-
PDF
- Split View
-
Views
-
Cite
Cite
Yulan Deng, Hao Luo, Zhenyu Yang, Lunxu Liu, LncAS2Cancer: a comprehensive database for alternative splicing of lncRNAs across human cancers, Briefings in Bioinformatics, Volume 22, Issue 3, May 2021, bbaa179, https://doi.org/10.1093/bib/bbaa179
- Share Icon Share
Abstract
Accumulating studies demonstrated that the roles of lncRNAs for tumorigenesis were isoform-dependent and their aberrant splicing patterns in cancers contributed to function specificity. However, there is no existing database focusing on cancer-related alternative splicing of lncRNAs. Here, we developed a comprehensive database called LncAS2Cancer, which collected 5335 bulk RNA sequencing and 1826 single-cell RNA sequencing samples, covering over 30 cancer types. By applying six state-of-the-art splicing algorithms, 50 859 alternative splicing events for 8 splicing types were identified and deposited in the database. In addition, the database contained the following information: (i) splicing patterns of lncRNAs under seven different conditions, such as gene interference, which facilitated to infer potential regulators; (ii) annotation information derived from eight sources and manual curation, to understand the functional impact of affected sequences; (iii) survival analysis to explore potential biomarkers; as well as (iv) a suite of tools to browse, search, visualize and download interesting information. LncAS2Cancer could not only confirm the known cancer-associated lncRNA isoforms but also indicate novel ones. Using the data deposited in LncAS2Cancer, we compared gene model and transcript overlap between lncRNAs and protein-coding genes and discusses how these factors, along with sequencing depth, affected the interpretation of splicing signals. Based on recurrent signals and potential confounders, we proposed a reliable score to prioritize splicing events for further elucidation. Together, with the broad collection of lncRNA splicing patterns and annotation, LncAS2Cancer will provide important new insights into the diverse functional roles of lncRNA isoforms in human cancers. LncAS2Cancer is freely available at https://lncrna2as.cd120.com/.
Introduction
It has been estimated that ~90% of multi-exon human genes undergo alternative splicing, which remarkably enriched functional diversity [1, 2]. Compared to normal samples, more than 30% of alternative splicing events were observed in tumor samples [3], producing a large amount of cancer-specific transcript isoforms. Some of them were known to translate into abnormal protein variants and subsequently contributed to cancer hallmarks [4]. Moreover, the dysregulation of splicing machinery in cancers, such as recurrent cancer-associated mutations, is important to understand tumorigenesis and could act as attractive therapeutic targets [5–7]. Therefore, previous studies have systematically investigated splicing patterns across different human cancers [8], deciphered their regulatory code [9, 10] and explored functional effects of abnormal protein domains [11, 12].
Long noncoding RNAs (lncRNAs) represent a kind of molecules that function as RNAs and actively take part in various biological processes, including carcinogenesis [13, 14]. Similar to protein-coding genes (PCGs), most of the lncRNA transcripts consist of multiple exons and are spliced into mature transcripts [15]. And previous studies suggested the alternative splicing of lncRNAs was unexpectedly universal [16, 17]. However, the splicing patterns of lncRNAs and isoform-specific roles were overlooked until several researchers reported their importance [18–24]. For example, in hepatocellular carcinoma, the long isoform of lncRNA PXN-AS1 prevented the degradation of mRNA PXN by binding to the 3′ untranslated region, while the short isoform inhibited the translation of PXN by interacting with its coding sequence [20]. Similarly, the long isoform of NEAT1, not the short one, sponged miR-106b-5p to regulate ATAD2 in papillary thyroid cancer [21]. These evidences supported a previous hypothesis that the alternative splicing of lncRNAs generated a repertoire to achieve function specificity [16, 25], suggesting that the lncRNA splicing patterns in human cancers might provide clues for their potential functional sequences. However, the studies for cancer-specific splicing patterns of lncRNAs are still in their infancy, and a comprehensive database for their regulators and pathological roles is still lacking.
