-
PDF
- Split View
-
Views
-
Cite
Cite
Chunyu Wang, Jiaming Shen, Christiana Charalambous, Jianxin Pan, Modeling biomarker variability in joint analysis of longitudinal and time-to-event data, Biostatistics, Volume 25, Issue 2, April 2024, Pages 577–596, https://doi.org/10.1093/biostatistics/kxad009
- Share Icon Share
Summary
The role of visit-to-visit variability of a biomarker in predicting related disease has been recognized in medical science. Existing measures of biological variability are criticized for being entangled with random variability resulted from measurement error or being unreliable due to limited measurements per individual. In this article, we propose a new measure to quantify the biological variability of a biomarker by evaluating the fluctuation of each individual-specific trajectory behind longitudinal measurements. Given a mixed-effects model for longitudinal data with the mean function over time specified by cubic splines, our proposed variability measure can be mathematically expressed as a quadratic form of random effects. A Cox model is assumed for time-to-event data by incorporating the defined variability as well as the current level of the underlying longitudinal trajectory as covariates, which, together with the longitudinal model, constitutes the joint modeling framework in this article. Asymptotic properties of maximum likelihood estimators are established for the present joint model. Estimation is implemented via an Expectation-Maximization (EM) algorithm with fully exponential Laplace approximation used in E-step to reduce the computation burden due to the increase of the random effects dimension. Simulation studies are conducted to reveal the advantage of the proposed method over the two-stage method, as well as a simpler joint modeling approach which does not take into account biomarker variability. Finally, we apply our model to investigate the effect of systolic blood pressure variability on cardiovascular events in the Medical Research Council elderly trial, which is also the motivating example for this article.
1 Introduction
Our research is motivated by the Medical Research Council (MRC) elderly trial in the United Kingdom which compared diuretic and β-blocker regimens versus placebo, in hypertensive patients aged 65–74 years. The clinical interest lies in the effects of active treatments as well as the impact of systolic blood pressure (SBP) profile on cardiovascular risk. Although it is widely believed in medicine that the underlying usual SBP is of great importance in predicting cardiovascular disease, more and more research has recently provided insight into the role of SBP variability. Instead of dismissing SBP variability as a random fluctuation, Howard and Rothwell (2009) showed that visit-to-visit variability of SBP is reproducible within individuals over time. That is, individuals with high (low) visit-to-visit variability at one time are more likely to possess high (low) variability at another time. This non-random nature of SBP variability is crucial as it warranted the exploration on the prognostic value of visit-to-visit variability. A pioneering piece of research on this topic was conducted by Rothwell and others (2010 b), in which the prognostic significance of visit-to-visit SBP variability was reliably established in predicting stroke and other vascular events. Their conclusion that SBP variability was a strong predictor, even stronger than mean SBP for the cohorts they studied, challenged the widespread belief (the usual blood-pressure hypothesis; Rothwell, 2010) in medicine and justified the incorporation of visit-to-visit SBP variability when analyzing cardiovascular disease. However, existing measures of SBP variability in clinical research (Yano, 2017), e.g., standard deviation (SD), coefficient of variation, and successive variation, are calculated from observed SBP measurements on a person-by-person basis, which are susceptible to the number of measurements involved in calculations. In fact, these sample-based measures tend to provide unreliable estimates of variability if they are calculated from a small number of measurements (Howard and Rothwell, 2009). Further, the association between visit-to-visit variability, when quantified by above measures, and the risk of disease might be underestimated due to regression dilution (Clarke and others, 1999).
To deal with issues involved in naive variability measures, a mixed-effects location scale model was proposed (Lyles and others, 1999), where a random within-individual variance was introduced to characterize the individual-specific variability of the longitudinal biomarker. Meanwhile, the error-free variability was incorporated as a predictor in a survival submodel to assess its effect on the event of interest. Unlike the case in sample-based variability measures, this modeling framework (i) uses the true within-individual variance rather than its estimated version as a predictor to avoid the regression dilution issue; (ii) allows borrowing strength across individuals by assuming that these within-individual variances come from a common population (Barrett and others, 2019; Gao and others, 2011). However, this modeling approach cannot distinguish the biological (systematic) variability with which we are concerned, from the measurement variability (fluctuation due to measurement errors) since the within-individual variance is a combination of both kinds of variability. If a complicated mean function is specified to capture the biomarker trend, the within-individual variance will be dominated by measurement variability. Therefore, the degree to which random within-individual variance reflects real biological variability is uncertain.
In this article, we propose a measure to characterize the inherent biological variability of a biomarker whose underlying trajectory possesses smooth and nonlinear shape. The idea comes from smoothing splines on quantifying the roughness of a curve. Specifically, we use the integrated squared second derivative, , to capture the cumulative variability of a biomarker trajectory for the i-th individual from t0 to current time t. The square root of the integral rather than the integral itself is used as a covariate in the survival submodel to ensure that the scale of our defined variability measure is consistent with that of . Figure 1 shows the fitted SBP trajectories (left, divided by 30) for six randomly selected patients in the MRC trial and their corresponding square root of cumulative variability (right). It is obvious that individuals with stable SBP level, e.g., individual 129 and 2295, have lower variability quantified by our proposed measure and that individuals with sharp fluctuation in SBP trajectory, e.g., individual 3800 and 1043, possess larger variability. From this perspective, it is reasonable to adopt this measure to characterize the biological variability behind the longitudinal measurements for each individual.
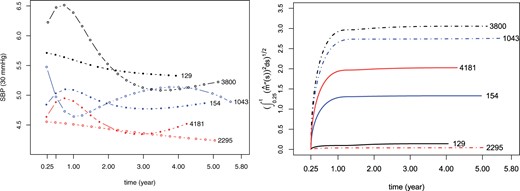
Fitted SBP trajectories (left) and the corresponding square root of cumulative variability (right). Numbers beside curves indicate patient IDs in the MRC trial. Solid circles (solid lines in the right) represent uncensored cases, while hollow circles (dashed lines in the right) represent censored cases.
Together with a mixed-effects model for longitudinal measurements, a survival hazard model which incorporates the proposed variability quantity as a predictor, constitutes the joint modeling framework in this article. Random effects are employed in both the mixed-effects model and the survival model to account for the dependence between the two types of outcomes. Based on the standard joint model which associates longitudinal and event outcomes through the current true level of longitudinal biomarker (see Tsiatis and Davidian, 2004; Tsiatis and others, 1995), several extensions have been proposed to characterize possibly more complex associations. Ye and others (2008) included the current slope of the biomarker trajectory when modeling survival hazard. Sylvestre and Abrahamowicz (2009) incorporated the integral of the longitudinal trajectory, representing the cumulative effects of longitudinal history. All of these extended associations share a common parameterization that they can be simplified to linear functions of random effects. Clearly, the association structure in our joint model is complicated by the inclusion of the proposed variability quantity and falls outside the linear forms. For estimation, the likelihood method is the most widely used approach in joint modeling literature (Hsieh and others, 2006). Allowing the baseline function to be totally unspecified, Zeng and Cai (2005) established the asymptotic properties for the semiparametric maximum likelihood estimator (MLE) in which the association structures were constrained to be linear forms of random effects. We here extend their theoretical result to the joint model with our derived association structure.
The remainder of this article is organized as follows. We illustrate the motivation and rationale of quantifying the biomarker variability by our proposed measure in Section 2. In Section sec:mod, we describe the joint modeling framework with longitudinal and survival submodels specified in detail. Section 4 gives the asymptotic properties of maximum likelihood estimates (MLEs) in the proposed joint model. Section 5 focuses on the technical issues in estimation when the expectation-maximization (EM) algorithm is applied, especially on the approximation method in the E-step. In Section 6, we compare the proposed joint modeling method with other existing approaches via simulation studies. An application of our methodology to analyze the MRC trial is presented in Section 7, followed by discussion in Section 8.
2 Motivation
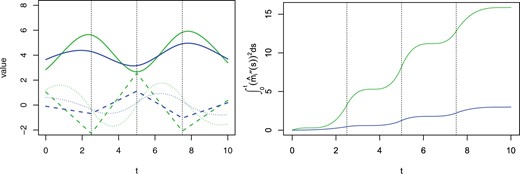
Left: simulated trajectories for i = 1, 2 (solid curves) and their first (dotted curves) and second (dashed lines) derivative functions. Right: values of over time for each trajectory. The dashed vertical lines in both subfigures denote the positions of interior knots.
3 Model
Let and Ci denote the event and censoring times, respectively, for the ith individual, . We can only observe and in practice, where is the indicator function. The longitudinal measurements for the ith individual are denoted by , which are intermittently collected with measurement errors at time points . Moreover, we denote the hypothetical trajectory behind Yi by , which, in this article, is assumed to be a smooth function of t. It is also assumed that Ci is independent of given covariates.
3.1 Longitudinal submodel
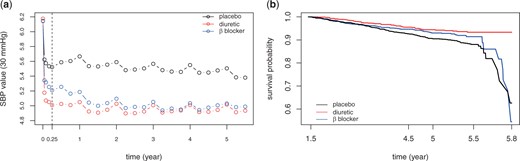
Preliminary analysis of SBP observations and cardiovascular events. (a) mean level of SBP observations (divided by 30) by randomized trials. (b) Kaplan-Meier survival curves of cardiovascular events by randomized trials.
