-
PDF
- Split View
-
Views
-
Cite
Cite
Brittany L Mitchell, Santiago Diaz-Torres, Svetlana Bivol, Gabriel Cuellar-Partida, International Headache Genetics Consortium , Zachary F Gerring, Nicholas G Martin, Sarah E Medland, Katrina L Grasby, Dale R Nyholt, Miguel E Rentería, Elucidating the relationship between migraine risk and brain structure using genetic data, Brain, Volume 145, Issue 9, September 2022, Pages 3214–3224, https://doi.org/10.1093/brain/awac105
- Share Icon Share
Abstract
Migraine is a highly common and debilitating disorder that often affects individuals in their most productive years of life. Previous studies have identified both genetic variants and brain morphometry differences associated with migraine risk. However, the relationship between migraine and brain morphometry has not been examined on a genetic level, and the causal nature of the association between brain structure and migraine risk has not been determined.
Using the largest available genome-wide association studies to date, we examined the genome-wide genetic overlap between migraine and intracranial volume, as well as the regional volumes of nine subcortical brain structures. We further focused the identification and biological annotation of genetic overlap between migraine and each brain structure on specific regions of the genome shared between migraine and brain structure. Finally, we examined whether the size of any of the examined brain regions causally increased migraine risk using a Mendelian randomization approach.
We observed a significant genome-wide negative genetic correlation between migraine risk and intracranial volume (rG = −0.11, P = 1 × 10−3) but not with any subcortical region. However, we identified jointly associated regional genomic overlap between migraine and every brain structure. Gene enrichment in these shared genomic regions pointed to possible links with neuronal signalling and vascular regulation. Finally, we provide evidence of a possible causal relationship between smaller total brain, hippocampal and ventral diencephalon volume and increased migraine risk, as well as a causal relationship between increased risk of migraine and a larger volume of the amygdala.
We leveraged the power of large genome-wide association studies to show evidence of shared genetic pathways that jointly influence migraine risk and several brain structures, suggesting that altered brain morphometry in individuals with high migraine risk may be genetically mediated. Further interrogation of these results showed support for the neurovascular hypothesis of migraine aetiology and shed light on potentially viable therapeutic targets.
Introduction
Migraine is a common and disabling neurological disorder characterized by severe and prolonged headache attacks, sensory sensitivity and brain dysfunction.1 It is estimated to affect approximately 25% of the global population and imposes a substantial personal and socioeconomic burden.2,3 Migraine is diagnosed based on clinical criteria and defined subtypes exist, the most common of which are migraines with and without aura—defined based on the presence of visual or spatial symptoms during the migraine attack (for a review of current diagnostic criteria, see Ashina et al.4). Notably, migraine attacks are twice as common in women than men,5,6 with substantial inter-individual variability in motor, sensory, autonomic, cognitive and emotional symptoms. Currently, the biological mechanisms underpinning migraine’s heterogeneity are poorly understood and need further investigation.
Around 40–60% of the variation in individual risk for migraine is heritable, highlighting the contribution of genes in migraine aetiology.7–9 Several advances have been made in understanding the genetic risk factors of migraine, especially in rarer migraine subtypes such as familial hemiplegic migraine, where candidate biomarker genes have been well established.10–12 However, the identification of genetic variants associated with common migraine subtypes has been hampered by the complex nature of the disorder. The 2016 genome-wide association study (GWAS) meta-analysis of 59 674 participants with migraine and 316 078 controls identified 44 independent single nucleotide polymorphisms (SNPs) in 38 loci that support the involvement of ion homeostasis, oxidative stress and vascular dysfunction in migraine aetiology.13
Brain imaging is a useful tool for identifying structural brain changes in individuals who suffer episodic or chronic migraine attacks. Several studies have examined the relationship between migraine and the grey matter volume of subcortical brain structures. Associations between migraine risk and decreased volume of almost all subcortical regions have been reported.14–19 However, to our knowledge, no study has simultaneously examined these relationships across all subcortical brain regions. Additionally, the specific intracellular mechanisms leading to changes in brain morphology are ill-defined; alterations in the density of neuronal dendrites or glial cells have been proposed as potential contributors to volumetric changes in grey matter.20 Altered brain morphology might explain cognitive, behavioural and sensory changes observed in people who experience migraine, but it is unknown whether these changes are a cause or consequence of migraine attacks. Differences in brain morphometry have also been linked to several risk factors for migraine, such as sex20,21 and age.22 Therefore, further exploration is needed to clarify the direction of these associations.
It is known that individual variation in both brain morphometry and migraine risk is influenced by genetic factors13,23,24 and that both genetics and brain imaging have been discussed as potential biomarkers for migraine.25 Despite phenotypic associations between brain volume and migraine risk, no study has yet explored whether these are mediated by genetic pathways. Therefore, exploring the genetic relationship between migraine and brain structure provides an opportunity for unique insight into migraine pathophysiology that may be used to identify future migraine-specific biomarkers that could separate migraine from other headache disorders or aid in the prediction of treatment response. In this study, we explored the genetic relationships between migraine risk and intracranial volume (ICV) and the regional volumes of nine subcortical structures and performed a causal inference analysis.
Materials and methods
Migraine GWAS data
We used the summary statistics from a GWAS meta-analysis of 59 674 participants with migraine and 316 078 controls13 that controlled for biological sex, age and genetic ancestry. This study identified 44 independent SNPs (38 loci) associated with migraine and a SNP-based heritability of 14.6%. The GWAS summary statistics included data from both the International Headache Genetics Consortium and 23andMe Inc. and were obtained via direct application and material transfer agreement approval. Notably, all cohorts included in the meta-analysis adjusted the GWAS results for sex.
