-
PDF
- Split View
-
Views
-
Cite
Cite
Giovanni Oliveros, Charles H Wallace, Osama Chaudry, Qiao Liu, Yue Qiu, Lei Xie, Patricia Rockwell, Maria E Figueiredo-Pereira, Peter A Serrano, Repurposing ibudilast to mitigate Alzheimer’s disease by targeting inflammation, Brain, Volume 146, Issue 3, March 2023, Pages 898–911, https://doi.org/10.1093/brain/awac136
- Share Icon Share
Abstract
Alzheimer’s disease is a multifactorial disease that exhibits cognitive deficits, neuronal loss, amyloid plaques, neurofibrillary tangles and neuroinflammation in the brain. Hence, a multi-target drug would improve treatment efficacy. We applied a new multi-scale predictive modelling framework that integrates machine learning with biophysics and systems pharmacology to screen drugs for Alzheimer’s disease using patients’ tissue samples. Our predictive modelling framework identified ibudilast as a drug with repurposing potential to treat Alzheimer’s disease. Ibudilast is a multi-target drug, as it is a phosphodiesterase inhibitor and toll-like receptor 4 (TLR4) antagonist. In addition, we predict that ibudilast inhibits off-target kinases (e.g. IRAK1 and GSG2). In Japan and other Asian countries, ibudilast is approved for treating asthma and stroke due to its anti-inflammatory potential.
Based on these previous studies and on our predictions, we tested for the first time the efficacy of ibudilast in Fisher transgenic 344-AD rats. This transgenic rat model is unique as it exhibits hippocampal-dependent spatial learning and memory deficits and Alzheimer’s disease pathology, including hippocampal amyloid plaques, tau paired-helical filaments, neuronal loss and microgliosis, in a progressive age-dependent manner that mimics the pathology observed in Alzheimer’s disease patients. Following long-term treatment with ibudilast, transgenic rats were evaluated at 11 months of age for spatial memory performance and Alzheimer’s disease pathology.
We demonstrate that ibudilast-treatment of transgenic rats mitigated hippocampal-dependent spatial memory deficits, as well as hippocampal (hilar subregion) amyloid plaque and tau paired-helical filament load, and microgliosis compared to untreated transgenic rat. Neuronal density analysed across all hippocampal regions was similar in ibudilast-treated transgenic compared to untreated transgenic rats. Interestingly, RNA sequencing analysis of hippocampal tissue showed that ibudilast-treatment affects gene expression levels of the TLR and ubiquitin-proteasome pathways differentially in male and female transgenic rats. Based on the TLR4 signalling pathway, our RNA sequencing data suggest that ibudilast-treatment inhibits IRAK1 activity by increasing expression of its negative regulator IRAK3, and/or by altering TRAF6 and other TLR-related ubiquitin ligase and conjugase levels.
Our results support that ibudilast can serve as a repurposed drug that targets multiple pathways including TLR signalling and the ubiquitin/proteasome pathway to reduce cognitive deficits and pathology relevant to Alzheimer’s disease.
Introduction
Alzheimer’s disease currently affects about 5.8 million Americans, the majority of whom are over the age of 65 years, with a subpopulation of patients under this age cut-off. Experts speculate that by 2050, expenditure set aside for Alzheimer’s disease will exceed $2.8 trillion and over 14 million people over the age of 65 years will be affected.1 The hallmarks of this disease consist of neuronal loss, amyloid plaques, neurofibrillary tangles and neuroinflammation detected in the brains of Alzheimer’s disease patients.2 In addition, cognitive deficits associated with Alzheimer’s disease include loss of memory and progressive impairment of thought and reasoning. However, the catalysts for setting Alzheimer’s disease into motion are still being investigated. Aside from the aforementioned changes, evidence has emerged to suggest that there is a genetic predisposition to Alzheimer’s disease that leaves vulnerable populations more at risk, with environmental factors and lifestyle possibly playing a role in disease progression.3
US Food and Drug Administration-approved drugs designed to treat Alzheimer’s disease by targeting plaques or tangles do not halt disease progression, having a reported 99.6% failure rate.4 This failure can be attributed to the complexity of Alzheimer’s disease, not just the plaques or tangles.2 One such factor contributing to the pathology of Alzheimer’s disease is neuroinflammation. This occurs when there is cellular damage in the brain, leading to recruitment of microglia and astrocytes to clear the damage and restore neuronal function.5 However, chronic neuroinflammation resulting from persistent activation of pro-inflammatory responses in combination with delays in anti-inflammatory responses causes buildup of cellular debris and neuronal damage. Selecting neuroinflammation as a therapeutic strategy to combat Alzheimer’s disease may prove beneficial, considering the promise this strategy has shown in treating other neurodegenerative disorders such as amyotrophic lateral sclerosis and multiple sclerosis.6,7 Therapeutics aimed at these two disorders showed promise by amplifying the neuroprotective properties of glial cells, a strategy that could be mimicked by targeting Alzheimer’s disease pathology from the neuroinflammatory perspective.
To identify existing drugs that can modulate neuroinflammation in Alzheimer’s disease, we applied a predictive modelling framework that integrates machine learning with biophysics and systems pharmacology to repurpose approved drugs for Alzheimer’s disease treatment. Our model predicted that ibudilast (IBU), a nonselective phosphodiesterase inhibitor and a TLR4 antagonist,8–12 inhibits IRAK1 as an off-target, modulates multiple Alzheimer’s disease-associated pathways and reverses the molecular phenotypes of Alzheimer’s disease patients. The primary mode of action of IBU is by way of neuroprotection and anti-inflammatory effects. In a clinical trial for opioid withdrawal, IBU was shown to be a TLR4 antagonist and to reduce glial cell activation.13 Moreover, IBU has shown promise in clinical trials against multiple sclerosis and amyotrophic lateral sclerosis.14
Results from a phase-two clinical trial for progressive multiple sclerosis reported that after 96 weeks of treatment, IBU-treated patients exhibited less brain atrophy than placebo-treated patients.15 IBU effects were significant, provided that intervention occurred in the early stages of the disease.16 Based on this study, we evaluated the effects of long-term (6 month) treatment of IBU in an age-dependent transgenic rat model of Alzheimer’s disease for improvement in spatial memory and mitigation of hippocampal Alzheimer’s disease pathology.
