-
PDF
- Split View
-
Views
-
Cite
Cite
Laurence Slama, Benjamin W Barrett, Alison G Abraham, Frank J Palella, Jared W Magnani, Jean Paul Viard, Jordan E Lake, Todd T Brown, for the mulicenter AIDS Cohort Study (MACS), Incident Proteinuria by HIV Serostatus Among Men With Pre–Diabetes Mellitus: The Multicenter AIDS Cohort Study, Clinical Infectious Diseases, Volume 79, Issue 2, 15 August 2024, Pages 469–476, https://doi.org/10.1093/cid/ciae065
- Share Icon Share
Abstract
Pre–diabetes mellitus (DM) is associated with proteinuria, a risk factor for chronic kidney disease. While people with human immunodeficiency virus (HIV; PWH) have a higher risk of proteinuria than people without HIV (PWOH), it is unknown whether incident proteinuria differs by HIV serostatus among prediabetic persons.
The urine protein-to-creatinine ratio was measured at semiannual visits among men in the Multicenter AIDS Cohort Study since April 2006. Men with pre-DM on or after April 2006 and no prevalent proteinuria or use of antidiabetic medications were included. Pre-DM was defined as a fasting glucose level of 100–125 mg/dL confirmed within a year by a repeated fasting glucose or hemoglobin A1c measurement of 5.7%–6.4%. Incident proteinuria was defined as a urine protein-to-creatinine ratio (UPCR) >200 mg/g, confirmed within a year. We used Poisson regression models to determine whether incident proteinuria in participants with pre-DM differed by HIV serostatus and, among PWH, whether HIV-specific factors were related to incident proteinuria.
Between 2006 and 2019, among 1276 men with pre-DM, proteinuria developed in 128 of 613 PWH (21%) and 50 of 663 PWOH (8%) over a median 10-year follow-up. After multivariable adjustment, the incidence of proteinuria in PWH with pre-DM was 3.3 times (95% confidence interval, 2.3–4.8 times) greater than in PWOH (P < .01). Among PWH, current CD4 cell count <50/µL (P < .01) and current use of protease inhibitors (P = .03) were associated with incident proteinuria, while lamivudine and integrase inhibitor use were associated with a lower risk.
Among men with pre-DM, the risk of incident proteinuria was 3 times higher in PWH. Strategies to preserve renal function are needed in this population.
Among aging people with human immunodeficiency virus (HIV; PWH) and receiving life-long combination antiretroviral therapy (ART), kidney disease, often in association with diabetes mellitus (DM) and high blood pressure (BP), has emerged as a significant cause of disease and death [1–3]. Urine protein-to-creatinine ratio (UPCR) and estimated glomerular filtration rate (eGFR) are commonly used as markers of kidney disease in the general population. In a previous Multicenter AIDS Cohort Study (MACS) analysis, proteinuria was almost 7 times more common in PWH than in people without HIV (PWOH), even after controlling for the presence of classic risk factors for kidney diseases, including DM [4]. In the general population, pre-DM, defined as an intermediate state of hyperglycemia, has been shown to be independently associated with incident proteinuria [5].
We have previously shown that having HIV is associated with a greater risk of incident DM among men with pre-DM [6]. In the current analysis, we sought to determine whether HIV was an independent risk factor for incident proteinuria among men with pre-DM. If so, PWH with pre-DM may particularly benefit from pharmacologic or nonpharmacologic strategies to preserve renal function, such as inhibitors of the renin-angiotensin-aldosterone system, sodium glucose transporter 2 inhibitors, or pharmacologic strategies for prevention of DM.
METHODS
Study Population
The MACS is a prospective cohort study of men who have sex with men (MSM) with or at risk for HIV at 4 centers in the United States (Baltimore, Maryland/Washington, DC; Pittsburgh, Pennsylvania/Columbus, Ohio; Chicago, Illinois; and Los Angeles, California), participants of which are now part of the MACS/WIHS (Women's Interagency HIV Study) Combined Cohort Study. Institutional review boards at each site approved the MACS protocol and forms, and each participant gave written informed consent. Details of the study design and follow-up methods have been published elsewhere [7] (MACS website at https://statepi.jhsph.edu/macs/macs.html). Briefly, participants attended semiannual study visits, which included a detailed interview, physical examination, and collection of biological specimens. For the present study, we included all MACS participants with confirmed pre-DM, as defined below. The study baseline was defined as the first visit with pre-DM at or after April 2006. Men with prevalent proteinuria or use of antidiabetic medications at the baseline visit were excluded from our analysis.
Laboratory Methods
Beginning in April 1999, glucose and hemoglobin A1c (HbA1c) were measured from serum samples at each semiannual visit. Glucose levels were measured using the combined hexokinase/glucose-6-phosphate dehydrogenase method at a central laboratory (Heinz Laboratory, Pittsburgh; coefficient of variation, 1.8%). HbA1c was measured by Quest Diagnostics using standard immunoassay assays (Roche Cobas Integra 800 analytical system; coefficient of variation, <3.3%). Protein and creatinine were measured from participant urine samples since April 2006. Standardized protocols were used to measure T-lymphocyte subsets. Plasma HIV-RNA levels were assessed using either the Roche standard, the Roche ultrasensitive, the Roche COBAS TaqMan, the Roche COBAS 6800/8800, or the Abbott m2000 RealTime assay.