Brain morphology GWAS data
We examined the relationship between 10 brain neuroimaging measures and migraine—this included ICV and nine subcortical regions of interest: brainstem, caudate nucleus, putamen, hippocampus, globus pallidus, thalamus, ventral diencephalon (hypothalamic region), nucleus accumbens and amygdala. To ensure we used the most powered GWAS data for each trait, summary statistics from GWAS meta-analyses of ICV and all subcortical regions of interest (n > 66 000) were used in this study. Briefly, these results were generated through the meta-analysis of GWAS results in over 66 000 participants from international datasets (the ENIGMA26 and CHARGE27 consortia, the UK Biobank28,29 and the ABCD cohort30,31) using Multi-Trait Analysis of GWAS software. Each cohort used MRI scan data obtained using various scanners and acquisition protocols, which were controlled for in the GWAS analyses. Phenotypes were defined as the mean volume of the left and right hemispheres (in cm3), with the exception of the brainstem, where the total volume was used. For more information on phenotype definitions and data acquisition for each cohort, see the corresponding reference. The ENIGMA-CHARGE summary statistics24 were obtained through direct application to the consortia. The UK Biobank and ABCD GWASs were conducted ‘in-house’ before meta-analysis. Importantly, individual subcortical region GWAS analyses controlled for the effects of total ICV, as well as sex, age (and their interaction effects) and genetic ancestry components. Table 1 summarizes the number of genome-wide significant SNPs and corresponding SNP-based heritability for ICV and each subcortical region of interest.
Summary of meta-analysis (n > 66 000) depicting number of independent significant loci and SNP-based heritability estimates per region of interest
Phenotype . | Number of independent genome-wide significant loci . | SNP-based heritability (SE) . | LDSC intercept with migraine (SE) . |
---|---|---|---|
ICV | 83 | 0.28 (0.02) | −0.003 (0.006) |
Brainstem | 80 | 0.22 (0.02) | 0.008 (0.006) |
Caudate nucleus | 67 | 0.19 (0.01) | 0.005 (0.007) |
Putamen | 67 | 0.19 (0.01) | −0.009 (0.007) |
Hippocampus | 49 | 0.22 (0.02) | −0.006 (0.006) |
Globus pallidus | 33 | 0.13 (0.01) | 0.001 (0.006) |
Thalamus | 29 | 0.14 (0.01) | 0.008 (0.006) |
Ventral diencephalon | 33 | 0.28 (0.02) | 0.006 (0.006) |
Nucleus accumbens | 32 | 0.14 (0.01) | 0.011 (0.006) |
Amygdala | 19 | 0.10 (0.01) | 0.001 (0.006) |
Phenotype . | Number of independent genome-wide significant loci . | SNP-based heritability (SE) . | LDSC intercept with migraine (SE) . |
---|---|---|---|
ICV | 83 | 0.28 (0.02) | −0.003 (0.006) |
Brainstem | 80 | 0.22 (0.02) | 0.008 (0.006) |
Caudate nucleus | 67 | 0.19 (0.01) | 0.005 (0.007) |
Putamen | 67 | 0.19 (0.01) | −0.009 (0.007) |
Hippocampus | 49 | 0.22 (0.02) | −0.006 (0.006) |
Globus pallidus | 33 | 0.13 (0.01) | 0.001 (0.006) |
Thalamus | 29 | 0.14 (0.01) | 0.008 (0.006) |
Ventral diencephalon | 33 | 0.28 (0.02) | 0.006 (0.006) |
Nucleus accumbens | 32 | 0.14 (0.01) | 0.011 (0.006) |
Amygdala | 19 | 0.10 (0.01) | 0.001 (0.006) |
LDSC = linkage disequilibrium score regression.
Summary of meta-analysis (n > 66 000) depicting number of independent significant loci and SNP-based heritability estimates per region of interest
Phenotype . | Number of independent genome-wide significant loci . | SNP-based heritability (SE) . | LDSC intercept with migraine (SE) . |
---|---|---|---|
ICV | 83 | 0.28 (0.02) | −0.003 (0.006) |
Brainstem | 80 | 0.22 (0.02) | 0.008 (0.006) |
Caudate nucleus | 67 | 0.19 (0.01) | 0.005 (0.007) |
Putamen | 67 | 0.19 (0.01) | −0.009 (0.007) |
Hippocampus | 49 | 0.22 (0.02) | −0.006 (0.006) |
Globus pallidus | 33 | 0.13 (0.01) | 0.001 (0.006) |
Thalamus | 29 | 0.14 (0.01) | 0.008 (0.006) |
Ventral diencephalon | 33 | 0.28 (0.02) | 0.006 (0.006) |
Nucleus accumbens | 32 | 0.14 (0.01) | 0.011 (0.006) |
Amygdala | 19 | 0.10 (0.01) | 0.001 (0.006) |
Phenotype . | Number of independent genome-wide significant loci . | SNP-based heritability (SE) . | LDSC intercept with migraine (SE) . |
---|---|---|---|
ICV | 83 | 0.28 (0.02) | −0.003 (0.006) |
Brainstem | 80 | 0.22 (0.02) | 0.008 (0.006) |
Caudate nucleus | 67 | 0.19 (0.01) | 0.005 (0.007) |
Putamen | 67 | 0.19 (0.01) | −0.009 (0.007) |
Hippocampus | 49 | 0.22 (0.02) | −0.006 (0.006) |
Globus pallidus | 33 | 0.13 (0.01) | 0.001 (0.006) |
Thalamus | 29 | 0.14 (0.01) | 0.008 (0.006) |
Ventral diencephalon | 33 | 0.28 (0.02) | 0.006 (0.006) |
Nucleus accumbens | 32 | 0.14 (0.01) | 0.011 (0.006) |
Amygdala | 19 | 0.10 (0.01) | 0.001 (0.006) |
LDSC = linkage disequilibrium score regression.