Materials and methods
TgF-344AD transgenic rat model of Alzheimer’s disease and ibudilast treatment
Fisher transgenic 344-AD (Tg-AD) rats expressing human Swedish amyloid precursor protein (APPsw) and Δ exon 9 presenelin-1 (PS1ΔE9)17 were purchased from the Rat Resource and Research Center (RRRC, Columbia, MO, USA) at 4 weeks of age. The Tg-AD rats (16 males, 13 females) and wild type (WT) (15 males, 15 females) were housed in pairs upon arrival and maintained on a 12 h light/dark cycle with food and water available ad libitum. The Institutional Animal Care and Use Committee at Hunter College approved all animal procedures.
At 5 months, Tg-AD and WT rats began IBU (cat. no. HY-B0763, MCE) treatment, 10 mg/kg body weight administered in rodent chow (Research Diets Inc.; Supplementary Figs 1 and 2). Untreated Tg-AD and WT controls received non-drug chow. Following 6 months of IBU treatment, rats (11 months of age) were tested for cognitive behaviour prior to sacrificing. Brains were examined by immunohistochemistry (IHC) and RNA sequencing (RNAseq) analyses.
Cognitive behaviour assessment
We used the Active Place Avoidance Task (aPAT) to assess hippocampal spatial learning and memory performance.18 The arena was comprised of a rotating platform that operated at one revolution per minute (rpm), with visual cues at the four walls of the room. The arena was divided into four quadrants, one of which was assigned as a shock zone. If a rat entered the shock zone and remained there for at least 1.5 s, it received a 0.2 amp shock. A shock was given every 1.5 s until the rat left the shock zone. An overhead camera was perched atop the arena to track the location of the rat as it performed the task. Rats were given a 10 min habituation trial (no shock), followed by six 10-min training trails, with a 10 min inter-trial interval in the home cage. Twenty-four hours after the last training trial, rats received one 5-min test trial with the shock zone turned off.
Immunofluorescence
At 11 months of age, the rats were anaesthetized intraperitoneally with ketamine (100 mg/kg) and xylazine (10 mg/kg) and transcardially perfused with cold RNAse-free 1× PBS. Left hippocampi were removed and snap frozen for RNAseq analysis. Right hemispheres were submersion-fixed with 4% paraformaldehyde for 48 h at 4C and cryoprotected (30% sucrose/PBS), then flash frozen in 2-methybutane and stored at −80C until cryostat sectioned. Coronal sections through the hippocampus (30 µm) were collected serially and stored at −20°C in cryoprotectant (30% glycerol and ethylene glycol in 1× PBS). IHC analyses were restricted to hippocampal sections −3.36 mm to −4.36 mm relative to bregma19 and followed a protocol for mounted sections described previously.20
Amyloid-β plaques and microglia
Primary antibodies were diluted at 1:1000 for amyloid-β (Aβ) (4G8 antibody, epitope amino acids 17–24, Biolegend—mouse—cat. no. 800708) and 1:500 for microglia (Iba1, Wako—rabbit—cat. no. 019-19741). Secondary antibodies included Alexa Fluor goat anti-mouse 568 (cat. no. A-11031) and Alexa Fluor goat anti-rabbit 488 (cat. no. A-11008), both at a 1:250 concentration and from Life Technologies, Thermo Fisher. Following immunostaining, VectaShield® mounting media with DAPI (cat. no. H-1200-10) was used and slides were stored at 4°C in the dark until imaged. We used a Zeiss Axio Imager microscope equipped with AxioVision software to capture whole hippocampal images as ZVI files that were loaded onto ImageJ (National Institutes of Health).
Ramified, reactive and amoeboid microglia phenotypes were analysed for circularity based on the ImageJ form factor (FF = 4π × area/perimeter2): ramified (FF < 0.50), reactive (FF, 0.50–0.70) and amoeboid (FF > 0.70), where microglia with an area between 50–1000 µm2 were included.21,22 Microglia signal intensity (O.D.) was quantified based on our previous reports.20,23
Neurons and tau paired helical filaments
A similar IHC protocol as for Aβ and Iba1 was used, with the following changes: (i) washes with 0.3% TritonX (Thermo Fisher, cat. no. PI85112) in 1X TBS (TBS-T); (ii) blocking with dry milk (5% by weight) in 0.3% TBS-T24; and (iii) primary antibodies prepared in the same blocking buffer. Primary antibodies diluted to 1:250: PHF1 antibody for tau paired helical filaments (PHFs) (courtesy of the late Dr Peter Davies) and NeuN (Millipore—chicken—cat. no. ABN91) for neuronal density. The secondary antibody cocktail was prepared in 20% Superblock/TBS in 0.03% TBS-T. Secondary antibodies used: Alexa Fluor IgG1 goat anti-mouse 568 (1:80 dilution, cat. no. A-21124) and Alexa Fluor goat anti-chicken 488 (1:250 dilution, cat. no. 11039) both from Life Technologies, Thermo Fisher.
Images of hippocampal subfields [cornu ammonis 1 (CA1), cornu ammonis 3 (CA3), dentate gyrus (DG) and subiculum (SB)] were analysed with custom batch-processing macroscripts created for each channel/marker. Pixel-intensities were calculated from the images at 16-bit intensity bins. The positive signal within each cropped image was extracted using a formula as previously described by Kerfoot et al.25 Positive signals were then measured, masks created and merged when co-localization analyses were required.
RNAseq analysis
Hippocampi of five IBU-treated and five untreated transgenic rats of each sex were analysed for gene expression by RNAseq at the UCLA Technology Center for Genomics & Bioinformatics outsourcing service. Gene expression data were normalized as reads per million using the Trimmed Mean of the M-values method. Differentially expressed genes from IBU-treated transgenic rats were determined using the edgeR program.26 Reads per million were analysed for fold-change, P-values and false discovery rate (FDR) for each gene (Supplementary Table 6).
Statistical analysis
For our behavioural analyses, we required a minimum of 14 animals per condition in order to reach statistical significance at the 5% level. We processed all brains for IHC and all were analysed for the various markers, except for four brains which were damaged during processing. For every IHC data set we ran a PRISM ROUT outlier test set at Q = 1%. There were no outliers detected for Aβ, PHF1 or NeuN datasets. There were three outliers detected for the Iba1 data set.
