-
PDF
- Split View
-
Views
-
Cite
Cite
Götz Fabian, Thomas Wächter, Michael Schroeder, Extending ontologies by finding siblings using set expansion techniques, Bioinformatics, Volume 28, Issue 12, June 2012, Pages i292–i300, https://doi.org/10.1093/bioinformatics/bts215
- Share Icon Share
Abstract
Motivation: Ontologies are an everyday tool in biomedicine to capture and represent knowledge. However, many ontologies lack a high degree of coverage in their domain and need to improve their overall quality and maturity. Automatically extending sets of existing terms will enable ontology engineers to systematically improve text-based ontologies level by level.
Results: We developed an approach to extend ontologies by discovering new terms which are in a sibling relationship to existing terms of an ontology. For this purpose, we combined two approaches which retrieve new terms from the web. The first approach extracts siblings by exploiting the structure of HTML documents, whereas the second approach uses text mining techniques to extract siblings from unstructured text. Our evaluation against MeSH (Medical Subject Headings) shows that our method for sibling discovery is able to suggest first-class ontology terms and can be used as an initial step towards assessing the completeness of ontologies. The evaluation yields a recall of 80% at a precision of 61% where the two independent approaches are complementing each other. For MeSH in particular, we show that it can be considered complete in its medical focus area. We integrated the work into DOG4DAG, an ontology generation plugin for the editors OBO-Edit and Protégé, making it the first plugin that supports sibling discovery on-the-fly.
Availability: Sibling discovery for ontology is available as part of DOG4DAG (www.biotec.tu-dresden.de/research/schroeder/dog4dag) for both Protégé 4.1 and OBO-Edit 2.1.
Contact: [email protected]; [email protected]
Supplementary information: Supplementary data are available at Bioinformatics online.
1 INTRODUCTION
During the last decade, the field of biomedicine has seen a data explosion, made evident by the overwhelming number of published articles, databases, nucleotide sequences and protein structures (Howe et al., 2008). Today, ontologies are used extensively in the biomedical and healthcare sector for information and data integration, such as gene product annotation (Ashburner et al., 2000), analysis of high-throughput data (Whetzel et al., 2006) and searching (Doms and Schroeder, 2005). If an ontology cannot maintain a high degree of coverage in its domain, its correctness and integrity will suffer, leading to missing results when trying to find documents or genes semantically associated with terms (Liu et al., 2011). However, to keep up with new information, ontologies must be revised and newly added terms need to be enriched with definitions, cross-references and additional properties. Since ontologies are manually curated, developing and maintaining them is often a slow, tedious and error-prone process. To mitigate this bottleneck, text mining and related techniques can be employed to enrich ontologies in a semi-automated fashion. Among the variety of ontology learning methods proposed in the past, mainly term recognition and pattern-based relationship extraction methods are used in the biomedical field (Liu et al., 2011).
In this article, we present an alternative approach to enhancing ontologies by automatically finding suitable co-hyponyms of terms, i.e. finding terms which are in a sibling relationship to each other. This approach can be used to extend ontologies in a horizontal way and therefore to complete a set of terms. For instance, an ontology that already includes the terms somatotrophs and trophoblasts (which are both endocrine cells) could be extended by automatically proposing more terms with the same parent term (Fig. 1). With this approach, ontology engineers can semi-automatically extend ontologies using two to three terms, which are the ‘seed terms’ for the algorithm. Many existing ontologies can be expanded in this way with minimal effort.
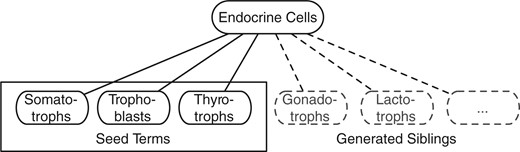
Sibling discovery example: Somatotrophs, Trophoblasts, and Thyrotrophs are known child terms of Endocrine Cells in MeSH, other children such as Gonadotrophs, Lactotrophs can be automatically found using our approach
Our method extracts siblings of existing terms on-the-fly using web sites returned by queries to search engines, thus implicitly incorporating full-text journal articles, patents, text books, wiki pages, etc. as indexed by the engines. In our methodology, we are integrating two approaches, which, when combined efficiently, improve the quality of the proposed siblings in terms of precision and recall.
Structure-based approach: The first approach extracts siblings from the structure of web sites. It is based on the observation that terms, which are in a sibling relationship to each other, are often located together in tables, lists or headings. If seed terms are found in such elements, the remaining content of those elements has a high probability of being semantically related to the seed terms. For instance, Figure 2 shows an excerpt of the Wikipedia page on the endocrine system. When given Somatotrophs and Gonadotrophs (both endocrine cells) as seed terms, a third (possible) endocrine cell, Corticotrophs, can be extracted. We do this by exploiting the structure of HTML documents, which are prevalent on the web.
![Excerpt of HTML code from the Wikipedia page on the endocrine system [http://en.wikipedia.org/wiki/Endocrine_system]](https://oup.silverchair-cdn.com/oup/backfile/Content_public/Journal/bioinformatics/28/12/10.1093_bioinformatics_bts215/3/m_bioinformatics_28_12_i292_f2.jpeg?Expires=1750192428&Signature=XF4zc5sNXZLaBo09zJmmAChHrkFyveQ~fQJkdigKU2A~1ysizdcLBUCwczb-HMt4XaYtxjM0FejKEpaGFfkh2aO-u-PlcEYaljJyJFDolczG6p~ws2WPGtqaxDqUaEpbTlL39BBGtM1Co3923SD6fMlX28WU4yasFhU19Kh3kYmioGmr-jmBQk9WQXSgqbIKsGIdQne2rrU~VKBfl9WevGoPsXiKqHcGntxxCvB~zsBoCGM5UdBWOHTAcS2-uZ5YZ9C03kiZDZkJLcJwIXlpj1y2zm0eRLuhBXjt8NWA30RUo5UD6B0hw1CQq8ELdQ85X-0cDoQztcqXWMSeKA9NuA__&Key-Pair-Id=APKAIE5G5CRDK6RD3PGA)
Excerpt of HTML code from the Wikipedia page on the endocrine system [http://en.wikipedia.org/wiki/Endocrine_system]
Text-based approach: The second approach finds candidate siblings from text by extracting them from enumerations in sentences. For instance, the following sentence contains an enumeration of endocrine cells: ‘…several adenohypophysial endocrine cells such as somatotrophs, thyrotrophs, and gonadotrophs’ [http://www.ncbi.nlm.nih.gov/pubmed/11478270]. In this sentence, the enumerated terms are somatotrophs, thyrotrophs and gonadotrophs, which are all adenohypophysial endocrine cells. These enumerations occur in many forms, but often have reoccurring patterns, which we can exploit. We can extract terms with great accuracy using morpho-syntactic pattern matching, meaning we analyze the pattern of the sentence and its enumerated terms and extract them subsequently. Further examples of extracted enumerations from sentences can be found in Table 1 and Supplementary Table S1.