Genetic correlations
The extent of sample overlap and genetic correlations between migraine and the brain regions of interest were assessed using bivariate linkage disequilibrium score regression.32 Significance levels were corrected for multiple testing using Bonferroni correction [0.05/10 (number of neuroimaging measures) = 0.005].
Pairwise GWAS
The pairwise GWAS method was used to further investigate the genetic overlap between migraine and all 10 brain regions of interest by identifying specific regions of the genome that are unique to each trait and those that are shared.33
Pairwise GWAS operates by first dividing the genome into 1703 approximately independent regions. For each region, pairwise GWAS was used to calculate the posterior probability of four different models: the region was unique to migraine, unique to the region of interest, shared by both traits through the same causal variants and associated with both traits but via separate causal variants. The model with the highest posterior probability (given the posterior probability is >0.5) was selected. To account for potential confounding due to sample overlap between traits, the software required the correlation between effect sizes in non-associated regions of the genome. To do this, fGWAS, a command-line tool implemented in pairwise GWAS was used to calculate the posterior probability of association for each region separately for both traits. Regions with a posterior probability of association <0.2 in both traits were selected, and the correlation in SNP effect sizes between the two traits was determined.
Functional annotation
SNPs in shared genomic regions identified from the pairwise-GWAS analysis were uploaded to the FUMA platform v1.3.6 for putative functional annotation. SNPs in these regions were mapped to protein-coding genes using gene-based and gene-set tests in MAGMA v1.08. A Bonferroni corrected significance threshold was applied (0.05/total number of genes in shared regions). Genes within these regions were used to conduct gene-set enrichment analyses against gene-sets in the Gene Ontology database and NHGRI-EBI GWAS Catalogue.
Mendelian randomization
Using Mendelian randomization (MR), we inferred the causal relationship between migraine and all examined volumes. MR uses SNPs that have been associated with a risk factor (i.e. ICV) as instrumental variables (IVs) to identify causal associations between the risk factor and an outcome (i.e. migraine) of interest. The process of random assortment of SNPs during meiosis effectively divides a population into effect and control groups for the risk factor based on each individual’s genetic profiles, akin to a randomized control trial. Comparing the size of the effect of the IVs on the risk factor against their effect on the outcome can provide evidence that the IVs are acting on the outcome via the risk factor (vertical pleiotropy), provided key assumptions are met.34 Unlike observational studies, causality can be inferred from MR analysis as the direction of effect is clear, from IV to risk factor, not the other way around.
As a sensitivity analysis, we performed MR analyses using alternative MR methods implemented in the ‘TwoSampleMR’ package in MR-Base.36 In order to select the IVs, genome-wide significant SNPs were clumped in Plink using the following parameters: --clump kb 1000 kb, --clump r2 <0.001. MR PRESSO was used to detect outliers and remove IVs that violated the exclusion restriction assumption (associated with both the instrument and the outcome).37 SNPs that were considered potential pleiotropic outliers were removed as well as any SNPs that were directly associated with migraine. We further tested the robustness of our results using four different MR methods, including inverse variance weighted, which combines a Wald-type estimator for each IV in a multiplicative random-effects model, weighted median and penalized weighted median, which adjusts for as many as half of the IVs having a pleiotropic effect, and weighted mode, which is the mode of the Wald-type estimates used to calculate the inverse variance weight with and without a standard error-based weighting to each IV.38 To further control for potential pleiotropy between our traits, we excluded all SNPs that lie in the shared genomic regions identified by pairwise GWAS from our IVs.
Lastly, we tested the joint causal relationship between migraine and subcortical brain regions that showed evidence of a causal effect in a multivariable model that accounted for the effect of ICV. We used MR-Base to perform multivariate MR, a method that uses IVs with multiple and potentially related exposures to estimate the effect of the exposure on the outcome, thereby controlling for the effect of the other exposures in the model.39
Data availability
The data that support the findings of this study are available from the corresponding authors upon reasonable request. The code used to conduct these analyses will be made public on acceptance of the article. The full results of all analyses are presented in the Supplementary Tables.
The full GWAS summary statistics for the 23andMe migraine discovery dataset will be made available through 23andMe to qualified researchers under an agreement with 23andMe that protects the privacy of the 23andMe participants. Visit https://research.23andme.com/collaborate/#dataset-access/for more information and to apply to access the data.
Results
Sample overlap and genetic correlations
The linkage disequilibrium score intercept between migraine and each brain region was not significantly different from zero—indicating that these traits are independent and that extent of sample overlap is negligible. We identified a significant negative genetic correlation between migraine risk and ICV (rG = −0.11, P = 0.001), suggesting that smaller brain volume is genetically correlated with increased risk of migraine. Notably, despite some previously reported phenotypic associations between subcortical brain structures and migraine risk, genetic correlations did not reach statistical significance for any subcortical structure (Fig. 1).
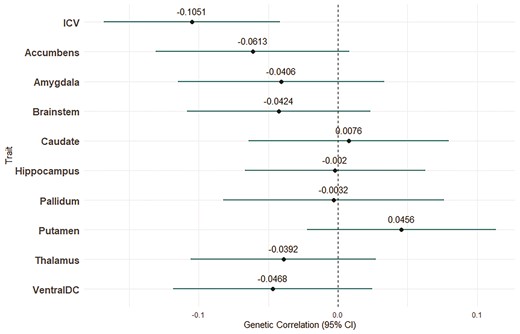
Genetic correlation estimates between migraine and subcortical regions and ICVs. Bars indicate 95% CIs.