For IHC and behavioural assessments, two-way ANOVAs were used to analyse differences between genotype and treatment. Repeated measures ANOVA were used to assess differences across time for behavioural analyses. For memory retention testing and microglial analysis, ordinary two-way ANOVA were used. Post hoc analyses used controlled Sidak’s-corrected t-test comparisons. One-tail independent t-tests were used for analysis of neuronal density, Aβ plaques and tau PHF accumulation. For image quantification, normalization of pixel intensity values across images was carried out utilizing the rolling ball algorithm.27 The macroscripts necessary for image processing and quantification were added to GitHub https://github.com/GiovanniOliveros33/Ibudilast-Manuscript). The alpha level was set at P < 0.05 with a 95% confidence interval for each effect. GraphPad’s Prism version 8 (La Jolla, CA, USA) was used.
To measure differences in gene expression between IBU treated and untreated male and female transgenic rats, multiple unpaired t-tests with Welch’s correction were used for each comparison. For these analyses, the expression level of each gene for male or female treated transgenic rats was the percent expression of their untreated counterparts normalized to 100%.
Kinase binding assay
To validate our computational predictions, we employed a competition biding assay to detect the binding of IBU to 425 human kinases. The proprietary KINOMEScanTM was performed by Eurofins/DiscoverX (Fremont, CA, USA). The tests were performed at 10 µM and 100 µM concentrations of levosimendan, respectively. Assay results were reported as % control, calculated as follows:
A lower % control score indicates a stronger interaction. The KINOMEScanTM experiment and data analysis were performed by Eurofins/DiscoverX (Fremont, CA, USA).
Multi-scale predictive modelling of drug action
Derivation of Alzheimer’s disease signature
We selected six out of ten human microglial RNAseq samples (four Alzheimer’s disease, two controls) obtained from ROSMAP projects through the AMP-AD Knowledge Portal28 (Supplementary Table 5). We selected the samples which were identified with unambiguous Alzheimer’s disease or as normal/unaffected controls. The four samples that were excluded from the analyses were samples diagnosed as reflecting a different disorder (labelled ‘Other’) not associated with Alzheimer’s disease. We used Salmon29 to quantify the transcripts and DEseq230 to perform the differential expression analysis. The top differentially expressed genes (adjusted P value < 0.05) were used as Alzheimer’s disease signatures for further analysis.
Phenotypic compound screening
The up- and downregulated genes in the Alzheimer’s disease signature, which also served as LINCS L1000 landmark genes, were used to screen drug-induced expression profiles in the L1000 dataset. The drug-induced expression profiles were processed by the Bayesian signature detection pipeline31 and compared to the Alzheimer’s disease signature by Gene Set Enrichment Analysis (GSEA).32 Drugs with the lowest scores are selected as candidates.
Prediction of genome-wide drug-target interactions
We applied 3D-REMAP to predict drug off-target binding of IBU as described previously.33
Text mining for drug-gene-disease associations
The details of methods were as previously described.33
Prediction of blood–brain barrier permeant
The blood–brain barrier permeant of chemical compounds was predicted using SwissADME.34
Data Availability
Data are reported within the text, table, figures and Supplementary material. The raw data supporting the conclusions of this manuscript will be made available upon publication.
Results
IBU is predicted to be a drug candidate for Alzheimer’s disease treatment
Our structure systems pharmacology pipeline for the first-time integrated structure-based genome-scale off-target predictions and chemical-induced gene expression predictions for screening approved or investigational drugs to target Alzheimer’s disease. The scheme of the pipeline is shown in Supplementary Fig. 3. We ranked drugs by their ability to reverse the gene expression profile of Alzheimer’s disease patients.35 We first obtained the differential gene expression profile of microglia from a group of Alzheimer’s disease patients versus healthy controls in the AMP-AD data portal36 and considered it as the molecular phenotypic signature of Alzheimer’s disease (Supplementary Table 1). Then we compared the drug-induced gene expression profile of approximately 20 000 drugs (dosage < 1 μM) with the Alzheimer’s disease signature such that the downregulated and upregulated genes in Alzheimer’s disease will be upregulated and downregulated by the drug, respectively. Of the top 100 ranked drugs (Supplementary Table 2), three of them are anti-inflammatory drugs, as shown in Supplementary Table 1. Because sulfasalazine and sasapyrine may not be able to permeate the blood–brain-barrier, we focused our studies on IBU.
Using a structure-augmented machine learning method for predicting genome-scale drug-target interactions, we predicted that protein kinases were the off-target of PDE3A inhibitors.37 The KinomeScanTM assay confirmed our predictions. IRAK1 and HASPIN (GSG2) were effectively inhibited by IBU under the concentration of 10 μM with a percentage control of 6.8 and 9.4, respectively (Supplementary Table 3). IRAK1 is involved in the TLR and interleukin-1 signalling pathways and plays a key role in regulating inflammation. Genome-wide association studies discovered that GSG2 is located in the locus associated with Alzheimer’s disease.38 Additionally, KIF proteins, the downstream target of GSG2, are related to Alzheimer’s disease.39 Thus, either or both genes could be involved in disease risk. Under a high concentration of 100 μM, IBU inhibits multiple kinases that are associated with Alzheimer’s disease and inflammation, such as PIK3C3, CDK4, JNK1, JNK3, HIPK2, HIPK3, TAOK1 and TAOK3 (Supplementary Table 4).
The hypothesis that IBU modulates multiple Alzheimer’s disease-associated pathways was further supported by text mining.33 We found that IBU was associated with multiple Alzheimer’s disease pathological processes including TLR/MYD88/NFκB pathways, the TNF pathway, the lipopolysaccharide synthesis pathway and herpes simplex virus infection with FDR < 1.0 × 10−3.
IBU improves spatial learning and memory
The hippocampal-dependent active place avoidance task was used to assess short-term working memory performance on 11-month-old Tg-AD and wild-type (WT) rats (Fig. 1A). Male and female performances were combined to four groups: wild-type untreated (WTNT), wild-type treated with IBU (WTTR), transgenic untreated (TGNT) and transgenic treated with IBU (TGTR). A three-way ANOVA of aPAT performance across six training trials, two drug treatments (IBU treatment and no treatment) and two genotypes (WT and transgenic) with Bonferroni’s post hoc analysis measuring latency to first entrance into the shock zone revealed an overall significant effect of training [F(4.528, 244.5) = 13.28; P < 0.01] and an overall significant effect of genotype [F(1,54) = 5.96; P = 0.02]. No post hoc differences were observed for any analyses performed under this repeated measures ANOVA, nor were IBU treatment effects observed under this three-way analysis. To determine whether short-term working memory deficits were more pronounced during the early acquisition phase (Trials 1–3) versus asymptotic performance phase (Trials 4–6), we analysed these phases separately. Our three-way ANOVA analysis of early acquisition in latency to first entrance showed significant training effects [F(1.794, 96.88) = 9.811; P < 0.01], genotype effects [F(1, 54) = 14.28; P < 0.01] and an effect of IBU treatment with respect to genotype [F(1, 54) = 7.582; P < 0.01]. No significant post hoc differences were observed, nor were there any significant effects observed during the asymptotic performance phase of training.
