-
PDF
- Split View
-
Views
-
Cite
Cite
M Cristina Vazquez Guillamet, Rodrigo Vazquez, Jonas Noe, Scott T Micek, Victoria J Fraser, Marin H Kollef, Impact of Baseline Characteristics on Future Episodes of Bloodstream Infections: Multistate Model in Septic Patients With Bloodstream Infections, Clinical Infectious Diseases, Volume 71, Issue 12, 15 December 2020, Pages 3103–3109, https://doi.org/10.1093/cid/ciz1206
- Share Icon Share
Abstract
Looking only at the index infection, studies have described risk factors for infections caused by resistant bacteria. We hypothesized that septic patients with bloodstream infections may transition across states characterized by different microbiology and that their trajectory is not uniform. We also hypothesized that baseline risk factors may influence subsequent blood culture results.
All adult septic patients with positive blood cultures over a 7-year period were included in the study. Baseline risk factors were recorded. We followed all survivors longitudinally and recorded subsequent blood culture results. We separated states into bacteremia caused by gram-positive cocci, susceptible gram-negative bacilli (sGNB), resistant GNB (rGNB), and Candida spp. Detrimental transitions were considered when transitioning to a culture with a higher mortality risk (rGNB and Candida spp.). A multistate Markov-like model was used to determine risk factors associated with detrimental transitions.
A total of 990 patients survived and experienced at least 1 transition, with a total of 4282 transitions. Inappropriate antibiotics, previous antibiotic exposure, and index bloodstream infection caused by either rGNB or Candida spp. were associated with detrimental transitions. Double antibiotic therapy (beta-lactam plus either an aminoglycoside or a fluoroquinolone) protected against detrimental transitions.
Baseline characteristics that include prescribed antibiotics can identify patients at risk for subsequent bloodstream infections caused by resistant bacteria. By altering the initial treatment, we could potentially influence future bacteremic states.
In general, and with few exceptions such as human immunodeficiency virus (HIV), infectious diseases have been regarded as acute diseases with a binary outcome: cured or not cured. Adequate treatment that stems mainly from appropriate antibiotics results in clearance of the infectious process and typically leads to improved short-term outcomes [1, 2]. However, the trajectory of infected patients may also include possible long-term impact [3, 4]. This would be similar to other acute disease states where patients may evolve across different stages including returning to healthy states. For instance, a patient with uncontrolled diabetes may experience myocardial infarction and may fully recover or develop congestive heart failure. We should view infectious diseases as having longitudinal pathology as well, where patients can transition from one episode of infection to the next at variable time intervals.
Sepsis caused by bloodstream infections is associated with significant mortality, morbidity, and healthcare costs [5, 6]. Multidrug-resistant (MDR) bacteria can amplify these detrimental outcomes [6, 7]. Classifying patients at risk for MDR microbes when they present with signs and symptoms of infection has become the focus of multiple studies. As such, many identified risk factors such as exposure to the healthcare system, prior administration of antibiotics, immunocompromised states, and structural lung disease predispose to infection with MDR microbes [8, 9]. The presence of such risk factors makes it possible to tailor empiric antibiotic regimens. However, this static approach is not used to examine the long-term impact of infections to include the etiology of subsequent infections.
We hypothesized that baseline patient and treatment characteristics could influence the transition between bacteremic states when assessed in a longitudinal manner. For this purpose, we used a Markov-like multistate model that had been applied previously to describe staged progressive diseases such as HIV [10]. Identification of the factors present at the time of the index bloodstream infection that are independently associated with subsequent MDR bloodstream infections, especially if modifiable, can help in the development of targeted strategies that can ameliorate this risk and improve outcomes.
METHODS
Settings and Participants
The study was conducted at Barnes Jewish Hospital (BJH), a 1350-bed academic center affiliated with the Washington University School of Medicine in St. Louis, Missouri. The Washington University School of Medicine Institutional Review Board approved this study and waived the need for informed consent. We used the hospital’s electronic health record system to collect clinical data including diagnoses, severity scores, laboratory results, microbiology data including antimicrobial susceptibility, imaging, and pharmacy data. This repository of clinical data has been provided by the Center for Clinical Excellence, BJC Healthcare, St. Louis, Missouri, and has been previously verified against manual extraction with a concordance of >95%.
All adult patients admitted to BJH between 1 January 2008 and 30 April 2015 with sepsis and true bloodstream infections, defined as ≥1 positive blood cultures for true pathogens and ≥2 positive blood cultures plus a concordant clinical scenario for frequent contaminants, were screened for inclusion. Septic shock was defined as the need for any of the following vasoactive agents: norepinephrine, vasopressin, phenylephrine, dopamine. We collected data on comorbidities; previous antibiotic use; nursing home residence; acuity of presentation; source of infection; and laboratory, microbiology, and imaging data, along with treatments rendered and in-hospital mortality. The definitions used for this cohort have been previously reported [11]. In brief, previous hospitalization was recorded for 30 days prior, while antibiotic use was recorded for the previous 90 days. All time stamps for the presence of central lines, duration of mechanical ventilation, nursing home residence, and imaging were manually verified. We then collected follow-up blood culture results until 30 April 2016, but only the first 6 months were included for each patient.