To this end, we developed a comprehensive database, called LncAS2Cancer. First, both bulk RNA sequencing and single-cell RNA sequencing datasets across more than 30 cancer types were collected, and 8 types of splicing patterns were identified by 6 state-of-the-art algorithms. In order to facilitate the exploration of their regulators and potential functional sequences, the affected sequences were manually curated from the published literature and various databases about mutation, miRNA binding site and protein binding site. Survival analysis of splicing event was performed when clinical outcome data was available. What’s more, LncAS2Cancer provided a user-friendly web interface to browse, search, visualize and download all above information. Subsequently, several case studies demonstrated that LncAS2Cancer could not only confirm the known cancer-associated lncRNA isoforms but also indicate novel ones. Finally, we investigated the characteristics of lncRNA splicing patterns by comparing to PCGs and described potential confounders for splicing signals. Collectively, LncAS2Cancer is the first comprehensive resource for splicing patterns of lncRNAs in human cancers, which will serve as a valuable bioinformatics platform to investigate the roles of lncRNA isoforms in tumorigenesis.
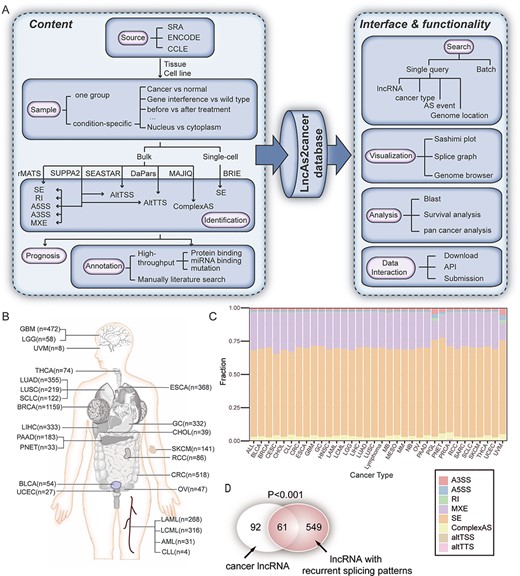
The overview of LncAS2Cancer. (A) The flowchart of database construction. (B) The number of samples in each cancer type. (C) Distribution of different splicing types across cancer types. (D) LncRNAs with recurrent differential splicing patterns were significantly enriched in cancer-associated lncRNAs.
Methods
Data collection
We first performed an extensive literature query of PubMed (https://www.ncbi.nlm.nih.gov/pubmed/), Sequence Read Archive [26] (SRA, https://www.ncbi.nlm.nih.gov/sra/), Encyclopedia of DNA Elements [27] (ENCODE, https://www.encodeproject.org/) and Cancer Cell Line Encyclopedia [28] (CCLE, https://portals.broadinstitute.org/ccle/) (Table S1). We kept the datasets according to the following criteria: (i) tumor patient samples, precancerous lesion samples (i.e. Barrett’s esophagus and liver cirrhosis), cancer cell lines and patient-derived xenograft samples were collected, (ii) paired-end RNA sequencing was required, (iii) read length should be longer than 50 bp, and (iv) only Smart-based methods were collected for single-cell RNA sequencing (scRNA-seq) (see Supplementary Methods). The gene models, i.e. the annotation of exons and introns for each transcript, were download from GENCODE (version 28, https://www.gencodegenes.org/) and FANTOM v5 [29]. Gene annotations were collected from GENCODE, NCBI, GeneCards (Version 4.10) [30] and Ensembl (release 96) [31].
Identification of lncRNA alternative splicing
The sequences of FASTQ format were aligned to the human genome hg38 using STAR [32] (version 2.5.4b). Because transcript models from GENCODE and FANTOM were complement to each other (Figure S1), both GENCODE v28 and FANTOM v5 were used as reference (see Supplementary Notes for details). The rMATS (version 4.0.2) [33] was used to detect five alternative splicing events, including skipped exon (SE), retained intron (RI), alternative 5′ splice site (A5SS), alternative 3′ splice site (A3SS) and mutually exclusive exons (MXE) for bulk RNA sequencing. The SUPPA2 (version 2.3) was used to detect SE, A5SS, A3SS, RI, MXE, alternative transcription start site (altTSS) and alternative transcription termination site (altTSS) [34]. DaPars [35] (version 0.9.1) and SEASTAR [36] (version 1.0.0) were used for altTTS and altTSS, respectively. The complex splicing (ComplexAS) was identified by MAJIQ [37] (version 2.0). The Percent Spliced In (PSI, Ψ) value [38] was used for rMATS and MAJIQ, and The Percentage of Distal Usage Index (PDUI) was used for SEASTAR and DaPars. PSI values were provided for samples whose splicing events with supporting reads >8. The threshold of significance was set as ΔΨ > 0.05, P < 0.05. For scRNA-seq, quality control was carried out by R package ‘scater’ [39] (version 1.10.1), and SE was identified by BRIE [40] (version v0.2.2). The threshold of significance was set as Bayesian factor > 10 (see Supplementary Methods and Notes for details).
