rained heavily. After a long period of rain, the fields of Zheng's family were crashed, and the high bank became valley. It began to snow in winter, and the ice was more than an inch thick. Normally the field was hot, and there was no frost and snow in Taiwan. |
1683 | Taiwan Province | Chia-yi County | Flood, rain, crop failure, temperature (including frost and dew) | 夏五月, 大雨水, 時霪雨連月, 鄭氏土田多沖陷, 有“高岸為谷”之歎。冬十一月, 始雨雪, 冰堅厚寸餘。諸羅有霜無雪, 是歲甫入版圖, 地氣自北而南, 信有矣。 | Volume twelve of ‘Tsu-lo Local Gazetteers’, published in Kang-xi period in Qing Dynasty |
In May, it rained heavily. After a long period of rain, the fields of Zheng's family were crashed, and the high bank became valley. In November, Winter, it snowed and iced over. Normally the field was hot, and there was no frost and snow in Tsu-lo (ancient name of Taiwan). It was believed that the climate was from Northern area, starting from the year Tso-lo became Qing’s territory. |
1683 | Taiwan Province | Tainan City | Flood, rain, flood, rain, crop failure, temperature (frost and dew), temperature (frost and dew), temperature (frost and dew), drought, rain | 春, 鯽魚潭涸。夏五月, 大雨水, 田園多沖陷。六月, 澎湖潮水漲四尺。秋八月壬子, 鹿耳門潮水漲。冬十有一月, 雨雪冰。是臺地氣暖, 從無霜雪, 是歲八月甫入版圖, 冬遂雨雪, 冰堅寸許, 地氣自北而南, 運屬一統故也。 | ‘Rebuilt Taiwan Local Gazetteers’, published in Qian-long period in Qing Dynasty |
In Spring, crucian pond dried up. In May, Summer, it rained a lot, and fields are mostly crashed. In June, the tide at Penghu rose four feet high. On August 18th, the tide at Luermen rose. In November, Winter, it snowed and iced over. Normally the field was hot, and there was no frost and snow in Taiwan. However, started from August when Taiwan was under Qing’s authority, it rained and snowed in Winter, and the ice was more than an inch thick. The climate was from the Northern Area. It is because of territorial unity. |
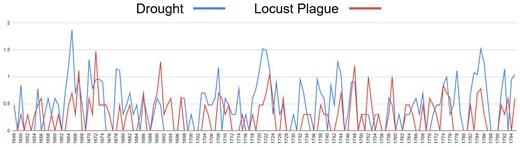
Fig. 3
The number of locust and drought records in Shandong after log-normalization (from 1647 C.E. to 1795 C.E.)
With the help of our platform, users can investigate the relationships between different climate events. Through keywords such as ‘locusts’ and ‘grasshoppers’, we had conducted statistics on the Locust Plague events, and compared them with the Drought events in Shandong Province. Because the frequency has an amplification effect, the intensity of ten times is actually not ten times stronger than that of one time. Therefore, we follow the way used for information retrieval and take the logarithm of frequency+1 to calculate the Pearson correlation coefficient between the log frequency of Locust Plague and that of Drought as shown in Fig. 3. The result is that the correlation coefficient value is 0.4 and significant, indicating that the two have a moderately positive correlation. Judging from the degree of graph overlap, the peaks and troughs almost correspond to each other (peak to peak, trough to trough). We can observe a certain degree of correlation between the occurrence of Drought and Locust Plague based on the number of records in historical texts alone, and it can also echo the saying that ‘the drought followed by the locust plague’. However, the number of locusts is not simply affected by drought. According to the existing references, the combined results of temperature, summer rainfall, and winter length must also be considered. Therefore, we are also actively collecting data such as precipitation and temperature for more detailed analysis of the causes of locust plagues.
6 Conclusion
In order to understand the impact of climate disasters and find a way to deal with them, tracing the historical climate event could be a solution. This study established a principle to classify meteorological phenomena from China's Three Thousand Years of Meteorological Records based on machine learning strategies, developed a Spatio-Temporal research platform, and built an instant response front-end interface. Since language model techniques are developing rapidly, getting good classification results becomes easier with well-prepared training instances. However, producing high-quality training instances is still costly for personal research. Therefore, we proposed a two-stage process of utilizing an unsupervised method to help collect training instances through briefly browsing our text material in the first stage, and then, in the second stage, use a supervised method to get better training performance.