MICROBIOLOGY
The minimum inhibitory concentration breakpoints and interpretative criteria were concordant with the Clinical and Laboratory Standards Institute guidelines, and we followed the changes for beta-lactam antibiotics [12]. We organized our cohort into the following 4 groups based on the initial blood culture results: gram-positive cocci (GPC), susceptible gram-negative bacilli (sGNB), resistant gram-negative bacilli (rGNB), and Candida spp. Since ceftriaxone is the most commonly used antibiotic when no resistant GNB are suspected, we used susceptibility to ceftriaxone to separate sGNB from rGNB. Given the potential for AmpC production, our microbiology laboratory reported Enterobacter and Serratia spp. as ceftriaxone-resistant. This classification was based on different mortality rates for these groups in our cohort (20.8% for sGNB, 28.9% for GPC, 34.3% for rGNB, and 44.7% for Candida spp.). Bacteremia caused by rGNB and Candida spp. was more likely to result in death. Consequently, polymicrobial cultures were assigned to the pathogen that carried the higher mortality (Candida spp. and rGNB > GPC and sGNB). Antibiotics lacking in vitro activity against the microbe isolated in the blood cultures and administered empirically for at least 24 hours defined inappropriate antibiotics. Clinical pharmacists approved all antibiotic orders in real time to ensure that dosing and the interval of administration were adequate for individual patients based on body size, renal function, and resuscitation status. Once susceptibilities were available, the empiric regimen was narrowed to a single agent.
Statistical Methods and Study Design
Continuous variables are reported as means or medians with standard deviation and interquartile range (IQR) when appropriate and compared using the t test or Wilcoxon- Mann-Whitney and analysis of variance tests. Categorical and binary variables are presented as percentages and compared using the χ2 or Fisher exact test. Baseline risk factors for future sepsis with bloodstream infections caused by MDR pathogens were measured in patients who had at least 2 episodes that required blood cultures to be drawn over a 6-month period. Blood cultures that were positive for the same pathogen and negative cultures drawn to confirm clearance of the index bacteremia were excluded when they occurred within 2 weeks for GNB or GPC and 6 weeks for Staphylococcus aureus (Figure 1).
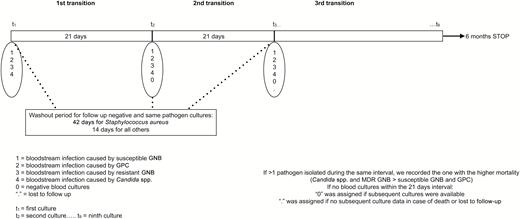
Timeline for assigning the transitions according to the availability of the blood culture results. Abbreviations: GNB, gram-negative bacilli; GPC, gram-positive cocci; MDR, multidrug-resistant.
Multistate Markov-like Transition Model
Markov models represent an optimal choice for analyzing repetitive events (eg, bloodstream infections) that occur at uncertain times and may include reversals in healthy states [13]. In brief, a patient will always be in 1 of a finite number of health states (negative culture or positive culture with various species). The total follow-up time is divided into equal intervals of time referred to as Markov cycles. Patients can move from one state to another or remain in the same state between cycles. These changes are referred to as transitions. Transitions, not patients, are the unit of analysis. Because our data, like most medical processes, do not strictly satisfy the Markov property in which the probability of a transition is independent of the previous state, we used a Markov-like multistate model.
The study’s follow-up period was 6 months. Since 25% of the follow-up sepsis episodes occurred at 23 days after the index bacteremic episode, we set the surveillance interval (ie, Markov cycle) at 21 days. All patients were screened for positive and negative cultures at 21-day intervals, leading to 8 intervals or transitions (Figure 1). The initial positive-culture groupings (GPC, sGNB, rGNB, Candida spp.) were the starting states for the transition matrix. The negative cultures state was added for subsequent Markov cycles (Figure 1) for 2 occasions: if, during the cycle, blood cultures were negative or if the patient had no blood cultures during that cycle but he/she had negative results during a subsequent cycle (ie, the patient remained in our system and did not display a reason for blood cultures to be checked and therefore were assumed to be in a negative-culture state). Death and loss to follow-up when no blood cultures were available were considered absorbing states (ie, patients cannot transition out of these states) and were excluded from the analyses. All prior transitions up until death and loss to follow-up were included in the analyses.
Since bacteremias caused by rGNB and Candida spp. carried a higher mortality in our cohort, we defined detrimental transitions as remaining or shifting to a state designated as rGNB or Candida bloodstream infections irrespective of the starting point. As such, GPC, sGNB, rGNB, Candida spp., and negative cultures that progressed to either rGNB or Candida spp. bloodstream infections were considered detrimental transitions. All other transitions were labeled “nondetrimental” (Supplementary Table 1).
Candidate predictors for detrimental transitions were considered based on patients’ baseline characteristics, severity of illness, and microbiology at the initial presentation along with the antibiotic therapy used to treat the index infection. A random forest tree algorithm with 500 trees and 5 variables sampled in every split was applied to rank the baseline variables in relationship to their importance in predicting the outcome of interest (ie, detrimental transitions). The 10 most relevant variables were included in the multivariable logistic regression analysis, looking at predictors of detrimental transitions. P values < .05 were considered significant.
We performed 2 sensitivity analyses: first, where only the first transition data were analyzed and, second, where transitions from negative culture to any positive-culture states were considered detrimental transitions. Analyses were done using StataCorp 2017 (StataCorp, College Station, TX)
RESULTS
Over the 6-year study period, 3715 septic patients were diagnosed with bloodstream infections. Of the initial cohort, 990 (26.6%) experienced at least 1 transition and were included in this study (Figure 2).