Genomic annotation of affected sequences
The affected sequences referred to the part of RNA sequences that were different between isoforms after alternative splicing (Figure S2) [41]. The RNA sequence transcribed from alternative exons may provide clues for isoform-specific regulation or function [20, 42]. To explore the potential function of affected sequences and regulators, annotations from multiple databases were integrated, including GENCODE, Ensembl, POSTAR2 [43], StarBase (V3.0) [44], UCSC [45], dbSNP build 146 [46], COSMIC (v70) [47] and GWAS catalog [48]. The affected sequences of differentially alternative splicing were also manually annotated (see Supplementary Methods for details).
Survival analysis of lncRNA splicing patterns
The R package ‘survival’ was used for survival analysis. Based on the median PSI values, patients were classified into two groups: high group (PSI > median) and low group (PSI ≤ median). It was noted samples with no available PSI values were classified into low group. Only more than 10 samples in both groups were considered for analysis. The survival curves were estimated using the Kaplan–Meier method, and the log-rank test was used to analyze differences in survival time. Cox proportional hazard regression model was used. The splicing events that were significant for both log-rank test and cox analysis (P < 0.05) were deposited in LncAS2Cancer.
LncAS2Cancer web interface
LncAS2Cancer was implemented by XAMPP [(Apache (2.4.39), MariaDB (10.1.39), PHP (7.3.5) and Perl (1.7.1)]. JavaScript and jQuery UI (v1.12.1) were also used to analyze and visualize data. Bootstrap (v3.3.7) was adopted to design web pages. We used Echart (version 4.0) and Genoverse for data visualization tools to better interaction. LncAS2Cancer is compatible with the popular browsers, including Chrome, Firefox and Safari. LncAS2Cancer will be regularly updated every 4–6 months. The whole datasets in this paper is available for download in LncAS2Cancer (https://lncrna2as.cd120.com/download/) and FigShare (https://figshare.com/authors/Hao_Luo/8948129).
Results
Global view of LncAS2Cancer
LncAS2Cancer is a database aimed to present a collection and annotation of lncRNA splicing across human cancers (Figure 1A). Currently, LncAS2Cancer included a total of 5335 bulk RNA sequencing and 1826 single-cell RNA-seq samples from 275 datasets, covering over 30 cancer types (Figure 1B, Table S2). Tumor samples and cancer cells under different conditions were collected (see Supplementary Methods), such as gene interference, therapy and subcellular localization. These samples allowed us to infer the condition-specific splicing patterns of lncRNAs, as well as their potential regulators.
Totally, 50 859 alternative splicing events of 4155 lncRNA were detected against GENCODE, and 151 216 alternative splicing events of 9316 lncRNA were detected against FANTOM. Eight splicing types were considered, in which SE and MXE were the most frequently detected events, accounting for more than 80% of all the splicing events. The distribution of the different types of alternative splicing events in each cancer was summarized in Figure 1C. For samples with group information, significant differential splicing patterns were identified, resulting in 6551 differential events. Among them, 2176 (33.2%) were cancer-specific (Tables S3 and S4). Notably, we found that the lncRNAs with recurrent differential splicing patterns were enriched in cancer lncRNAs from Lnc2Cancer (v2.0) [49] (Figure 1D).
In order to explore the potential function of affected sequences, annotations from multiple databases were integrated, including protein binding sites and miRNA binding sites around the affected sequences. And the functional effects of splicing sequence were also manually curated from the published literature. In a previous study [19] (Figure 3A), cancer risk-associated SNP regulated lncRNA isoform preference through promoter-to-enhancer switching. Therefore, SNPs and mutations from dbSNP [46], COSMIC [47] and GWAS catalog [48] were also deposited in the database. The distribution of different annotations for eight splicing types was described in Table 1. In addition, survival analysis was performed in datasets with survival information, to explore whether the alternative splicing of lncRNAs could act as a potential prognostic biomarker.