Pre-DM Assessment
We restricted the glucose measures to serum samples obtained while the participant was fasting (self-report of ≥6 hours without food). Pre-DM was defined as a fasting glucose level between 100 and 125 mg/dL (ie, baseline visit), confirmed within a year by either a fasting glucose level in the pre-DM range (100–125 mg/dL) or an HbA1c measurement of 5.7%–6.4%.
Incident Proteinuria Assessment
Incident proteinuria was defined as a UPCR >200 mg/g, confirmed within a year by an additional UPCR >200 mg/g. This definition of proteinuria was used to minimize the likelihood of proteinuria being caused by a process other than chronic kidney disease. The timing of the incident event was set to the time of the first UPCR >200 mg/g.
Statistical Analysis
Baseline characteristics were compared according to HIV serostatus using Wilcoxon rank sum and Fisher exact tests. Poisson regression models were used to determine whether the risk for incident proteinuria among men with pre-DM differed by HIV serostatus. Multivariable regression models were adjusted for age (in years), race/ethnicity (non-Latino white or nonwhite), education (college degree earned or not), smoking status (never, former, or current smoker), categorical eGFR (using the race-free CKD-EPI equation [Chronic Kidney Disease Epidemiology Collaboration] equation) [8] (eGFR; <60, 60–90 or >90 mL/min/1.73 m2), body mass index (BMI [calculated as weight in kilograms divided by height in meters squared]), dyslipidemia, angiotensin-converting enzyme inhibitor (ACEI)/angiotensin II receptor blocker (ARB) use, high BP (vs normal or low BP; defined as systolic BP ≥140 mm Hg or diastolic BP ≥90 mm Hg, and hepatitis C virus (HCV) infection (last measured negative or last measured positive antibody or HCV RNA).
We also performed a subset analysis among PWH to estimate relationships between factors related to HIV or its treatment and incident proteinuria. Models including only PWH adjusted for the variables listed above and estimated the associations with the following HIV-related variables: suppressed HIV viral load (<500 copies/mL; vs unsuppressed), history of clinical AIDS (yes/no), current CD4 cell count <500/µL, CD4 cell count nadir <200/µL, and cumulative years of ART and highly active ART use before the baseline visit. We also fitted a series of multivariable regression models among PWH that adjusted for all the variables listed above and separately included a different HIV medication in each model, to assess the effect of HIV treatment on incident proteinuria risk. Missing values for continuous variables were linearly interpolated via bordering nonmissing values.
Sensitivity Analyses
Since anatomic fat distribution is a strong determinant of risk for insulin resistance and DM in particular among PWH with lipodystrophy, we added waist and hip circumference (recorded to the nearest centimeter by tape measure) to the multivariable model (excluding BMI), to evaluate the contribution of regional fat distribution to incident proteinuria. In addition, because we sought to specifically evaluate incident proteinuria in persons with pre-DM and because worsening hyperglycemia into the DM range may accelerate renal dysfunction, we fit a multivariable model for a study population that excluded any individual in whom overt DM developed over follow-up. Finally, we stratified the models by ACEI/ARB use to explore whether the association between HIV status and incident proteinuria differed between those receiving and those not receiving these interventions that can decrease proteinuria.
RESULTS
Baseline Characteristics
Of the 2746 men with a visit between 1 April 2006 and 30 September 2019, 1446 (53%) had an observed case of confirmed pre-DM at or after April 2006. Of these, 94 men (13 PWOH [14%] and 81 PWH [86%]) were excluded because they had prevalent proteinuria at the first visit with pre-DM. Another 76 men (37 PWOH [49%] and 39 PWH [51%]) were excluded because they reported anti-DM medication use at baseline. Among the remaining 1276 men with pre-DM (663 PWOH and 613 PWH), PWH were younger than PWOH (median age, 50 vs 54 years, respectively), had lower BMIs (median, 26 vs 27), and were more likely to be nonwhite (48% vs 27%), to be current smokers (30% vs 24%), have an eGFR >90 mL/min/1.73 m2 (59% vs 51%), and to have HCV (7% vs 4%) (all P ≤ .04 for comparison with PWOH) (Table 1).
Baseline Participant Characteristics of 1276 Men With Pre–Diabetes Mellitus
Characteristic . | Participants, No. (%) . | P Value . | |
---|---|---|---|
PWOH (n = 663) . | PWH (n = 613) . | ||
Age, median (IQR), y | 53.7 (47.3–59.9) | 49.5 (43.9–55.6) | <.01a |
Nonwhite race/ethnicity | 179 (27) | 293 (48) | <.01a |
College degree | 410 (62) | 281 (46) | <.01a |
Smoking status | |||
Never smoked | 208 (32) | 171 (29) | .04a |
Former smoker | 287 (44) | 246 (41) | |
Current smoker | 157 (24) | 182 (30) | |
eGFR,b mL/min/1.73 m2 | |||
<60 | 11 (2) | 19 (3) | <.01a |
60–90 | 316 (48) | 231 (38) | |
>90 | 336 (51) | 363 (59) | |
BMI, median (IQR)c | 27.2 (24.4–30.3) | 25.7 (23.3–28.8) | <.01a |
Dyslipidemia | 514 (78) | 492 (81) | .27 |
Current ACEI/ARB use | 117 (18) | 97 (17) | .50 |
High blood pressure | 156 (24) | 135 (22) | .55 |
Hepatitis C infection | 24 (4) | 42 (7) | .01a |
Characteristic . | Participants, No. (%) . | P Value . | |
---|---|---|---|
PWOH (n = 663) . | PWH (n = 613) . | ||
Age, median (IQR), y | 53.7 (47.3–59.9) | 49.5 (43.9–55.6) | <.01a |
Nonwhite race/ethnicity | 179 (27) | 293 (48) | <.01a |
College degree | 410 (62) | 281 (46) | <.01a |
Smoking status | |||
Never smoked | 208 (32) | 171 (29) | .04a |
Former smoker | 287 (44) | 246 (41) | |
Current smoker | 157 (24) | 182 (30) | |
eGFR,b mL/min/1.73 m2 | |||
<60 | 11 (2) | 19 (3) | <.01a |
60–90 | 316 (48) | 231 (38) | |
>90 | 336 (51) | 363 (59) | |
BMI, median (IQR)c | 27.2 (24.4–30.3) | 25.7 (23.3–28.8) | <.01a |
Dyslipidemia | 514 (78) | 492 (81) | .27 |
Current ACEI/ARB use | 117 (18) | 97 (17) | .50 |
High blood pressure | 156 (24) | 135 (22) | .55 |
Hepatitis C infection | 24 (4) | 42 (7) | .01a |
Abbreviations: ACEI, angiotensin-converting enzyme inhibitor; ARB, angiotensin II receptor blocker; BMI, body mass index; eGFR, estimated glomerular filtration rate; IQR, interquartile range; PWH, people with human immunodeficiency virus (HIV); PWOH, people without HIV.