Note that (3.3) is a sieve approximation (Rice and Wu, 2001) to the unknown function of time. The performance of (3.3) depends on the choice for the number of knots and their locations. A pragmatic approach is to place interior knots at locations where there is high curvature in longitudinal responses and where more observations are collected, and then use AIC or BIC (calculated on longitudinal data only) to determine the number of knots (Rizopoulos and others, 2009; Shi and others, 1996).
3.2 Survival submodel
4 Inference on semiparametric model
The maximum likelihood estimate is defined as , where consists of all right-continuous step functions only with positive jumps at . Let denote the true parameter for and be the true cumulative baseline function. Under assumptions given in Section SA of the supplementary material available at Biostatistics online, we establish the consistency and asymptotic normality of . Moreover, by showing the influence function (each coordinate) of falls into a linear span of score functions, is proved to be semiparametrically efficient.
Let be the metric space of all bounded functions in and let d denote the dimension of . The next theorem states the asymptotic distribution of .
Under conditions specified in Section SA of the supplementary material available at Biostatistics online, converges in distribution to a Gaussian random element in . Further, weakly converges to a multivariate normal distribution with mean zero and variance equal to the semiparametric efficiency bound.
Proofs of the above theorems are similar in spirit to Zeng and Cai (2005) and can be found in Section SA of the supplementary material available at Biostatistics online. As the analytical form for the asymptotic covariance matrix is not available (Hsieh and others, 2006), we employ a boostrap method to numerically estimate the standard errors of . Basically, we generate J bootstrap samples (each of size m) by sampling with replacement from the original observed data and estimate based on each bootstrap sample. The SD of J bootstrap estimates of is then an estimate of the standard error of .
The asymptotic theorems developed in this section aim to extend the asymptotic properties of the semiparametric MLE in standard join models (with a linear association structure in terms of random effects) to our proposed joint models with a complex association structure in (3.6). However, we should note that (3.3) is an approximation (a regression spline estimator) to an unknown smooth trajectory and the consequence of this approximation behavior has not been taken into account in Theorems 4.1 and 4.2. Consistency of the regression spline estimator definitely requires the dimension of spline bases q increasing to infinity at an appropriate rate of m (q should be written as qm). See Agarwal and Studden (1980) for the order of qm under which the regression spline estimator and its derivatives achieve the optimal global convergence rate. Similar to regression dilution with error-prone covariates, it is expected that the survival parameters, especially α1 and α2, will be estimated with bias. We illustrate this phenomenon in Section 6.3 where the true biomarker trajectory is set to be a smooth function but not exactly a spline function given by (3.3).
5 Estimation with EM algorithm
5.1 M-step
5.2 E-step
As indicated in (5.2), only the mode of , namely , and second derivatives of and around are required to achieve a second order approximation of . Given that in the present model ’s cannot be obtained analytically, Newton–Raphson method is employed to find the value numerically. See Section B of the supplementary material available at Biostatistics online for specific calculations.
5.3 Impact on asymptotic properties
Note that the asymptotic properties, including consistency of and semiparametric efficiency of , established in Section 4, may be affected due to approximation method used in the E-step. Based on investigations in Vonesh (1996) and Rizopoulos and others (2009), the resulting convergence rate of when a fully exponential Laplace approximation is applied becomes where comes from the approximation error in the E-step. Therefore, will be consistent only as both m and go to infinity, and the consistency rate will still be if grows at a rate greater than . Moreover, if grows faster than , the asymptotic behavior remains the same as shown in Theorem 4.2. This can be illustrated from the difference between , the fully exponential Laplace based Q function, and , the true Q function. Since , the resulting estimate, when is negligible, will act as if it is calculated from the true Q function or exact likelihood function. Semiparametric efficiency of still holds in this case.
6 Simulation studies
6.1 Case 1: General simulation study
We specify a longitudinal submodel with three evenly spaced interior knots (q = 7) within the follow-up time interval and observation times . Regression coefficients are set as . The variance (covariance matrix) of measurement error and random effects are specified as and , respectively. The baseline covariates xi and outside stimulus zi are not incorporated in the longitudinal model for simplicity. For the survival submodel, we consider a binary covariate w taking values 0 or 1, each with probability 1/2 and its effect is assumed to be . The baseline hazard function is specified as if t > 5 and 0 otherwise. Censoring times are independently generated from , leading to a 50% censoring rate. Truncated by event times and censoring times, the number of longitudinal observations ni varies between 11 and 21. The simulation setting described above aims to define a simple case to numerically examine the performance of estimation methods. 500 Monte Carlo (MC) replications are conducted and the sample size is fixed as m = 1000.
Table 1 shows the performance of the proposed joint modeling (JM) method against the two-stage (TS) method (Wu and others, 2012). Both methods are evaluated in terms of bias, Monte Carlo SD, bootstrap SD and 95% coverage probability (CP). The estimates of survival parameters, namely, , and α2, show larger biases under TS than those obtained from the JM method. In particular, the effects of the longitudinal level and variability on survival hazard are underestimated when the TS method is employed. CPs for the TS estimates of γ and α2 suffer from severe under-coverage due to large biases. Both methods provide good performance in estimating parameters related to the longitudinal submodel. Moreover, s are quite close to SDs, which implies that bootstrap SD performs well in estimating the standard error of obtained from both JM and TS methods and hence can be used in the real data analysis. For survival parameters, the standard errors of the JM estimates are slightly larger than those of the TS estimates. This is mainly due to the fact that the randomness of bi in the survival submodel is removed when the TS method is used. Additionally, the standard errors of the ’s and ’s increase with index j since the latter elements in β and D correspond to the basis functions with support over later time intervals in which longitudinal observations are sparse.
Simulation results of the JM and TS methods in case 1. Average is the average value of parameter estimates in 500 MC replications; SD is the MC standard deviation of the estimates across simulated data sets; is the mean of bootstrap standard deviations calculated on bootstrap samples for each MC sample; CP is the 95% coverage probability defined as the percentage of repetitions in which true value falls into the calculated confidence interval.
. | JM . | TS . | ||||||
---|---|---|---|---|---|---|---|---|
True . | . | SD . | . | CP . | . | SD . | . | CP . |
Survival | ||||||||
–1.9786 | 0.1011 | 0.0990 | 0.946 | –1.8218 | 0.0878 | 0.0898 | 0.458 | |
0.1976 | 0.0310 | 0.0303 | 0.940 | 0.1667 | 0.0270 | 0.0273 | 0.760 | |
0.2917 | 0.0301 | 0.0290 | 0.940 | 0.2389 | 0.0267 | 0.0256 | 0.364 | |
Longitudinal | ||||||||
5.9584 | 0.0674 | 0.0663 | 0.912 | 5.9966 | 0.0670 | 0.0663 | 0.932 | |
3.0902 | 0.0797 | 0.0794 | 0.910 | 3.0124 | 0.0788 | 0.0793 | 0.938 | |
6.9584 | 0.0803 | 0.0826 | 0.920 | 6.9771 | 0.0806 | 0.0824 | 0.942 | |
0.9824 | 0.0875 | 0.0863 | 0.948 | 1.0385 | 0.0873 | 0.0862 | 0.938 | |
7.9500 | 0.1140 | 0.1220 | 0.930 | 7.8822 | 0.1129 | 0.1205 | 0.820 | |
4.9798 | 0.1717 | 0.1678 | 0.952 | 4.8884 | 0.1713 | 0.1670 | 0.900 | |
4.0011 | 0.2238 | 0.2285 | 0.944 | 4.0036 | 0.2197 | 0.2259 | 0.942 | |
3.0139 | 0.1819 | 0.1868 | 0.950 | 2.9978 | 0.1830 | 0.1868 | 0.950 | |
4.0455 | 0.2518 | 0.2569 | 0.942 | 3.9903 | 0.2513 | 0.2553 | 0.948 | |
3.9946 | 0.2418 | 0.2517 | 0.948 | 3.9933 | 0.2433 | 0.2537 | 0.944 | |
4.9966 | 0.2720 | 0.2785 | 0.946 | 4.9919 | 0.2723 | 0.2782 | 0.948 | |
3.9482 | 0.3825 | 0.3703 | 0.940 | 3.9326 | 0.3830 | 0.3691 | 0.944 | |
2.9812 | 0.5057 | 0.5220 | 0.942 | 2.9705 | 0.5023 | 0.6196 | 0.946 | |
3.8998 | 0.7397 | 0.7366 | 0.942 | 3.8914 | 0.7338 | 0.7324 | 0.946 | |
0.9983 | 0.0141 | 0.0142 | 0.940 | 0.9998 | 0.0142 | 0.0143 | 0.942 |
. | JM . | TS . | ||||||
---|---|---|---|---|---|---|---|---|
True . | . | SD . | . | CP . | . | SD . | . | CP . |
Survival | ||||||||
–1.9786 | 0.1011 | 0.0990 | 0.946 | –1.8218 | 0.0878 | 0.0898 | 0.458 | |
0.1976 | 0.0310 | 0.0303 | 0.940 | 0.1667 | 0.0270 | 0.0273 | 0.760 | |
0.2917 | 0.0301 | 0.0290 | 0.940 | 0.2389 | 0.0267 | 0.0256 | 0.364 | |
Longitudinal | ||||||||
5.9584 | 0.0674 | 0.0663 | 0.912 | 5.9966 | 0.0670 | 0.0663 | 0.932 | |
3.0902 | 0.0797 | 0.0794 | 0.910 | 3.0124 | 0.0788 | 0.0793 | 0.938 | |
6.9584 | 0.0803 | 0.0826 | 0.920 | 6.9771 | 0.0806 | 0.0824 | 0.942 | |
0.9824 | 0.0875 | 0.0863 | 0.948 | 1.0385 | 0.0873 | 0.0862 | 0.938 | |
7.9500 | 0.1140 | 0.1220 | 0.930 | 7.8822 | 0.1129 | 0.1205 | 0.820 | |
4.9798 | 0.1717 | 0.1678 | 0.952 | 4.8884 | 0.1713 | 0.1670 | 0.900 | |
4.0011 | 0.2238 | 0.2285 | 0.944 | 4.0036 | 0.2197 | 0.2259 | 0.942 | |
3.0139 | 0.1819 | 0.1868 | 0.950 | 2.9978 | 0.1830 | 0.1868 | 0.950 | |
4.0455 | 0.2518 | 0.2569 | 0.942 | 3.9903 | 0.2513 | 0.2553 | 0.948 | |
3.9946 | 0.2418 | 0.2517 | 0.948 | 3.9933 | 0.2433 | 0.2537 | 0.944 | |
4.9966 | 0.2720 | 0.2785 | 0.946 | 4.9919 | 0.2723 | 0.2782 | 0.948 | |
3.9482 | 0.3825 | 0.3703 | 0.940 | 3.9326 | 0.3830 | 0.3691 | 0.944 | |
2.9812 | 0.5057 | 0.5220 | 0.942 | 2.9705 | 0.5023 | 0.6196 | 0.946 | |
3.8998 | 0.7397 | 0.7366 | 0.942 | 3.8914 | 0.7338 | 0.7324 | 0.946 | |
0.9983 | 0.0141 | 0.0142 | 0.940 | 0.9998 | 0.0142 | 0.0143 | 0.942 |
Simulation results of the JM and TS methods in case 1. Average is the average value of parameter estimates in 500 MC replications; SD is the MC standard deviation of the estimates across simulated data sets; is the mean of bootstrap standard deviations calculated on bootstrap samples for each MC sample; CP is the 95% coverage probability defined as the percentage of repetitions in which true value falls into the calculated confidence interval.