Topic . | Seed terms (from MeSH) . | Extracted snippet . | Discovered terms . | Website . |
---|---|---|---|---|
Particles | Heavy Ions, Neutrons, Protons | A particle, such as an electron, proton, or neutron, having… | Electron | answers.com |
GnRH | Goserelin, Nafarelink, Buserelin | GnRH agonist analogues such as buserelin, goserelin, lupron, and decapeptyl inhibit the action… | Lupron, decapeptyl | ncbi.nlm.nih.gov |
Berberideae species | Mahonia, Caulophyllum, Epimedium | …only included four genera (Berberis, Epimedium, Mahonia,Vancouveria), with the other… | Berberis, Vancouveria | righthealth.com |
Bacillus species | Bacillus cereus, Bacillus megaterium, Bacillus subtilis | Microorganisms of the Bacillus species include Bacillus cereus, Bacillus mycoides, Bacillus subtilis, Bacillus anthracis, and Bacillus thuringiensis. | Bacillus mycoides, Bacillus anthracis, Bacillus thuringiensis | freepatentsonline.com |
European countries | Netherlands, Finland, Austria | …Shakira's most successful song in Europe, where it topped many of the medium sized charts, including Austria, Denmark, Finland, Norway and Sweden. | Denmark, Norway, Sweden | en.wikipedia.org |
Topic . | Seed terms (from MeSH) . | Extracted snippet . | Discovered terms . | Website . |
---|---|---|---|---|
Particles | Heavy Ions, Neutrons, Protons | A particle, such as an electron, proton, or neutron, having… | Electron | answers.com |
GnRH | Goserelin, Nafarelink, Buserelin | GnRH agonist analogues such as buserelin, goserelin, lupron, and decapeptyl inhibit the action… | Lupron, decapeptyl | ncbi.nlm.nih.gov |
Berberideae species | Mahonia, Caulophyllum, Epimedium | …only included four genera (Berberis, Epimedium, Mahonia,Vancouveria), with the other… | Berberis, Vancouveria | righthealth.com |
Bacillus species | Bacillus cereus, Bacillus megaterium, Bacillus subtilis | Microorganisms of the Bacillus species include Bacillus cereus, Bacillus mycoides, Bacillus subtilis, Bacillus anthracis, and Bacillus thuringiensis. | Bacillus mycoides, Bacillus anthracis, Bacillus thuringiensis | freepatentsonline.com |
European countries | Netherlands, Finland, Austria | …Shakira's most successful song in Europe, where it topped many of the medium sized charts, including Austria, Denmark, Finland, Norway and Sweden. | Denmark, Norway, Sweden | en.wikipedia.org |
Seed terms and discovered terms are printed bold in the extracted snippet.
Topic . | Seed terms (from MeSH) . | Extracted snippet . | Discovered terms . | Website . |
---|---|---|---|---|
Particles | Heavy Ions, Neutrons, Protons | A particle, such as an electron, proton, or neutron, having… | Electron | answers.com |
GnRH | Goserelin, Nafarelink, Buserelin | GnRH agonist analogues such as buserelin, goserelin, lupron, and decapeptyl inhibit the action… | Lupron, decapeptyl | ncbi.nlm.nih.gov |
Berberideae species | Mahonia, Caulophyllum, Epimedium | …only included four genera (Berberis, Epimedium, Mahonia,Vancouveria), with the other… | Berberis, Vancouveria | righthealth.com |
Bacillus species | Bacillus cereus, Bacillus megaterium, Bacillus subtilis | Microorganisms of the Bacillus species include Bacillus cereus, Bacillus mycoides, Bacillus subtilis, Bacillus anthracis, and Bacillus thuringiensis. | Bacillus mycoides, Bacillus anthracis, Bacillus thuringiensis | freepatentsonline.com |
European countries | Netherlands, Finland, Austria | …Shakira's most successful song in Europe, where it topped many of the medium sized charts, including Austria, Denmark, Finland, Norway and Sweden. | Denmark, Norway, Sweden | en.wikipedia.org |
Topic . | Seed terms (from MeSH) . | Extracted snippet . | Discovered terms . | Website . |
---|---|---|---|---|
Particles | Heavy Ions, Neutrons, Protons | A particle, such as an electron, proton, or neutron, having… | Electron | answers.com |
GnRH | Goserelin, Nafarelink, Buserelin | GnRH agonist analogues such as buserelin, goserelin, lupron, and decapeptyl inhibit the action… | Lupron, decapeptyl | ncbi.nlm.nih.gov |
Berberideae species | Mahonia, Caulophyllum, Epimedium | …only included four genera (Berberis, Epimedium, Mahonia,Vancouveria), with the other… | Berberis, Vancouveria | righthealth.com |
Bacillus species | Bacillus cereus, Bacillus megaterium, Bacillus subtilis | Microorganisms of the Bacillus species include Bacillus cereus, Bacillus mycoides, Bacillus subtilis, Bacillus anthracis, and Bacillus thuringiensis. | Bacillus mycoides, Bacillus anthracis, Bacillus thuringiensis | freepatentsonline.com |
European countries | Netherlands, Finland, Austria | …Shakira's most successful song in Europe, where it topped many of the medium sized charts, including Austria, Denmark, Finland, Norway and Sweden. | Denmark, Norway, Sweden | en.wikipedia.org |
Seed terms and discovered terms are printed bold in the extracted snippet.
Finally, the results of both approaches are combined to obtain a single ranked list of terms.
Generating siblings from seed terms is done in an interactive manner and only takes a few seconds. We optimized our method for biomedical ontologies by adapting both approaches to the peculiarities of biomedical terminology. Nonetheless, the method is suitable for ontologies of other domains. Since this work has been integrated as part of the DOG4DAG plugin (Wächter and Schroeder, 2010) into OBO-Edit and now also into Protégé, ontologies for both the OBO and the OWL format can be enriched seamlessly using sibling discovery.
The rest of this article is organized as follows. First, we compare our method to previous work in sibling generation. Next, we describe the approach and evaluate it using MeSH (Medical Subject Headings), a widely used ontology, as well as the Text REtrieval Conference Entity List Completion (TREC ELC) task. Furthermore, we show how the DOG4DAG plugin can be leveraged to extend ontologies using sibling generation. Finally, we discuss our approach and the results and propose future work.
2 RELATED WORK
The domain of ontology learning, including many approaches employing the web as a corpus, is a field of intensive research. Sibling generation using set expansion has been discussed in a number of studies which include approaches exploiting textual patterns, the HTML structure of web pages and distributional similarity (DS) of terms.
A number of text-based approaches incorporate Hearst patterns (1992) to find parent--child relationships in free text using lexico-syntactic pattern matching. Sibling generation is included in KnowItAll (Etzioni et al., 2005), a generic information extraction engine for unsupervised named-entity extraction. Using search results from the web, facts, terms and relations are extracted using bootstrapped patterns. Shi et al. (2008) and Zhang et al. (2009) also find siblings with sentence patterns and predefined HTML tag patterns. However, our system works on arbitrary tags and is not restricted to specific tags for lists or tables. Paşca (2004) retrieves siblings from the web in an unsupervised manner using pattern learning and part-of-speech (POS) and noun phrase (NP) tagging. Candidate siblings are ranked based on co-occurrence frequency. Also, Kozareva et al. (2008) built a pattern-based system for learning specific semantic classes (e.g. countries or singers). Contrary to our approach, they only used one highly specific surface pattern and did not incorporate NP chunking to correctly separate NPs from each other.