Pairwise GWAS
We further investigated the relationship between migraine risk and ICV, as well as all nine subcortical regions, using pairwise GWAS. Using a posterior probability of association greater than 0.5 as a cut-off, we identified six regions of the genome that were shared between ICV and migraine through the same causal variants (Fig. 2). Interestingly, despite non-significant global genetic correlations, we identified specific shared genomic regions between all subcortical regions of interest and migraine, with the exception of the ventral diencephalon, which had no shared regions with a posterior probability of association >0.5. The number of shared genomic regions varied substantially between regions of interest; the caudate had the most shared regions with migraine (11) and both the putamen and pallidum had only one (Fig. 2 and Supplementary Tables 1–10). In several instances, multiple brain regions had the same genomic region shared with migraine, for example, the genomic region at 6: 148920352 was significantly shared between migraine and the amygdala, hippocampus and the thalamus. An ideogram depicting shared genomic regions between migraine and all examined brain regions was created using http://visualization.ritchielab.org/phenograms/plot.
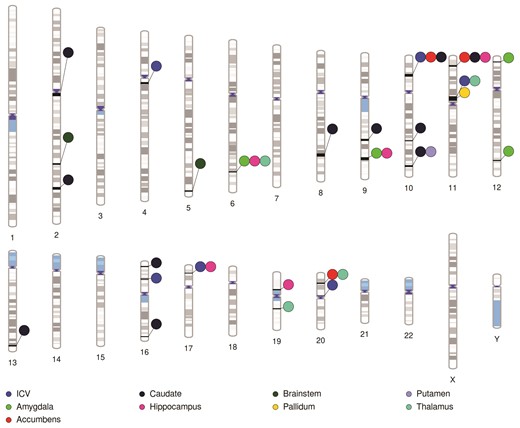
Ideogram highlighting regions of the genome identified by pairwise-GWAS as likely to be causally shared between migraine and all examined brain volumes.
Functional annotation
For each region of interest, we mapped SNPs in the identified genomic regions that are shared through the same causal variants to protein-coding genes using MAGMA. All brain regions of interest and migraine had significantly enriched genes for at least one of the shared genomic regions after Bonferroni correction for multiple testing. Here, we focused on genes that showed significant enrichment in both traits as they provide the strongest candidates for shared biological pathways. Overall, 47 genes were significantly enriched in migraine as well as at least one brain region.
Twelve genes showed significant enrichment in both ICV and migraine. The majority of these genes mapped to the shared region on chromosome 11 (Supplementary Table 11). The caudate and the thalamus had the most shared genes with migraine among the subcortical region of interest (nine and 10 genes, respectively). Results from the gene-based tests for each brain region and migraine can be found in Supplementary Tables 11–19. Note that as the ventral diencephalon had no shared genomic regions with migraine with a PPA>0.5, it was not included in the gene-based tests.
Gene-set enrichment analysis of the 47 genes enriched in both migraine and at least one brain region of interest was conducted, and six gene-sets were significantly enriched for Gene Ontology biological processes. These gene-sets primarily implicate biological processes involved in lipid metabolism and signalling, purinergic signalling and receptor clustering (Supplementary Table 20). These gene-sets are shared with those from several disorders reported in the GWAS catalogue, including immune response, neurodevelopmental disorders such as autism and schizophrenia, coronary artery disease, blood coagulation and Alzheimer’s disease (Supplementary Table 21). Providing validation to these results is the overlap between our enriched gene-sets and those reported in previous GWASs of migraine, headache and hippocampal volume.
Mendelian randomization
We observed a causal relationship between smaller ICV and migraine risk using GSMR [odds ratio (OR) 0.91 (0.85–0.97), P = 0.006; Fig. 3 and Table 2]. Sensitivity analyses were conducted in MR-BASE, but not all MR estimates reached statistical significance [ORIVW 0.95 (0.87–1.03), P = 0.16]. Nevertheless, all MR methods showed a negative effect of larger ICV on migraine risk (Fig. 3). There was no evidence of reverse causality through association via bidirectional MR analysis, indicating that genetic predisposition for migraine does not influence ICV, with the estimated OR from GSMR being 0.95 (95% CI 0.89–1.02, P = 0.16).
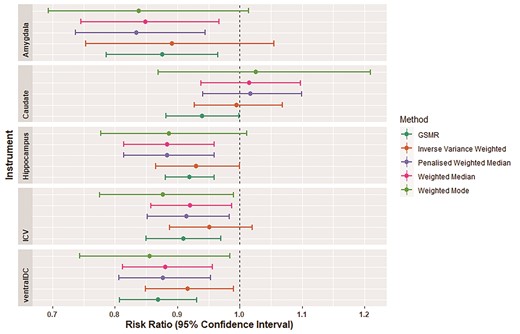
MR results for the three brain structures with evidence to be causally associated with increased migraine risk. Bars indicate 95% CIs.