. | JM . | TS . | ||||||
---|---|---|---|---|---|---|---|---|
True . | . | SD . | . | CP . | . | SD . | . | CP . |
Survival | ||||||||
–1.9786 | 0.1011 | 0.0990 | 0.946 | –1.8218 | 0.0878 | 0.0898 | 0.458 | |
0.1976 | 0.0310 | 0.0303 | 0.940 | 0.1667 | 0.0270 | 0.0273 | 0.760 | |
0.2917 | 0.0301 | 0.0290 | 0.940 | 0.2389 | 0.0267 | 0.0256 | 0.364 | |
Longitudinal | ||||||||
5.9584 | 0.0674 | 0.0663 | 0.912 | 5.9966 | 0.0670 | 0.0663 | 0.932 | |
3.0902 | 0.0797 | 0.0794 | 0.910 | 3.0124 | 0.0788 | 0.0793 | 0.938 | |
6.9584 | 0.0803 | 0.0826 | 0.920 | 6.9771 | 0.0806 | 0.0824 | 0.942 | |
0.9824 | 0.0875 | 0.0863 | 0.948 | 1.0385 | 0.0873 | 0.0862 | 0.938 | |
7.9500 | 0.1140 | 0.1220 | 0.930 | 7.8822 | 0.1129 | 0.1205 | 0.820 | |
4.9798 | 0.1717 | 0.1678 | 0.952 | 4.8884 | 0.1713 | 0.1670 | 0.900 | |
4.0011 | 0.2238 | 0.2285 | 0.944 | 4.0036 | 0.2197 | 0.2259 | 0.942 | |
3.0139 | 0.1819 | 0.1868 | 0.950 | 2.9978 | 0.1830 | 0.1868 | 0.950 | |
4.0455 | 0.2518 | 0.2569 | 0.942 | 3.9903 | 0.2513 | 0.2553 | 0.948 | |
3.9946 | 0.2418 | 0.2517 | 0.948 | 3.9933 | 0.2433 | 0.2537 | 0.944 | |
4.9966 | 0.2720 | 0.2785 | 0.946 | 4.9919 | 0.2723 | 0.2782 | 0.948 | |
3.9482 | 0.3825 | 0.3703 | 0.940 | 3.9326 | 0.3830 | 0.3691 | 0.944 | |
2.9812 | 0.5057 | 0.5220 | 0.942 | 2.9705 | 0.5023 | 0.6196 | 0.946 | |
3.8998 | 0.7397 | 0.7366 | 0.942 | 3.8914 | 0.7338 | 0.7324 | 0.946 | |
0.9983 | 0.0141 | 0.0142 | 0.940 | 0.9998 | 0.0142 | 0.0143 | 0.942 |
. | JM . | TS . | ||||||
---|---|---|---|---|---|---|---|---|
True . | . | SD . | . | CP . | . | SD . | . | CP . |
Survival | ||||||||
–1.9786 | 0.1011 | 0.0990 | 0.946 | –1.8218 | 0.0878 | 0.0898 | 0.458 | |
0.1976 | 0.0310 | 0.0303 | 0.940 | 0.1667 | 0.0270 | 0.0273 | 0.760 | |
0.2917 | 0.0301 | 0.0290 | 0.940 | 0.2389 | 0.0267 | 0.0256 | 0.364 | |
Longitudinal | ||||||||
5.9584 | 0.0674 | 0.0663 | 0.912 | 5.9966 | 0.0670 | 0.0663 | 0.932 | |
3.0902 | 0.0797 | 0.0794 | 0.910 | 3.0124 | 0.0788 | 0.0793 | 0.938 | |
6.9584 | 0.0803 | 0.0826 | 0.920 | 6.9771 | 0.0806 | 0.0824 | 0.942 | |
0.9824 | 0.0875 | 0.0863 | 0.948 | 1.0385 | 0.0873 | 0.0862 | 0.938 | |
7.9500 | 0.1140 | 0.1220 | 0.930 | 7.8822 | 0.1129 | 0.1205 | 0.820 | |
4.9798 | 0.1717 | 0.1678 | 0.952 | 4.8884 | 0.1713 | 0.1670 | 0.900 | |
4.0011 | 0.2238 | 0.2285 | 0.944 | 4.0036 | 0.2197 | 0.2259 | 0.942 | |
3.0139 | 0.1819 | 0.1868 | 0.950 | 2.9978 | 0.1830 | 0.1868 | 0.950 | |
4.0455 | 0.2518 | 0.2569 | 0.942 | 3.9903 | 0.2513 | 0.2553 | 0.948 | |
3.9946 | 0.2418 | 0.2517 | 0.948 | 3.9933 | 0.2433 | 0.2537 | 0.944 | |
4.9966 | 0.2720 | 0.2785 | 0.946 | 4.9919 | 0.2723 | 0.2782 | 0.948 | |
3.9482 | 0.3825 | 0.3703 | 0.940 | 3.9326 | 0.3830 | 0.3691 | 0.944 | |
2.9812 | 0.5057 | 0.5220 | 0.942 | 2.9705 | 0.5023 | 0.6196 | 0.946 | |
3.8998 | 0.7397 | 0.7366 | 0.942 | 3.8914 | 0.7338 | 0.7324 | 0.946 | |
0.9983 | 0.0141 | 0.0142 | 0.940 | 0.9998 | 0.0142 | 0.0143 | 0.942 |
6.2 Case 2: Simulation study based on the MRC trial
A second simulation is conducted to investigate the performance of the proposed method in analyzing the MRC trial by generating data similar to the real data. To this end, parameters are set around their estimated values. Covariates and observation schedules for longitudinal and survival outcomes are designed to mimic the real data (see Section SC.1 of the supplementary material available at Biostatistics online for details). Truncated by event times and censoring times, ni varies between 10 and 26 and thus with m = 3700. The approximation error in the E-step is expected to have little effect on the JM estimation. Before showing the final estimation result, we first illustrate the validity of the knots selection criteria mentioned in Section 3.1. By calculating AIC and BIC based on longitudinal data only, the true model can be effectively selected as indicated in Table 2.
