-
PDF
- Split View
-
Views
-
Cite
Cite
Kun Zhang, Yan Fu, Wen-Feng Zeng, Kun He, Hao Chi, Chao Liu, Yan-Chang Li, Yuan Gao, Ping Xu, Si-Min He, A note on the false discovery rate of novel peptides in proteogenomics, Bioinformatics, Volume 31, Issue 20, October 2015, Pages 3249–3253, https://doi.org/10.1093/bioinformatics/btv340
- Share Icon Share
Abstract
Motivation: Proteogenomics has been well accepted as a tool to discover novel genes. In most conventional proteogenomic studies, a global false discovery rate is used to filter out false positives for identifying credible novel peptides. However, it has been found that the actual level of false positives in novel peptides is often out of control and behaves differently for different genomes.
Results: To quantitatively model this problem, we theoretically analyze the subgroup false discovery rates of annotated and novel peptides. Our analysis shows that the annotation completeness ratio of a genome is the dominant factor influencing the subgroup FDR of novel peptides. Experimental results on two real datasets of Escherichia coli and Mycobacterium tuberculosis support our conjecture.
Contact: [email protected] or [email protected] or [email protected]
Supplementary information: Supplementary data are available at Bioinformatics online.
1 Introduction
Tandem mass spectrometry (MS/MS)-based proteogenomics (Jaffe et al., 2004) has been applied to refinement of annotated genes, discovery of novel genes (Kim et al., 2014), personal genomics and disease-related studies (Zhang et al., 2014). Unlike traditional genomic annotation techniques, such as in silico (ab initio or comparative) or cDNA-seq-based methods, proteogenomics allows validating protein-coding genes directly at the protein level, which is more favorable (Renuse et al., 2011). To identify novel genes, researchers usually search the experimental MS/MS spectra against a large protein database that is constructed from the genomic or transcriptomic sequences and filter the search results to control the false discovery rate (FDR). It has been observed previously that, under a fixed FDR, the inflated database generated by, e.g. six-open-reading-frame (6-ORF) translation of a whole genome significantly decreases the sensitivity of peptide identification (Blakeley et al., 2012). However, few studies probe into the effect of the large database on the estimated FDR, especially for the novel peptides.
Most proteogenomic studies estimate a global FDR for all peptide identifications (Borchert et al., 2010; Chaerkady et al., 2011; Merrihew et al., 2008). That is, the identifications of annotated peptides and novel peptides are subject to FDR estimation in combination. Some researchers noted the high actual FDR of novel peptides and therefore employed more stringent filtering strategies, e.g. post error probability (Brosch et al., 2011) or separate FDRs for annotated and novel peptides (Branca et al., 2014). Recently, Krug et al. (2013) highlighted that for well-annotated genomes, such as the Escherichia coli genome, the post error probability distribution of novel peptide hits is almost identical to that of decoy hits, indicating that most novel peptides were false positives. Krug’s work implied that the identification accuracy of novel peptides is greatly affected by the completeness of genome annotation.
In fact, the relationship between annotated and novel peptides is quite similar to that between unmodified and modified peptides. Recently, Fu has formally studied the factors that influence the subgroup FDRs of differently modified peptides (Fu, 2012; Fu and Qian, 2014). Here, we follow the same framework as in Fu’s work to quantitatively investigate the subgroup FDRs of annotated and novel peptides identified by 6-ORF translation search.
2 Methods
To make the relationship in Equation (1) computable, we need to evaluate and , for both annotated and novel peptides. Note that we assume can be readily estimated using some methods, e.g. the commonly used target-decoy search strategy (Elias and Gygi, 2007). For convenience of discussion, we introduce two quantities: (i) θ, the annotation completeness ratio, defined as the length ratio of the currently annotated genes to all genes on the genome; (ii) μ, the annotation length ratio, defined as the length ratio of currently annotated genes to the whole genome. Presuming that only one gene exists at any genomic locus, the value scope of μ is . Actually, μ varies across species even if their genomes are well annotated (for E.coli it is about 0.88 and for Homo sapiens, it is less than 0.02). However, for a poorly annotated genome, μ is relatively small, and we have if no gene is annotated.