aSignificant at P < .05.
beGFR determined using the race-free CKD-EPI (Chronic Kidney Disease Epidemiology Collaboration) equation.
cBMI calculated as weight in kilograms divided by height in meters squared.
Baseline Participant Characteristics of 1276 Men With Pre–Diabetes Mellitus
Characteristic . | Participants, No. (%) . | P Value . | |
---|---|---|---|
PWOH (n = 663) . | PWH (n = 613) . | ||
Age, median (IQR), y | 53.7 (47.3–59.9) | 49.5 (43.9–55.6) | <.01a |
Nonwhite race/ethnicity | 179 (27) | 293 (48) | <.01a |
College degree | 410 (62) | 281 (46) | <.01a |
Smoking status | |||
Never smoked | 208 (32) | 171 (29) | .04a |
Former smoker | 287 (44) | 246 (41) | |
Current smoker | 157 (24) | 182 (30) | |
eGFR,b mL/min/1.73 m2 | |||
<60 | 11 (2) | 19 (3) | <.01a |
60–90 | 316 (48) | 231 (38) | |
>90 | 336 (51) | 363 (59) | |
BMI, median (IQR)c | 27.2 (24.4–30.3) | 25.7 (23.3–28.8) | <.01a |
Dyslipidemia | 514 (78) | 492 (81) | .27 |
Current ACEI/ARB use | 117 (18) | 97 (17) | .50 |
High blood pressure | 156 (24) | 135 (22) | .55 |
Hepatitis C infection | 24 (4) | 42 (7) | .01a |
Characteristic . | Participants, No. (%) . | P Value . | |
---|---|---|---|
PWOH (n = 663) . | PWH (n = 613) . | ||
Age, median (IQR), y | 53.7 (47.3–59.9) | 49.5 (43.9–55.6) | <.01a |
Nonwhite race/ethnicity | 179 (27) | 293 (48) | <.01a |
College degree | 410 (62) | 281 (46) | <.01a |
Smoking status | |||
Never smoked | 208 (32) | 171 (29) | .04a |
Former smoker | 287 (44) | 246 (41) | |
Current smoker | 157 (24) | 182 (30) | |
eGFR,b mL/min/1.73 m2 | |||
<60 | 11 (2) | 19 (3) | <.01a |
60–90 | 316 (48) | 231 (38) | |
>90 | 336 (51) | 363 (59) | |
BMI, median (IQR)c | 27.2 (24.4–30.3) | 25.7 (23.3–28.8) | <.01a |
Dyslipidemia | 514 (78) | 492 (81) | .27 |
Current ACEI/ARB use | 117 (18) | 97 (17) | .50 |
High blood pressure | 156 (24) | 135 (22) | .55 |
Hepatitis C infection | 24 (4) | 42 (7) | .01a |
Abbreviations: ACEI, angiotensin-converting enzyme inhibitor; ARB, angiotensin II receptor blocker; BMI, body mass index; eGFR, estimated glomerular filtration rate; IQR, interquartile range; PWH, people with human immunodeficiency virus (HIV); PWOH, people without HIV.
aSignificant at P < .05.
beGFR determined using the race-free CKD-EPI (Chronic Kidney Disease Epidemiology Collaboration) equation.
cBMI calculated as weight in kilograms divided by height in meters squared.
Incident Proteinuria Among Men With Pre-DM: Differences by HIV Serostatus
At baseline, among the 1276 men with pre-DM (663 PWOH and 613 PWH), incident proteinuria developed in 50 PWOH (8%) and 128 PWH (21%) over a median of 10 years of follow-up (Figure 1). In a multivariable regression model, PWH had a 3.3-fold (95% confidence interval [CI], 2.3–4.8-fold) higher incidence of proteinuria compared with PWOH (P < .01) (Figure 2). In addition, a categorically lower eGFR (<60 vs >90 mL/min/1.73 m2, respectively) (incidence rate [IR] ratio [IRR], 3.5 [95% CI, 2.0–6.2]), current HCV (2.1 [1.2–3.9]), current hypertension (2.0 [1.4–2.7]), and current cigarette smoking (1.7 [1.1–2.7]) were all associated with a higher risk of incident proteinuria (Figure 2). Moreover, PWH had a greater adjusted IR of proteinuria (per 100 000 person-years) than PWOH from 0 to 9 years after study baseline. The difference in adjusted IR of proteinuria by HIV serostatus remained relatively stable between 1 and 6 years after the confirmed pre-DM visit (Supplementary Figure 1).