Number . | Location . | AIC . | BIC . |
---|---|---|---|
q = 5 | 0.25 | 97 065 | 97 285 |
1 | 101 295 | 101 515 | |
1.5 | 102 593 | 102 813 | |
q = 6 | (0.25, 0.5) | 96 273 | 96 529 |
(0.5, 1) | 96 034 | 96 290 | |
(0.25, 1) | 95 657 | 95 913 | |
(0.25, 1.5) | 95 508 | 95 765 | |
q = 7 | (0.25, 1, 1.5) | 95 947 | 96 240 |
Number . | Location . | AIC . | BIC . |
---|---|---|---|
q = 5 | 0.25 | 97 065 | 97 285 |
1 | 101 295 | 101 515 | |
1.5 | 102 593 | 102 813 | |
q = 6 | (0.25, 0.5) | 96 273 | 96 529 |
(0.5, 1) | 96 034 | 96 290 | |
(0.25, 1) | 95 657 | 95 913 | |
(0.25, 1.5) | 95 508 | 95 765 | |
q = 7 | (0.25, 1, 1.5) | 95 947 | 96 240 |
Number . | Location . | AIC . | BIC . |
---|---|---|---|
q = 5 | 0.25 | 97 065 | 97 285 |
1 | 101 295 | 101 515 | |
1.5 | 102 593 | 102 813 | |
q = 6 | (0.25, 0.5) | 96 273 | 96 529 |
(0.5, 1) | 96 034 | 96 290 | |
(0.25, 1) | 95 657 | 95 913 | |
(0.25, 1.5) | 95 508 | 95 765 | |
q = 7 | (0.25, 1, 1.5) | 95 947 | 96 240 |
Number . | Location . | AIC . | BIC . |
---|---|---|---|
q = 5 | 0.25 | 97 065 | 97 285 |
1 | 101 295 | 101 515 | |
1.5 | 102 593 | 102 813 | |
q = 6 | (0.25, 0.5) | 96 273 | 96 529 |
(0.5, 1) | 96 034 | 96 290 | |
(0.25, 1) | 95 657 | 95 913 | |
(0.25, 1.5) | 95 508 | 95 765 | |
q = 7 | (0.25, 1, 1.5) | 95 947 | 96 240 |
Table 3 shows the estimation results in case 2. Due to the high censoring rate, biases and standard errors increase for estimates of survival parameters compared with those in case 1. For parameters of particular interest , estimates obtained from the JM method perform slightly better than those from the TS method in terms of bias and CP, but the improvement is not as significant as that in case 1. An important reason is that the predicted random effects from the longitudinal model, , are close to bi, as the measurement error involved in longitudinal observations is small () in case 2.
Simulation results of the JM and TS methods in case 2. Average is the average value of parameter estimates in 500 MC replications; SD is the MC standard deviation of the estimates across simulated data sets; is the mean of bootstrap standard deviations calculated on bootstrap samples for each MC sample; CP is the 95% coverage probability defined as the percentage of repetitions in which true value falls into the calculated confidence interval.
. | JM . | TS . | ||||||
---|---|---|---|---|---|---|---|---|
True . | . | SD . | . | CP . | . | SD . | . | CP . |
Survival | ||||||||
–0.4190 | 0.1564 | 0.1549 | 0.948 | –0.4222 | 0.1556 | 0.1537 | 0.936 | |
–0.1180 | 0.1397 | 0.1417 | 0.944 | –0.1202 | 0.1393 | 0.1410 | 0.942 | |
0.7195 | 0.1016 | 0.1013 | 0.946 | 0.7288 | 0.1015 | 0.1012 | 0.940 | |
0.2025 | 0.1327 | 0.1319 | 0.950 | 0.1994 | 0.1326 | 0.1319 | 0.948 | |
0.3221 | 0.2036 | 0.2138 | 0.946 | 0.3157 | 0.2032 | 0.2137 | 0.944 | |
0.3416 | 0.1441 | 0.1384 | 0.934 | 0.3236 | 0.1389 | 0.1332 | 0.920 | |
0.2037 | 0.0844 | 0.0827 | 0.932 | 0.1758 | 0.0845 | 0.0823 | 0.870 | |
Longitudinal | ||||||||
6.1932 | 0.1241 | 0.1242 | 0.946 | 6.1944 | 0.1242 | 0.1240 | 0.948 | |
4.4954 | 0.1212 | 0.1221 | 0.946 | 4.4947 | 0.1211 | 0.1221 | 0.948 | |
4.9946 | 0.1199 | 0.1223 | 0.940 | 4.9950 | 0.1199 | 0.1222 | 0.940 | |
4.6951 | 0.1231 | 0.1226 | 0.944 | 4.6936 | 0.1231 | 0.1228 | 0.944 | |
4.9950 | 0.1260 | 0.1264 | 0.944 | 4.9907 | 0.1262 | 0.1262 | 0.946 | |
4.4860 | 0.1278 | 0.1276 | 0.946 | 4.4851 | 0.1278 | 0.1277 | 0.946 | |
6.7988 | 0.1301 | 0.1276 | 0.942 | 6.7887 | 0.1301 | 0.1275 | 0.942 | |
4.1939 | 0.1214 | 0.1217 | 0.940 | 4.1935 | 0.1214 | 0.1216 | 0.940 | |
4.4935 | 0.1212 | 0.1222 | 0.936 | 4.4939 | 0.1212 | 0.1214 | 0.934 | |
4.0976 | 0.1234 | 0.1240 | 0.948 | 4.0968 | 0.1234 | 0.1238 | 0.944 | |
4.4911 | 0.1307 | 0.1316 | 0.944 | 4.4922 | 0.1308 | 0.1315 | 0.946 | |
4.1955 | 0.1353 | 0.1357 | 0.948 | 4.1950 | 0.1352 | 0.1354 | 0.946 | |
6.3959 | 0.1277 | 0.1278 | 0.950 | 6.3969 | 0.1278 | 0.1277 | 0.948 | |
4.4951 | 0.1209 | 0.1218 | 0.934 | 3.0124 | 0.1209 | 0.1218 | 0.934 | |
4.5925 | 0.1208 | 0.1217 | 0.936 | 4.5931 | 0.1208 | 0.1217 | 0.936 | |
4.0972 | 0.1246 | 0.1243 | 0.940 | 4.0961 | 0.1246 | 0.1245 | 0.940 | |
4.4901 | 0.1331 | 0.1330 | 0.942 | 4.4915 | 0.1332 | 0.1329 | 0.946 | |
4.2920 | 0.1331 | 0.1335 | 0.936 | 4.2914 | 0.1331 | 0.1335 | 0.938 | |
–0.0495 | 0.0084 | 0.0085 | 0.952 | –0.0496 | 0.0084 | 0.0085 | 0.952 | |
0.0297 | 0.0124 | 0.0111 | 0.948 | 0.0297 | 0.0124 | 0.0112 | 0.948 | |
0.0907 | 0.0172 | 0.0175 | 0.942 | 0.0908 | 0.0172 | 0.0175 | 0.940 | |
0.0801 | 0.0042 | 0.0042 | 0.948 | 0.0799 | 0.0042 | 0.0042 | 0.978 | |
0.1993 | 0.0062 | 0.0063 | 0.942 | 0.1995 | 0.0062 | 0.0064 | 0.946 | |
0.1994 | 0.0070 | 0.0069 | 0.954 | 0.1997 | 0.0071 | 0.0069 | 0.958 | |
0.3988 | 0.0160 | 0.0170 | 0.952 | 0.3993 | 0.0160 | 0.0170 | 0.952 | |
0.4975 | 0.0268 | 0.0263 | 0.954 | 0.4971 | 0.0268 | 0.0264 | 0.950 | |
0.2975 | 0.0320 | 0.0322 | 0.952 | 0.2976 | 0.0320 | 0.0323 | 0.952 | |
0.1764 | 0.0010 | 0.0010 | 0.930 | 0.1764 | 0.0010 | 0.0010 | 0.930 |
. | JM . | TS . | ||||||
---|---|---|---|---|---|---|---|---|
True . | . | SD . | . | CP . | . | SD . | . | CP . |
Survival | ||||||||
–0.4190 | 0.1564 | 0.1549 | 0.948 | –0.4222 | 0.1556 | 0.1537 | 0.936 | |
–0.1180 | 0.1397 | 0.1417 | 0.944 | –0.1202 | 0.1393 | 0.1410 | 0.942 | |
0.7195 | 0.1016 | 0.1013 | 0.946 | 0.7288 | 0.1015 | 0.1012 | 0.940 | |
0.2025 | 0.1327 | 0.1319 | 0.950 | 0.1994 | 0.1326 | 0.1319 | 0.948 | |
0.3221 | 0.2036 | 0.2138 | 0.946 | 0.3157 | 0.2032 | 0.2137 | 0.944 | |
0.3416 | 0.1441 | 0.1384 | 0.934 | 0.3236 | 0.1389 | 0.1332 | 0.920 | |
0.2037 | 0.0844 | 0.0827 | 0.932 | 0.1758 | 0.0845 | 0.0823 | 0.870 | |
Longitudinal | ||||||||
6.1932 | 0.1241 | 0.1242 | 0.946 | 6.1944 | 0.1242 | 0.1240 | 0.948 | |
4.4954 | 0.1212 | 0.1221 | 0.946 | 4.4947 | 0.1211 | 0.1221 | 0.948 | |
4.9946 | 0.1199 | 0.1223 | 0.940 | 4.9950 | 0.1199 | 0.1222 | 0.940 | |
4.6951 | 0.1231 | 0.1226 | 0.944 | 4.6936 | 0.1231 | 0.1228 | 0.944 | |
4.9950 | 0.1260 | 0.1264 | 0.944 | 4.9907 | 0.1262 | 0.1262 | 0.946 | |
4.4860 | 0.1278 | 0.1276 | 0.946 | 4.4851 | 0.1278 | 0.1277 | 0.946 | |