Several systems have also been developed for a structure-based approach. The systems SEAL (Wang and Cohen, 2007) and XTREEM (Brunzel and Spiliopoulou, 2006) both exploit semi-structured HTML documents to expand sets using a number of given seed terms. Wang and Cohen presented SEAL, a system which expands seeds by querying search engines and automatically inducing wrappers for each web page. In XTREEM, semantic sibling associations are extracted from web pages by grouping paths in DOM trees which include seed terms. However, their system does not return a ranked list of candidate siblings, but rather sets of sibling clusters. KnowItAll was also extended to include a ‘List Extractor’ component which extracts facts by exploiting the HTML structure of web pages. Shinzato et al. (2004) also extract siblings from HTML documents and rank the candidate siblings using cosine similarity.
Other approaches exploit DS to find siblings in text by looking at the context of each term. For instance, Lin et al. (2001) generate sibling sets with an unsupervised algorithm on a newspaper corpus and on MEDLINE abstracts. Similarly, Pantel et al. (2009) expand sets of terms by DS in a semi-supervised approach using seed items for each set. In general, DS approaches generally yield lower performance than pattern-based approaches when extracting proper nouns (Shi et al., 2010).
None of the set expansion methods have effectively combined both approaches for on-the-fly sibling discovery. Furthermore, the presented systems usually do not have any background knowledge in form of an ontology and only take a number of seed terms as an input. In contrast, our method also takes the parent term and lexical variants such as synonyms and abbreviations into account. Additionally, we optimized our method for the peculiarities of biomedical terminology and ontologies.
In terms of integrating ontology learning tools into editors such as OBO-Edit (Day-Richter et al., 2007) or Protégé [http://protege.stanford.edu], two plugins currently exist: DOG4DAG (Wächter and Schroeder, 2010) and TerMine (Frantzi et al., 2000). We extended our plugin DOG4DAG to become the first integrated tool that supports sibling generation so far.
3 METHODS
In this section, we present our 2-fold approach to sibling generation from a given set of seed terms using the web as a corpus. The whole pipeline is summarized in Figure 3.
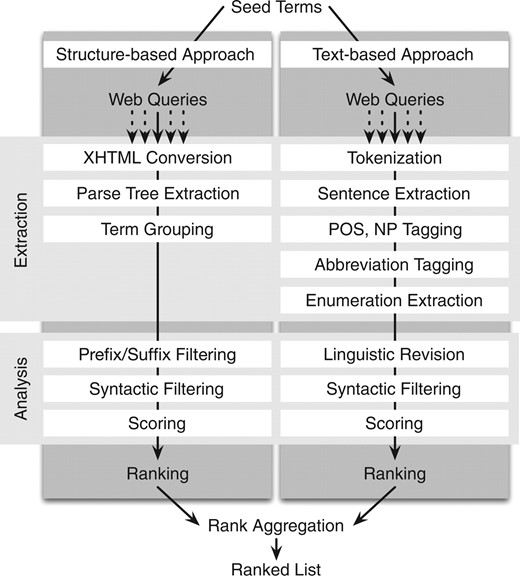
Overview of the sibling generation pipeline. Using seed terms, candidate siblings are generated, which are then aggregated into a final candidate sibling list
To complete an existing set of terms with an identical parent, a subset of these terms is selected as seed terms. The parent term and the already existing siblings are also added to the input as well. In addition to the label of the term, its lexical variants (synonyms, abbreviations, etc.) are included in the query and used for ranking if available. Using these seed terms, we query search engines and use the results to retrieve candidate siblings.
3.1 Structure-based approach
In the first approach, search engines are queried for web sites containing the seed terms. After downloading the web pages, their parse trees are generated, and candidate siblings are extracted by finding paths identical to those of the seed terms.
Query engines: Query search engines (currently Yahoo! and Bing/MSN Live) with the selected seed terms and retrieve the search results. The queries are constructed by concatenating seed terms (in quotation marks) by the AND operator, thus ensuring that both terms occur in the web page. If more than two seed terms are used, query all pairwise combinations. At present, both search engines return 50 search results for every query.
Download pages: Download the web pages of the search results, parse and convert them to valid XHTML documents using HTMLCleaner [http://htmlcleaner.sourceforge.net/]. This step is required because many web pages contain invalid syntax, e.g. missing closing tags. The result of this cleaning process is a parse tree. We can represent the structure of a web page in such a parse tree, whose nodes contain the tags (such as <td>) and their textual contents.
Traverse parse tree: Traverse the parse tree in a depth-first search and find nodes whose text contains a seed term. Build the paths from the root node to the nodes containing the term (e.g. <html> … <table><tr><td>) if the seed term was found inside a table data cell). Extract the terms from nodes which have the same parent node inside the parse tree and the identical HTML tag as the seed term node.
Group candidates: Group all extracted candidate siblings into a candidate sibling set. If the seed term is preceded or followed by a string (such as ‘function of seed term’) in the text, all candidate siblings are also required to include this string.
3.2 Text-based approach
The second approach uses textual patterns to extract candidate siblings from enumerations in text. By querying search engines, we retrieve text snippets on-the-fly, from which siblings are extracted, filtered and ranked.
Pattern extraction and expansion: We built a small, manually annotated corpus containing sentences with typical enumerations. Whenever a sentence is added to the corpus, the annotated sentences are preprocessed automatically. From each sentence, head terms, enumeration items and words in between are extracted. To form the basis for patterns, head terms and enumerations are replaced with placeholders and the surrounding text is removed. These sentences are expanded and altered to allow for more variation. For instance, commas are added after introductory phrases and conjunctions are changed (e.g. ‘and’ is replaced with ‘or’). From these patterns, regular expressions are created automatically, which are used to match sentences and extract enumeration items and the head term. To add a new type of enumeration, one can simply add the new sentence to the corpus, which in turn leads to new generalized patterns. The generated regular expressions are stored on disk and are loaded for sibling generation.
Web search: Like the structure-based approach, web search is used to retrieve snippets. In the queries, the introductory phrase is included to find relevant results. Additionally, the NEAR operator of the search engine is used to force the seed terms to appear close to each other, which in most cases means in the same sentence. We do not retrieve the whole website, but instead use snippets (usually 300 characters long) provided by the search engines containing the search terms, and thus the enumeration. Again, pairwise combinations are used for the web queries.
Text processing and enumeration extraction: The retrieved snippets are first tokenized and then processed using sentence, POS, and NP tagging. For POS tagging, the LingPipe Tagger [http://alias-i.com/lingpipe/] trained on the MEDLINE corpus is used. Phrases of the pattern [adj|verb]*[fill]{2}[noun]+ are regarded as NPs (fill are words like ‘of’, ‘the’, ‘for’, etc.). Furthermore, abbreviations are extracted by checking if a candidate term contains a short form after the long form in brackets. If both forms match, the short form (i.e. the abbreviation) is grouped with the long form. The sentences are now matched against the regular expressions of the pipeline (‘morphosyntactic matching’). If a sentence contains multiple enumerations, all enumerations are extracted separately. To find as many enumerations as possible, three sets of regular expressions are used for matching and finding enumerations.