Summary of GSMR results between migraine and nine subcortical regions of interest
Instrument trait . | Outcome trait . | Number of IVs . | Odds ratio . | P-value . |
---|---|---|---|---|
ICV | Migraine | 41 | 0.91 | 6.0 × 10−3* |
Migraine | ICV | 21 | 0.97 | 0.23 |
Brainstem | Migraine | 43 | 0.98 | 0.60 |
Migraine | Brainstem | 21 | 0.96 | 0.14 |
Caudate nucleus | Migraine | 36 | 1.06 | 0.04* |
Migraine | Caudate nucleus | 21 | 0.94 | 0.10 |
Putamen | Migraine | 39 | 0.94 | 0.08 |
Migraine | Putamen | 21 | 1.03 | 0.14 |
Hippocampus | Migraine | 35 | 0.92 | 0.01* |
Migraine | Hippocampus | 20 | 0.96 | 0.11 |
Pallidum | Migraine | 23 | 1.01 | 0.69 |
Migraine | Pallidum | 21 | 1.01 | 0.62 |
Thalamus | Migraine | 16 | 0.99 | 0.96 |
Migraine | Thalamus | 22 | 0.97 | 0.20 |
Ventral diencephalon | Migraine | 23 | 0.86 | 3.60 × 10−6* |
Migraine | Ventral diencephalon | 22 | 0.96 | 0.26 |
Nucleus accumbens | Migraine | 20 | 1.02 | 0.50 |
Migraine | Nucleus accumbens | 22 | 0.95 | 0.06 |
Amygdala | Migraine | 15 | 0.87 | 0.003* |
Migraine | Amygdala | 21 | 0.88 | 4.81 × 10−7* |
Instrument trait . | Outcome trait . | Number of IVs . | Odds ratio . | P-value . |
---|---|---|---|---|
ICV | Migraine | 41 | 0.91 | 6.0 × 10−3* |
Migraine | ICV | 21 | 0.97 | 0.23 |
Brainstem | Migraine | 43 | 0.98 | 0.60 |
Migraine | Brainstem | 21 | 0.96 | 0.14 |
Caudate nucleus | Migraine | 36 | 1.06 | 0.04* |
Migraine | Caudate nucleus | 21 | 0.94 | 0.10 |
Putamen | Migraine | 39 | 0.94 | 0.08 |
Migraine | Putamen | 21 | 1.03 | 0.14 |
Hippocampus | Migraine | 35 | 0.92 | 0.01* |
Migraine | Hippocampus | 20 | 0.96 | 0.11 |
Pallidum | Migraine | 23 | 1.01 | 0.69 |
Migraine | Pallidum | 21 | 1.01 | 0.62 |
Thalamus | Migraine | 16 | 0.99 | 0.96 |
Migraine | Thalamus | 22 | 0.97 | 0.20 |
Ventral diencephalon | Migraine | 23 | 0.86 | 3.60 × 10−6* |
Migraine | Ventral diencephalon | 22 | 0.96 | 0.26 |
Nucleus accumbens | Migraine | 20 | 1.02 | 0.50 |
Migraine | Nucleus accumbens | 22 | 0.95 | 0.06 |
Amygdala | Migraine | 15 | 0.87 | 0.003* |
Migraine | Amygdala | 21 | 0.88 | 4.81 × 10−7* |
Significant associations P < 0.05.
Summary of GSMR results between migraine and nine subcortical regions of interest
Instrument trait . | Outcome trait . | Number of IVs . | Odds ratio . | P-value . |
---|---|---|---|---|
ICV | Migraine | 41 | 0.91 | 6.0 × 10−3* |
Migraine | ICV | 21 | 0.97 | 0.23 |
Brainstem | Migraine | 43 | 0.98 | 0.60 |
Migraine | Brainstem | 21 | 0.96 | 0.14 |
Caudate nucleus | Migraine | 36 | 1.06 | 0.04* |
Migraine | Caudate nucleus | 21 | 0.94 | 0.10 |
Putamen | Migraine | 39 | 0.94 | 0.08 |
Migraine | Putamen | 21 | 1.03 | 0.14 |
Hippocampus | Migraine | 35 | 0.92 | 0.01* |
Migraine | Hippocampus | 20 | 0.96 | 0.11 |
Pallidum | Migraine | 23 | 1.01 | 0.69 |
Migraine | Pallidum | 21 | 1.01 | 0.62 |
Thalamus | Migraine | 16 | 0.99 | 0.96 |
Migraine | Thalamus | 22 | 0.97 | 0.20 |
Ventral diencephalon | Migraine | 23 | 0.86 | 3.60 × 10−6* |
Migraine | Ventral diencephalon | 22 | 0.96 | 0.26 |
Nucleus accumbens | Migraine | 20 | 1.02 | 0.50 |
Migraine | Nucleus accumbens | 22 | 0.95 | 0.06 |
Amygdala | Migraine | 15 | 0.87 | 0.003* |
Migraine | Amygdala | 21 | 0.88 | 4.81 × 10−7* |
Instrument trait . | Outcome trait . | Number of IVs . | Odds ratio . | P-value . |
---|---|---|---|---|
ICV | Migraine | 41 | 0.91 | 6.0 × 10−3* |
Migraine | ICV | 21 | 0.97 | 0.23 |
Brainstem | Migraine | 43 | 0.98 | 0.60 |
Migraine | Brainstem | 21 | 0.96 | 0.14 |
Caudate nucleus | Migraine | 36 | 1.06 | 0.04* |
Migraine | Caudate nucleus | 21 | 0.94 | 0.10 |
Putamen | Migraine | 39 | 0.94 | 0.08 |
Migraine | Putamen | 21 | 1.03 | 0.14 |
Hippocampus | Migraine | 35 | 0.92 | 0.01* |
Migraine | Hippocampus | 20 | 0.96 | 0.11 |
Pallidum | Migraine | 23 | 1.01 | 0.69 |
Migraine | Pallidum | 21 | 1.01 | 0.62 |
Thalamus | Migraine | 16 | 0.99 | 0.96 |
Migraine | Thalamus | 22 | 0.97 | 0.20 |
Ventral diencephalon | Migraine | 23 | 0.86 | 3.60 × 10−6* |
Migraine | Ventral diencephalon | 22 | 0.96 | 0.26 |
Nucleus accumbens | Migraine | 20 | 1.02 | 0.50 |
Migraine | Nucleus accumbens | 22 | 0.95 | 0.06 |
Amygdala | Migraine | 15 | 0.87 | 0.003* |
Migraine | Amygdala | 21 | 0.88 | 4.81 × 10−7* |
Significant associations P < 0.05.