6.7988 | 0.1301 | 0.1276 | 0.942 | 6.7887 | 0.1301 | 0.1275 | 0.942 | |
4.1939 | 0.1214 | 0.1217 | 0.940 | 4.1935 | 0.1214 | 0.1216 | 0.940 | |
4.4935 | 0.1212 | 0.1222 | 0.936 | 4.4939 | 0.1212 | 0.1214 | 0.934 | |
4.0976 | 0.1234 | 0.1240 | 0.948 | 4.0968 | 0.1234 | 0.1238 | 0.944 | |
4.4911 | 0.1307 | 0.1316 | 0.944 | 4.4922 | 0.1308 | 0.1315 | 0.946 | |
4.1955 | 0.1353 | 0.1357 | 0.948 | 4.1950 | 0.1352 | 0.1354 | 0.946 | |
6.3959 | 0.1277 | 0.1278 | 0.950 | 6.3969 | 0.1278 | 0.1277 | 0.948 | |
4.4951 | 0.1209 | 0.1218 | 0.934 | 3.0124 | 0.1209 | 0.1218 | 0.934 | |
4.5925 | 0.1208 | 0.1217 | 0.936 | 4.5931 | 0.1208 | 0.1217 | 0.936 | |
4.0972 | 0.1246 | 0.1243 | 0.940 | 4.0961 | 0.1246 | 0.1245 | 0.940 | |
4.4901 | 0.1331 | 0.1330 | 0.942 | 4.4915 | 0.1332 | 0.1329 | 0.946 | |
4.2920 | 0.1331 | 0.1335 | 0.936 | 4.2914 | 0.1331 | 0.1335 | 0.938 | |
–0.0495 | 0.0084 | 0.0085 | 0.952 | –0.0496 | 0.0084 | 0.0085 | 0.952 | |
0.0297 | 0.0124 | 0.0111 | 0.948 | 0.0297 | 0.0124 | 0.0112 | 0.948 | |
0.0907 | 0.0172 | 0.0175 | 0.942 | 0.0908 | 0.0172 | 0.0175 | 0.940 | |
0.0801 | 0.0042 | 0.0042 | 0.948 | 0.0799 | 0.0042 | 0.0042 | 0.978 | |
0.1993 | 0.0062 | 0.0063 | 0.942 | 0.1995 | 0.0062 | 0.0064 | 0.946 | |
0.1994 | 0.0070 | 0.0069 | 0.954 | 0.1997 | 0.0071 | 0.0069 | 0.958 | |
0.3988 | 0.0160 | 0.0170 | 0.952 | 0.3993 | 0.0160 | 0.0170 | 0.952 | |
0.4975 | 0.0268 | 0.0263 | 0.954 | 0.4971 | 0.0268 | 0.0264 | 0.950 | |
0.2975 | 0.0320 | 0.0322 | 0.952 | 0.2976 | 0.0320 | 0.0323 | 0.952 | |
0.1764 | 0.0010 | 0.0010 | 0.930 | 0.1764 | 0.0010 | 0.0010 | 0.930 |
Simulation results of the JM and TS methods in case 2. Average is the average value of parameter estimates in 500 MC replications; SD is the MC standard deviation of the estimates across simulated data sets; is the mean of bootstrap standard deviations calculated on bootstrap samples for each MC sample; CP is the 95% coverage probability defined as the percentage of repetitions in which true value falls into the calculated confidence interval.
. | JM . | TS . | ||||||
---|---|---|---|---|---|---|---|---|
True . | . | SD . | . | CP . | . | SD . | . | CP . |
Survival | ||||||||
–0.4190 | 0.1564 | 0.1549 | 0.948 | –0.4222 | 0.1556 | 0.1537 | 0.936 | |
–0.1180 | 0.1397 | 0.1417 | 0.944 | –0.1202 | 0.1393 | 0.1410 | 0.942 | |
0.7195 | 0.1016 | 0.1013 | 0.946 | 0.7288 | 0.1015 | 0.1012 | 0.940 | |
0.2025 | 0.1327 | 0.1319 | 0.950 | 0.1994 | 0.1326 | 0.1319 | 0.948 | |
0.3221 | 0.2036 | 0.2138 | 0.946 | 0.3157 | 0.2032 | 0.2137 | 0.944 | |
0.3416 | 0.1441 | 0.1384 | 0.934 | 0.3236 | 0.1389 | 0.1332 | 0.920 | |
0.2037 | 0.0844 | 0.0827 | 0.932 | 0.1758 | 0.0845 | 0.0823 | 0.870 | |
Longitudinal | ||||||||
6.1932 | 0.1241 | 0.1242 | 0.946 | 6.1944 | 0.1242 | 0.1240 | 0.948 | |
4.4954 | 0.1212 | 0.1221 | 0.946 | 4.4947 | 0.1211 | 0.1221 | 0.948 | |
4.9946 | 0.1199 | 0.1223 | 0.940 | 4.9950 | 0.1199 | 0.1222 | 0.940 | |
4.6951 | 0.1231 | 0.1226 | 0.944 | 4.6936 | 0.1231 | 0.1228 | 0.944 | |
4.9950 | 0.1260 | 0.1264 | 0.944 | 4.9907 | 0.1262 | 0.1262 | 0.946 | |
4.4860 | 0.1278 | 0.1276 | 0.946 | 4.4851 | 0.1278 | 0.1277 | 0.946 | |
6.7988 | 0.1301 | 0.1276 | 0.942 | 6.7887 | 0.1301 | 0.1275 | 0.942 | |
4.1939 | 0.1214 | 0.1217 | 0.940 | 4.1935 | 0.1214 | 0.1216 | 0.940 | |
4.4935 | 0.1212 | 0.1222 | 0.936 | 4.4939 | 0.1212 | 0.1214 | 0.934 | |
4.0976 | 0.1234 | 0.1240 | 0.948 | 4.0968 | 0.1234 | 0.1238 | 0.944 | |
4.4911 | 0.1307 | 0.1316 | 0.944 | 4.4922 | 0.1308 | 0.1315 | 0.946 | |
4.1955 | 0.1353 | 0.1357 | 0.948 | 4.1950 | 0.1352 | 0.1354 | 0.946 | |
6.3959 | 0.1277 | 0.1278 | 0.950 | 6.3969 | 0.1278 | 0.1277 | 0.948 | |
4.4951 | 0.1209 | 0.1218 | 0.934 | 3.0124 | 0.1209 | 0.1218 | 0.934 | |
4.5925 | 0.1208 | 0.1217 | 0.936 | 4.5931 | 0.1208 | 0.1217 | 0.936 | |
4.0972 | 0.1246 | 0.1243 | 0.940 | 4.0961 | 0.1246 | 0.1245 | 0.940 | |
4.4901 | 0.1331 | 0.1330 | 0.942 | 4.4915 | 0.1332 | 0.1329 | 0.946 | |
4.2920 | 0.1331 | 0.1335 | 0.936 | 4.2914 | 0.1331 | 0.1335 | 0.938 | |
–0.0495 | 0.0084 | 0.0085 | 0.952 | –0.0496 | 0.0084 | 0.0085 | 0.952 | |
0.0297 | 0.0124 | 0.0111 | 0.948 | 0.0297 | 0.0124 | 0.0112 | 0.948 | |
0.0907 | 0.0172 | 0.0175 | 0.942 | 0.0908 | 0.0172 | 0.0175 | 0.940 | |
0.0801 | 0.0042 | 0.0042 | 0.948 | 0.0799 | 0.0042 | 0.0042 | 0.978 | |
0.1993 | 0.0062 | 0.0063 | 0.942 | 0.1995 | 0.0062 | 0.0064 | 0.946 | |
0.1994 | 0.0070 | 0.0069 | 0.954 | 0.1997 | 0.0071 | 0.0069 | 0.958 | |
0.3988 | 0.0160 | 0.0170 | 0.952 | 0.3993 | 0.0160 | 0.0170 | 0.952 | |
0.4975 | 0.0268 | 0.0263 | 0.954 | 0.4971 | 0.0268 | 0.0264 | 0.950 | |
0.2975 | 0.0320 | 0.0322 | 0.952 | 0.2976 | 0.0320 | 0.0323 | 0.952 | |
0.1764 | 0.0010 | 0.0010 | 0.930 | 0.1764 | 0.0010 | 0.0010 | 0.930 |
. | JM . | TS . | ||||||
---|---|---|---|---|---|---|---|---|
True . | . | SD . | . | CP . | . | SD . | . | CP . |
Survival | ||||||||
–0.4190 | 0.1564 | 0.1549 | 0.948 | –0.4222 | 0.1556 | 0.1537 | 0.936 | |
–0.1180 | 0.1397 | 0.1417 | 0.944 | –0.1202 | 0.1393 | 0.1410 | 0.942 | |
0.7195 | 0.1016 | 0.1013 | 0.946 | 0.7288 | 0.1015 | 0.1012 | 0.940 | |
0.2025 | 0.1327 | 0.1319 | 0.950 | 0.1994 | 0.1326 | 0.1319 | 0.948 | |
0.3221 | 0.2036 | 0.2138 | 0.946 | 0.3157 | 0.2032 | 0.2137 | 0.944 | |
0.3416 | 0.1441 | 0.1384 | 0.934 | 0.3236 | 0.1389 | 0.1332 | 0.920 | |
0.2037 | 0.0844 | 0.0827 | 0.932 | 0.1758 | 0.0845 | 0.0823 | 0.870 | |
Longitudinal | ||||||||
6.1932 | 0.1241 | 0.1242 | 0.946 | 6.1944 | 0.1242 | 0.1240 | 0.948 | |
4.4954 | 0.1212 | 0.1221 | 0.946 | 4.4947 | 0.1211 | 0.1221 | 0.948 | |
4.9946 | 0.1199 | 0.1223 | 0.940 | 4.9950 | 0.1199 | 0.1222 | 0.940 | |
4.6951 | 0.1231 | 0.1226 | 0.944 | 4.6936 | 0.1231 | 0.1228 | 0.944 | |
4.9950 | 0.1260 | 0.1264 | 0.944 | 4.9907 | 0.1262 | 0.1262 | 0.946 | |
4.4860 | 0.1278 | 0.1276 | 0.946 | 4.4851 | 0.1278 | 0.1277 | 0.946 | |
6.7988 | 0.1301 | 0.1276 | 0.942 | 6.7887 | 0.1301 | 0.1275 | 0.942 | |
4.1939 | 0.1214 | 0.1217 | 0.940 | 4.1935 | 0.1214 | 0.1216 | 0.940 | |
4.4935 | 0.1212 | 0.1222 | 0.936 | 4.4939 | 0.1212 | 0.1214 | 0.934 | |
4.0976 | 0.1234 | 0.1240 | 0.948 | 4.0968 | 0.1234 | 0.1238 | 0.944 | |