Summary statistics of alternative splicing events and annotation from multiple sources
. | Total . | Manual annotation . | Protein binding . | miRNA binding . | Subcellular localization . | GWAS . | COSMIC . |
---|---|---|---|---|---|---|---|
SE | 33 528 | 60 | 6402 | 585 | 274 | 279 | 1742 |
RI | 147 | 2 | 63 | 41 | 8 | 9 | 18 |
A3SS | 765 | 16 | 234 | 80 | 17 | 20 | 38 |
A5SS | 532 | 0 | 129 | 53 | 12 | 5 | 43 |
MXE | 10 934 | 105 | 111 | 51 | 87 | 4 | 40 |
altTSS | 512 | 0 | 118 | 19 | 5 | 3 | 6 |
altTTS | 2045 | 91 | 637 | 214 | 82 | 37 | 89 |
complexAS | 2396 | 47 | 743 | 215 | 4 | 73 | 210 |
. | Total . | Manual annotation . | Protein binding . | miRNA binding . | Subcellular localization . | GWAS . | COSMIC . |
---|---|---|---|---|---|---|---|
SE | 33 528 | 60 | 6402 | 585 | 274 | 279 | 1742 |
RI | 147 | 2 | 63 | 41 | 8 | 9 | 18 |
A3SS | 765 | 16 | 234 | 80 | 17 | 20 | 38 |
A5SS | 532 | 0 | 129 | 53 | 12 | 5 | 43 |
MXE | 10 934 | 105 | 111 | 51 | 87 | 4 | 40 |
altTSS | 512 | 0 | 118 | 19 | 5 | 3 | 6 |
altTTS | 2045 | 91 | 637 | 214 | 82 | 37 | 89 |
complexAS | 2396 | 47 | 743 | 215 | 4 | 73 | 210 |
Abbreviations: skipped exon (SE), retained intron (RI), alternative 5′ splice site (A5SS), alternative 3′ splice site (A3SS), mutually exclusive exons (MXE), alternative transcription termination site (altTTS), alternative transcription start site (altTSS), complex splicing (ComplexAS).
Summary statistics of alternative splicing events and annotation from multiple sources
. | Total . | Manual annotation . | Protein binding . | miRNA binding . | Subcellular localization . | GWAS . | COSMIC . |
---|---|---|---|---|---|---|---|
SE | 33 528 | 60 | 6402 | 585 | 274 | 279 | 1742 |
RI | 147 | 2 | 63 | 41 | 8 | 9 | 18 |
A3SS | 765 | 16 | 234 | 80 | 17 | 20 | 38 |
A5SS | 532 | 0 | 129 | 53 | 12 | 5 | 43 |
MXE | 10 934 | 105 | 111 | 51 | 87 | 4 | 40 |
altTSS | 512 | 0 | 118 | 19 | 5 | 3 | 6 |
altTTS | 2045 | 91 | 637 | 214 | 82 | 37 | 89 |
complexAS | 2396 | 47 | 743 | 215 | 4 | 73 | 210 |
. | Total . | Manual annotation . | Protein binding . | miRNA binding . | Subcellular localization . | GWAS . | COSMIC . |
---|---|---|---|---|---|---|---|
SE | 33 528 | 60 | 6402 | 585 | 274 | 279 | 1742 |
RI | 147 | 2 | 63 | 41 | 8 | 9 | 18 |
A3SS | 765 | 16 | 234 | 80 | 17 | 20 | 38 |
A5SS | 532 | 0 | 129 | 53 | 12 | 5 | 43 |
MXE | 10 934 | 105 | 111 | 51 | 87 | 4 | 40 |
altTSS | 512 | 0 | 118 | 19 | 5 | 3 | 6 |
altTTS | 2045 | 91 | 637 | 214 | 82 | 37 | 89 |
complexAS | 2396 | 47 | 743 | 215 | 4 | 73 | 210 |
Abbreviations: skipped exon (SE), retained intron (RI), alternative 5′ splice site (A5SS), alternative 3′ splice site (A3SS), mutually exclusive exons (MXE), alternative transcription termination site (altTTS), alternative transcription start site (altTSS), complex splicing (ComplexAS).