We further tested the causal relationship between each of the nine subcortical brain regions and migraine. A significant causal association was observed between smaller hippocampal, caudate nucleus, amygdala and ventral diencephalon volumes and migraine risk (Fig. 3 and Table 2). To corroborate these results, we conducted sensitivity analyses in MR-BASE. For the hippocampus and amygdala, the majority of sensitivity analyses reached statistical significance, and all were in a consistent direction to that estimated using GSMR. None of the sensitivity analyses reached statistical significance for the caudate nucleus. However, the effect of the ventral diencephalon remained significant across all analyses after multiple testing correction (Fig. 3). None of the other examined regions of interest showed a statistically significant causal relationship with migraine (Table 2). However, when examining the causal effect of migraine on the volume of each brain region, we found a strong and significant causal effect for migraine risk causing smaller amygdala volume [GSMR OR = 0.88 (0.75–0.97) P = 4.8 × 10−07]. Migraine risk was not significantly causal for any other subcortical region of interest.
We then tested the causal relationship between the region of interest with evidence of a causal association with migraine risk when simultaneously accounting for the effect of ICV in a multivariable MR model. Results showed that when including ICV in the same model, the causal relationship between smaller hippocampal and ventral diencephalon volume and increased migraine risk remained significant, indicating that these associations are not driven by the effect of ICV or collider bias [OR = 0.90 (0.83–0.99), P = 0.03 and OR = 0.88 (0.73–0.94), P = 0.006, respectively].
Discussion
In this study, we set out to examine the genetic relationship between migraine risk and brain structure. We found evidence of a significant negative genetic correlation between migraine risk and ICV on the genome-wide level. In contrast, we did not find evidence supporting a genome-wide genetic relationship between migraine and any of the nine subcortical regions of interest examined. It is important to note that the GWAS results of the nine subcortical regions that were used in this study were conducted while controlling for total ICV, therefore highlighting associated variants as independent of overall brain size. To better understand these associations on a phenotypic level, it is important that future imaging studies account for the overall effect of brain volume when investigating the specific region of interest (e.g. via brain-volume normalized volumes of subcortical structures).
We further delineated the shared genetic architecture between migraine and ICV by identifying six specific shared regions of the genome. Gene-based tests identified 12 genes in these regions that were significantly enriched in both migraine and ICV. One of the genes, ATG13, has been implicated in autophagy and immune response and has previously been associated with headaches.40 Other genes, including DGKZ have been associated with skeletal muscle growth and regulation, whereas MDK and REST have been implicated in neuroregulation, circulatory system development and the modulation of neuroinflammation.
Despite the lack of global genetic association, almost every subcortical region of interest had at least one genomic region that was significantly shared with migraine risk. Gene-based tests conducted on these genomic regions revealed a host of enriched genes that may underlie the associations between migraine and the morphology of various brain structures. One of the most common subcortical brain regions epidemiologically associated with migraine is the brainstem. Several studies have reported volumetric changes in the brainstem in migraine patients, as well as a negative correlation between brainstem volume and hypersensitivities and pain cascades experienced in migraine.16–18 On the other hand, a functional association between the brainstem and migraine risk has also been proposed, implicating potential neuronal activation or connectivity mechanisms.41–43 The lack of genetic correlation between migraine and the brainstem suggested that common genetic effects across the genome do not explain this observed association. However, we did find evidence of two regions of the genome that were significantly shared between migraine and the brainstem. Significantly enriched genes that are shared between these traits provide possible mechanisms underlying their association. These include homeobox (HOX) genes (EVX2, HOXD1, HOXD3), which are involved in the regulation of embryonic neural development. HOX genes are central to events involved in specifying the hindbrain territory (which includes the brainstem)44 and are involved in neuronal specification and connectivity. Differential expression of HOX genes in neuronal types after development has been linked to synapse formation and plasticity45 and synaptic plasticity is well-recognized in some forms of chronic pain.46,47 Therefore, HOX gene expression may provide an interesting exploratory drug target for some forms of pain, including migraine.
Another subcortical region often associated with migraine risk is the hippocampus. Despite the lack of global genetic correlation, we identified five shared genomic regions and provided some evidence of a causal association between decreased hippocampal volume and migraine risk. Hippocampal volume has been negatively correlated with the total number and frequency of migraine attacks,48 and a longitudinal study reported decreased hippocampal volumes in individuals diagnosed with episodic migraine.49 We found five genes significantly enriched in both migraine and the hippocampus in the shared genomic regions. One particularly interesting finding is the enrichment of ASTN2 which has repeatedly been associated with migraine13,50,51 as well as hippocampal volume.52ASTN2 functions in neuronal migration, and its overexpression increases synaptic activity.53 It has also been linked to neurodevelopmental disorders such as attention-deficit hyperactivity disorder and schizophrenia54,55 and the earlier age of onset of Alzheimer’s disease.56 Therefore, genetic variation at the ASTN2 locus could contribute to differences in brain morphometry, especially that of the hippocampus, as well as have a role in migraine pathophysiology.