4.4911 | 0.1307 | 0.1316 | 0.944 | 4.4922 | 0.1308 | 0.1315 | 0.946 | |
4.1955 | 0.1353 | 0.1357 | 0.948 | 4.1950 | 0.1352 | 0.1354 | 0.946 | |
6.3959 | 0.1277 | 0.1278 | 0.950 | 6.3969 | 0.1278 | 0.1277 | 0.948 | |
4.4951 | 0.1209 | 0.1218 | 0.934 | 3.0124 | 0.1209 | 0.1218 | 0.934 | |
4.5925 | 0.1208 | 0.1217 | 0.936 | 4.5931 | 0.1208 | 0.1217 | 0.936 | |
4.0972 | 0.1246 | 0.1243 | 0.940 | 4.0961 | 0.1246 | 0.1245 | 0.940 | |
4.4901 | 0.1331 | 0.1330 | 0.942 | 4.4915 | 0.1332 | 0.1329 | 0.946 | |
4.2920 | 0.1331 | 0.1335 | 0.936 | 4.2914 | 0.1331 | 0.1335 | 0.938 | |
–0.0495 | 0.0084 | 0.0085 | 0.952 | –0.0496 | 0.0084 | 0.0085 | 0.952 | |
0.0297 | 0.0124 | 0.0111 | 0.948 | 0.0297 | 0.0124 | 0.0112 | 0.948 | |
0.0907 | 0.0172 | 0.0175 | 0.942 | 0.0908 | 0.0172 | 0.0175 | 0.940 | |
0.0801 | 0.0042 | 0.0042 | 0.948 | 0.0799 | 0.0042 | 0.0042 | 0.978 | |
0.1993 | 0.0062 | 0.0063 | 0.942 | 0.1995 | 0.0062 | 0.0064 | 0.946 | |
0.1994 | 0.0070 | 0.0069 | 0.954 | 0.1997 | 0.0071 | 0.0069 | 0.958 | |
0.3988 | 0.0160 | 0.0170 | 0.952 | 0.3993 | 0.0160 | 0.0170 | 0.952 | |
0.4975 | 0.0268 | 0.0263 | 0.954 | 0.4971 | 0.0268 | 0.0264 | 0.950 | |
0.2975 | 0.0320 | 0.0322 | 0.952 | 0.2976 | 0.0320 | 0.0323 | 0.952 | |
0.1764 | 0.0010 | 0.0010 | 0.930 | 0.1764 | 0.0010 | 0.0010 | 0.930 |
6.3 Case 3: Simulation study for a nonparametric setting
In this final simulation study, we examine the performance of our proposed method (the spline based sieve approximation to a biomarker trajectory and the EM algorithm in estimation) when the true biomarker trajectory is not exactly a spline function as specified in (3.3). We set the true longitudinal trajectory for the i-th individual as for , where and are independent random parameters generated from and U(4, 8), respectively. Obviously, corresponds to the degree of fluctuation for the i-th trajectory and is related to the biomarker level. The measurement times are specified as (every 0.5 year after 2 years) during the follow-up interval and the measurement error is generated from , independently of and . The baseline covariates xi and outside stimulus zi are not incorporated for simplicity. For the survival submodel, the baseline hazard function is specified as if t > 2 and 0 otherwise. We generate a binary baseline covariate wi from Bernoulli distribution with probability 0.5. Censoring times Ci, are independently generated from U(3, 10), which, together with the end of study (τ = 6), lead to approximately 40% censoring. Truncated by event times and right censoring, the number of longitudinal observations ni varies between 6 and 14.
We fit the joint model given by (3.1), (3.2), (3.3) and (3.6) to the simulated data. Note that the spline regression model (3.3), in this case, is an approximation or working model. To determine the number and location of interior knots, we first specify several candidate knot patterns based on quantiles of measurement times and the follow-up interval (see Section SC.2 of the supplementary material available at Biostatistics online), and then select the best pattern among them via AIC and BIC, which are calculated by simply fitting the longitudinal submodel. Table 4 shows the estimation result for survival parameters obtained from different methods. In addition to JM and TS mentioned before, the performance of the true model (a benchmark) where the biomarker level and variability are specified from the true as in the data generating process, and that of the standard joint model (called “No Variability” in Table 4) without biomaker variability, are also reported in Table 4. It is expected that JM and TS may not perform as well as in case 1 and case 2 due to the extra bias resulting from the approximation in (3.3). Compared with γ and α1, the estimation of α2, in both JM and TS, suffers from significant bias and thus under-coverage of CP. However, if the biomarker variability is removed, as implemented with the standard joint model, both γ and α1 are estimated with substantial bias. In this sense, taking the biomarker variability into account benefits the estimation of effects of the other predictors. Table 5 shows the estimation results when the biomarker variability is not involved in generating event times, i.e., the true value of α2 is zero. These three models produce similar estimates and the null effect of biomarker variability can be successfully detected. A more challenging case (case 4) where is no longer a polynomial function but a sine function instead, is considered in Section SC.3 of the supplementary material available at Biostatistics online. JM and TS show very similar performance in this scenario since approximating a sine function via a spline function is the dominant source of bias.
Simulation results in case 3 among 500 MC replications. SD is the MC standard deviation of the estimates across simulated data sets; CP is the 95% coverage probability.
. | JM . | TS . | No Variability . | True model . | ||||||||
---|---|---|---|---|---|---|---|---|---|---|---|---|
True . | . | SD . | CP . | . | SD . | CP . | . | SD . | CP . | . | SD . | CP . |
–0.9884 | 0.0778 | 0.944 | –0.9664 | 0.0761 | 0.920 | –0.8983 | 0.0765 | 0.726 | –0.9978 | 0.0760 | 0.948 | |
0.2918 | 0.0319 | 0.936 | 0.2805 | 0.0303 | 0.894 | 0.2260 | 0.0319 | 0.356 | 0.3020 | 0.0314 | 0.952 | |
0.2674 | 0.0301 | 0.720 | 0.2438 | 0.0275 | 0.664 | – | – | – | 0.3009 | 0.0282 | 0.960 |
. | JM . | TS . | No Variability . | True model . | ||||||||
---|---|---|---|---|---|---|---|---|---|---|---|---|
True . | . | SD . | CP . | . | SD . | CP . | . | SD . | CP . | . | SD . | CP . |
–0.9884 | 0.0778 | 0.944 | –0.9664 | 0.0761 | 0.920 | –0.8983 | 0.0765 | 0.726 | –0.9978 | 0.0760 | 0.948 | |
0.2918 | 0.0319 | 0.936 | 0.2805 | 0.0303 | 0.894 | 0.2260 | 0.0319 | 0.356 | 0.3020 | 0.0314 | 0.952 | |
0.2674 | 0.0301 | 0.720 | 0.2438 | 0.0275 | 0.664 | – | – | – | 0.3009 | 0.0282 | 0.960 |
Simulation results in case 3 among 500 MC replications. SD is the MC standard deviation of the estimates across simulated data sets; CP is the 95% coverage probability.