Database usage
In the LncAS2Cancer, users can search, browse and download interesting information. In the search page, users can select a specific lncRNA name, lncRNA type, location, AS type, AS ID, annotation, disease, cell line or database to query splicing events across all the datasets. The multi-condition searching, batch search and API in the tools pages were also available (Figure S3). For gene annotation files, this database returned the results of GENCODE as default. Users can choose FANTOM in advanced search to search alternative splicing events by FANTOM annotation. Moreover, users could compare a piece of sequence to blast against alternative splicing events. Users can also download basic information of splicing events data in the result table or API. The whole datasets could be accessed in download section or FigShare. More details on how to use the database could be found in the ‘Help’ page and a guide button in the lower right corner of the website.
As an example, the results after searching ‘TUG1’ and details of search table (‘ID’ 99 309) were shown in Figure 2. There were several sections in the detail page. First, the basic information of TUG1, such as alias, location and function, was shown in Figure 2B. Then splice graph of the splicing event was shown, providing the read distributions across the different exons. The details of significantly differential splicing were shown in sashimi plot, and the PSI value of each sample could be obtained by clicking ‘detail’ in group information. Also, the distribution of PSI values of the splicing signal was compared across multiple cancers or tissues. Subsequent, manually curated annotation and genome browser (including miRNA binding sites, RBP binding sites, GWAS, COSMIC, dbSNP, repeat elements and regulatory features) were used to explore the functional effect of such splicing event. Furthermore, survival plots for splicing events were provided if data available (Figure 2H).
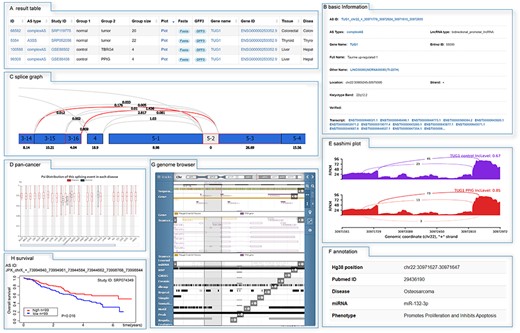
The detailed information of search results. (A) The search result of TUG1. The detail information was accessed by clicking on the ‘ID’ 99 309, including (B) basic information, (C) splice graph, (D) the distribution of PSI values across multiple cancers or tissues, (E) sashimi plot, (F) manual annotation and (G) genome browser.
The known lncRNA isoforms in cancers
We summarized splicing patterns of lncRNAs isoforms in tumorigenesis, described their regulators and further investigated whether they could be found in LncAS2Cancer.
Several pioneering studies demonstrated that the roles of lncRNAs for tumorigenesis were isoform-dependent. In prostate cancer, the long isoform of PCAT19, not the short one, interacted with HNRNPAB and subsequently activated cell cycle genes [19] (Figure 3A). In other cases, both isoforms might be functional, in spite of their expression pattern. For example, short isoform of NEAT1, NEAT1_1, was highly expressed in various cell types, and the expression of NEAT1_2 was cell type specific [50]. While NEAT1_1 could increase active chromatin marks at the PSMA promoter, acting as a critical modulator of prostate cancer [51], NEAT1_2, an essential components paraspeckle, promoted the progression of hepatocellular carcinoma by mediating IL-6 induced STAT3 phosphorylation [52]. NEAT1_2 was also reported to sponge miRNAs in cytoplasm, despite that NEAT1 was nuclear-enriched [21] (Figure 3C). Interestingly, different lncRNA isoforms might exert opposite functions on the same oncogene. Pvt1a increased the protein stability of Myc, but Pvt1b repressed the expression of Myc in cis [18] (Figure 3B). Similarly, the long isoform of PXN-AS1 (PXN-AS1-L) upregulated PXN mRNA and protein expression, but the short form (PXN-AS1-S) inhibited PXN mRNA translation elongation [20] (Figure 3D).