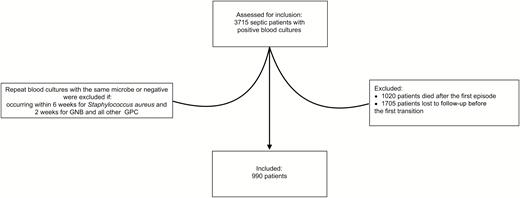
CONSORT diagram for the study cohort of septic patients with bloodstream infections. Abbreviations: CONSORT, Consolidated Standards of Reporting Trials; GNB, gram-negative bacilli; GPC, gram-positive cocci.
Mean age was 54.4 years (Table 1); significant comorbidities were noted (40.5% of patients were immunocompromised); and patients had high-acuity presentations (42.5% were in shock). Initial cultures were mainly attributed to GPC (47.1%), while 19.2% were caused by resistant GNB and 10.9% by Candida spp. (Table 1). The most common bacterial species were methicillin-resistant Staphylococcus aureus, Escherichia coli, Klebsiella spp., methicillin-susceptible S. aureus, and Pseudomonas spp. Beta-lactams constituted the main antimicrobial therapy, with more than 95% of the patients receiving them. Empiric combination therapy with a beta-lactam plus either an aminoglycoside or a fluoroquinolone was prescribed for 45% of patients. Longer hospitalization prior to the bloodstream infection and higher likelihood for inappropriate empiric therapy characterized both the candidemia and rGNB groups (Table 1).
Patient Characteristics for the Entire Cohort and According to Baseline Culture Results
Characteristic . | Entire Cohort, N = 990 . | Susceptible Gram-negative Bacilli, n = 226 . | Gram-positive Cocci, n = 466 . | Resistant Gram-negative Bacilli,a n = 190 . | Candida spp., n = 108 . | P Valueb . |
---|---|---|---|---|---|---|
Age, mean ± standard deviation, y | 54.4 ± 15 | 55.2 ± 14.4 | 53.9 ± 15.1 | 53.3 ± 14.1 | 56.7 ± 17 | .178 |
Male gender (%) | 573 (57.9) | 131 (58) | 279 (59.9) | 112 (59) | 51 (47.2) | .118 |
Prior antibiotics within 90 days (%) | 593 (59.9) | 127 (56.2) | 246 (52.8) | 134 (70.5) | 86 (79.6) | .000 |
Prior bacteremia within 30 days (%) | 190 (19.2) | 46 (20.4) | 86 (18.5) | 38 (20) | 20 (18.5) | .000 |
Admission source | .000 | |||||
Home (%) | 661 (66.8) | 182 (80.5) | 298 (64) | 129 (67.9) | 60 (55.6) | |
Skilled nursing facility | 99 (10%) | 17 (7.5%) | 45 (9.7%) | 25 (13.2%) | 12 (11.1%) | |
Other | 230 (23.2%) | 27 (12%) | 123 (26.4%) | 36 (19%) | 36 (33.3%) | |
Charlson comorbidity index, median (IQR) | 5 (3 to 7) | 5 (3 to 7) | 4 (2 to 7) | 5 (3 to 7) | 5 (2 to 8) | .016 |
No history of surgery (%) | 730 (73.8) | 162 (76.7) | 370 (79.4) | 140 (73.7) | 58 (53.7) | .000 |
Central line (%) | 652 (65.9) | 147 (65) | 263 (56.4) | 150 (79) | 92 (85.2) | .000 |
Duration of hospitalization prior to bacteremia, median (IQR), days | 2 (0, 12) | 2 (0,10) | 0 (0,10) | 7 (0, 17) | 7 (0, 19.5) | .000 |
Septic shock (%) | 421(42.5) | 104 (46) | 179 (38.4% | 92 (48.4) | (46 42.6) | .07 |
Mechanical ventilation (%) | 296(29.9) | 51(22.6) | 137(29.4) | 64(33.7) | 44(40.7) | .004 |
Apache II, median (IQR) | 14(11,18) | 14(10, 18) | 14(11, 18) | 15(11, 19) | 15(10, 18) | .589 |
Microbiologyc | MRSA 145 (14.6%), MSSA 93 (9.4%), Pseudomonas spp. 57 (5.8%), Klebsiella spp. 104 (10.5%) | Escherichia coli 74 (32.7%), Klebsiella spp. 85 (37.6%) | MRSA 145 (31.1%), MSSA 93 (20%), Streptococcus spp. 69 (14.8%) | Pseudomonas spp. 57 (30%), Acinetobacter spp. 14 (7.4%), Enterobacter spp. 42 (22.1%), Klebsiella spp. 19 (10%) | Candida albicans 37 (34.3%), Candida glabrata 22 (20.4%) | |