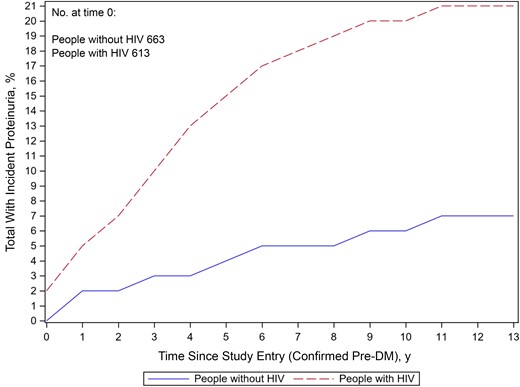
Incident proteinuria among men with pre–diabetes mellitus (DM) over a median of 10 years of follow-up Differences by human immunodeficiency virus (HIV) serostatus. (Percentages displayed in the figure differ slightly from those reported in the text owing to HIV seroconversion over follow-up.)
![Multivariable regression model for incident proteinuria among 1276 men with pre–diabetes mellitus (DM). Forest plot of multivariate Poisson regression model results, among 1276 men with pre-DM between 1 April 2006 and 30 September 2019. Incident proteinuria is the outcome, human immunodeficiency virus (HIV) serostatus is the main exposure, and controls are age, race/ethnicity, education, smoking status, categorical estimated glomerular filtration rate (eGFR; determined using the race-free CKD-EPI [Chronic Kidney Disease Epidemiology Collaboration] equation), body mass index (BMI; calculated as weight in kilograms divided by height in meters squared), dyslipidemia, angiotensin-converting enzyme inhibitor (ACEI)/angiotensin II receptor blocker (ARB) use, high blood pressure (BP), and hepatitis C virus (HCV) infection. Error bars represent 95% confidence intervals; dashed vertical line, incidence rate ratio (IRR) of 1.0 (no difference in proteinuria risk by exposure). Abbreviation: Ref, reference.](https://oup.silverchair-cdn.com/oup/backfile/Content_public/Journal/cid/79/2/10.1093_cid_ciae065/1/m_ciae065f2.jpeg?Expires=1750199838&Signature=cZeeoYJORHSNNNvIgM8MMR35rs5f9NEYOKREG7j88Clw78TPG8pDTZpmPkpHpU7oh7u5OFz46dexUiqnKBZ-giybxgqhF-cRdaE~usltn02bnMSyPrOJVldFAvY9lon89nY0kEkpU24c~B9ZfIJqSDkGXW~QLy5YJuQEtbFVVZQacwe6eV6rI8lkM1G9tfBriPOe1I7ET8DlNtrJvnepxKoEZSNmAPsdkkiiSpCKGirmQghOkJX1XYPwYCJMcIAJCJEhnudYTMk2NHBJXQ0PRosRKxlYkeRogvokxj5IRbCFfxKqSrgPAQPdTkOpIv2VDpSixDJMJxBRQYqEtCjSeg__&Key-Pair-Id=APKAIE5G5CRDK6RD3PGA)
Multivariable regression model for incident proteinuria among 1276 men with pre–diabetes mellitus (DM). Forest plot of multivariate Poisson regression model results, among 1276 men with pre-DM between 1 April 2006 and 30 September 2019. Incident proteinuria is the outcome, human immunodeficiency virus (HIV) serostatus is the main exposure, and controls are age, race/ethnicity, education, smoking status, categorical estimated glomerular filtration rate (eGFR; determined using the race-free CKD-EPI [Chronic Kidney Disease Epidemiology Collaboration] equation), body mass index (BMI; calculated as weight in kilograms divided by height in meters squared), dyslipidemia, angiotensin-converting enzyme inhibitor (ACEI)/angiotensin II receptor blocker (ARB) use, high blood pressure (BP), and hepatitis C virus (HCV) infection. Error bars represent 95% confidence intervals; dashed vertical line, incidence rate ratio (IRR) of 1.0 (no difference in proteinuria risk by exposure). Abbreviation: Ref, reference.
Incident Proteinuria Among Men With Pre-DM and HIV
In a multivariable regression model restricted to men with HIV, a CD4 cell count <500/µL was significantly associated with a 2 times greater incidence of proteinuria relative to a CD4 cell count ≥500/µL (IRR, 2.0 [95% CI, 1.3–3.0]; P < .01), while a history of clinical AIDS was borderline significantly associated with increased proteinuria risk (1.7 [.9–2.9]; P = .08) (Figure 3).