Suppose that the annotated and novel peptides could be equally likely retrieved by the search engine and their scores are identically distributed, then apparently, we have and . depends on the sizes of the annotated database and the translated database. Given the genome length L, the summed length of annotated genes is μL. If falsely identified peptides distribute uniformly on the genome, we have and . So far, we have obtained the probabilities that an identified peptide is an annotated or novel peptide given that this identification is true or false and scores better than x, as summarized in Table 1.
Identification type . | True positive . | False positive . |
---|---|---|
Annotated identification | ||
Novel identification |
Identification type . | True positive . | False positive . |
---|---|---|
Annotated identification | ||
Novel identification |
Identification type . | True positive . | False positive . |
---|---|---|
Annotated identification | ||
Novel identification |
Identification type . | True positive . | False positive . |
---|---|---|
Annotated identification | ||
Novel identification |
The value of represents the length proportion of all genes on the genome and is a constant for a certain species. Therefore, we can conclude that depends only on .
If a genome has never been annotated (θ = 0, µ = 0), then no identification could be an annotated peptide and , which is consistent with the assumption. If a genome is completely annotated (θ = 1), then , meaning that all novel peptide identifications are false, which is also consistent with the assumption; in this case, as and µ are usually very small, approximately equals of . If we take , the is only 1.5‰ for E.coli (µ = 0.88) and 0.03‰ for H.sapiens (µ < 0.02), much more conservative than . On the other hand, if we assume , and , then we have and = 9/10. The calculated is 90.1%, as large as 90 times of . In contrast, the is only 1‰. We can see that for well annotated genomes, is overestimated, while is underestimated, if a global FDR is controlled.
Since , we have . It is easy to deduce from Equation (3) that μ, as a parameter quantitatively reflecting the length ratio of the annotated genes to the whole genome, has little influence on . Let us take , then for different θ, the upper and lower bounds (occurring when and , respectively) of can be calculated and are depicted by the dashed blue and solid red lines, respectively, in Figure 1. It is worth emphasizing that is less than 10% if θ does not exceed 90%. However, as θ increases over 90%, ramps up quickly to a much higher level. Thus, we conclude that θ significantly affects . Nevertheless, given , the maximum difference between the upper and lower bounds of is less than 4.6%, and thus μ does not substantially change , just as we deduced above.
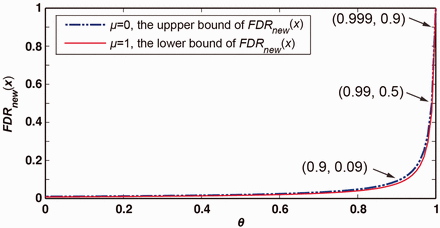
3 Results
By removing some genes from a completely annotated database based on their summed length, we could perform numerical simulation of θ. Then, experimental , which is the ratio of the number of identified annotated decoys to the number of annotated targets, and , which is the ratio of the number of identified novel decoys to the number of novel targets, can be calculated and compared to the theoretical ones [computed by Equations (2) and (3)]. On the basis of this idea, we experimentally validated our theoretical model on two species, i.e. E.coli and Mycobacterium tuberculosis.
3.1 Results on E.coli
Krug et al. (2013) concluded that the E.coli genome might have achieved a complete annotation. We downloaded one of these E.coli’s datasets from PeptideAtlas, which consisted of six raw files (trypsin digested, SAX_01∼SAX_06), 300 032 MS/MS spectra in total. The latest genome sequence and annotation data (NC_000913.3) were downloaded from the NCBI website. First, a stop-to-stop 6-ORF translation was applied to the E.coli’s genome, and a commonly used contaminant database containing porcine trypsin and human keratins was concatenated. The simply reversed form of each protein sequence in the combined database was added, resulting in a combined target-decoy database to facilitate FDR estimation (Elias and Gygi, 2007). Next, the MS/MS spectra were searched against the combined database using pFind studio (version 2.8). Peptides were digested in silico with up to two missed cleavages allowed, and carbamidomethylation on cysteine was set as the fix modification and oxidation on methionine as the variable modification. The precursor and fragment mass error tolerances were set to ± 20 ppm and ± 0.5 Da, respectively. A global peptide-level FDR of 1% was used for quality assessment, which allowed us to identify 12 835 peptides. Among these peptides, 113 were contaminant ones, 12 619 were annotated ones and 103 were novel ones. Since the E.coli’s genome has been almost completely annotated, the 103 novel target identifications should be mostly false ones. Just as expected, among the 128 decoy identifications above the threshold of , 97 were novel decoys, very close to the number of novel targets, indicating that the subgroup FDR of novel peptides was close to 1 (97/103 = 0.94).