The first set consists of regular expressions including the head term, the introductory phrase, the enumeration items and a conjunction to separate the last two items (this conjunction does not exist if the phrase located is at the end). The search results are matched against the regular expressions. If a match occurs, the enumerated items are extracted and subsequently analyzed. If a seed term occurs among the extracted items, the remaining items become a candidate sibling set. If a snippet does not match a regular expression of this set, the next set is used. It matches all sentences which include the head term, introductory phrase and enumeration items. The last set matches all enumerations which include a conjunction at the end, but do not have an introductory phrase. Note that each set is more generic than the previous one.
Finally, all extracted terms are matched by checking if they are NPs (‘linguistic revision’). This is especially important for the last phrase (after the conjunction) where it is not possible to determine the end of the phrase reliably without the NP tagger.
In addition, enumerations in sentences without any introductory phrases, conjunctions or head terms are also retrieved. For this, the search engines are queried using only the seed terms concatenated by the NEAR operator. The separators between the phrases are automatically recognized and all enumerated items are subsequently extracted.
3.3 Syntactic filtering
To improve the accuracy of the extracted candidate siblings, a number of syntactic filter steps are used. We set a minimum length of 3 and a maximum length of 50 characters for each generated candidate sibling. By using a minimum length of 3 characters, gene and protein family names like ‘p53’ or ‘WNT’ are still regarded as valid items. By limiting the length to 50 characters, we can exclude any spurious NPs. In addition, we use a stop-word list to remove unnecessary words like ‘other’, ‘many more’ or ‘etc’ from the extracted siblings. Additionally, all candidate sibling sets containing less than three terms (including the seed terms) are dropped.
Duplicated candidate sibling sets (with the same siblings) are automatically discarded, since they are most likely retrieved from identical web pages.
3.4 Ranking
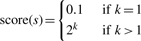
Thus, our system rewards siblings sets which contain a larger amount of seed terms and gives a low score (0.1) to sibling sets with only one seed term, since this co-occurrence may be coincidental. If a candidate sibling occurs in multiple candidate sibling sets, the scores are added up to yield a score for each candidate sibling. If a lexical variant such as a synonym or abbreviation of a seed term occurs in the candidate sibling set, it is also counted as a seed term.
In addition, the retrieved candidate siblings are re-ranked by the following measures:
Hypernym matching: For the text-based approach, we identify the head term of the enumeration. If this term matches the hypernym of the seed terms (i.e. their parent term), it is preferred. Since the head term in the text almost always occurs in plural, we stem the extracted head terms first.
Compound term matching [as proposed by Ogren et al. (2004)]: Biomedical terminology often consists of multiple compound terms, e.g. subterms of the MeSH term Stem Cells include Adult Stem CellsHematopoietic Stem Cells and Mesenchymal Stem Cells. We prefer the candidate siblings whose parent term is a suffix of this sibling.
Combining of ranked lists of methods: We examined several methods for rank aggregation of both methods. In our evaluation, summing up the normalized scores of the candidate siblings with identical labels and merging both lists by sorting them by their normalized scores yielded the best results. Previously, other ranking methods have been evaluated (Wang and Cohen, 2007, Brunzel and Spiliopoulou, 2006) and shown to have no significant impact on the overall results.
3.5 Evaluation
To evaluate our method, we used the 2011 MeSH [http://www.nlm.nih.gov/mesh]. For this purpose, we randomly took a sample of 1000 terms in MeSH and chose three random child terms as seed terms for each set. For the selection of the parent term, we required them to have at least five child terms so the system is able to find potentially at least two siblings if three of the child terms are used as seed terms. Additionally, all child terms which consist of more than two words were not used as seed terms since they rarely occur in free text. Terms with artificial descriptor names (e.g. ‘Surgical Procedures, Operative’) were cleaned up. The 16 top-level categories were also ignored, since the terms are not semantically related, but are rather categorical. We batch-processed all 1000 term sets automatically using the implemented system.
We selected MeSH because it is a thesaurus with a broad coverage (it comprises 26 142 terms and 203 554 lexical variants). Although most of its terms are from the biomedical domain, it also contains terms from other domains, for example in the top-level categories Geographicals or Humanities.
4 RESULTS
4.1 MeSH evaluation
Recall: First, we evaluated how many of the remaining siblings were found. Of the 7922 siblings which were contained in the sets (not counting the seed terms), 6284 (79.3%) were discovered when using both approaches. For 601 of the 1000 selected sets, all siblings were discovered (Fig. 4). Hence, our approach can find most of the existing siblings of the selected sibling sets. The results from both approaches have an overlap of 72.5%. Of the correct results, 35.0% were contributed exclusively by the structure-based approach, and 22.6% were contributed exclusively by the text-based approach. This shows that our idea of combining both approaches is reasonable and improves the overall results.

Distribution of the recall of discovered siblings in all results using both approaches. For 601 of the 1000 sets, all siblings were found resulting in an average recall of 79.3 overall

We used a cut-off rank of 10 when evaluating precision (if the sibling set contains <10 siblings, we used the number of siblings in the set). Over the 1000 selected seed terms, the average precision using the structure-based approach is 53.0%, whereas the average precision for the text-based approach is 48.0%. When combining the two approaches using rank aggregation, the precision is 60.8%. The results show that rank aggregation improves precision when compared with the single approaches (Fig. 5).
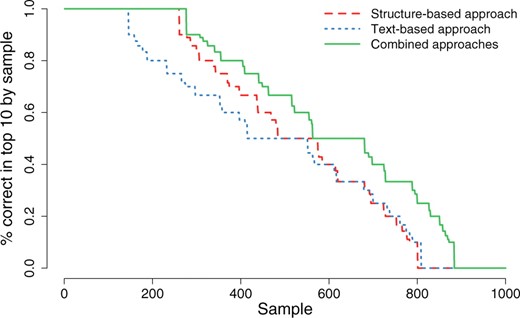
Distribution of the precision of the siblings found from the 1000 randomly selected MeSH terms in the Top 10. Half of the tested sets were automatically extended with a precison of >75%. The structure-based approach has shown a higher precision than the text-based approach
Since recall and precision do not take the ranking of the generated siblings into account, we also examined the percentage of correct siblings for each position within the top 10 (Fig. 6). Overall, the structure-based approach yields better results than the text-based approach. Combining the results improves the performance compared with the single approaches. In the highest ranked result, 76.6% are correct using rank aggregation, whereas 64.7% and 66.4% are correct for the structure-based and text-based approach, respectively. Thus, generated siblings which are true siblings in MeSH are ranked higher than siblings not belonging to the sibling sets of our MeSH evaluation. If the descendants of the siblings are also taken into account, the percentage of correctly generated siblings increases to 81.3% for the top ranked result (70.8% and 69.5% for the structure-based and text-based approach, respectively).