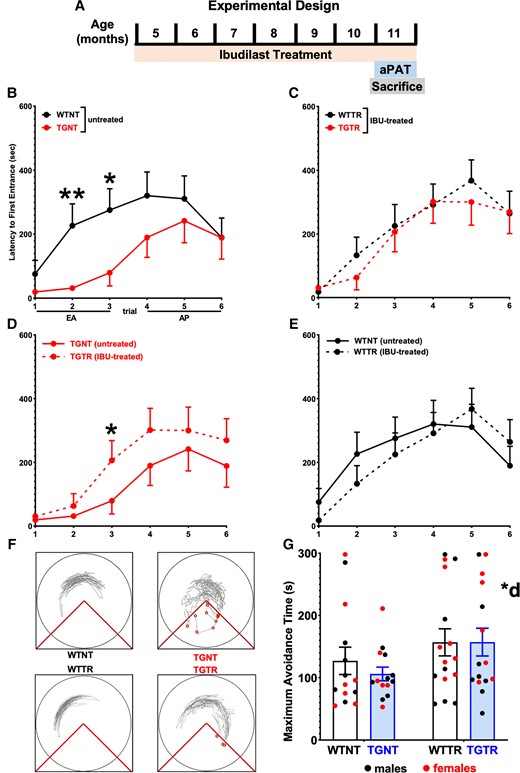
IBU treatment mitigates spatial learning and memory performance deficits. (A) Experimental timeline. (B–E) Latency to first entrance during training. (B) Early acquisition (EA) shows significant differences between WTNT versus TGNT rats. (D) TGTR perform significantly better than TGNT during EA. No differences in EA between (E)WTNT versus WTTR and (C) WTTR and TGTR. (B–E) No differences were observed in asymptotic performance (Trials 4–6) across all comparisons. (F) Track tracing of individual rat performance for Trial 3 across treatment conditions. (G) Test data show overall significant improvement in maximum time to avoid during test following IBU treated versus untreated. Repeated measures two-way ANOVA with Sidak’s post hoc tests were used in B–E. Ordinary two-way ANOVA with Sidak’s post hoc analysis was used in G. n = 14 WTNT, n = 13 TGNT, n = 15 TGTR, n = 16 WTTR. *P < 0.05, **P < 0.01. AP = asymptotic performance; *d = IBU drug treatment effect.
Independent two-way repeated measures ANOVA with Sidak’s post hoc tests were performed across training trials to isolate the effects of either genotype (analyses of WTNT versus TGNT and WTTR versus TGTR) or IBU treatment (WTNT versus WTTR and TGNT versus TGTR) across training trials, again assessing latency to first entrance to the shock zone while considering the early acquisition and asymptotic performance phases of training. Our results show that at 11 months of age, WTNT rats performed significantly better during acquisition (Trials 1–3) compared to TGNT [F(1,25) = 28.53, P < 0.01] with post hoc differences at Trials 2 and 3 (t = 2.839, P < 0.01 and t = 3.494, P = 0.01, respectively; Fig. 1B). Following 6 months of IBU-chow or control chow, TGTR rats show significant improvement during acquisition compared to TGNT controls [F(1,26) = 4.603, P < 0.01] with post hoc differences at Trial 3 (t = 2.639, P = 0.05; Fig. 1D). This enhanced performance was not observed between WTNT and WTTR conditions [F(1,28) = 2.525, P = 0.1233], suggesting beneficial effects of IBU-treatment under pathological conditions (Fig. 1E). TGTR rats performed equivalently to WTTR controls [F(1,29) = 0.4481, P = 0.5085; Fig. 1C]. There were no significant effects observed during asymptotic performance (Trials 4–6) across genotypes or treatments. We also saw that TGTR rats performed significantly worse than WTNT rats in the early acquisition phase [F(1,27) = 6.621, P = 0.02], but not in asymptotic performance phase [F(1,27) = 0.063, P = 0.80] (Supplementary Fig. 4A), while TGNT rats performed significantly worse than WTTR rats [F(1,27) = 4.052, P = 0.03] in early acquisition (Supplementary Fig. 4B). Figure 1F shows the tracking for individual rats during Trial 3 across the four treatment conditions. Twenty-four hours after the last training trials we tested rats for avoidance of the shock zone in the absence of shock. The results show that IBU treated rats performed better than controls for max latency to avoid the shock zone, regardless of genotype [F(1,55) = 4.033, P = 0.0495] (Fig. 1G).
IBU significantly reduces Aβ plaque burden in the dentate gyrus
We evaluated Aβ plaque burden in the hippocampus and within its discrete subregions (Fig. 2A and B), between IBU-treated and untreated Tg-AD rats. We found that Aβ plaque burden following IBU-treatment was not significantly different from untreated Tg-AD rats in the hippocampus collapsed across subregions (Fig. 2C; t = 0.08131, P = 0.9359). Analyses of subregions separately shows significant reduction in Aβ plaque load in the dentate gyrus (Fig. 2F; t = 3.449, P = 0.021). However, no significant effects in CA1 (Fig. 2D; t = 0.5707, P = 0.5735), CA3 (Fig. 2E; t = 0.3351, P = 0.7405) and SB (Fig. 2G; t = 0.3020, P = 0.7652) were identified. Alongside quantification of Aβ plaque load, we also quantified the percent positive signal for DAPI for all rat hippocampi included in the analysis for the entire hippocampus and its regions CA1, CA3, DG and SB. Hippocampi and corresponding regions were compared between transgenic untreated and transgenic-IBU treated rats using two-tailed unpaired t-tests with Welch’s corrections. We found no significant differences between percent positive DAPI signal throughout the hippocampus (P = 0.85) or the subregions CA1 (P = 0.26), CA3 (P = 0.42), DG (P = 0.86) and SB (P = 0.81) between transgenic untreated and IBU-treated transgenic rats (Supplementary Table 7).