Inappropriate empiric antibiotics (%) | 232 (23.4) | 14 (6.2) | 104 (22.3) | 60 (31.6) | 54 (50) | .000 |
Initial therapy containing a beta-lactam (%) | 824 (95.8) | 209 (99.1) | 396 (94.1) | 155 (98.7) | 64 (90.1) | .000 |
Initial double therapy: beta-lactam plus either a fluoroquinolone or an aminoglycoside (%) | 445 (45) | 131 (58) | 182 (39.1) | 105 (55.3) | 27 (25) | .000 |
Characteristic . | Entire Cohort, N = 990 . | Susceptible Gram-negative Bacilli, n = 226 . | Gram-positive Cocci, n = 466 . | Resistant Gram-negative Bacilli,a n = 190 . | Candida spp., n = 108 . | P Valueb . |
---|---|---|---|---|---|---|
Age, mean ± standard deviation, y | 54.4 ± 15 | 55.2 ± 14.4 | 53.9 ± 15.1 | 53.3 ± 14.1 | 56.7 ± 17 | .178 |
Male gender (%) | 573 (57.9) | 131 (58) | 279 (59.9) | 112 (59) | 51 (47.2) | .118 |
Prior antibiotics within 90 days (%) | 593 (59.9) | 127 (56.2) | 246 (52.8) | 134 (70.5) | 86 (79.6) | .000 |
Prior bacteremia within 30 days (%) | 190 (19.2) | 46 (20.4) | 86 (18.5) | 38 (20) | 20 (18.5) | .000 |
Admission source | .000 | |||||
Home (%) | 661 (66.8) | 182 (80.5) | 298 (64) | 129 (67.9) | 60 (55.6) | |
Skilled nursing facility | 99 (10%) | 17 (7.5%) | 45 (9.7%) | 25 (13.2%) | 12 (11.1%) | |
Other | 230 (23.2%) | 27 (12%) | 123 (26.4%) | 36 (19%) | 36 (33.3%) | |
Charlson comorbidity index, median (IQR) | 5 (3 to 7) | 5 (3 to 7) | 4 (2 to 7) | 5 (3 to 7) | 5 (2 to 8) | .016 |
No history of surgery (%) | 730 (73.8) | 162 (76.7) | 370 (79.4) | 140 (73.7) | 58 (53.7) | .000 |
Central line (%) | 652 (65.9) | 147 (65) | 263 (56.4) | 150 (79) | 92 (85.2) | .000 |
Duration of hospitalization prior to bacteremia, median (IQR), days | 2 (0, 12) | 2 (0,10) | 0 (0,10) | 7 (0, 17) | 7 (0, 19.5) | .000 |
Septic shock (%) | 421(42.5) | 104 (46) | 179 (38.4% | 92 (48.4) | (46 42.6) | .07 |
Mechanical ventilation (%) | 296(29.9) | 51(22.6) | 137(29.4) | 64(33.7) | 44(40.7) | .004 |
Apache II, median (IQR) | 14(11,18) | 14(10, 18) | 14(11, 18) | 15(11, 19) | 15(10, 18) | .589 |
Microbiologyc | MRSA 145 (14.6%), MSSA 93 (9.4%), Pseudomonas spp. 57 (5.8%), Klebsiella spp. 104 (10.5%) | Escherichia coli 74 (32.7%), Klebsiella spp. 85 (37.6%) | MRSA 145 (31.1%), MSSA 93 (20%), Streptococcus spp. 69 (14.8%) | Pseudomonas spp. 57 (30%), Acinetobacter spp. 14 (7.4%), Enterobacter spp. 42 (22.1%), Klebsiella spp. 19 (10%) | Candida albicans 37 (34.3%), Candida glabrata 22 (20.4%) | |
Inappropriate empiric antibiotics (%) | 232 (23.4) | 14 (6.2) | 104 (22.3) | 60 (31.6) | 54 (50) | .000 |
Initial therapy containing a beta-lactam (%) | 824 (95.8) | 209 (99.1) | 396 (94.1) | 155 (98.7) | 64 (90.1) | .000 |
Initial double therapy: beta-lactam plus either a fluoroquinolone or an aminoglycoside (%) | 445 (45) | 131 (58) | 182 (39.1) | 105 (55.3) | 27 (25) | .000 |
Abbreviations: IQR, interquartile range; MRSA, methicillin-resistant Staphylococcus aureus; MSSA, methicillin-susceptible Staphylococcus aureus.
aResistant gram-negative bacilli defined as resistant to ceftriaxone.
bP value calculated from χ2/Fisher exact test between the 4 groups.
cOther microbiology species isolated: Enterococcus spp., Serratia, Citrobacter, anaerobes, Stenotrophomonas, Achromobacter, coagulase-negative Staphylococcus, Burkholderia, Candida parapsilosis, Candida tropicalis, and Candida krusei.