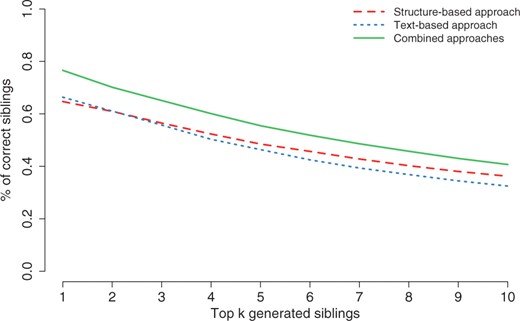
Percentage of correctly generated siblings in the top 10. In general, ontologies can be extended using the top generated terms with high confidence. For the top 1 terms, 76.6% are generated correctly
Table 2 shows three examples of generated siblings with varying recall and precision. Lipids has an overall recall of 100% and a precision of 90%. Europe contains 22 siblings, of which 15 were found (68% recall). However, it only has a precision of 70% within the top 10. When looking closer at the results, all of the top 10 terms are correct, although some are actually a child term of a sibling. An example of a term where only 4 of 24 siblings were found (16.66 recall) is Environment, which contains many generic terms (e.g. Confined Spaces or Ecosystem).
Parent term . | Lipids . | Europe . | Environment . | |||
---|---|---|---|---|---|---|
Seed terms . | Sphingolipids, Lipoproteins, Lipopeptides . | Netherlands, Finland, Austria . | Fires, Greenhouse Effect, Water Movements . | |||
Rank . | Generated term . | Relation . | Generated term . | Relation . | Generated term . | Relation . |
1 | Waxes | Sibling | Belgium | Sibling | Pollution | Not in MeSH |
2 | Sterols | Sibling | Denmark | Child | Environmental Monitoring | Unrelated |
3 | Lipopolysaccharides | Sibling | France | Sibling | Water Vapour | Sibling |
4 | Phospholipids | Sibling | Sweden | Child | Mars | Unrelated |
5 | Glycolipids | Sibling | Norway | Child | Deposition | Not in MeSH |
6 | Fatty acids | Sibling | Italy | Sibling | Air | Child |
7 | Oils | Sibling | Switzerland | Sibling | Oxygen | Unrelated |
8 | Peptidoglycans | Sibling | Spain | Sibling | GDP | Not in MeSH |
9 | Lipofuscin | Sibling | Ireland | Sibling | Ozone | Unrelated |
10 | Membrane Lipids | Sibling | Hungary | Child | Clouds | Not in MeSH |
Parent term . | Lipids . | Europe . | Environment . | |||
---|---|---|---|---|---|---|
Seed terms . | Sphingolipids, Lipoproteins, Lipopeptides . | Netherlands, Finland, Austria . | Fires, Greenhouse Effect, Water Movements . | |||
Rank . | Generated term . | Relation . | Generated term . | Relation . | Generated term . | Relation . |
1 | Waxes | Sibling | Belgium | Sibling | Pollution | Not in MeSH |
2 | Sterols | Sibling | Denmark | Child | Environmental Monitoring | Unrelated |
3 | Lipopolysaccharides | Sibling | France | Sibling | Water Vapour | Sibling |
4 | Phospholipids | Sibling | Sweden | Child | Mars | Unrelated |
5 | Glycolipids | Sibling | Norway | Child | Deposition | Not in MeSH |
6 | Fatty acids | Sibling | Italy | Sibling | Air | Child |
7 | Oils | Sibling | Switzerland | Sibling | Oxygen | Unrelated |
8 | Peptidoglycans | Sibling | Spain | Sibling | GDP | Not in MeSH |
9 | Lipofuscin | Sibling | Ireland | Sibling | Ozone | Unrelated |
10 | Membrane Lipids | Sibling | Hungary | Child | Clouds | Not in MeSH |
True siblings in MeSH are printed in bold. The relation of the generated siblings is given relative to the location of the seed terms in MeSH. The relation ‘Unrelated’ means that the generated term is neither a sibling nor a child of a sibling, but occurs elsewhere in MeSH.
Parent term . | Lipids . | Europe . | Environment . | |||
---|---|---|---|---|---|---|
Seed terms . | Sphingolipids, Lipoproteins, Lipopeptides . | Netherlands, Finland, Austria . | Fires, Greenhouse Effect, Water Movements . | |||
Rank . | Generated term . | Relation . | Generated term . | Relation . | Generated term . | Relation . |
1 | Waxes | Sibling | Belgium | Sibling | Pollution | Not in MeSH |
2 | Sterols | Sibling | Denmark | Child | Environmental Monitoring | Unrelated |
3 | Lipopolysaccharides | Sibling | France | Sibling | Water Vapour | Sibling |
4 | Phospholipids | Sibling | Sweden | Child | Mars | Unrelated |
5 | Glycolipids | Sibling | Norway | Child | Deposition | Not in MeSH |
6 | Fatty acids | Sibling | Italy | Sibling | Air | Child |
7 | Oils | Sibling | Switzerland | Sibling | Oxygen | Unrelated |
8 | Peptidoglycans | Sibling | Spain | Sibling | GDP | Not in MeSH |
9 | Lipofuscin | Sibling | Ireland | Sibling | Ozone | Unrelated |
10 | Membrane Lipids | Sibling | Hungary | Child | Clouds | Not in MeSH |
Parent term . | Lipids . | Europe . | Environment . | |||
---|---|---|---|---|---|---|
Seed terms . | Sphingolipids, Lipoproteins, Lipopeptides . | Netherlands, Finland, Austria . | Fires, Greenhouse Effect, Water Movements . | |||
Rank . | Generated term . | Relation . | Generated term . | Relation . | Generated term . | Relation . |
1 | Waxes | Sibling | Belgium | Sibling | Pollution | Not in MeSH |
2 | Sterols | Sibling | Denmark | Child | Environmental Monitoring | Unrelated |
3 | Lipopolysaccharides | Sibling | France | Sibling | Water Vapour | Sibling |
4 | Phospholipids | Sibling | Sweden | Child | Mars | Unrelated |
5 | Glycolipids | Sibling | Norway | Child | Deposition | Not in MeSH |
6 | Fatty acids | Sibling | Italy | Sibling | Air | Child |
7 | Oils | Sibling | Switzerland | Sibling | Oxygen | Unrelated |
8 | Peptidoglycans | Sibling | Spain | Sibling | GDP | Not in MeSH |
9 | Lipofuscin | Sibling | Ireland | Sibling | Ozone | Unrelated |
10 | Membrane Lipids | Sibling | Hungary | Child | Clouds | Not in MeSH |
True siblings in MeSH are printed in bold. The relation of the generated siblings is given relative to the location of the seed terms in MeSH. The relation ‘Unrelated’ means that the generated term is neither a sibling nor a child of a sibling, but occurs elsewhere in MeSH.
Number of seed terms: The presented algorithm can use any number of seed terms as an input. However, to attain satisfactory results, a reasonable number of seed terms should be between two and four. If only one seed term is used, the algorithm may find candidate siblings which fit another meaning of the input than the intended one. If five or more seed terms are used, the execution time is too long, since the number of queries grows quadratically due to querying all pairwise combinations of the seed terms. We also evaluated the same 1000 randomly selected sets with one and two seed terms. When using two seed terms, recall decreases from 79.3% to 68.2% and precision from 60.8% to 51.5% (compared with three seed terms). When only one seed term is used, recall and precision drop to 25.2% and 15.3%, respectively. This shows that using only two seed terms produces satisfactory results, which can still support ontology engineers in ontology extension.
Overfitting: One issue we had to deal with are websites listing MeSH terms, yielding a very high precision and recall. However, we decided to retain these websites in the results, since they rarely occur and are difficult to filter out correctly.