![Multivariable regression model for incident proteinuria among 613 men with pre–diabetes mellitus (DM) and human immunodeficiency virus (HIV). Forest plot of multivariate Poisson regression model results, among 613 men with pre-DM and HIV between 1 April 2006 and 30 September 2019. Incident proteinuria is the outcome, and covariates are age, race/ethnicity, education, smoking status, categorical estimated glomerular filtration rate (eGFR; determined using the race-free CKD-EPI [Chronic Kidney Disease Epidemiology Collaboration] equation), body mass index (BMI), dyslipidemia, angiotensin-converting enzyme inhibitor (ACEI)/angiotensin II receptor blocker (ARB) use, high blood pressure (BP), hepatitis C virus (HCV) infection, suppressed HIV viral load (VL), history of clinical AIDS, current CD4 cell count <500/µL, CD4 cell count nadir <200/µL, and cumulative years of antiretroviral therapy (ART) and highly active ART (HAART) use before the baseline visit. Error bars represent 95% confidence intervals (CIs); dashed vertical line, incidence rate ratio (IRR) of 1.0 (no difference in proteinuria risk by exposure). Abbreviation: Ref, reference.](https://oup.silverchair-cdn.com/oup/backfile/Content_public/Journal/cid/79/2/10.1093_cid_ciae065/1/m_ciae065f3.jpeg?Expires=1750199838&Signature=qgSSZQg4aMHGwOricte8~VQkkgpii3R9pktsFVBfrRwomrqNO5tToSD1pCrlcLA5kuG5ay5xjNerUBnLhOEWHSggq~yoXhg7nei~F2Ax112I6LLyClGCzVOHdm4aHJZu9YKdlPHgG9yz5hFQcXhiH9VtdBRpO0e9ThHAByluW5NBw0chWMf92CPU4CxTjoUVpxIMNr3mSB9uPLdykvibTSTPmbSYysBLIjLttgGNxm8WoOSZZtMWNDDA7ALggplkaeERVzUc-dG0pdGve1G51jitJ3A0DFJnxnInjxX-J9Zu61MyxefIE37IbQlbCFiFUtyxXLeGHQVtc-3DJDVwvg__&Key-Pair-Id=APKAIE5G5CRDK6RD3PGA)
Multivariable regression model for incident proteinuria among 613 men with pre–diabetes mellitus (DM) and human immunodeficiency virus (HIV). Forest plot of multivariate Poisson regression model results, among 613 men with pre-DM and HIV between 1 April 2006 and 30 September 2019. Incident proteinuria is the outcome, and covariates are age, race/ethnicity, education, smoking status, categorical estimated glomerular filtration rate (eGFR; determined using the race-free CKD-EPI [Chronic Kidney Disease Epidemiology Collaboration] equation), body mass index (BMI), dyslipidemia, angiotensin-converting enzyme inhibitor (ACEI)/angiotensin II receptor blocker (ARB) use, high blood pressure (BP), hepatitis C virus (HCV) infection, suppressed HIV viral load (VL), history of clinical AIDS, current CD4 cell count <500/µL, CD4 cell count nadir <200/µL, and cumulative years of antiretroviral therapy (ART) and highly active ART (HAART) use before the baseline visit. Error bars represent 95% confidence intervals (CIs); dashed vertical line, incidence rate ratio (IRR) of 1.0 (no difference in proteinuria risk by exposure). Abbreviation: Ref, reference.
In separate adjusted Poisson regression models, cumulative years of amprenavir use (IRR, 3.3 [95% CI, 1.1–9.4]) and current use of protease inhibitors (1.5 [1.0–2.3]) were significantly associated with incident proteinuria (P = .03) (Table 2). Cumulative years of lamivudine use was significantly associated with a reduced risk for incident proteinuria (IRR, 0.8 [95% CI, .7–1.0]; P = .04), as was integrase inhibitor (INSTI) use—regardless if it was categorized as ever (0.5 [.3–.8]), cumulative (0.5 [.3–1.0]), or current use (0.5 [.3–.9]) (all P ≤ .03) (Table 2). Use of tenofovir disoproxil fumarate (TDF) was not associated with incident proteinuria (IRR, 1.3 [95% CI, .8–2.3]; P = .3).
Multivariable Poisson Regression Model Results for Incident Proteinuria in 613 Men With Pre–Diabetes Mellitus and Human Immunodeficiency Virusa
HIV Medication Exposureb . | IRR (95% CI) . | P Value . |
---|---|---|
Lamivudine cumulative use, per 5 y | 0.8 (.7–1.0) | .04 |
Amprenavir cumulative use, per 5 y | 3.3 (1.1–9.4) | .03 |
INSTI use ever (Ref: never used INSTIs) | 0.5 (.3–.8) | <.01 |
INSTI cumulative use, per 5 y | 0.5 (.3–1.0) | .03 |
INSTI use currently (Ref: not currently using INSTIs) | 0.5 (.3–.9) | ≤.01 |
PI use currently (Ref: not currently using PIs) | 1.5 (1.0–2.3) | .03 |
HIV Medication Exposureb . | IRR (95% CI) . | P Value . |
---|---|---|
Lamivudine cumulative use, per 5 y | 0.8 (.7–1.0) | .04 |
Amprenavir cumulative use, per 5 y | 3.3 (1.1–9.4) | .03 |
INSTI use ever (Ref: never used INSTIs) | 0.5 (.3–.8) | <.01 |
INSTI cumulative use, per 5 y | 0.5 (.3–1.0) | .03 |
INSTI use currently (Ref: not currently using INSTIs) | 0.5 (.3–.9) | ≤.01 |
PI use currently (Ref: not currently using PIs) | 1.5 (1.0–2.3) | .03 |
Abbreviations: CI, confidence interval; INSTI, integrase inhibitor; IRR, incidence rate ratio; PI, protease inhibitor; Ref, reference.
aModels also controlled for age, race/ethnicity, education, smoking status, categorical estimated glomerular filtration rate (determined using the race-free CKD-EPI [Chronic Kidney Disease Epidemiology Collaboration] equation), body mass index, dyslipidemia, angiotensin-converting enzyme inhibitor/angiotensin II receptor blocker use, high blood pressure, hepatitis C virus infection, suppressed human immunodeficiency virus (HIV) viral load, history of clinical AIDS, current CD4 cell count <500/µL, and CD4 cell count nadir before the baseline visit <200/µL.
bHIV medications were included in separate regression models. Only statistically significant results are presented. Also explored were cumulative exposure to antiretroviral therapy (ART), retrovir, stavudine, ritonavir, combivir, trizivir, tenofovir, atazanavir, darunavir, dolutegravir, didanosine, saquinavir, nelfinavir, emtricitabine, zalcitabine, abacavir, indinavir, lopinavir, nevirapine, delavirdine, efavirenz, enfuvirtide, elvitegravir, thymidine analogs, nucleoside reverse-transcriptase inhibitors, PIs, nonnucleoside reverse-transcriptase inhibitors, or highly active ART; any use ever of stavudine, tenofovir, dolutegravir, thymidine analogues, or PIs; and current use of highly active ART or tenofovir.