Patient Characteristics for the Entire Cohort and According to Baseline Culture Results
Characteristic . | Entire Cohort, N = 990 . | Susceptible Gram-negative Bacilli, n = 226 . | Gram-positive Cocci, n = 466 . | Resistant Gram-negative Bacilli,a n = 190 . | Candida spp., n = 108 . | P Valueb . |
---|---|---|---|---|---|---|
Age, mean ± standard deviation, y | 54.4 ± 15 | 55.2 ± 14.4 | 53.9 ± 15.1 | 53.3 ± 14.1 | 56.7 ± 17 | .178 |
Male gender (%) | 573 (57.9) | 131 (58) | 279 (59.9) | 112 (59) | 51 (47.2) | .118 |
Prior antibiotics within 90 days (%) | 593 (59.9) | 127 (56.2) | 246 (52.8) | 134 (70.5) | 86 (79.6) | .000 |
Prior bacteremia within 30 days (%) | 190 (19.2) | 46 (20.4) | 86 (18.5) | 38 (20) | 20 (18.5) | .000 |
Admission source | .000 | |||||
Home (%) | 661 (66.8) | 182 (80.5) | 298 (64) | 129 (67.9) | 60 (55.6) | |
Skilled nursing facility | 99 (10%) | 17 (7.5%) | 45 (9.7%) | 25 (13.2%) | 12 (11.1%) | |
Other | 230 (23.2%) | 27 (12%) | 123 (26.4%) | 36 (19%) | 36 (33.3%) | |
Charlson comorbidity index, median (IQR) | 5 (3 to 7) | 5 (3 to 7) | 4 (2 to 7) | 5 (3 to 7) | 5 (2 to 8) | .016 |
No history of surgery (%) | 730 (73.8) | 162 (76.7) | 370 (79.4) | 140 (73.7) | 58 (53.7) | .000 |
Central line (%) | 652 (65.9) | 147 (65) | 263 (56.4) | 150 (79) | 92 (85.2) | .000 |
Duration of hospitalization prior to bacteremia, median (IQR), days | 2 (0, 12) | 2 (0,10) | 0 (0,10) | 7 (0, 17) | 7 (0, 19.5) | .000 |
Septic shock (%) | 421(42.5) | 104 (46) | 179 (38.4% | 92 (48.4) | (46 42.6) | .07 |
Mechanical ventilation (%) | 296(29.9) | 51(22.6) | 137(29.4) | 64(33.7) | 44(40.7) | .004 |
Apache II, median (IQR) | 14(11,18) | 14(10, 18) | 14(11, 18) | 15(11, 19) | 15(10, 18) | .589 |
Microbiologyc | MRSA 145 (14.6%), MSSA 93 (9.4%), Pseudomonas spp. 57 (5.8%), Klebsiella spp. 104 (10.5%) | Escherichia coli 74 (32.7%), Klebsiella spp. 85 (37.6%) | MRSA 145 (31.1%), MSSA 93 (20%), Streptococcus spp. 69 (14.8%) | Pseudomonas spp. 57 (30%), Acinetobacter spp. 14 (7.4%), Enterobacter spp. 42 (22.1%), Klebsiella spp. 19 (10%) | Candida albicans 37 (34.3%), Candida glabrata 22 (20.4%) | |
Inappropriate empiric antibiotics (%) | 232 (23.4) | 14 (6.2) | 104 (22.3) | 60 (31.6) | 54 (50) | .000 |
Initial therapy containing a beta-lactam (%) | 824 (95.8) | 209 (99.1) | 396 (94.1) | 155 (98.7) | 64 (90.1) | .000 |
Initial double therapy: beta-lactam plus either a fluoroquinolone or an aminoglycoside (%) | 445 (45) | 131 (58) | 182 (39.1) | 105 (55.3) | 27 (25) | .000 |
Characteristic . | Entire Cohort, N = 990 . | Susceptible Gram-negative Bacilli, n = 226 . | Gram-positive Cocci, n = 466 . | Resistant Gram-negative Bacilli,a n = 190 . | Candida spp., n = 108 . | P Valueb . |
---|---|---|---|---|---|---|
Age, mean ± standard deviation, y | 54.4 ± 15 | 55.2 ± 14.4 | 53.9 ± 15.1 | 53.3 ± 14.1 | 56.7 ± 17 | .178 |
Male gender (%) | 573 (57.9) | 131 (58) | 279 (59.9) | 112 (59) | 51 (47.2) | .118 |
Prior antibiotics within 90 days (%) | 593 (59.9) | 127 (56.2) | 246 (52.8) | 134 (70.5) | 86 (79.6) | .000 |
Prior bacteremia within 30 days (%) | 190 (19.2) | 46 (20.4) | 86 (18.5) | 38 (20) | 20 (18.5) | .000 |
Admission source | .000 | |||||
Home (%) | 661 (66.8) | 182 (80.5) | 298 (64) | 129 (67.9) | 60 (55.6) | |
Skilled nursing facility | 99 (10%) | 17 (7.5%) | 45 (9.7%) | 25 (13.2%) | 12 (11.1%) | |
Other | 230 (23.2%) | 27 (12%) | 123 (26.4%) | 36 (19%) | 36 (33.3%) | |
Charlson comorbidity index, median (IQR) | 5 (3 to 7) | 5 (3 to 7) | 4 (2 to 7) | 5 (3 to 7) | 5 (2 to 8) | .016 |
No history of surgery (%) | 730 (73.8) | 162 (76.7) | 370 (79.4) | 140 (73.7) | 58 (53.7) | .000 |
Central line (%) | 652 (65.9) | 147 (65) | 263 (56.4) | 150 (79) | 92 (85.2) | .000 |
Duration of hospitalization prior to bacteremia, median (IQR), days | 2 (0, 12) | 2 (0,10) | 0 (0,10) | 7 (0, 17) | 7 (0, 19.5) | .000 |
Septic shock (%) | 421(42.5) | 104 (46) | 179 (38.4% | 92 (48.4) | (46 42.6) | .07 |
Mechanical ventilation (%) | 296(29.9) | 51(22.6) | 137(29.4) | 64(33.7) | 44(40.7) | .004 |
Apache II, median (IQR) | 14(11,18) | 14(10, 18) | 14(11, 18) | 15(11, 19) | 15(10, 18) | .589 |
Microbiologyc | MRSA 145 (14.6%), MSSA 93 (9.4%), Pseudomonas spp. 57 (5.8%), Klebsiella spp. 104 (10.5%) | Escherichia coli 74 (32.7%), Klebsiella spp. 85 (37.6%) | MRSA 145 (31.1%), MSSA 93 (20%), Streptococcus spp. 69 (14.8%) | Pseudomonas spp. 57 (30%), Acinetobacter spp. 14 (7.4%), Enterobacter spp. 42 (22.1%), Klebsiella spp. 19 (10%) | Candida albicans 37 (34.3%), Candida glabrata 22 (20.4%) | |
Inappropriate empiric antibiotics (%) | 232 (23.4) | 14 (6.2) | 104 (22.3) | 60 (31.6) | 54 (50) | .000 |
Initial therapy containing a beta-lactam (%) | 824 (95.8) | 209 (99.1) | 396 (94.1) | 155 (98.7) | 64 (90.1) | .000 |
Initial double therapy: beta-lactam plus either a fluoroquinolone or an aminoglycoside (%) | 445 (45) | 131 (58) | 182 (39.1) | 105 (55.3) | 27 (25) | .000 |
Abbreviations: IQR, interquartile range; MRSA, methicillin-resistant Staphylococcus aureus; MSSA, methicillin-susceptible Staphylococcus aureus.