In the climate case study, we collected users’ feedback, improved the front-end interface of our platform, and enhanced the precision of a mass of meteorological data analytics. Although the research platform only contains the meteorological data from 1647 C.E. to 1795 C.E. for now, we hope to expand the capacity of the database and establish a mature Spatio-Temporal research platform in the future.
Funding
This work was supported by the Center for GIS, RCHSS, Acdemia Sinica, Development of Big Historical Text Information Extraction Techniques for Compiling Research-oriented Knowledge Bases under grant AS-ASCDC-110-101, Automatic Wikipedia Article Generation Based on Articles in Other Languages under grant MOST 109-2221-E-008 -058 -MY3.
References
Chinea-Rios
M.
, Sanchis-Trilles
G.
, Casacuberta
F.
(
2015
).
Sentence clustering using continuous vector space representation
.
Paper presented at the Iberian Conference on Pattern Recognition and Image Analysis
.
Chu
K.-c. J. S. S.
(
1973
). A preliminary study on the climatic fluctuations during the last 5,000 years in China.
Scientia Sinica
,
16
(
2
):
226
–
56
.
Devlin
J.
, Chang
M.-W.
, Lee
K.
, Toutanova
K.
(
2018
). Bert: pre-training of deep bidirectional transformers for language understanding.
arXiv preprint arXiv:1810.04805.Hammouda
K. M.
, Kamel
M. S.
(
2004
).
Efficient phrase-based document indexing for Web document clustering
.
IEEE Transactions on Knowledge and Data Engineering
,
16
(
10
):
1279
–
96
. doi:
Ko-Chen
C.
(
1973
).
A preliminary study on the climatic fluctuations during the last 5,000 years in China
.
Scientia Sinica
,
16
(
2
):
226
–
56
.
Kotlerman
L.
, Dagan
I.
, Gorodetsky
M.
, Daya
E.
(
2012a
). Sentence clustering via projection over term clusters. Paper presented at the Proceedings of the First Joint Conference on Lexical and Computational Semantics - Volume 1: Proceedings of the main conference and the shared task, and Volume 2: Proceedings of the Sixth International Workshop on Semantic Evaluation, Montréal, Canada.
Kotlerman
L.
, Dagan
I.
, Gorodetsky
M.
, Daya
E.
(
2012b
). Sentence clustering via projection over term clusters. Paper presented at the SEM 2012: The First Joint Conference on Lexical and Computational Semantics–Volume 1: Proceedings of the main conference and the shared task, and Volume 2: Proceedings of the Sixth International Workshop on Semantic Evaluation (SemEval 2012).
MacQueen
J.
(
1967
). Some methods for classification and analysis of multivariate observations. Paper presented at the Proceedings of the Fifth Berkeley Symposium on Mathematical Statistics and Probability, Volume 1: Statistics, Berkeley, CA.
Mikolov
T.
, Chen
K.
, Corrado
G.
, Dean
J.
(
2013
).
Efficient estimation of word representations in vector space
.
arXiv preprint arXiv
:
1301
.
3781
.
Qian
G.
, Sural
S.
, Gu
Y.
, Pramanik
S.
(
2004
).
Similarity between Euclidean and cosine angle distance for nearest neighbor queries
.
Paper presented at the Proceedings of the 2004 ACM Symposium on Applied Computing
,
Nicosia, Cyprus
.
Solomon
S
, Manning
M
, Marquis
M
, Qin
D.
(
2007
).
Climate Change 2007-The Physical Science Basis: Working Group I Contribution to the Fourth Assessment Report of the IPCC
, Vol.
4
. Cambridge, UK and New York, NY, USA:
Cambridge University Press, p. 996
.
Wang
P. K.
, Lin
K.-H. E.
, Liao
Y.-C.
et al. (
2018
).
Construction of the REACHES climate database based on historical documents of China
.
Scientific Data
,
5
(
1
):
1
–
14
.
Wang
D.
, Li
T.
, Zhu
S.
, Ding
C.
(
2008
). Multi-document summarization via sentence-level semantic analysis and symmetric matrix factorization. Paper presented at the Proceedings of the 31st Annual International ACM SIGIR Conference on Research and Development in Information Retrieval, Singapore.
Zhang
D. e.
(
2004
).
A Compendium of Chinese Meteorological Records of the Last 3,000 Years
.
Nanjing
:
Jiangsu Education Publishing House
.
© The Author(s) 2022. Published by Oxford University Press on behalf of EADH.
This is an Open Access article distributed under the terms of the Creative Commons Attribution License (
https://creativecommons.org/licenses/by/4.0/), which permits unrestricted reuse, distribution, and reproduction in any medium, provided the original work is properly cited.