Multivariable Poisson Regression Model Results for Incident Proteinuria in 613 Men With Pre–Diabetes Mellitus and Human Immunodeficiency Virusa
HIV Medication Exposureb . | IRR (95% CI) . | P Value . |
---|---|---|
Lamivudine cumulative use, per 5 y | 0.8 (.7–1.0) | .04 |
Amprenavir cumulative use, per 5 y | 3.3 (1.1–9.4) | .03 |
INSTI use ever (Ref: never used INSTIs) | 0.5 (.3–.8) | <.01 |
INSTI cumulative use, per 5 y | 0.5 (.3–1.0) | .03 |
INSTI use currently (Ref: not currently using INSTIs) | 0.5 (.3–.9) | ≤.01 |
PI use currently (Ref: not currently using PIs) | 1.5 (1.0–2.3) | .03 |
HIV Medication Exposureb . | IRR (95% CI) . | P Value . |
---|---|---|
Lamivudine cumulative use, per 5 y | 0.8 (.7–1.0) | .04 |
Amprenavir cumulative use, per 5 y | 3.3 (1.1–9.4) | .03 |
INSTI use ever (Ref: never used INSTIs) | 0.5 (.3–.8) | <.01 |
INSTI cumulative use, per 5 y | 0.5 (.3–1.0) | .03 |
INSTI use currently (Ref: not currently using INSTIs) | 0.5 (.3–.9) | ≤.01 |
PI use currently (Ref: not currently using PIs) | 1.5 (1.0–2.3) | .03 |
Abbreviations: CI, confidence interval; INSTI, integrase inhibitor; IRR, incidence rate ratio; PI, protease inhibitor; Ref, reference.
aModels also controlled for age, race/ethnicity, education, smoking status, categorical estimated glomerular filtration rate (determined using the race-free CKD-EPI [Chronic Kidney Disease Epidemiology Collaboration] equation), body mass index, dyslipidemia, angiotensin-converting enzyme inhibitor/angiotensin II receptor blocker use, high blood pressure, hepatitis C virus infection, suppressed human immunodeficiency virus (HIV) viral load, history of clinical AIDS, current CD4 cell count <500/µL, and CD4 cell count nadir before the baseline visit <200/µL.
bHIV medications were included in separate regression models. Only statistically significant results are presented. Also explored were cumulative exposure to antiretroviral therapy (ART), retrovir, stavudine, ritonavir, combivir, trizivir, tenofovir, atazanavir, darunavir, dolutegravir, didanosine, saquinavir, nelfinavir, emtricitabine, zalcitabine, abacavir, indinavir, lopinavir, nevirapine, delavirdine, efavirenz, enfuvirtide, elvitegravir, thymidine analogs, nucleoside reverse-transcriptase inhibitors, PIs, nonnucleoside reverse-transcriptase inhibitors, or highly active ART; any use ever of stavudine, tenofovir, dolutegravir, thymidine analogues, or PIs; and current use of highly active ART or tenofovir.
Multivariate Regression Models: Sensitivity Analyses
When waist and hip circumference replaced BMI in the multivariate Poisson model, results were comparable: HIV serostatus remained significantly associated with proteinuria risk, with PWH having a proteinuria IR 3.3 times greater than PWOH. The same was true when we stratified the models by ACEI/ARB use. The adjusted IRR was 3.1 [95% CI, 2.0–4.8] comparing ACEI/ARB nonusers with HIV versus without HIV (P < .01), while the adjusted IRR was 4.0 [2.1–7.8] comparing ACEI/ARB users with versus without HIV (P < .01).
In the multivariate model that excluded men who developed DM over follow-up (n = 1048 pre-DM men remaining), PWH had a 5.0 times [95% CI, 3.1–7.9 times] greater IR of proteinuria than PWOH (P < .01). APOL1 risk variants are known drivers of proteinuria and chronic kidney disease among black individuals [9]. However, when we restricted our analysis to men of self-reported black race (n = 302), incident proteinuria by HIV serostatus remained statistically significant (IRR, 2.3 [95% CI, 1.2–4.7]), P = .02.
DISCUSSION
Pre-DM is a very common condition that increases not only the risk of DM but also the risk of end-organ complications, such as kidney disease, cardiovascular disease, and neuropathy, in the general population [10–15]. We have previously shown in the MACS that among men with pre-DM, the risk of developing DM was 40% higher among PWH than among PWOH after adjustment for competing DM risk factors [6]. In this analysis, which spanned a median 10 years of follow-up and included 1276 participants, we showed that the risk of incident proteinuria in men with pre-DM was 3 times greater among PWH than among PWOH after adjustment for traditional risk factors. Given that proteinuria is strongly associated with subsequent declines in glomerular filtration rate and chronic kidney disease, our findings suggest that persons with pre-DM and HIV may particularly benefit from interventions to preserve kidney function and that further study is warranted.