Siblings in abstracts and full-text articles: We evaluated the number of siblings extracted from snippets found in MEDLINE abstracts and PubMed Central full-text articles, if one of them was part of the web search results. While only 59 abstracts contained siblings in our evaluation, 117 full-text articles contained enumerations which were used to generate siblings. This is especially noteworthy since MEDLINE contains 19 million abstracts [http://www.nlm.nih.gov/pubs/factsheets/medline.html], whereas PubMed Central only contains 2.3 million full-text articles. This shows that for text mining in biomedical literature, full-text articles, patent information and website contents should always be taken into consideration, and can sometimes even be a more informative resource than just MEDLINE abstracts.
4.2 TREC ELC task
Since 2010, the TREC has included a task with a similar goal as part of the Entity track: Entity List Completion (ELC) (Balog et al., 2011). The task contains eight topics, each including a description of the task and a list of examples. This list should be expanded by finding entities from a given set which are in a sibling relationship to the examples. Finally, results have to be mapped to a given set of URIs. We skipped the last step since this was not in the scope of our work and should in general not decrease recall and precision. As a corpus, the English portion of the ClueWeb09 dataset, comprising ~500 million webpages, was used in the ELC task.
To test whether our system is capable of finding siblings from the topics, we performed a simple experiment. From the provided examples of each topic, we picked three, generated siblings from them using our approach, and checked whether the results correspond to the results in the provided sets (Table 3 and Supplementary Table S2). All topics except one are not taken from the biomedical domain. The evaluation shows that our method can find the majority of the correct siblings (R-precision: 55.3%) and is capable of finding almost all siblings (Recall: 86.7%). This shows that our system can generate siblings in any domain and is thus universal. Compared with the other contestants of the ELC task, R-precision was 24.1% better than the best result (recall was not measured), although our evaluation method differs in some points from the ELC task.
Domain . | Siblings . | Recall (%) . | R-precision (%) . |
---|---|---|---|
Professional sports teams | 8 | 75.0 | 62.5 |
Pharmaceutical products | 1 | 100.0 | 100.0 |
Airlines | 45 | 84.4 | 24.4 |
Companies | 10 | 60.0 | 20.0 |
Airlines II | 27 | 96.2 | 40.7 |
Universities | 10 | 100.0 | 70.0 |
Television Chefs | 40 | 77.5 | 45.0 |
Whisky distilleries | 5 | 100.0 | 80.0 |
Average | 18.25 | 86.7 | 55.3 |
Domain . | Siblings . | Recall (%) . | R-precision (%) . |
---|---|---|---|
Professional sports teams | 8 | 75.0 | 62.5 |
Pharmaceutical products | 1 | 100.0 | 100.0 |
Airlines | 45 | 84.4 | 24.4 |
Companies | 10 | 60.0 | 20.0 |
Airlines II | 27 | 96.2 | 40.7 |
Universities | 10 | 100.0 | 70.0 |
Television Chefs | 40 | 77.5 | 45.0 |
Whisky distilleries | 5 | 100.0 | 80.0 |
Average | 18.25 | 86.7 | 55.3 |
Only a subset of the available topics from the entity track was suitable for this task (a full description of the tasks is given in Supplementary Table S2). The column ‘Siblings’ shows the number of siblings that can be found. Recall is the percentage of found siblings. R-precision is the precision at the R-th position where R is the number of expected siblings.
Domain . | Siblings . | Recall (%) . | R-precision (%) . |
---|---|---|---|
Professional sports teams | 8 | 75.0 | 62.5 |
Pharmaceutical products | 1 | 100.0 | 100.0 |
Airlines | 45 | 84.4 | 24.4 |
Companies | 10 | 60.0 | 20.0 |
Airlines II | 27 | 96.2 | 40.7 |
Universities | 10 | 100.0 | 70.0 |
Television Chefs | 40 | 77.5 | 45.0 |
Whisky distilleries | 5 | 100.0 | 80.0 |
Average | 18.25 | 86.7 | 55.3 |
Domain . | Siblings . | Recall (%) . | R-precision (%) . |
---|---|---|---|
Professional sports teams | 8 | 75.0 | 62.5 |
Pharmaceutical products | 1 | 100.0 | 100.0 |
Airlines | 45 | 84.4 | 24.4 |
Companies | 10 | 60.0 | 20.0 |
Airlines II | 27 | 96.2 | 40.7 |
Universities | 10 | 100.0 | 70.0 |
Television Chefs | 40 | 77.5 | 45.0 |
Whisky distilleries | 5 | 100.0 | 80.0 |
Average | 18.25 | 86.7 | 55.3 |
Only a subset of the available topics from the entity track was suitable for this task (a full description of the tasks is given in Supplementary Table S2). The column ‘Siblings’ shows the number of siblings that can be found. Recall is the percentage of found siblings. R-precision is the precision at the R-th position where R is the number of expected siblings.
4.3 Runtime
Our approach works on-the-fly, meaning every time siblings are generated, the pipeline (Fig. 3) is re-run with the given seed terms. By generating siblings on-the-fly, the results are always up-to-date and seed terms do not have to be biomedical terminology, but can be from any domain. Even though the retrieval and extraction process is highly parallelized, generating siblings can take up to 9 s. The overwhelming amount of time is spent with querying the web search engines (on average 2.38 s) and retrieving websites (on average 5.92 s). However, by caching recent sibling generations, existing results are returned almost immediately.
4.4 Ontology generation plugin
We integrated this work into DOG4DAG (Wächter and Schroeder, 2010), our ontology generation plugin for Protégé and OBO-Edit (see Fig. 7 for a screenshot of the plugin in Protégé). Siblings can be generated by either selecting a term with at least two child terms or by manually typing the seed terms. For each sibling, the plugin automatically checks for cross-references to other biomedical ontologies using the EBI Ontology Lookup Service (Côté et al., 2008) or the BioPortal web service (Whetzel et al., 2011). This way, biocurators can identify other ontologies of interest and link their terms to them. Furthermore, generated terms are automatically mapped to terms in the currently loaded ontology to help biocurators link terms to other ontologies. Finally, already existing terms are printed in bold face, allowing the user to quickly spot them in the loaded ontology.
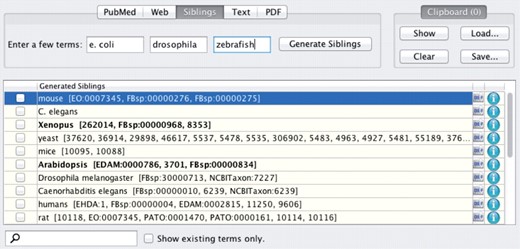
Screenshot of sibling generation results in the DOG4DAG plugin for Protégé
For experimentation, we loaded the Human Disease Ontology (DO) [http://diseaseontology.sourceforge.net] (as of 15/12/2011) into OBO-Edit and selected three genetic skin diseases terms (cutis laxa, Hailey–Hailey disease and Rothmund–Thomson syndrome) as seed terms for sibling generation. The candidate siblings can be found in Table 4. Almost all of the generated terms are also genetic skin diseases. Most of them are already part of the ontology (the terms in bold face). However, many of them are simply categorized as monogenic diseases and could be added to genetic skin diseases right away. However, a number of candidate siblings, such as Naegeli syndrome are not yet part of the ontology yet and can also be added to the parent term.