. | JM . | TS . | No Variability . | True model . | ||||||||
---|---|---|---|---|---|---|---|---|---|---|---|---|
True . | . | SD . | CP . | . | SD . | CP . | . | SD . | CP . | . | SD . | CP . |
–0.9884 | 0.0778 | 0.944 | –0.9664 | 0.0761 | 0.920 | –0.8983 | 0.0765 | 0.726 | –0.9978 | 0.0760 | 0.948 | |
0.2918 | 0.0319 | 0.936 | 0.2805 | 0.0303 | 0.894 | 0.2260 | 0.0319 | 0.356 | 0.3020 | 0.0314 | 0.952 | |
0.2674 | 0.0301 | 0.720 | 0.2438 | 0.0275 | 0.664 | – | – | – | 0.3009 | 0.0282 | 0.960 |
. | JM . | TS . | No Variability . | True model . | ||||||||
---|---|---|---|---|---|---|---|---|---|---|---|---|
True . | . | SD . | CP . | . | SD . | CP . | . | SD . | CP . | . | SD . | CP . |
–0.9884 | 0.0778 | 0.944 | –0.9664 | 0.0761 | 0.920 | –0.8983 | 0.0765 | 0.726 | –0.9978 | 0.0760 | 0.948 | |
0.2918 | 0.0319 | 0.936 | 0.2805 | 0.0303 | 0.894 | 0.2260 | 0.0319 | 0.356 | 0.3020 | 0.0314 | 0.952 | |
0.2674 | 0.0301 | 0.720 | 0.2438 | 0.0275 | 0.664 | – | – | – | 0.3009 | 0.0282 | 0.960 |
Simulation results in case 3 among 500 MC replications when the true value of α2 is set as zero. SD is the MC standard deviation of the estimates across simulated data sets; CP is the 95% coverage probability.
. | JM . | TS . | No Variability . | True model . | ||||||||
---|---|---|---|---|---|---|---|---|---|---|---|---|
True . | . | SD . | CP . | . | SD . | CP . | . | SD . | CP . | . | SD . | CP . |
–0.9995 | 0.0893 | 0.946 | –1.0013 | 0.0897 | 0.946 | –1.0016 | 0.0914 | 0.946 | –1.0049 | 0.0897 | 0.948 | |
0.2898 | 0.0392 | 0.926 | 0.2827 | 0.0374 | 0.924 | 0.2875 | 0.0382 | 0.924 | 0.3019 | 0.0340 | 0.950 | |
0.0022 | 0.0331 | 0.948 | –0.0047 | 0.0345 | 0.950 | – | – | – | 0.0004 | 0.0305 | 0.952 |
. | JM . | TS . | No Variability . | True model . | ||||||||
---|---|---|---|---|---|---|---|---|---|---|---|---|
True . | . | SD . | CP . | . | SD . | CP . | . | SD . | CP . | . | SD . | CP . |
–0.9995 | 0.0893 | 0.946 | –1.0013 | 0.0897 | 0.946 | –1.0016 | 0.0914 | 0.946 | –1.0049 | 0.0897 | 0.948 | |
0.2898 | 0.0392 | 0.926 | 0.2827 | 0.0374 | 0.924 | 0.2875 | 0.0382 | 0.924 | 0.3019 | 0.0340 | 0.950 | |
0.0022 | 0.0331 | 0.948 | –0.0047 | 0.0345 | 0.950 | – | – | – | 0.0004 | 0.0305 | 0.952 |
Simulation results in case 3 among 500 MC replications when the true value of α2 is set as zero. SD is the MC standard deviation of the estimates across simulated data sets; CP is the 95% coverage probability.
. | JM . | TS . | No Variability . | True model . | ||||||||
---|---|---|---|---|---|---|---|---|---|---|---|---|
True . | . | SD . | CP . | . | SD . | CP . | . | SD . | CP . | . | SD . | CP . |
–0.9995 | 0.0893 | 0.946 | –1.0013 | 0.0897 | 0.946 | –1.0016 | 0.0914 | 0.946 | –1.0049 | 0.0897 | 0.948 | |
0.2898 | 0.0392 | 0.926 | 0.2827 | 0.0374 | 0.924 | 0.2875 | 0.0382 | 0.924 | 0.3019 | 0.0340 | 0.950 | |
0.0022 | 0.0331 | 0.948 | –0.0047 | 0.0345 | 0.950 | – | – | – | 0.0004 | 0.0305 | 0.952 |
. | JM . | TS . | No Variability . | True model . | ||||||||
---|---|---|---|---|---|---|---|---|---|---|---|---|
True . | . | SD . | CP . | . | SD . | CP . | . | SD . | CP . | . | SD . | CP . |
–0.9995 | 0.0893 | 0.946 | –1.0013 | 0.0897 | 0.946 | –1.0016 | 0.0914 | 0.946 | –1.0049 | 0.0897 | 0.948 | |
0.2898 | 0.0392 | 0.926 | 0.2827 | 0.0374 | 0.924 | 0.2875 | 0.0382 | 0.924 | 0.3019 | 0.0340 | 0.950 | |
0.0022 | 0.0331 | 0.948 | –0.0047 | 0.0345 | 0.950 | – | – | – | 0.0004 | 0.0305 | 0.952 |
7 Real-data analysis
In the MRC trial, 4396 patients aged 65–74 were randomized to receive diuretic, β blocker or placebo. SBP values were measured fornightly for the first month, then monthly to 3 months and every 3 months thereafter. Cardiovascular (CV) events including stroke, whether non-fatal or fatal; and myocardial infarction, whether fatal or nonfatal were recorded during the follow-up. Figure 3(a) illustrates the change in group average of SBP observations (divided by 30) over time. Several points are highlighted here. (i) The average SBP trends are different in different treatment groups and therefore a treatment-dependent longitudinal model is preferred for modeling SBP trajectories. (ii) The regular peaks, occurring at the end of each year (1, 2, 3, 4, 5 years) as well as the entry visit (0 year), correspond to the measurements made by doctors with the other measurements made by nurses. The marked increase at these time points can be explained by the “white coat” effect, a pressor response to the presence of doctors or other kind of stressful circumstances (Lever and Brennan, 1993; Party and others, 1992), which, in our model, is treated as an outside stimulus and is excluded from the inherent SBP trend (Carr and others, 2012). (iii) All groups experienced immediate drop-off after entry. The drop was steepest in the first 2-weeks, then continued, more gently, for about 3 months (0.25 year) followed by a comparatively stable stage. Therefore global polynomials of lower degree may not perform well in capturing the SBP trend over time and so cubic B-spline basis functions are used instead.
Table 6 shows the estimation results from the proposed joint model (JM), the standard joint model (No Variability) and the naive proportional hazard (PH) model. Both the proposed and standard joint models are estimated with the algorithm in Section 5 and the standard errors are obtained via bootstrap. The association between CV and the defined measure of SBP variability (α2), after adjustment for underlying SBP level and baseline covariates, is significant under JM. Specifically, the hazard ratio associated with 1-SD increase in SBP variability (SD for defined SBP variability: 0.535) is 1.14 (95% confidence interval: 1.02–1.27) and 1.20 (95% confidence interval: 1.02–1.40) for underlying SBP level (SD for SBP value: 0.485). The effect of diuretic treatment (γ1), after adjusting for other baseline predictors, SBP level and variability, still appears to be highly significant in decreasing CV risk, while the effect of β blocker (γ2) is weak. Both joint models lead to similar conclusion on this point. Compared with the estimation results given by PH, the treatment effects (γ1 and γ2) are attenuated by incorporating SBP relevant predictors in joint models. It seems that the effects of diuretic and β blocker treatments on CV risk can be better captured (explained) through their impact on SBP level and variability together since the treatment effects diminish more in the proposed model than those in the standard joint model. In terms of model performance, the AIC (BIC) values for the proposed and standard joint models are 121 003.6 (121 221.9) and 121 204.3 (121 416.4), respectively.
Estimation results for MRC data under different models. JM refers to the proposed joint model; No Variability refers to the standard joint model with the restriction and PH is the proportional hazards model involving baseline covariates only. in both JM and No Variability denote the standard deviation based on 100 bootstrap samples and SD’s in PH are obtained from R function “coxph”.