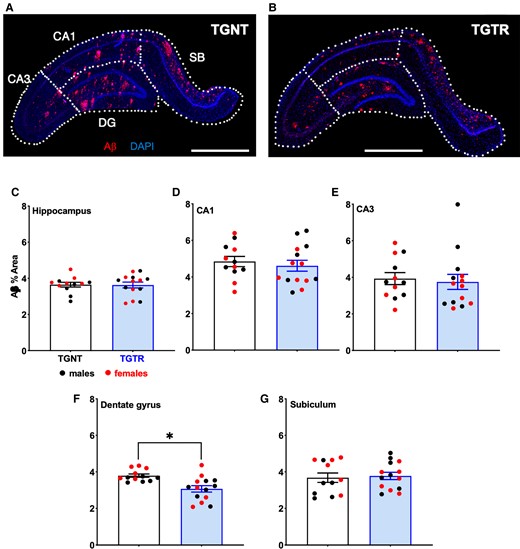
IBU treatment reduces Aβ plaque load regionally across hippocampal subregions. Immunohistochemistry for Aβ plaque load and DAPI for (A) untreated and (B) IBU-treated Tg-AD rats. Scale bar = 1000 µm for A and B. Plaque load was not reduced across hippocampal subregions (C) collapsed or in (D) CA1, (E) CA3 and (G) SB. (F) IBU-treatment significantly reduced plaque load in DG. Unpaired one-tail t-tests with Welch’s corrections were used in C–G. n = 12 TGNT, n = 14 TGTR. *P < 0.05.
IBU significantly reduces tau PHF levels in the dentate gyrus
We used PHF1 staining (red) to analyse tau PHF levels in the hippocampus of Tg-AD rats (Fig. 3A and B). Tau PHF levels were not significantly altered following treatment with IBU when collapsed across hippocampal subregions (Fig. 3C; t = 0.4079, P = 0.6870). Regional analysis of hippocampal subregions showed significant reduction in tau PHF levels in the DG (Fig. 3F; t = 7.295, P < 0.0001). No significant differences between treatments in CA1 (Fig. 3D; t = 1.419, P = 0.1688), CA3 (Fig. 3E; t = 0.9579, P = 0.3485) and SB (Fig. 3G; t = 0.2824, P = 0.7807) were identified.
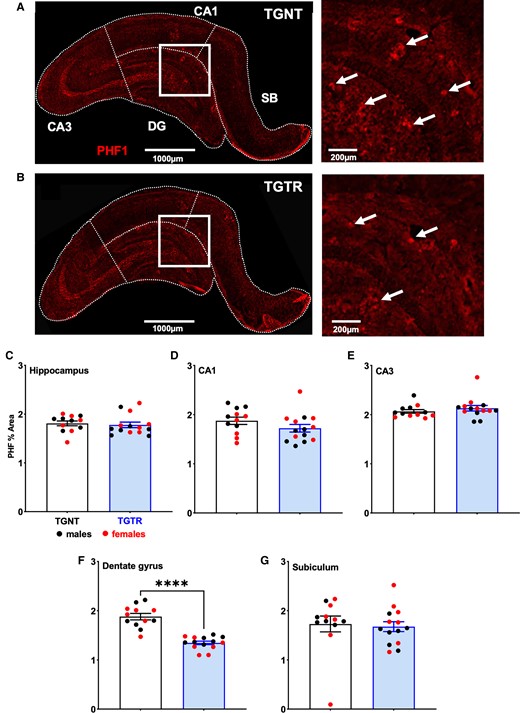
Tau PHFs in the hippocampus are regionally decreased by IBU treatment. Immunohistochemistry for tau PHF (red, PHF1 antibody) across hippocampal subfields for (A) TGNT and (B) TGTR rats. Scale bars = 1000 µm for A and B large panels, 200 µm for A and B small panels. IBU did not affect tau PHF1 reactivity across hippocampal subregions (C) collapsed or in (D) CA1, (E) CA3 and (G) SB. (F) However, IBU-treatment significantly reduced tau PHF1 reactivity in DG. Unpaired one-tail t-tests with Welch’s corrections were used in C–G. n = 12 TGNT, n = 14 TGTR. *P < 0.05.
Effect of IBU on hippocampal neuronal density
Neuronal density across hippocampal subregions (CA1 and CA3 pyramidal cell layers, DG granular cell layer and SB) were assessed with NeuN staining to quantify differentiated adult neurons. Consistent with previous studies,40 we observed a significant loss in NeuN signal in the granular cell layer of DG in untreated transgenic compared to wild type rats (unpublished work, P < 0.01) (Fig. 4A and B). There was significant neuronal loss in the overall hippocampus (P = 0.04; Supplementary Table 8), with greater neuronal loss in the granular cell layer of the dentate gyrus (P < 0.01; Supplementary Table 8). There was no significant neuronal loss in the CA1 (P = 0.24; Supplementary Table 8), CA3 (P = 0.18; Supplementary Table 8) or SB (P = 0.12; Supplementary Table 8). Neuronal density analysed across all hippocampal subregions was similar in IBU-treated transgenic compared to untreated transgenic rats (Fig. 4C; t = 0.2237, P = 0.8250). Additional comparisons showed no significant differences within specific pyramidal cell layers [CA1 (Fig. 4D; t = 1.216, P = 0.2358) or CA3 (Fig. 4E; t = 1.498, P = 0.1474)], granule cell layer of DG (Fig. 4F; t = 1.186, P = 0.2491) or SB (Fig. 4G; t = 1.088, P = 0.2874)].
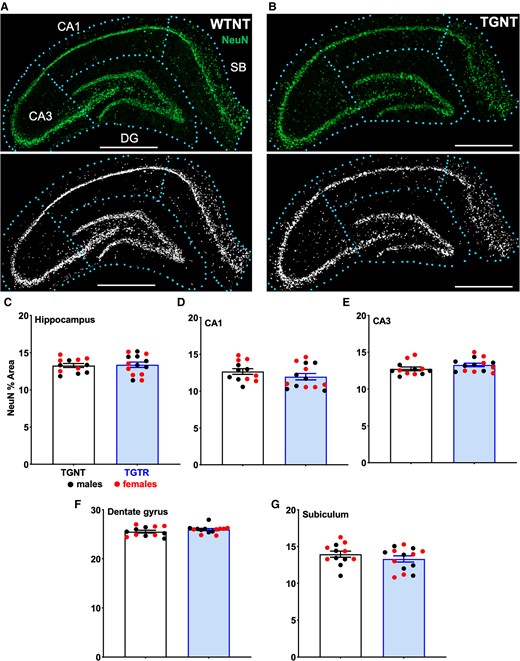
Effect of IBU on hippocampal neuronal density. (A) WTNT rats had significantly higher neuronal density (NeuN staining) in the DG area compared to (B) TGNT rats, P < 0.0001. Corresponding masks are shown in A and B. Analysis of neuronal density between TGNT and TGTR rats showed no significant difference in (C) the hippocampus collapsed across all subregions, (D) CA1, (E) CA3, (F) DG and (G) SB. Scale bar = 1000 µm. Unpaired one-tail t-tests with Welch’s corrections were used in C–G. n = 12 TGNT, n = 14 TGTR. *P < 0.05.