Generated siblings for genetic skin diseases (DOID:1698) using the child terms cutis laxa (DOID:3144), Hailey–Hailey disease (DOID:0050429), and Rothmund–Thomson disease (DOID:2732) as seed terms
Rank . | Generated sibling . | Parent term . |
---|---|---|
1 | Cockayne syndrome | Monogenic diseases |
2 | Xeroderma pigmentosum | Monogenic diseases |
3 | Hypohidrotic ectodermal dysplasia | Monogenic diseases |
4 | Incontinentia pigmenti | Genetic skin disease |
5 | Dyskeratosis congenita | Genetic skin disease |
6 | Erythrokeratodermia veriabilis | Genetic skin disease |
7 | Clouston syndrome | Monogenic disease |
8 | Proteus syndrome | Physical disorder |
9 | Erythropoietic Protoporphyria | Acute porphyria |
10 | Naegeli syndrome | Not yet part of DO |
Rank . | Generated sibling . | Parent term . |
---|---|---|
1 | Cockayne syndrome | Monogenic diseases |
2 | Xeroderma pigmentosum | Monogenic diseases |
3 | Hypohidrotic ectodermal dysplasia | Monogenic diseases |
4 | Incontinentia pigmenti | Genetic skin disease |
5 | Dyskeratosis congenita | Genetic skin disease |
6 | Erythrokeratodermia veriabilis | Genetic skin disease |
7 | Clouston syndrome | Monogenic disease |
8 | Proteus syndrome | Physical disorder |
9 | Erythropoietic Protoporphyria | Acute porphyria |
10 | Naegeli syndrome | Not yet part of DO |
Most of the terms are already part of the loaded human disease ontology (printed in bold). However, Naegeli syndrome is a term that can be added to genetic skin diseases.
Generated siblings for genetic skin diseases (DOID:1698) using the child terms cutis laxa (DOID:3144), Hailey–Hailey disease (DOID:0050429), and Rothmund–Thomson disease (DOID:2732) as seed terms
Rank . | Generated sibling . | Parent term . |
---|---|---|
1 | Cockayne syndrome | Monogenic diseases |
2 | Xeroderma pigmentosum | Monogenic diseases |
3 | Hypohidrotic ectodermal dysplasia | Monogenic diseases |
4 | Incontinentia pigmenti | Genetic skin disease |
5 | Dyskeratosis congenita | Genetic skin disease |
6 | Erythrokeratodermia veriabilis | Genetic skin disease |
7 | Clouston syndrome | Monogenic disease |
8 | Proteus syndrome | Physical disorder |
9 | Erythropoietic Protoporphyria | Acute porphyria |
10 | Naegeli syndrome | Not yet part of DO |
Rank . | Generated sibling . | Parent term . |
---|---|---|
1 | Cockayne syndrome | Monogenic diseases |
2 | Xeroderma pigmentosum | Monogenic diseases |
3 | Hypohidrotic ectodermal dysplasia | Monogenic diseases |
4 | Incontinentia pigmenti | Genetic skin disease |
5 | Dyskeratosis congenita | Genetic skin disease |
6 | Erythrokeratodermia veriabilis | Genetic skin disease |
7 | Clouston syndrome | Monogenic disease |
8 | Proteus syndrome | Physical disorder |
9 | Erythropoietic Protoporphyria | Acute porphyria |
10 | Naegeli syndrome | Not yet part of DO |
Most of the terms are already part of the loaded human disease ontology (printed in bold). However, Naegeli syndrome is a term that can be added to genetic skin diseases.
5 DISCUSSION
5.1 Text-based versus structure-based approach
First, we will look at the results of the two approaches and discuss individual advantages and disadvantages.
When examining the quantity of sibling candidates alone, the structure-based approach yields more results, because in contrast to the text-based approach it requires only that seed terms occur on the same web page, but not necessarily close to each other. Even very distant terms can be semantically related, like headings separated by multiple paragraphs. As long as the headings are on the same path in the parse tree, they will be discovered. On the other hand, this also leads to false positives, since not all headings on the same path are necessarily semantically related. In contrast, the search queries in the text-based approach include the introductory phrase (except for the most generic search pattern) and thus potentially find less results.
The structure-based approach has a number of other advantages. First, it works on arbitrary HTML documents, meaning almost all of the web search results can be utilized. Additionally, being able to exploit the structure of a document also means that this approach works independently of the language.
The second, text-based approach is based on an entirely different idea. Here, siblings are generated from text by finding enumerations in sentences and extracting the individual terms and the head term (if available) from text. Regular expressions can match these patterns in sentences with great variability. By automatically generating regular expressions, we do not need to be concerned about errors and omissions when creating the regular expressions. The expressions have been optimized for biomedical and chemical terms. For instance, they allow non-ASCII characters, punctuation inside terms (e.g. ‘1,3-Butadiene’), and multi word terms.
The fact that the text-based approach finds less results lies in the very nature of the pattern-based approach, which usually yields low recall (Hearst, 1992) and also fits the observations of Etzioni et al. (2005): their structure-based ‘List Extractor’ component finds about five times more results than the text-based approach. Contrary to our initial assumption, the precision is equal or lower than the structure-based approach in the MeSH evaluation (Fig. 5). This is mainly due to the format of the retrieved snippets which often contain truncated phrases and parts of sentences, making POS tagging and subsequent extraction of the enumerated terms harder.
Both approaches have a significant overlap in terms of generated siblings. This shows that each of them generates correct results independently. Nonetheless, each approach generates siblings which the other method does not discover.
5.2 Assessment of the completeness of ontologies
While there exist guidelines and tools that help to assess or even ensure the technical quality or consistency of a domain ontology (e.g. Yao et al., 2011), it is much harder to determine whether or not an ontology covers all aspects of the domain, hence it is hard to judge on completeness. With the help of our set expansion method for sibling discovery, we are able to provide some judgement by comparing the generated siblings with those already existing in the ontology.
Overall completeness of MeSH: Considering the evaluation for MeSH in Section 4.1, the generated siblings for the 1000 random sibling sets can be divided into five categories which are listed in Table 5. Almost 50% of the terms are generated correctly as a sibling or descendant of a seed term and as such are siblings where the automatic method agrees with our gold standard MeSH. Another 30.1% of the generated siblings occur in MeSH but not as sibling and further 13.4% are not present in MeSH but exist as term label within the UMLS Metathesaurus [The UMLS Metathesaurus (Bodenreider, 2004) is a collection of controlled vocabularies in the biomedical domain (including MeSH) and currently contains over 1 000 000 terms in total]. In summary, Table 5 shows that over 75% of the generated terms are part of MeSH, which indicates that MeSH is for the most part complete with regard to its term base. We also manually evaluated a sample of 100 generated sibling terms, which were not part of MeSH but can be found in the UMLS Metathesaurus. From these terms, as much as 52% were found to be true siblings of the seed terms (Table 6 and Supplementary Table S3) and are as such good candidates to be added to MeSH in the future.
