-
PDF
- Split View
-
Views
-
Cite
Cite
Wenyi Xu, Fangyi Liu, Han Tang, Lijie Tan, Hao Yin, 392. MACHINE LEARNING TO PREDICT LYMPH NODE METASTASIS IN T1 ESOPHAGEAL SQUAMOUS CELL CARCINOMA: A MULTICENTER STUDY, Diseases of the Esophagus, Volume 37, Issue Supplement_1, September 2024, doae057.148, https://doi.org/10.1093/dote/doae057.148
- Share Icon Share
Abstract
Lymph node metastases (LNM) is a relatively uncommon but possible complication of T1 esophageal squamous cell carcinoma (ESCC). Existing models do poorly when it comes to quantifying this risk. As a branch of artificial intelligence, machine learning (ML) algorithms have developed and become more prominent. Up to now, no individualized ML prediction model aimed at predicting LNM in T1 esophageal squamous cell carcinoma patients is available. This study aimed to develop a machine learning model for LNM in patients with T1 esophageal squamous cell carcinoma.
Patients with T1 squamous cell carcinoma treated with surgery between January 2010 and September 2021 from 3 institutions were included. Machine-learning models were developed using data on patients’ age and sex, depth of tumor invasion, tumor size, tumor location, macroscopic tumor type, lymphatic and vascular invasion, and histologic grade. Elastic net regression (ELR), random forest (RF), extreme gradient boosting (XGB), and a combined (ensemble) model of these was generated. Use Area Under Curve (AUC) to evaluate the predictive ability of models. The contribution of each factor was calculated. To meet clinical needs, we designed the model as a user-friendly website.
926 and 341 patients were included in the derivation and validation cohorts. The lymph node metastasis rate was 14.8%. The models all exhibited potent discriminating power. The elastic net regression model performed best with externally validated AUC of 0.803 (AUC 0.773 for RF, 0.791 for XGB, 0.799 for ensemble) whereas the NCCN guidelines identified patients with LNM with an AUC of 0.576 and logistic model with an AUC of 0. 670. The most important features were lymphatic and vascular invasion as well as depth of tumor invasion.
The model created utilizing machine learning approaches had excellent performance estimating the likelihood of LNM in T1 ESCC, which can help patients achieve personalized treatment options.
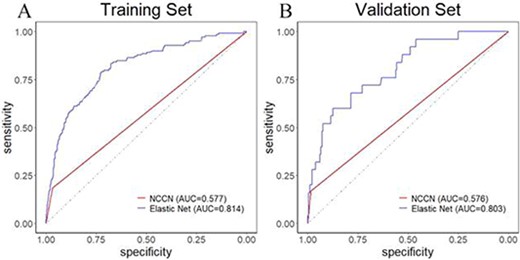