aResistant gram-negative bacilli defined as resistant to ceftriaxone.
bP value calculated from χ2/Fisher exact test between the 4 groups.
cOther microbiology species isolated: Enterococcus spp., Serratia, Citrobacter, anaerobes, Stenotrophomonas, Achromobacter, coagulase-negative Staphylococcus, Burkholderia, Candida parapsilosis, Candida tropicalis, and Candida krusei.
During the 6-month follow-up period, the study population experienced 4282 transitions. The median number of transitions per patient was 3 (interquartile range, 2–5). Of the study patients, 25% developed a second bloodstream infection within the first 23 days and 50% within 60 days. Nondetrimental transitions exceeded detrimental transitions, with 4044 (94.5%) nondetrimental vs 238 (5.5%) detrimental transitions. Table 2 and Supplementary Figure 1 depict the transitions between the 5 states for a 21-day cycle. Of the 4282 transitions, 3722 (86.9%) were to negative culture, 96 (2.2%) to sGNB, 226 (5.3%) to GPC, 125 (2.9%) to rGNB, and 113 (2.6%) to Candida states. In general, transitions to negative culture, GPC, and sGNB happened from every state but were less frequent from rGNB and Candida states. The opposite also held true; transitions to rGNB and Candida states occurred from all states but were more frequent from rGNB and Candida.
State . | Negative Culture . | Susceptible GNB . | GPC . | Resistant GNB . | Candida spp. . |
---|---|---|---|---|---|
Negative culture | 87.9 | 90.4 | 86.4 | 83.3 | 67.6 |
Susceptible GNB | 2.7 | 1.5 | 1.1 | 0 | 1.4 |
GPC | 5.1 | 4.2 | 3.6 | 6.8 | 14.8 |
Resistant GNB | 2.7 | 1.9 | 3.3 | 3.4 | 7 |
Candida spp. | 1.6 | 1.9 | 5.6 | 6.4 | 9.2 |
State . | Negative Culture . | Susceptible GNB . | GPC . | Resistant GNB . | Candida spp. . |
---|---|---|---|---|---|
Negative culture | 87.9 | 90.4 | 86.4 | 83.3 | 67.6 |
Susceptible GNB | 2.7 | 1.5 | 1.1 | 0 | 1.4 |
GPC | 5.1 | 4.2 | 3.6 | 6.8 | 14.8 |
Resistant GNB | 2.7 | 1.9 | 3.3 | 3.4 | 7 |
Candida spp. | 1.6 | 1.9 | 5.6 | 6.4 | 9.2 |
Column names indicate initial states and horizontal rows show states they transition to. Numbers represent % of transitions per columns.
Abbreviations: GNB, gram-negative bacilli; GPC, gram-positive cocci.
State . | Negative Culture . | Susceptible GNB . | GPC . | Resistant GNB . | Candida spp. . |
---|---|---|---|---|---|
Negative culture | 87.9 | 90.4 | 86.4 | 83.3 | 67.6 |
Susceptible GNB | 2.7 | 1.5 | 1.1 | 0 | 1.4 |
GPC | 5.1 | 4.2 | 3.6 | 6.8 | 14.8 |
Resistant GNB | 2.7 | 1.9 | 3.3 | 3.4 | 7 |
Candida spp. | 1.6 | 1.9 | 5.6 | 6.4 | 9.2 |
State . | Negative Culture . | Susceptible GNB . | GPC . | Resistant GNB . | Candida spp. . |
---|---|---|---|---|---|
Negative culture | 87.9 | 90.4 | 86.4 | 83.3 | 67.6 |
Susceptible GNB | 2.7 | 1.5 | 1.1 | 0 | 1.4 |
GPC | 5.1 | 4.2 | 3.6 | 6.8 | 14.8 |
Resistant GNB | 2.7 | 1.9 | 3.3 | 3.4 | 7 |
Candida spp. | 1.6 | 1.9 | 5.6 | 6.4 | 9.2 |
Column names indicate initial states and horizontal rows show states they transition to. Numbers represent % of transitions per columns.