To simulate θ, we randomly removed some genes from the annotated database to vary the ratio of their summed length to all genes. Using the same global FDR threshold of 1%, we obtained the numbers of targets and decoys separately on annotated and novel peptides, allowing us to calculate the experimental and . Given θ and μ, the theoretical and were also calculated through Equations (2) and (3). Our simulation showed that, the experimental were close to the theoretical value of 1.5‰, with a minimum of 2.1‰ and a maximum of 3.6‰. Moreover, the experimental fits well with the theoretical counterpart, as shown in Figure 2A. On the other hand, we can deduce the value of θ from the experimental based on Equation (3). As shown in Figure 2, the pairs of sampled and deduced values of θ distribute diagonally, indicating that the deduced θ could be used as an estimate of the real annotation completeness ratio.
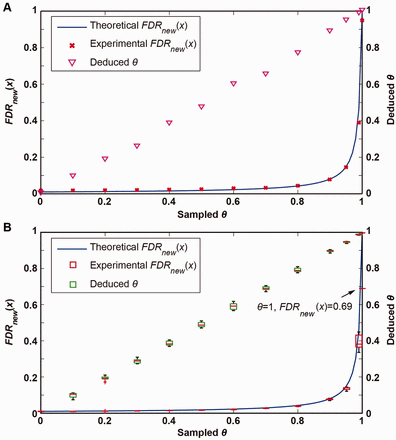
Simulation results on the E.coli and M.tuberculosis datasets. To simulate partial annotation, we randomly removed some annotated genes from the database. Gene sampling was performed on the basis of θ, with a step of 0.1 from 0 to 1, and in addition, 0.95 and 0.99 were also appended. (A) The experimental obtained on the E.coli dataset as shown by red crosses fits well with the theoretical value (blue line). The deduced values for θ were approximately identical to the sampled ones, as shown by magenta triangles on the diagonal line. (B) On the M.tuberculosis dataset, genes were sampled 10 times for each value of θ. The experimental values as shown by red boxes fit well with the theoretical values (blue line) when θ is less than 0.9. As truly novel peptides may exist, the experimental diverges from the theoretical counterpart. The experimental is 0.69 when sampled θ = 1, and the deduced θ is 0.996 correspondingly. However, all deduced values for θ still match the sampled ones (green box), since the annotation completeness ratio is very close to 1.
We also found that if we remove the ‘novel genes’ from the annotated database and search the spectra of novel peptides (identified by 6-ORF translation search) against the partially annotated database, then these spectra will be assigned with false peptides and random scores (see Supplementary Materials S2 and Supplementary Data for more details).
3.2 Results on M.tuberculosis
M.tuberculosis is another organism that has undergone several proteogenomic studies (de Souza et al., 2008; Kelkar et al., 2011).
The annotation length ratio of M.tuberculosis is about 0.91, and its genome is featured by the high GC content. Therefore, we selected M.tuberculosis as the second organism to verify our model. A dataset of 503 933 MS/MS spectra was generated (see Supplementary Method for details of the data) and were searched against the translated genome (NC_018143.2) under the same parameter setting as for E.coli. The search resulted in 28 545 target peptides identified, including 682 contaminant ones, 27 528 annotated ones and 335 novel ones. Meanwhile, a total of 230 novel decoys were obtained above the peptide-level FDR of 1%, leading to an experimental of 0.69 (=230/335) and a deduced θ of 0.996, which was calculated through Equation (3). The disparity between the numbers of novel targets and decoys implies that a set of truly novel peptides may exist in M.tuberculosis. We performed a blast search for the 105 (=335 − 230) top-scoring novel peptides and found that 68 of them have been annotated in other mycobacterial species, meaning that they are conservative and reliable. The rest of them should be experimentally validated through further efforts.