Similar to findings in the general population [16], the prevalence of pre-DM was high in the MACS population, with >50% of MACS participants categorized as pre-DM during the study interval. Among men with pre-DM, the characteristics of those with HIV differed from those of men without HIV. Men with pre-DM and HIV were on average younger, had a lower BMI, were more likely to be nonwhite, and were more likely to have HCV. Persons with pre-DM are at risk for multiple negative health outcomes, including renal dysfunction. At the baseline visit, 6.5% of MACS men with pre-DM had prevalent proteinuria (UPCR >200 mg/g), equivalent to an albumin-to-creatinine ratio of 52 mg/g. This is comparable to findings from the US National Health and Nutrition Examination Survey in 2011–2014, in which 7.7% of persons with pre-DM had prevalent microalbuminuria (albumin-to-creatinine ratio ≥30 mg/g) [17].
One major strength of our study was its longitudinal design. After we excluded men with significant proteinuria at baseline, 14% developed incident proteinuria (UPCR ≥200 mg/g, confirmed on a subsequent visit) over the median 10-year study interval. Of 9 studies in a 2016 systematic review, studies examining the relationship between pre-DM and incident chronic kidney disease, only 1 reported on the outcome of proteinuria alone and found that pre-DM was associated with incident dipstick proteinuria (relative risk, 1.31 [95% CI, 1.16–1.48]) [18]. In a subsequent large study of Japanese adults, proteinuria developed in 2.1% of >116 000 persons with pre-DM over 2 years (defined as a dipstick urinary protein scores of ≥1+; equivalent to a UPCR ≥150 mg/g), which was approximately 30% that in those with normoglycemia [5]. In the Chronic Renal Insufficiency Cohort [19], a US-based multicenter cohort of persons with chronic kidney disease, 37% of 847 individuals with pre-DM had proteinuria progression (doubling of UPCR to ≥0.22 g/g creatinine) over a 7.5 year follow-up period; these results were 23% higher than in those with normoglycemia.
The central finding of our study was that HIV serostatus was the factor second-most strongly associated with incident proteinuria in men with pre-DM, with a relative risk of 3.3. These results are similar to those of a previous MACS analysis [4], which showed that prevalent proteinuria was nearly 7 times more common in men with HIV on ART than in those without HIV, after multivariable adjustment. Our findings extend these results by examining incident rather than prevalent proteinuria and focusing on men with pre-DM, a population at increased risk for renal complications that may particularly benefit from preventive strategies.
Among HIV disease and treatment variables, we found that a lower CD4 cell count and certain ART exposures were associated with incident proteinuria. Similar to the MACS prevalence analysis, we found that a current CD4 cell count <500/µL was associated with a 2-fold increase in incident proteinuria among PWH with pre-DM, although no statistically significant association was seen with nadir CD4 cell count, history of AIDS, or other markers of immunologic function. In previous MACS analyses, proteinuria and renal dysfunction have been associated with markers of systemic inflammation [4, 20]. We hypothesize that immunologic dysfunction and resulting systemic inflammation in those with incomplete T-cell recovery is also driving the risk of proteinuria in PWH with pre-DM.
Specific ART medications may contribute to proteinuria risk in men with HIV and pre-DM. We found that current protease inhibitor use was associated with incident proteinuria, as seen in previous studies [4], while INSTI use was associated with a lower risk of proteinuria. This latter finding requires confirmation in other studies, especially given the known effects of INSTI use on weight and metabolic outcomes [21, 22]. Of note, in our analysis tenofovir use was not associated with incident proteinuria, which we suspect is because men who were receiving TDF and had proteinuria at baseline were excluded from the analysis by design, obscuring any overall association between TDF use and proteinuria. Indeed, 399 of 613 PWH (65%) who were included had ever taken TDF, while 66 of 81 (81%) who were excluded at baseline owing to prevalent proteinuria had ever taken TDF (P = .005). Given that the effect of TDF on renal tubular dysfunction is heterogeneous, we hypothesize that those included in our analysis who were receiving TDF were less at risk for incident proteinuria than those who were excluded.
Other than HIV serostatus, we identified several other risk factors associated with incident proteinuria. For example, hypertension, a well-known risk factor for proteinuria, had an IRR of 2.0 [95% CI, 1.4–2.7] (with high BP defined as a systolic BP of ≥140 mm Hg or a diastolic BP of ≥90 mm Hg). When we expanded the definition of hypertension to also include those receiving antihypertensive medications, the point estimates for both the effect of hypertension on incident proteinuria and the effect of HIV on incident proteinuria were unchanged compared with the initial analysis (data not shown). In addition to well-known risk factors for incident proteinuria, such as renal impairment and hypertension, HCV was also a strong risk factor, as seen in previous analyses [4, 23]. Early evidence suggests that HCV treatment and cure may decrease the risk of proteinuria and renal disease [24]
Taken together, our findings suggest that PWH with pre-DM may represent an important target population for prevention strategies. This may include recommendations that promote more aggressive lifestyle changes or metformin use to reduce the progression to overt DM and its related complications, such as renal disease. The use of sodium glucose transporter 2 inhibitor treatment, which has favorable effects on protein excretion and glycemic control, should be evaluated in this population [25], as well as blockade of the renin-angiotensin-aldosterone system, which has been shown to be activated in PWH [26].