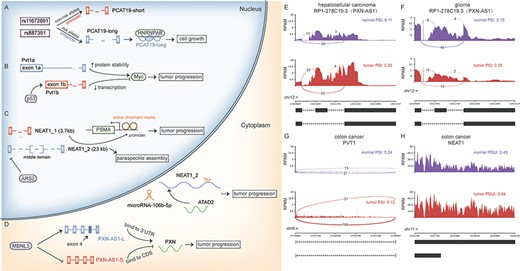
Known lncRNA isoforms were associated with different functions in tumorigenesis. Different functions of lncRNA isoforms and their regulations, including (A) PCAT19, (B) PVT1, (C) NEAT1 and (D) PXN-AS1.The same alternative splicing event of PXN-AS1 was identified in a hepatocellular carcinoma dataset (E) and a glioma dataset (F). (G–H) The switches between PVT1a and PVT1b and NEAT1_1 and NEAT1_2 were observed in colon cancer. CDS, coding sequences.
Since the function of lncRNA isoforms might be distinct, their tipping balance was tightly regulated. The risk-associated SNP rs11672691 of prostate cancer was located in the promoter of PCAT19-short and enhancer of PCAT19-long, respectively, with bifunctional activities. The linkage disequilibrium SNP rs887391 of risk variant decreased the interaction between transcription factors and the promoter of PCAT19-short, leading to weaker promoter activity and stronger enhancer. Through such a promoter-to-enhancer switching mechanism, the risk alleles regulated the reciprocal expression of PCAT19 isoforms. In hepatocellular carcinoma, MBNL3 promoted the inclusion of exon 4 of PXN-AS1, preferring PXN-AS1-L to PXN-AS1-S. Similarly, p53 induced Pvt1b expression in response to oncogenic stress, and ARS2 regulated the stability of NEAT1 isoforms.
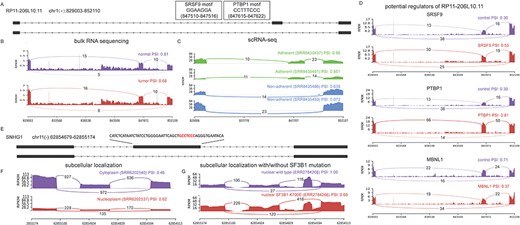
Case studies for lncRNA splicing patterns and their potential regulators. (A) The structure of a SE event. Binding motifs of SRSF9 and PTBP1 were observed in the upstream of the skipped exon (B and C). This event was detected in colon cancer from bulk and single-cell sequencing datasets. (D) The gene interference experiments datasets showed that SRSF9, PTBP1 and MBNL1 affected the exon inclusion. (E) Structure of a SE event for SNHG1. A motif (GCTCCC) of nuclear localization was located in the skipped exon. (F) The exon inclusion efficiency of SNHG1 was higher in the nucleoplasm than cytoplasm. (G) SF3B1 mutation (K700E) was associated with splicing efficiency of SNHG1.
The splicing of known lncRNA isoforms and their potential regulators could be explored using LncAS2Cancer. In SRP056696 (hepatocellular carcinoma), compared to normal samples, PXN-AS1 (also called RP1-278C19.3) significantly increased the inclusion of exon 4 in cancer samples (Figure 3E), which was consistent with the oncogenic role of PXN-AS1-L in liver cancer [19] (Figure 3D). Except for hepatocellular carcinoma, the same aberrant splicing was also found in glioma (Figure 3F, SRP127187), suggesting potential roles across cancers. Also, the switch between PVT1a and PVT1b [18] (Figure 3B and G) and NEAT1_1 and NEAT1_2 [51, 52] (Figure 3C and H) could be found in colon cancer (SRP119775) from LncAS2Cancer.
Case studies for lncRNA splicing patterns and their potential regulators
Besides known lncRNA isoforms in cancer, LncAS2Cancer could be used to explore novel lncRNA splicing patterns and their potential regulatory mechanisms during tumorigenesis. For example, comparing with non-tumor colon tissue, RP11-206 L10.11 showed decreased exon inclusion efficiency in colon cancer samples from a bulk sequencing dataset (SRP119775, AS ID: RP11-206 L10.11_chr1_ + _847654_847806_829003_829104_851927_852110, Figure 4B). Also, from another scRNA-seq, the same splicing pattern was observed in breast cancer cells with high proliferation (SRP178543, labeled as ‘Non-adherent’ in Figure 4C), showing a recurrent pattern. In order to explore potential regulators of such splicing event, we searched for RNA interference experiments that significantly affected this alternative splicing event in LncAS2Cancer. We found that knockdown of SRSF9 (GSE80856) or PTBP1 (GSE80895) increased the exon inclusion and knockdown of MBNL1 (GSE88116) promoted exon skipping (Figure 4D). Moreover, motifs of SRSF9 and PTBP1 recorded in ATtRACT database [53] were located in ~100 bp upstream of the skipped exon (Figure 4A), further supporting their potential regulatory roles.