IBU specifically reduces amoeboid/ramified microglia in the hippocampus
Microglia exhibit a remarkable variety of morphologies that are associated with their specific functions. Based on shape and function (Fig. 5A), we considered three microglia groups defined as follows: (i) Ramified, actively engaged in neuronal maintenance and providing neurotrophic factors; (ii) Reactive, responsive to CNS injury; and (iii) Amoeboid, with amorphous cell bodies with pseudopodia that remove cell debris.21 Quantification of total microglia throughout the hippocampus showed that there was an increase in total microglia, particularly in CA1 [F(1,47) = 0.02; P < 0.01; Supplementary Table 9] in transgenic rats when compared to wild-type. IBU treatment remediated this region-specific increase in total microglia [F(1,47) = 5.60; P = 0.02; Supplementary Table 9].
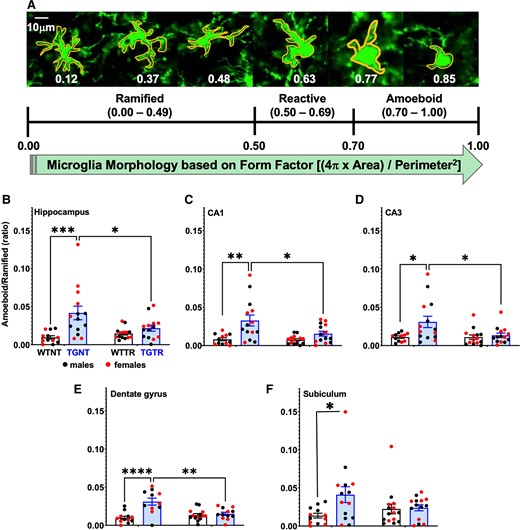
IBU treatment reduces hippocampal amoeboid/ramified microglia. (A) Microglia immunohistochemical analysis (Iba1 antibody) identified three different types of microglia morphology expressed by specific form factor ranges for circularity. Hippocampal amoeboid/ramified microglia ratio is significantly increased in TGNT compared to WTNT rats in the (B) hippocampus collapsed across all subregions (C) CA1, (D) CA3, (E) DG and (F) SB. IBU treatment prevented this rise in the hippocampus collapsed across all subregions and in each individual subregion. Ordinary two-way ANOVA with Sidak’s post hoc tests were used in B–F. n = −12 TGNT, n = 14 WTNT, n = 14 TGTR, n = 14 WTTR. *P < 0.05, **P < 0.01, ***P < 0.001.
Microglia analysis using Iba1 staining (Fig. 5A) showed that in the hippocampus collapsed across subregions, there is a significant reduction in amoeboid/ramified ratio following IBU-treatment [F(1,51) = 13.83, P < 0.01; Fig. 5B] post hoc differences between WTNT versus TGNT (t = 4.236, P < 0.01) and between TGNT and TGTR (t = 2.746, P = 0.0491). A similar pattern was observed in the hippocampal subregions: CA1 [F(1,51) = 4.512, P = 0.0386; Fig. 5C] post hoc differences between WTNT versus TGNT (t = 4.044, P < 0.01) and between TGNT and TGTR (t = 2.946, P = 0.0289); CA3 [F(1,51) = 6.298, P = 0.0154; Fig. 5D] post hoc differences between WTNT versus TGNT (t = 3.114, P < 0.0182) and between TGNT and TGTR (t = 2.863, P = 0.0362); DG [F(1,51) = 16.32, P < 0.01; Fig. 5E] post hoc differences between WTNT versus TGNT (t = 3.931, P < 0.01); and SB [F(1,51) = 4.167, P = 0.0464; Fig. 5F] post hoc differences between WTNT versus TGNT (t = 2.779, P = 0.0448).
Sex-dependent changes in TLR and ubiquitin-proteasome pathway gene expression in treated Tg-AD rats
Our RNAseq analysis reports output measures as reads per million, as well as FDR and P values (Supplementary Table 6) and the gene expression for TGTR is shown as percent relative to TGNT (Fig. 6).
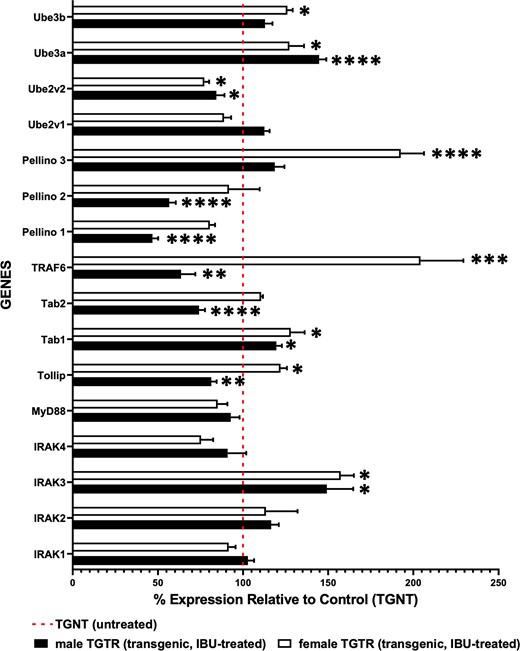
RNA sequencing data for TGTR and TGNT rats show differential and sex-dependent expression of genes involved in the TLR and UP pathways. These genes encode for IRAK-specific substrates, IRAK isoforms and ubiquitin ligases and conjugating enzymes. We analysed male and female hippocampal tissues separately for mRNA expression in TGTR relative to TGNT. The dotted red line represents 100% of the gene expression for male or female TGNT rats. Multiple unpaired one-tail t-tests with Welch’s corrections were used for analysis of RNA sequencing data for all genes listed in Fig. 6. (n = 5 TGNT males, n = 5 TGNT females, n = 5 TGTR males, n = 5 TGTR females). *P < 0.05, **P < 0.01, ***P < 0.001, ****P < 0.0001.