Pairwise GWAS results showed several regions of the genome that were shared between migraine and more than one brain region. These included three genomic regions that were each shared between migraine and three different regions of interest and five genomic regions that were shared between migraine and two different regions of interest. Gene enrichment in these regions predominantly implicates genes involved in vascular and haemodynamic regulation, as well as pain phenotypes; these include MRVI1, CHRM4, ARMS2, MLLT10 and JAG1. Genetic variation in MRVI1 has been linked to migraine with aura and without aura.57MRVI1 and CHRM4 function in the parasympathetic nervous system and are involved in smooth muscle contractility and vascular regulation. MRVI1 has been associated with blood pressure and arterial stiffness,58 platelet aggregation59 and moyamoya, a rare condition where the blood vessels that supply blood to the brain become narrow.60 The function of ARMS2 is still poorly understood; however, it has been associated with age-related macular degeneration (a vascular disease)61 as well as migraine.13 Furthermore, MLLT10 plays a crucial role in haematopoiesis and has been linked to multisite chronic pain62 and brain tumours.63 Lastly, JAG1 encodes proteins that play a role throughout life in the development of new blood cells and genetic variation is associated with pulmonary arterial hypertension.64
The co-enrichment of vascular and pain phenotypes is perhaps unsurprising given that haemodynamic changes have been strongly implicated in migraine pathophysiology.13,65 Gene-set enrichment analysis showed enriched gene-sets involved in lipid kinase activity and purinergic signalling, both of which have been implicated in neuronal signalling as well as pain signalling and reception. Functionally, this may occur through many molecular mechanisms. For instance, many nociceptive (pain-producing) receptors are present in the brain that, when activated, use lipid kinases to signal for the reduction of ion channels, causing an overall increase in neuronal excitability and haemodynamic changes, leading to pain and stimuli sensitization.66,67 Additionally, purinergic signalling has also been shown to be involved in visceral, neuropathic and inflammatory pain,68 primarily through the conversion of purines to ATP. ATP is involved in vasoconstriction and vasodilation, cortical spreading depression (a wave of neuronal depolarization followed by suppression of brain activity, involving dramatic changes in neural and vascular function), and the excitation of glial cells, all of which are associated with pain. Lastly, the action of purines in so many cell types has been shown to contribute to the initiation and amplification of migraine pain (for a review, see Cieślak et al.69).
The enrichment of these gene-sets has also been reported in several published GWASs in the GWAS catalogue. These include vascular-related phenotypes, such as coronary artery disease and blood coagulation, and neurodevelopmental and neurodegenerative disorders. Together with our gene-based test results, the enrichment of these genes-sets points to specific molecular pathways involved in both vascular and neuronal dysfunction that may be common among these disorders as well as brain morphology and are, at least to some extent, genetically mediated. Interestingly, only hippocampal volume had overlapping gene-sets with our results, perhaps indicating that this brain region specifically is involved in the pathophysiology of migraine. This was supported by evidence of a causal relationship between smaller hippocampal volume and increased risk of migraine, a result that remained significant even after controlling for the causal effect of total ICV.
We also observed a significant causal effect between the smaller ventral diencephalon and amygdala volumes and increased migraine risk. Similar to the brainstem, the ventral diencephalon, which encompasses the hypothalamic region, functions in pain perception through the modulation of the trigeminovascular system, and it has been hypothesized that dysfunction of these brain regions contributes to the cascade of events that result in migraine symptoms.70,71 Although our MR analysis identified potential causal relationships between both ICV and various subcortical structure volumes and migraine risk, this method does not identify what drives this causal association. Our findings, coupled with the previous evidence already discussed, suggest that this association may be driven by cerebral blood flow. This is additionally supported by a recent study that found associations between genetic variants for migraine and cerebral blood flow72 and the fact that smaller brain volume has been associated with increased cerebral blood flow.73 Another possible explanation is the mediating effect of sex. Migraine is far more common in females than males, but women also tend to have smaller brain volumes.21 Therefore, these associations may reflect the observed sex differences between both migraine risk and brain morphometry.
Our finding of a significant bi-directional causal effect for migraine risk causing smaller amygdala volume, but also—to a lesser extent—smaller amygdala volumes increasing migraine risk, is of interest as it suggests a genetic vulnerability to migraine influences amygdala morphology. Animal models indicate that acute and chronic stress induces long-term functional and morphological alterations in the amygdala and hippocampus.74 Hence, the neurological stress induced by migraine, or a premorbid vulnerability to migraine, may have toxic and lasting effects on amygdala morphology, which in turn may increase the sensitivity of the migraine brain to stress—a known risk factor for migraine.25 On the other hand, the amygdala is part of the limbic system and several studies have reported an association between amygdala activation and connectivity with increased migraine risk.75,76 It is possible that chronic migraine attacks alter the morphology of the amygdala, which in turn alters the level of activation of the amygdala, thereby influencing nociceptive processing. Although beyond the scope of the current study, it will be interesting to examine the relationship between migraine and amygdala volume with respect to migraine chronicity and age of onset.
This study has some limitations. First, the GWAS results used in this study were performed using cohorts of European ancestry. Thus, caution should be taken when comparing or generalizing our results with other ancestry groups. These GWASs were also conducted on both men and women, and sex differences are known to exist in both brain morphometry and migraine risk. This reduces our power to detect any sex-specific associations between brain morphometry and migraine. MR requires a strict set of assumptions to be met, which is often difficult when examining polygenic traits. Although we have attempted to exclude any SNPs that are known to be pleiotropic, we cannot guarantee that this assumption has not been violated.