. | JM . | No Variability . | PH . | |||
---|---|---|---|---|---|---|
Parameters . | . | . | . | . | . | SD . |
Survival | ||||||
γ1 | –0.4095 | 0.1389 | –0.4425 | 0.1386 | –0.5974 | 0.1581 |
γ2 | –0.0967 | 0.1701 | –0.1263 | 0.1658 | –0.2679 | 0.1386 |
γ3 | 0.7081 | 0.1228 | 0.7121 | 0.1264 | 0.6827 | 0.1159 |
γ4 | 0.2219 | 0.1430 | 0.2215 | 0.1405 | 0.2280 | 0.1406 |
γ5 | 0.3557 | 0.2142 | 0.3584 | 0.2110 | 0.3581 | 0.2092 |
α1 | 0.3693 | 0.1659 | 0.3015 | 0.1372 | – | – |
α2 | 0.2378 | 0.1035 | – | – | – | – |
Longitudinal | ||||||
η1 | –0.0466 | 0.0106 | –0.0471 | 0.0102 | – | – |
η2 | 0.0330 | 0.0146 | 0.0328 | 0.0147 | – | – |
η3 | 0.0928 | 0.0208 | 0.0927 | 0.0204 | – | – |
ξ | 0.0789 | 0.0049 | 0.0796 | 0.0047 | – | – |
D22 | 0.2189 | 0.0069 | 0.2219 | 0.0068 | – | – |
D33 | 0.2161 | 0.0082 | 0.2206 | 0.0080 | – | – |
D44 | 0.4064 | 0.0175 | 0.4090 | 0.0178 | – | – |
D55 | 0.5362 | 0.0221 | 0.5395 | 0.0218 | – | – |
D66 | 0.3037 | 0.0128 | 0.3036 | 0.0125 | – | – |
0.1798 | 0.0016 | 0.1798 | 0.0016 | – | – |
. | JM . | No Variability . | PH . | |||
---|---|---|---|---|---|---|
Parameters . | . | . | . | . | . | SD . |
Survival | ||||||
γ1 | –0.4095 | 0.1389 | –0.4425 | 0.1386 | –0.5974 | 0.1581 |
γ2 | –0.0967 | 0.1701 | –0.1263 | 0.1658 | –0.2679 | 0.1386 |
γ3 | 0.7081 | 0.1228 | 0.7121 | 0.1264 | 0.6827 | 0.1159 |
γ4 | 0.2219 | 0.1430 | 0.2215 | 0.1405 | 0.2280 | 0.1406 |
γ5 | 0.3557 | 0.2142 | 0.3584 | 0.2110 | 0.3581 | 0.2092 |
α1 | 0.3693 | 0.1659 | 0.3015 | 0.1372 | – | – |
α2 | 0.2378 | 0.1035 | – | – | – | – |
Longitudinal | ||||||
η1 | –0.0466 | 0.0106 | –0.0471 | 0.0102 | – | – |
η2 | 0.0330 | 0.0146 | 0.0328 | 0.0147 | – | – |
η3 | 0.0928 | 0.0208 | 0.0927 | 0.0204 | – | – |
ξ | 0.0789 | 0.0049 | 0.0796 | 0.0047 | – | – |
D22 | 0.2189 | 0.0069 | 0.2219 | 0.0068 | – | – |
D33 | 0.2161 | 0.0082 | 0.2206 | 0.0080 | – | – |
D44 | 0.4064 | 0.0175 | 0.4090 | 0.0178 | – | – |
D55 | 0.5362 | 0.0221 | 0.5395 | 0.0218 | – | – |
D66 | 0.3037 | 0.0128 | 0.3036 | 0.0125 | – | – |
0.1798 | 0.0016 | 0.1798 | 0.0016 | – | – |
Estimation results for MRC data under different models. JM refers to the proposed joint model; No Variability refers to the standard joint model with the restriction and PH is the proportional hazards model involving baseline covariates only. in both JM and No Variability denote the standard deviation based on 100 bootstrap samples and SD’s in PH are obtained from R function “coxph”.
. | JM . | No Variability . | PH . | |||
---|---|---|---|---|---|---|
Parameters . | . | . | . | . | . | SD . |
Survival | ||||||
γ1 | –0.4095 | 0.1389 | –0.4425 | 0.1386 | –0.5974 | 0.1581 |
γ2 | –0.0967 | 0.1701 | –0.1263 | 0.1658 | –0.2679 | 0.1386 |
γ3 | 0.7081 | 0.1228 | 0.7121 | 0.1264 | 0.6827 | 0.1159 |
γ4 | 0.2219 | 0.1430 | 0.2215 | 0.1405 | 0.2280 | 0.1406 |
γ5 | 0.3557 | 0.2142 | 0.3584 | 0.2110 | 0.3581 | 0.2092 |
α1 | 0.3693 | 0.1659 | 0.3015 | 0.1372 | – | – |
α2 | 0.2378 | 0.1035 | – | – | – | – |
Longitudinal | ||||||
η1 | –0.0466 | 0.0106 | –0.0471 | 0.0102 | – | – |
η2 | 0.0330 | 0.0146 | 0.0328 | 0.0147 | – | – |
η3 | 0.0928 | 0.0208 | 0.0927 | 0.0204 | – | – |
ξ | 0.0789 | 0.0049 | 0.0796 | 0.0047 | – | – |
D22 | 0.2189 | 0.0069 | 0.2219 | 0.0068 | – | – |
D33 | 0.2161 | 0.0082 | 0.2206 | 0.0080 | – | – |
D44 | 0.4064 | 0.0175 | 0.4090 | 0.0178 | – | – |
D55 | 0.5362 | 0.0221 | 0.5395 | 0.0218 | – | – |
D66 | 0.3037 | 0.0128 | 0.3036 | 0.0125 | – | – |
0.1798 | 0.0016 | 0.1798 | 0.0016 | – | – |
. | JM . | No Variability . | PH . | |||
---|---|---|---|---|---|---|
Parameters . | . | . | . | . | . | SD . |
Survival | ||||||
γ1 | –0.4095 | 0.1389 | –0.4425 | 0.1386 | –0.5974 | 0.1581 |
γ2 | –0.0967 | 0.1701 | –0.1263 | 0.1658 | –0.2679 | 0.1386 |
γ3 | 0.7081 | 0.1228 | 0.7121 | 0.1264 | 0.6827 | 0.1159 |
γ4 | 0.2219 | 0.1430 | 0.2215 | 0.1405 | 0.2280 | 0.1406 |
γ5 | 0.3557 | 0.2142 | 0.3584 | 0.2110 | 0.3581 | 0.2092 |
α1 | 0.3693 | 0.1659 | 0.3015 | 0.1372 | – | – |
α2 | 0.2378 | 0.1035 | – | – | – | – |
Longitudinal | ||||||
η1 | –0.0466 | 0.0106 | –0.0471 | 0.0102 | – | – |
η2 | 0.0330 | 0.0146 | 0.0328 | 0.0147 | – | – |
η3 | 0.0928 | 0.0208 | 0.0927 | 0.0204 | – | – |
ξ | 0.0789 | 0.0049 | 0.0796 | 0.0047 | – | – |
D22 | 0.2189 | 0.0069 | 0.2219 | 0.0068 | – | – |
D33 | 0.2161 | 0.0082 | 0.2206 | 0.0080 | – | – |
D44 | 0.4064 | 0.0175 | 0.4090 | 0.0178 | – | – |
D55 | 0.5362 | 0.0221 | 0.5395 | 0.0218 | – | – |
D66 | 0.3037 | 0.0128 | 0.3036 | 0.0125 | – | – |
0.1798 | 0.0016 | 0.1798 | 0.0016 | – | – |
Figure 4 shows the fitted SBP trajectory and variability in each treatment group. Both diuretic and β blocker treatments significantly reduce SBP level compared with that in the placebo group. Although the diuretic group exhibits a more immediate drop in SBP value after entry, both active treatment groups reach similar SBP level after two years. However, the square root of cumulative SBP variability appears to be larger in the β blocker group than the other two groups, which concurs with existing findings in medicine that β blockers tend to induce excessive intraindividual SBP variability over time (Carr and others, 2012; Rothwell and others, 2010 a).
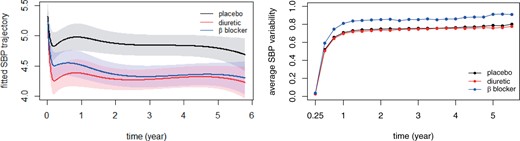
Fitted SBP trajectories and group average SBP variability under JM. Left: fitted SBP mean trends in different treatment groups and corresponding 95% confidence intervals. Right: group average of estimated SBP variability by different treatments.
8 Discussion
This article focuses on how to characterize the biological variability or instability of underlying biomarker trajectories in joint modeling of longitudinal and time-to-event data. Borrowing ideas from the roughness penalty in smoothing splines and penalized splines, we propose a similar quantity to evaluate the fluctuation of subject-specific longitudinal trajectories which are modeled by mixed-effects regression splines. To support the use of spline bases in modeling longitudinal data and further our proposed variability measure, longitudinal observations should present nonlinear profiles and observation times should not be very sparse. To ensure the convergence rate of MLEs, it is required that grows at a rate greater than . Otherwise, approximation errors resulting from calculating expectations in the EM algorithm will dominate the estimator’s asymptotic behavior.
The performance of the proposed variability measure depends heavily on the number and locations of knots. For example, trajectories in the right-hand graph of Figure 1 tend to flatten out after time 1 due to the fact that no interior knots are placed in the later period (knot locations in this case are 0.25 and 1.5), whereas similar phenomena do not occur in Figure 2 as the interior knots, in the toy example, are evenly spaced. Increasing the number of knots may alleviate the sensitivity to the locations of knots at the price of increasing model complexity. Although standard methods, e.g., AIC and BIC, provide a way to choose the knot sequence, further exploration and more attention should be given to this issue.
In some cases, it might be more reasonable to use the average variability or the variability over a recent period as a predictor. More generally, we can consider a weighted variability where the weight function can be specified within a parametric family with unknown parameters or be modeled in a nonparametric way. The time window over which the biomarker variability is more relevant in predicting survival events can be implied from the estimate of . Since is still a quadratic form of random effects, the methodology developed in this article can be straightforwardly applied to the weighted form.
9 Supplementary Material
R code for the simulations is available at https://github.com/cywang0315/R_simulations. Supplementary material is available at http://biostatistics.oxfordjournals.org.
Acknowledgments
We are grateful to the two anonymous reviewers and the associate editor for their very helpful comments and suggestions. We thank the Medical Research Council (MRC) of the UK for access to the MRC elderly trial data.
Conflict of Interest: The authors have declared no conflict of interest.
Funding
Our work was supported in part by the National Natural Science Foundation of China (12271047), and in part by the Guangdong Provincial Key Laboratory of Interdisciplinary Research and Application for Data Science, BNU-HKBU United International College (2022B1212010006).
References