The same simulation experiment of θ as done on E.coli was also tested on M.tuberculosis. The deduced values of θ are in line with the theoretical ones on differently sampled θ, as shown in Figure 2B. The experimental also fits well with the theoretical counterpart for smaller θ. However, the experimental apparently deviates from its theoretical value when θ ≥ 0.95, because of the existence of truly novel peptides. This result shows that M.tuberculosis is not so completely annotated as E.coli, and is sensitive to the incomplete annotation, even when θ approaches 1.
In some previous researches, a filter of protein length is applied to the translated database. To test the influence of this operation on our model, we used four length filters of 30, 50, 100 and 500 to modify the original translated database of M.tuberculosis. The search results upon these four modified databases show that, a small length filter (≤100) causes minor effect on the theoretical estimation of and the deduced θ calculated by Equation (3). However, a large filter such as 500, which removes 80% of annotated genes in M.tuberculosis, greatly shrinks the sample space of novel peptides and biases the estimation. In this situation, Equation (3) should be modified to fit the experimental data consequently (Supplementary Fig. S2). Therefore, to appropriately use the estimation in Equation (3), a suitable length filter (e.g. ≤ 100) is necessary.
4 Discussions
Above, we have assumed that the annotated and novel peptides distribute equally in the expressed and unexpressed proteins. Since not all proteins are expressed in a specific sample under a specific condition, estimation of becomes harder. In fact, novel peptides in nearly completely annotated organisms may have lower expression levels or worse scores, because more abundant and better scoring peptides are easier to annotate, and accordingly, is smaller. This can lead to a larger and hence less credible novel peptide identifications.
The peptide variants resulted from DNA mutation or mRNA alternative splicing are sometimes also considered as novel peptides. However, these variants are much like protein modifications, the sample space of which is difficult to estimate explicitly. Definitely, great care should be taken when reporting these variants, because the search space of these peptides become even larger, and according to Fu’s (2012) corollary, more false positives will appear if a global FDR is used.
In this article, we have revealed that the genome annotation completeness ratio is the dominant factor influencing the identification accuracy of novel peptides identified by 6-ORF translation search when a global FDR is used for quality assessment. The subgroup FDR of novel peptides will be seriously under-estimated if the genome has been well annotated (e.g. when the annotation completeness ratio exceeds 90%). For such well-annotated genomes, separate FDR control is very necessary as recently suggested by Nesvizhskii (2014).
However, with a stringent FDR control (e.g. 1%), many low scoring but true peptide identifications may be excluded along with false positives. To increase the sensitivity and specificity of novel-gene discovery, one should reduce the size of searched database as much as possible (Nesvizhskii, 2014). For example, when the transcriptome information (especially from the strand-specific cDNA-seq data) is available, it is apparently more favorable to search against the transcriptome as well than to search against the genome alone. If the transcriptome information is unavailable, it would be also helpful to reduce the 6-OFR translation database by removing sequences that are predicted to be hardly possible to be real proteins. From the point of view of data analysis, we believe that intelligent post-processing of search results is a promising resolution to sensitive discovery of novel peptides and genes. For example, machine learning techniques (Kall et al., 2007) can be used to discriminate true and false identifications of novel peptides and to re-score and re-rank the peptides using specific information in proteogenomics. Alternatively, the search results from multiple search engines can be combined properly to generate a set of reliable identifications (Kelkar et al., 2011).
Funding
This work was supported by the International S&T Cooperation Program of China (2014DFB30010), the Strategic Priority Research Program of Chinese Academy of Sciences (XDB13040600), the National Key Basic Research Program of China (2011CB910600, 2013CB911201, 2010CB912701, 2015CB554406), the National Natural Science Foundation of China (31170780, 31470809, 31400698), the National High-Tech Research and Development Program of China (SS2012AA020502, 2011AA02A114), the International Collaboration Program (2014DFB30020), the National Megaprojects for Key Infectious Diseases (2013zx10003002), and the NCMIS CAS.
Conflict of Interest: none declared.
References
Author notes
Associate Editor: Burkhard Rost