Abbreviations: GNB, gram-negative bacilli; GPC, gram-positive cocci.
Recent use of antibiotics, inappropriate empiric therapy, and initial bloodstream infection caused by rGNB or Candida spp. were significantly associated with detrimental transitions (Table 3). Empiric double therapy with beta-lactams plus either an aminoglycoside or a fluoroquinolone was protective against detrimental transitions (odds ratio, 0.6; 95% confidence interval, 04. to 0.7; P = .000).
Characteristic . | Odds Ratio (95% Confidence Interval) . | P Value . |
---|---|---|
Prior antibiotics within 90 days | 1.5 (1.1–2) | .005 |
Initial inappropriate antibiotics | 1.6 (1.2–2.1) | .003 |
Resistant gram-negative bacilli as cause of the initial bloodstream infection | 2 (1.5–2.8) | .000 |
Candida as cause of the initial bloodstream infection | 1.5 (1.02–2.3) | .04 |
Initial double therapya | 0.6 (.4–.7) | .000 |
Characteristic . | Odds Ratio (95% Confidence Interval) . | P Value . |
---|---|---|
Prior antibiotics within 90 days | 1.5 (1.1–2) | .005 |
Initial inappropriate antibiotics | 1.6 (1.2–2.1) | .003 |
Resistant gram-negative bacilli as cause of the initial bloodstream infection | 2 (1.5–2.8) | .000 |
Candida as cause of the initial bloodstream infection | 1.5 (1.02–2.3) | .04 |
Initial double therapya | 0.6 (.4–.7) | .000 |
A multivariable logistic regression model was fit to assess factors associated with “detrimental transitions.” The StataCorp program chi-squared automatic interaction detection forest was used to construct a random forest with 500 trees and 5 variables sampled in every split. A variable importance measure was used to rank the variables in relation to their importance in predicting a detrimental transition. These variables (admission source, presence of central vein catheter, total parenteral nutrition, double initial antibiotic regimen, prior bacteremia, inappropriate initial empiric antibiotic therapy, gender, prior antibiotics, hemodialysis, immunosuppression) along with age and initial microbiology groups (susceptible gram-positive bacilli, gram-positive cocci, resistant gram-negative bacilli, Candida spp.) were included in the multivariable logistic regression.
aBeta-lactam plus either a fluoroquinolone or aminoglycoside.
Characteristic . | Odds Ratio (95% Confidence Interval) . | P Value . |
---|---|---|
Prior antibiotics within 90 days | 1.5 (1.1–2) | .005 |
Initial inappropriate antibiotics | 1.6 (1.2–2.1) | .003 |
Resistant gram-negative bacilli as cause of the initial bloodstream infection | 2 (1.5–2.8) | .000 |
Candida as cause of the initial bloodstream infection | 1.5 (1.02–2.3) | .04 |
Initial double therapya | 0.6 (.4–.7) | .000 |
Characteristic . | Odds Ratio (95% Confidence Interval) . | P Value . |
---|---|---|
Prior antibiotics within 90 days | 1.5 (1.1–2) | .005 |
Initial inappropriate antibiotics | 1.6 (1.2–2.1) | .003 |
Resistant gram-negative bacilli as cause of the initial bloodstream infection | 2 (1.5–2.8) | .000 |
Candida as cause of the initial bloodstream infection | 1.5 (1.02–2.3) | .04 |
Initial double therapya | 0.6 (.4–.7) | .000 |
A multivariable logistic regression model was fit to assess factors associated with “detrimental transitions.” The StataCorp program chi-squared automatic interaction detection forest was used to construct a random forest with 500 trees and 5 variables sampled in every split. A variable importance measure was used to rank the variables in relation to their importance in predicting a detrimental transition. These variables (admission source, presence of central vein catheter, total parenteral nutrition, double initial antibiotic regimen, prior bacteremia, inappropriate initial empiric antibiotic therapy, gender, prior antibiotics, hemodialysis, immunosuppression) along with age and initial microbiology groups (susceptible gram-positive bacilli, gram-positive cocci, resistant gram-negative bacilli, Candida spp.) were included in the multivariable logistic regression.
aBeta-lactam plus either a fluoroquinolone or aminoglycoside.
Sensitivity analyses that looked at only the first transition confirmed the same risk and protective factors for detrimental transitions. Labeling transitions between negative cultures and any positive cultures as detrimental transitions yielded the same risk factors.
DISCUSSION
Using a novel analytical approach, we determined that baseline features including treatment characteristics may influence the microbiology of subsequent bloodstream infections in septic patients with bacteremia. Traditionally, it has been assumed that patients progress from infections caused by susceptible microbes to either negative cultures or MDR pathogens when they continue to spend time in contact with the healthcare system and are exposed to broad-spectrum antibiotics, especially in areas known to harbor antibiotic-resistant pathogens [14, 15]. Our observational study challenges these assumptions and suggests that other trajectories are possible in which patients, for instance, may transition back to bacteremia caused by susceptible pathogens even shortly after experiencing an antibiotic-resistant infection.