Category . | Percentage (%) . |
---|---|
Sibling of seed term in MeSH | 40.7 |
Descendant of seed term in MeSH | 6.5 |
Occurs elsewhere in MeSH | 30.1 |
Occurs in UMLS, but not in MeSH | 13.4 |
Not found in UMLS | 9.3 |
Category . | Percentage (%) . |
---|---|
Sibling of seed term in MeSH | 40.7 |
Descendant of seed term in MeSH | 6.5 |
Occurs elsewhere in MeSH | 30.1 |
Occurs in UMLS, but not in MeSH | 13.4 |
Not found in UMLS | 9.3 |
In all, 47.2% of the terms are highly relevant and 90.3% are correct biomedical terminology. (Please note that the top 10 results are taken into account, no matter how many siblings the seed terms have in MeSH.)
Category . | Percentage (%) . |
---|---|
Sibling of seed term in MeSH | 40.7 |
Descendant of seed term in MeSH | 6.5 |
Occurs elsewhere in MeSH | 30.1 |
Occurs in UMLS, but not in MeSH | 13.4 |
Not found in UMLS | 9.3 |
Category . | Percentage (%) . |
---|---|
Sibling of seed term in MeSH | 40.7 |
Descendant of seed term in MeSH | 6.5 |
Occurs elsewhere in MeSH | 30.1 |
Occurs in UMLS, but not in MeSH | 13.4 |
Not found in UMLS | 9.3 |
In all, 47.2% of the terms are highly relevant and 90.3% are correct biomedical terminology. (Please note that the top 10 results are taken into account, no matter how many siblings the seed terms have in MeSH.)
Manual evaluation of the terms from the categories ‘Occurs elsewhere in MeSH’ and ‘Occurs in UMLS, but not in MeSH’
Category . | True siblings (%) . | Related siblings (%) . | False siblings (%) . |
---|---|---|---|
Occurs elsewhere in MeSH | 16 | 47 | 37 |
Occurs in UMLS, but not in MeSH | 52 | 16 | 32 |
Category . | True siblings (%) . | Related siblings (%) . | False siblings (%) . |
---|---|---|---|
Occurs elsewhere in MeSH | 16 | 47 | 37 |
Occurs in UMLS, but not in MeSH | 52 | 16 | 32 |
True siblings are generated terms that can be added to existing seed terms. Related siblings are terms with a similar subject, but are not true siblings of the seed terms. False siblings are terms which are not related to the seed terms.
Manual evaluation of the terms from the categories ‘Occurs elsewhere in MeSH’ and ‘Occurs in UMLS, but not in MeSH’
Category . | True siblings (%) . | Related siblings (%) . | False siblings (%) . |
---|---|---|---|
Occurs elsewhere in MeSH | 16 | 47 | 37 |
Occurs in UMLS, but not in MeSH | 52 | 16 | 32 |
Category . | True siblings (%) . | Related siblings (%) . | False siblings (%) . |
---|---|---|---|
Occurs elsewhere in MeSH | 16 | 47 | 37 |
Occurs in UMLS, but not in MeSH | 52 | 16 | 32 |
True siblings are generated terms that can be added to existing seed terms. Related siblings are terms with a similar subject, but are not true siblings of the seed terms. False siblings are terms which are not related to the seed terms.
Consistency of MeSH: Finally, we also evaluated a sample of 100 generated siblings which were not siblings of the seed terms but occurred at a different position within MeSH (see ‘Occurs elsewhere in MeSH’ in Table 6 and Supplementary Table S4). Only as few as 16% were found to be true siblings (Table 6). This indicates that MeSH is for the most part modelled correctly with regard to the location of its siblings.
Both results demonstrate that sibling generation is a powerful tool to assess the completeness of ontologies.
5.3 Adherence to ontology design criteria and naming conventions
Additionally, we examined the generated siblings regarding criteria for ontology design and naming conventions. In Schober et al. (2009), conventions for OBO Foundry ontologies were presented. The conventions support unified ontology development and help developers avoid mistakes when working on ontologies. Overall, the generated siblings adhere to the proposed design guidelines and naming conventions. For instance, new terms should incorporate the genus-differentia style for names. Since we prefer candidate siblings containing this style, these are ranked higher in the results. Another convention is that acronyms should be expanded. Because we included an abbreviation tagger in the processing pipeline (Fig. 3), they are automatically expanded, if possible. Finally, since the text-based approach only allows NPs as candidate siblings, we avoid the use of conjunctions.
5.4 Limitations and future work
Generally, our work is based on the assumption that terms can in principle be found in text and that the web is representative for a domain to be modelled by the ontology. Although we developed our approach as generic as possible, some limitations are nonetheless inherent.
First, we can only generate siblings for natural language terms which are semantically related and discussed in the literature or on websites in general. Furthermore, we do not take the specific relationship type and synonymous terms into account.
Overall, the system works best for completing a set of terms with the same semantic type. However, it cannot explicitly recognize the context of the given seed terms. We will consider on incorporating contextual information in the query to increase the precision of the generated siblings. Additionally, we will work on the improvement of recall of the text-based approach by two means. First, if the retrieved snippet is not a full sentence, fetch the whole webpage and extend the existing snippet to complete the sentence. Second, extend the number of patterns for text-based sibling discovery using a bootstrapped pattern learning approach, similar to the ones presented in Etzioni et al. (2005) and Kozareva et al. (2008). We also plan to further improve the scalability of sibling generation when more than four seed terms are used. Finally, we will investigate whether using a higher number of seed terms can effectively improve the precision of the retrieved candidate siblings.
6 CONCLUSION
In this work, we presented an approach to extend ontologies systematically by finding new terms similar to two or three provided terms. We combined two very different methods and used a simple rank aggregation strategy to combine the results. By taking the peculiarities of biomedical terminology into consideration, we used hypernym matching and compound term matching to improve the ranking of terms which fulfill these criteria.
The evaluation using MeSH shows that our approach can successfully support ontology engineers by semi-automatically completing existing sets of siblings. Additionally, our approach can also serve as a first step towards evaluating the completeness of ontologies. We showed that MeSH covers the biomedical domain as the vast majority of siblings suggested by the method are already contained. Nonetheless, a significant number of good candidates for incorporation could be suggested with high precision. Furthermore, our evaluation suggests that text mining in the biomedical domain gains significantly from full-text resources such as PubMed Central.
In particular, when evaluating set expansion for sibling discovery using 1000 randomly selected term sets from MeSH, our approach finds 79.3% of the existing siblings in the sets using three seed terms. Both methods contribute to the results. However, the structure-based approach finds slightly more true positives than the text-based approach. When only two seed terms are used, the method still produces satisfactory results, but recall and precision drop to 68.2% and 51.5%, respectively. The generated terms fulfill ontology naming conventions and need no post-editing.
Our method is universal, meaning the system allows sibling generation for any domain, as shown by the evaluation using the TREC ELC task, where 86.7% of the siblings were discovered with a precision of 55.3%. Additionally, the method can in principle generate siblings for any language, since the structure-based approach works independently of the language.
Since this work is integrated into the DOG4DAG plugin, ontologies of all common formats can be extended seamlessly in Protégé and OBO-Edit and generated terms are cross-referenced to other biomedical ontologies.
Funding: PONTE and GeneCloud.
Conflict of Interest: none declared.