Of particular interest are the effects IBU has on the mRNA expression of genes involved in TLR signalling, since IBU is a TLR4 antagonist. Thus, we carried-out hippocampal RNAseq analysis of TGTR versus TGNT rats separately in males and females (n = 5 per condition). Genes of interest included members of the IRAK family, such as IRAK isoforms, downstream targets of IRAK activation, and specific ubiquitin ligases and substrates (Fig. 6 and Supplementary Table 6), which eventually converge on the NFκB transcription factor. Among the 16 genes of interest, five of them were unchanged in both sexes, including the adaptor MyD88, the ubiquitin conjugase Ube2v1 and kinases IRAK 1, 2 and 4. The remaining 11 genes were either up- or down-regulated across sexes, or differentially expressed between them.
Our findings show that IBU-treatment affects gene expression levels of the TLR and UP pathways differentially in male and female transgenic rats.
Sex-dependent changes in cAMP and interferon pathway gene expression in treated Tg-AD rats
We also investigated the effects of IBU on gene expression with respect to cAMP and interferon signalling pathways. The genes associated with the cAMP signalling and interferon pathways in males and females are given in Supplementary Tables 11 and 12, respectively. RNAseq analyses showed that cAMP signalling was a canonical pathway in both males and females with gene expression values that were modulated significantly by IBU (P values < 0.5 and FDR values < 0.05). Interestingly, IBU inhibition of phosphodiesterases (PDEs) leads to an upregulation of PDE 10A and cAMP-specific PDE 4b and 4d as well as adenylate cyclase 1 (Adcy1) and the cAMP dependent protein kinases Prkaca and Prkacb in females but not males. Similarly, the gene expression for metabotropic glutamate receptors 4 (Grm4) and 8 (Grm8), which are G protein-coupled receptors, are highly upregulated by IBU in females. Grm4 and Grm8 are linked to the inhibition of the cyclic AMP cascade.
Unlike the cAMP pathway, IBU had a modest effect on the expression of most genes associated with interferon signalling in both males and females. Only in males, IBU reduced the expression levels of the interferon lambda receptor 1 (Ifnlr1) but not significantly. Nevertheless, genes that encode interferon regulatory factors such as Ifrd1, Ifrd2, Irf2, Irf3, Irf4 and protein kinases Prkrir and Prkra in males were modulated significantly by IBU (P values < 0.05; FDR < 0.05). In females, the gene expression of interferon signalling genes that were significantly modulated by IBU (P values < 0.05, FDR < 0.05) included the regulatory factors Irf6 and Irf7 and a downregulation in interferon gamma receptor 1 (Ifngr1).
Discussion
Due to the multifactorial nature of Alzheimer’s disease, conventional one-drug-one-target approaches are unfruitful in discovering effective therapeutics. The low success rate of target-based screening has revived interest in phenotypic screening based on cell-lines or animal models, since it could identify drug leads in physiologically relevant conditions. However, phenotypic screening is relatively low-throughput, expensive, and difficult in the target deconvolution. Furthermore, drug responses in cell lines or animal models can be significantly different from that in a patient’s tissue. The lack of mechanistic understanding of drug actions and a knowledge gap between a model system and an individual patient makes it challenging to optimize drug lead compounds and to realize personalized medicine. To overcome these challenges, we developed a new multi-scale modelling approach that bridged target-based and phenotype-based drug repurposing. Our approach can not only use a patient’s tissue sample directly for phenotype-based compound screening, but also deconvolute genome-wide drug-target interactions. We successfully identified IBU as a promising polypharmacological agent for the treatment of Alzheimer’s disease, demonstrating the potential for integrating machine learning, biophysics and systems biology for the multi-scale modelling of drug actions. With the rapid advances in machine learning, especially deep learning techniques, we can further boost the performance of the multi-scale modelling and achieve personalized drug discovery.
We demonstrate for the first time in a transgenic rat model of Alzheimer’s disease (Tg-AD) that IBU-treatment mitigates cognitive deficits and hippocampal pathology associated with Alzheimer’s disease. This transgenic rat model37 is unique as it exhibits hippocampal-dependent spatial learning and memory deficits and hippocampal Alzheimer’s disease pathology, including plaques, tau PHFs, neuronal loss and microgliosis, in a progressive age-dependent manner that mimics the pathology observed in Alzheimer’s disease patients.
We chose to initiate IBU-treatment at an early age (five months) based on previous studies with multiple sclerosis patients showing that IBU was effective only when administered in the early stage of the disease.16 In our studies, the long-term daily IBU-treatment (six months) was well tolerated by the Tg-AD rats as we did not identify any adverse effects. The IBU-treatment significantly improved spatial learning and memory and reduced Alzheimer’s disease pathology, although some of the pathology was still detectable albeit at significantly lower levels. Overexpression of the human APPsw (2.6-fold) and PS1ΔE9 (6.2-fold) driving the robust pathology may account for the incomplete protection provided by IBU.
The preventive effects of IBU-treatment that we observed in the Tg-AD rats were most prominent in the DG, a regional effect of IBU that remains to be investigated. However, since the DG is known to be vulnerable to ageing and to be affected in the early stages of Alzheimer’s disease, it is possible that the DG is also the most responsive to treatment.41,42 Overall, our data clearly demonstrate the potential of IBU to lessen deficits in spatial learning and memory, to mitigate plaque and tau PHF pathology and to diminish gliosis in the hippocampus of the Tg-AD rats. IBU treatment did not remediate the decrease in differentiated neurons observed exclusively in the DG granular cell layer of the Tg-AD rats. Notably, the cognitive deficits of Tg-AD rats were significantly improved following IBU treatment. This significant improvement could be mediated by several compensatory mechanisms induced by IBU-treatment, such as (i) increased cerebral blood flow15,43; (ii) reduction in neuroinflammation44; (iii) promotion of neurotrophic factors45; (iv) enhanced synaptic plasticity46; or (v) other alternative mechanisms, all of which will be addressed in future studies. Our RNAseq analyses showed that IBU treatment: (i) reduces inflammation by decreasing the levels of the pro inflammatory cytokine IL6, interferon receptor 1, the macrophage migration inhibitory factor (MIF), microglial associated proteins such as transmembrane protein 119 (TMEM119), arachidonate 5-lipoxygenase activating protein (Alox5ap), toll like receptor 7 (TLR7), lysosomal protein transmembrane 5 (LAPTM5) and triggering receptor expressed on myeloid cells 2 (TREM2); (ii) increases expression of synaptic proteins such as synaptotagmin 2 (SYT2), which regulates vesicular transport, syntaxins (STX), which participates in neurotransmitter release, and cadherin (CDH1), which maintains synapse structure; and (iii) reduces the expression of the neurotrophic factor BDNF by 2-fold in both male and female treated rats. These findings suggest that IBU provides neuroprotection independent of growth factor upregulation. Furthermore, inhibition of TLR4 and the reduction in proinflammatory factors by IBU-treatment supports its benefits on cerebral blood flow. The RNAseq data were further evaluated by performing unbiased gene ontology enrichment analyses (Supplementary Fig. 5). In these analyses, changes in the transcript levels of TGTR male and female rats versus TGNT rats were organized and prioritized according to their function and relationships. The Ingenuity Pathway Analysis (IPA) summary lists the canonical pathways most affected by IBU-treatment in TGTR male and TGTR female rats (Supplementary Table 10).