Additionally, the delineation of the brain regions used in this study was dependent on available neuroimaging GWAS data and may lack resolution that would be obtained when using vertex-wide or genetically informative parcellations. However, well-powered GWAS data of this type is not yet available. Lastly, many of the analyses conducted in this study are dependent on the power of the original GWAS. Therefore, a null finding in our study does not necessarily reflect a true lack of association. Nonetheless, and importantly, our genetics-based approach is immune to potential influence from non-genetic (i.e. environmental) confounding factors, and our genetic overlap and causal inference analyses allow us to assess and produce results that are robust to potential confounding due to genetic confounding (e.g. pleiotropy). This work complements and extends the findings from previous observational neuroimaging studies on two main fronts. First, the results from pairwise-GWAS identified specific regions of the genome that are shared between migraine and brain structure; this enables the enhanced resolution and dissection of the specific biological pathways that may underlie these associations which have previously not been explored. Secondly, the use of these genetic approaches allows the identification and characterization of associations between migraine risk and brain structure (and their causal nature) that are robust to confounding effects of disease, medication, environmental effects, and reverse causation.
To our knowledge, this is the first study to examine the genetic relationship between migraine, ICV and subcortical structure and provide evidence of the direction of causation. We leveraged the power of large GWAS to show evidence of shared genetic pathways involved in neuronal signalling and vascular regulation, as well as a potential causal relationship between decreased ICV and hippocampal volume and increased migraine risk. Although we are still a long way from understanding the neurobiology of migraine, further interrogation of these pathways and brain structures may produce a better understanding of the underlying mechanisms, improve our ability to prevent or treat attacks and shed light on potentially viable therapeutic targets.
Acknowledgements
We would like to thank the research participants and employees of 23andMe for making this work possible.
Funding
This work was conducted using published GWAS summary statistics and therefore no funding sources directly contributed to this work.
Competing interests
G.C.P. contributed to this study while employed at The University of Queensland. He is now an employee of 23andMe, Inc. and he may hold stock or stock options. All other authors report no comepting interests.
Supplementary material
Supplementary material is available at Brain online.
Appendix 1
The International Headache Genetics Consortium
Full details are provided in the Supplementary material.
Padhraig Gormley, Verneri Anttila, Bendik S. Winsvold, Priit Palta, Tonu Esko, Tune H. Pers, Kai-How Farh, Ester Cuenca-Leon, Mikko Muona, Nicholas A. Furlotte, Tobias Kurth, Andres Ingason, George McMahon, Lannie Ligthart, Gisela M. Terwindt, Mikko Kallela, Tobias M. Freilinger, Caroline Ran, Scott G. Gordon, Anine H. Stam, Stacy Steinberg, Guntram Borck, Markku Koiranen, Lydia Quaye, Hieab H. H. Adams, Terho Lehtimäki, Antti-Pekka Sarin, Juho Wedenoja, David A. Hinds, Julie E. Buring, Markus Schürks, Paul M. Ridker, Maria Gudlaug Hrafnsdottir, Hreinn Stefansson, Susan M. Ring, Jouke-Jan Hottenga, Brenda W. J. H. Penninx, Markus Färkkilä, Ville Artto, Mari Kaunisto, Salli Vepsäläinen, Rainer Malik, Andrew C. Heath, Pamela A. F. Madden, Nicholas G. Martin, Grant W. Montgomery, Mitja Kurki, Mart Kals, Reedik Mägi, Kalle Pärn, Eija Hämäläinen, Hailiang Huang, Andrea E. Byrnes, Lude Franke, Jie Huang, Evie Stergiakouli, Phil H. Lee, Cynthia Sandor, Caleb Webber, Zameel Cader, Bertram Muller-Myhsok, Stefan Schreiber, Thomas Meitinger, Johan G. Eriksson, Veikko Salomaa, Kauko Heikkilä, Elizabeth Loehrer, Andre G. Uitterlinden, Albert Hofman, Cornelia M. van Duijn, Lynn Cherkas, Linda M. Pedersen, Audun Stubhaug, Christopher S. Nielsen, Minna Männikkö, Evelin Mihailov, Lili Milani, Hartmut Göbel, Ann-Louise Esserlind, Anne Francke Christensen, Thomas Folkmann Hansen, Thomas Werge, Sigrid Børte, Bru Cormand, Else Eising, Lyn Griffiths, Eija Hamalainen, Marjo Hiekkala, Risto Kajanne, Lenore Launer, Terho Lehtimaki, Davor Leslsel, Alfons Macaya, Massimo Mangino, Nancy Pedersen, Danielle Posthuma, Patricia Pozo-Rosich, Alice Pressman, Celia Sintas, Marta Vila-Pueyo, Huiying Zhao. Jaakko Kaprio, Arpo J. Aromaa, Olli Raitakari, M. Arfan Ikram, Tim Spector, Marjo-Riitta Järvelin, Andres Metspalu, Christian Kubisch, David P. Strachan, Michel D. Ferrari, Andrea C. Belin, Martin Dichgans, Maija Wessman, Arn M. J. M. van den Maagdenberg, John-Anker Zwart, Dorret I. Boomsma, George Davey Smith, Kari Stefansson, Nicholas Eriksson, Mark J. Daly, Benjamin M. Neale, Jes Olesen, Daniel I. Chasman, Dale R. Nyholt and Aarno Palotie.
References
Abbreviations
- GSMR =
generalized summary-data-based Mendelian randomization
- GWAS =
genome-wide association study
- ICV =
intracranial volume
- IV =
instrumental variable
- MR =
Mendelian randomization
- SNP =
single nucleotide polymorphism
Author notes
Dale R Nyholt and Miguel E Rentería contributed equally to this work.