Our study has several limitations. First, we did not perform oral glucose tolerance testing, which may have resulted in our underestimating the population with pre-DM. Second, our study included only men, so it is unclear whether our findings are generalizable to women with HIV and pre-DM. Third, because we required incident proteinuria cases to have 2 UPCR measurements within a year of each other, participants with more missed study visits would be less likely to be classified as a case patient. This may be a concern if the reasons for missing a study visit were related to a participant having proteinuria.
In conclusion, among men with pre-DM, HIV seropositivity was associated with a greater risk of incident proteinuria after adjustment for traditional proteinuria risk factors. Our findings suggest the need for implementation of early screening and prevention strategies among men with HIV and pre-DM in order to reduce the risk of incident proteinuria and long-term kidney disease.
Supplementary Data
Supplementary materials are available at Clinical Infectious Diseases online. Consisting of data provided by the authors to benefit the reader, the posted materials are not copyedited and are the sole responsibility of the authors, so questions or comments should be addressed to the corresponding author.
Notes
Acknowledgments. The authors gratefully acknowledge the contributions of the study participants and dedication of the staff at the Multicenter AIDS Cohort Study (MACS)/WIHS (Women’s Interagency HIV Study) Combined Cohort Study (MWCCS) sites.
Author contributions. L. S. and T. T. B. conceived and designed the study. B. W. B. and A. G. A. were responsible for data acquisition and statistical analysis. L. S., B. W. B., A. G. A., and T. T. B. performed the analysis, interpreted the results, and wrote the first draft of the report. All authors critically revised the report and approved the final version.
MWCCS (principal investigators). Atlanta Clinical Research Site (CRS) (Ighovwerha Ofotokun, Anandi Sheth, and Gina Wingood); Baltimore CRS (Todd Brown and Joseph Margolick); Bronx CRS (Kathryn Anastos, David Hanna, and Anjali Sharma); Brooklyn CRS (Deborah Gustafson and Tracey Wilson); Data Analysis and Coordination Center (Gypsyamber D'Souza, Stephen Gange, and Elizabeth Topper); Chicago-Cook County CRS (Mardge Cohen and Audrey French); Chicago-Northwestern CRS (Steven Wolinsky, Frank J Palella, and Valentina Stosor); Northern California CRS (Bradley Aouizerat, Jennifer Price, and Phyllis Tien); Los Angeles CRS (Roger Detels and Matthew Mimiaga); Metropolitan Washington CRS (Seble Kassaye and Daniel Merenstein); Miami CRS (Maria Alcaide, Margaret Fischl, and Deborah Jones); Pittsburgh CRS (Jeremy Martinson and Charles Rinaldo); University of Alabama-Birmingham/University of Mississipi CRS (Mirjam-Colette Kempf, Jodie Dionne-Odom, Deborah Konkle-Parker, and James B. Brock); University of North Carolina CRS (Bradley Drummond and Michelle Floris-Moore).
Disclaimer. The contents of this publication are solely the responsibility of the authors and do not represent the official views of the National Institutes of Health (NIH).
Financial support.
The MWCCS is funded primarily by the National Heart, Lung, and Blood Institute (NHLBI), with additional cofunding from the Eunice Kennedy Shriver National Institute of Child Health & Human Development (NICHD), the National Institute on Aging (NIA), the National Institute of Dental & Craniofacial Research (NIDCR), the National Institute of Allergy and Infectious Diseases (NIAID), the National Institute of Neurological Disorders and Stroke (NINDS), the National Institute of Mental Health (NIMH), the National Institute on Drug Abuse (NIDA), the National Institute of Nursing Research (NINR), the National Cancer Institute (NCI), the National Institute on Alcohol Abuse and Alcoholism (NIAAA), the National Institute on Deafness and Other Communication Disorders (NIDCD), the National Institute of Diabetes and Digestive and Kidney Diseases (NIDDK), the National Institute on Minority Health and Health Disparities (NIMHD), and in coordination and alignment with the research priorities of the NIH, Office of AIDS Research (OAR). The NIH grant numbers supporting the MWCCS sites are: U01-HL146241, U01-HL146201, U01-HL146204, U01-HL146193, U01-HL146245, U01-HL146240, U01-HL146242, U01-HL146333, U01-HL146205, U01-HL146203, U01-HL146208, U01-HL146192, U01-HL146194. MWCCS data collection is also supported by NIH grants UL1-TR000004, UL1-TR003098, UL1-TR001881, P30-AI-050409, P30-AI-073961, P30-AI-050410, P30-AI-027767, P30-MH-116867, UL1-TR001409, KL2-TR001432, and TL1-TR001431.
References
Author notes
Potential conflicts of interest. L. S. declares consulting fees, support for attending meetings, and payment for presentation from Gilead Sciences, ViiV Healthcare, and Merck, outside the present work. A. G. A. declares a National Heart, Lung, and Blood Institute grant paid to her institution. F. J. P. declares payment from ViiV, Gilead Sciences, Janssen, Merck, and EMD Serono, outside the present work. J. W. M. declares a NIH/NHLBI research grant to the University of Pittsburgh and is an American Heart Association committee member. J. E. L. declares research support from Gilead Sciences, Pfizer, Oncoimmune, and CytoDyn, all paid to her institution; consulting fees for Theratechnologies; and stock options from CytoDyn. T. T. B. declares consulting fees from Merck, Janssen, Gilead Sciences, and ViiV Healthcare, outside the present work. All other authors report no potential conflicts.
All authors have submitted the ICMJE Form for Disclosure of Potential Conflicts of Interest. Conflicts that the editors consider relevant to the content of the manuscript have been disclosed.