Detrimental transitions appear to be influenced by the initial microbiology results and by the antibiotic treatments associated with both the index infection and other infections that occured within the prior 90 days. Having a bloodstream infection caused by resistant microbes (rGNB and Candida spp.) may intuitively imply multiple exposures to the healthcare system [16, 17]. Indeed, this cluster of patients seemed to experience repeated transitions to states of drug-resistant bacteremias. Not unexpectedly, infections attributed to resistant GNB and Candida spp. occurred in sicker patients with prolonged hospital stays.
In addition to the initial microbiology, we found that antibiotics, both previous exposures and acutely prescribed treatments for the index bacteremia, were associated with detrimental transitions. It has been well described across types of infections and pathogens that initial appropriate antibiotics reduce mortality in sepsis [1, 18]. Our study also suggests that initial inappropriate antibiotic therapy may lead to detrimental transitions defined by bloodstream infection caused by rGNB and Candida spp. To avoid the competing effect of mortality, we excluded patients who died during their hospitalization. One plausible hypothesis may be that inappropriate antibiotics prolong the overall course of therapy, with very short exposures to aminoglycosides and fluoroquinolones preventing emergence of resistance [19, 20]. Prolonged courses, especially at low doses, can do the opposite [21–23]. Preventing the microbiome from recovering leaves room for colonization and then subsequent infection with resistant, hospital-adapted microbes [24].
We also found that combination therapy with a beta-lactam and either an aminoglycoside or a fluoroquinolone administered for the index episode is protective against future detrimental transitions. In multiple other infections such as tuberculosis and HIV, it is accepted that development of resistance is avoided by using multiple agents concomitantly [25]. Double therapy has been commonly used in cystic fibrosis patients [26, 27]. A general review of 173 trials found that combination therapy, usually with beta-lactam and aminoglycosides, reduced the emergence of bacterial resistance from 5.6% to 3.1% [28]. Double therapy may also protect against emergence of resistance by increasing the heterogeneity of selection pressure. Early and effective treatment should minimize the spread of resistance [29]. Even though resistance has been connected to antibiotic consumption [30], historical withdrawals have failed to show a consistent benefit in reducing resistance [31].
The use of transition probabilities is common in areas of scientific inquiry such as engineering where they are used to calculate infrastructure repair intervals. Their use in the medical literature is uncommon. Markov models have been used to study chronic diseases such as HIV and hypertension [10, 32]. More recently, this methodology has been applied to chronic obstructive pulmonary disease to prove it is not uniformly progressive [33]. Markov models also provide an elevated platform from which to evaluate a disease process, making it possible to discern the impact of baseline characteristics on future events. For example, imagine a situation where a therapy will only affect cure of a disease state or alternatively will affect the transition probability to that disease state (eg, primary or secondary prevention). In the former case, physicians will be able to cure the disease state (eg, stenting for myocardial infarction); however, because the transition probability remains unaltered, the disease state will recur (eg, myocardial infarction in untreated diabetes). In sepsis survivors, long-term mortality is influenced by characteristics present at the index hospitalization [34]. If we continue to focus only on the acute treatment, patients may continue to return with subsequent episodes of sepsis.
The strength of our analysis is that we included septic patients with bloodstream infections in an effort to minimize the misclassification bias inherent to culture-negative and pneumonia-driven sepsis. We felt that a robust microbiologic diagnosis would be beneficial when testing a new methodology. The limitations of our study include having a single center and the inherent flaws of retrospective study design. It is also likely that we did not identify all risk factors for detrimental transitions. We did not account for the duration of the antibiotic therapy administered, as this hypothesis stemmed from our findings. We also did not control for transmissibility of resistant microbes. Since we excluded patients lost to follow-up, there is a chance that we failed to capture predominantly negative cultures. By keeping the inclusion and follow-up criteria consistent, we may have missed some infected nonseptic patients or septic patients without blood cultures. Consistent with previous studies, we have looked at only the baseline characteristics, but subsequent antibiotics administered may add important information.
In most chronic diseases, clinicians focus arduously on secondary prevention. This aspect has been lacking from the study of infectious diseases. Our findings add to the growing body of literature that demonstrates the “chronicity” of sepsis and infectious diseases with readmissions after the index episode [35, 36] and point to modifiable risk factors that may impact the trajectory of septic patients with bloodstream infections. We hope that our study will help clinicians anticipate the trajectory of resistance during antibiotic therapy. Moreover, it may help in the design of future studies aimed at preventing detrimental transitions.
In summary, our findings suggest that baseline characteristics including prescribed antibiotics can identify patients at risk for subsequent bloodstream infections caused by resistant microbes. By altering the initial treatment of bloodstream infections, we may be able to influence not only the index episode but, more importantly, future bacteremic states. Appropriate empiric antibiotics including combination regimens may protect against future resistant infections. As our understanding of the trajectory of sepsis evolves and survival improves, we may start to focus more on modifying the transitions and not just on treating the acute disease states.
Supplementary Data
Supplementary materials are available at Clinical Infectious Diseases online. Consisting of data provided by the authors to benefit the reader, the posted materials are not copyedited and are the sole responsibility of the authors, so questions or comments should be addressed to the corresponding author.
Notes
Financial support. M. H. K.’s efforts are supported by the Barnes-Jewish Hospital Foundation.
Potential conflicts of interest. All other authors report no potential conflicts. All authors have submitted the ICMJE Form for Disclosure of Potential Conflicts of Interest. Conflicts that the editors consider relevant to the content of the manuscript have been disclosed.
References