A previous study in mice supported the preventive effects of 15 days of IBU-treatment (intraperitoneally) prior to receiving bilateral intracerebroventricular injections of Aβ1–42.47 IBU-treatment significantly ameliorated spatial learning and memory deficits and decreased hippocampal neuroinflammatory and apoptotic responses in the Aβ-injected mice. In a 1-methyl-4-phenyl-1,2,3,6-tetrahydropyridine (MPTP) mouse model of Parkinson’s disease, IBU was administered subcutaneously BID for 9 days, starting 2 days prior to MPTP intoxication.48 IBU diminished astroglial activation in the MPTP-treated mice but had no impact on striatal dopaminergic cell survival 7 days after acute MPTP intoxication. These two mouse studies evaluated the efficacy of a short-term IBU-treatment, in contrast to our studies that evaluated the effects of a long-term IBU-treatment using a transgenic rat model of Alzheimer’s disease that exhibits progressive age-dependent pathology. This is important because Alzheimer’s disease is a progressive neurodegenerative disorder with ageing being the greatest risk factor. Thus, it is critical to evaluate the long-term effects of potential therapeutics.
IBU was first described as a non-specific PDE inhibitor, but more recently was shown to also act as a TLR4 antagonist.8,9,11,12,48,49 IBU crosses the blood–brain barrier, has a half-life of about 19 h, improves blood flow to the brain and protects against neuroinflammation.43 The anti-inflammatory effects of IBU could be mediated by its PDE-inhibiting properties or by its TLR4 inactivation, and it is unclear which property potentiates the other, or if both work in synchrony. IBU-treatment exhibited immunomodulatory effects in multiple sclerosis and amyotrophic lateral sclerosis by shifting the pro-inflammatory phenotype of microglia to an anti-inflammatory phenotype.50,51 Based on these findings, we propose that the beneficial effects of IBU observed in our studies can be attributed, in part, to the anti-inflammatory effects of IBU.
The RNAseq analysis revealed that the TLR and ubiquitin-proteasome (UP) pathways are differentially altered by sex following IBU-treatment of the Tg-AD rats. We know from the literature that there are gender differences in TLR signalling with respect to pain, chronic fatigue, and immune response.52–54 However, we found no reports that TLR signalling is differentially affected with respect to gender in Alzheimer’s disease. To the best of our knowledge, the sex differences observed in our RNAseq data are novel findings that have not been reported. We will discuss two potential mechanisms that could mediate the anti-inflammatory action of IBU on the TLR pathway. For example, IBU-induced upregulation of IRAK3, a central negative regulator of TLR signalling that prevents IRAK1 from activating TRAF6 through phosphorylation, thus preventing NFκB activation. Furthermore, the TLR pathway is dependent on the function of the UP pathway at several steps, such as (i) degradation of IRAK1 after phosphorylation of TRAF6; (ii) degradation of TRAF6 after activation of the TAK/TAB complex; and (iii) degradation of IκB, which is required for NFκB nuclear translocation and activation. IBU-induced alterations on the expression of genes coding for components of the UPP, as shown in our data, indicate that this degradation pathway is recruited for modulation of inflammation via the TLR pathway.
Our study shows that six months of IBU treatment delivered orally, mitigates the progressive Alzheimer’s disease pathology observed in 11-month-old Tg-AD rats. However, there are certain limitations that warrant further investigation. For example, (i) our experimental design addresses how IBU treatment could be useful in the prevention of Alzheimer’s disease pathology. Future studies are needed to determine the effects of IBU on slowing or mitigating Alzheimer’s disease pathology in its advanced stages; (ii) our analysis focused on characterizing the learning and memory deficits in Tg-AD rats but did not fully characterized the impact that anxiety plays on spatial learning and memory; (iii) while our results demonstrate that inhibiting the targets of IBU is sufficient to reverse several Alzheimer’s phenotypes in transgenic rats, additional experiments are needed to determine whether it is necessary to inhibit all the targets simultaneously, or whether the same effect can be achieved with a smaller subset.
In Japan and other Asian countries, IBU is approved for treating asthma and stroke.49 Based on our results, the outcome and details of the IBU-induced alterations in the TLR and UP pathways will be addressed in future studies. However, their significance is crucial as IBU is a TLR4 antagonist. Interestingly, TLR4-mediated induction of apoptosis is observed mostly in aging and not young neurons.55 TLR4 mediated signalling, via still unclear mechanisms, seems to contribute to the pathology of age-related neurodegenerative diseases, including Alzheimer’s disease.55 Using TLR4 antagonists, such as IBU, could offer an efficient means to prevent the damaging events associated with neuroinflammation in Alzheimer’s disease. Overall, our results support that IBU can be repurposed as a potential therapeutic to treat memory deficits and pathology in Alzheimer’s disease.
Acknowledgements
We thank the late Dr Peter Davies (Albert Einstein University, New York, NY, USA) for generously providing the PHF1 antibody.
Funding
This work was supported in part by NIGMS R01GM122845 of NIH and NIA R01AG057555 of NIH to L.X., NIGMS Training Grant RISE 5R25GM060665 to G.O. and O.C. and the City University of New York (Ph.D. program in Biochemistry, Graduate Center).
Competing interests
The authors report no competing interests.
Supplementary material
Supplementary material is available at Brain online.
References
Author notes
Lei Xie, Patricia Rockwell, Maria E Figueiredo-Pereira and Peter A Serrano contributed equally to this work.