The preference for subcellular localization could be also investigated in LncAS2Cancer. For example, lncRNA SNHG1 has been reported to function in both nucleus and cytosol [54, 55]. In LncAS2Cancer, exon inclusion efficiency of SNHG1 was higher in nucleoplasm than cytoplasm (SRP120954, Figure 4F, AS ID: SNHG1_chr11_-_62854888_62854938_62854080_62854551_62855133_62855174). Consistent with it, a reported motif of nuclear localization of lncRNAs [56], ‘GCCTCC’, was located in the skipped exon (Figure 4E). In a sequencing dataset at nuclear level, cancer cells with SF3B1 mutation (K700E) showed decreased exon inclusion of such event (Figure 4G, ERP110734), suggesting a potential regulatory role of splicing factor SF3B1.
Confounders for the signals of lncRNA alternative splicing
The availability of a large sample size in LncAS2Cancer made it possible to study the difference of alternative splicing between lncRNAs and PCGs.
Comparison of gene models between lncRNAs and PCGs
In the gene annotation files from GENCODE, transcripts with level 1 meant all splice junctions of the transcript were supported by at least one non-suspect RNA, thus representing the most reliable annotation. There were 37.29% (28 700/76 974) and 10.55% (1684/15 961) transcripts scored as 1 for PCGs and lncRNAs, respectively. The fractions of constitutive exon for lncRNAs were significantly lower than those of PCG (Figure 5A, Kolmogorov–Smirnov test, P < 0.0001). Although level 1 transcripts harbored more constitutive exons for both lncRNAs and PCGs (P < 0.0001), level 1 lncRNA transcripts still showed less constitutive exons, compared to level 1 PCGs (P < 0.0001).
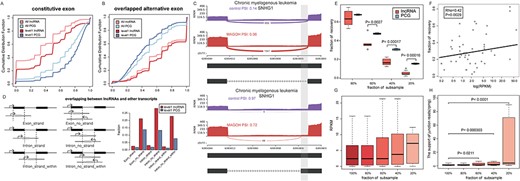
Confounders for the signals of lncRNA alternative splicing. (A) The fractions of constitutive exon for lncRNAs were significantly lower than those of PCGs. (B) The fractions of alternative exon for lncRNAs were higher than those of PCGs. (C) An example of SNHG1 showed the structure of lncRNA isoforms complicated the identification of potential splicing patterns. (D) The comparisons of the fractions of level 1 transcripts between lncRNAs and PCGs in different overlapping situation. (E) In subsample analysis, less alternative splicing events of lncRNAs were recovered, compared to PCGs. (F) The expression of exons in SE events was positively correlated with the fraction of recovery. The exon expression (G) and splice site alignments (H) of detected events in subsample analysis suggested that the splice site alignments were more sensitive to sequencing depth.
Next, we compared the fractions of alternative exons that overlapped with exons in other isoforms. We found that the fraction of such exons was significantly higher in lncRNAs, in all transcripts or only level 1 transcripts (P < 0.0001, Figure 5B). Deciphering the signal for overlapped exons may be sophisticated [57]. For example, in chronic myelogenous leukemia (GSE80930), two significant retained intron events were identified for the exact region chr11(−): 62 853 803–62 854 064 of lncRNA in SNHG1 (Figure 5C), and there was only 16 bp difference in their exon boundaries. If only one of the two events was meaningful, identification of both events might increase the burden of multiple hypotheses test.
The overlap between lncRNAs and other genes
The lncRNAs isoforms might also overlap with exons of other genes, and the influence was different for strand-specific and non-strand-specific datasets. In the gene annotation files from GENCODE, 2.5% (28/1114) of level 1 lncRNA transcripts harbored exons that overlapped with exons of other genes in the same strand, and it increased to 21.1% (236/1114) without considering strand information (Figure 5D). By contrast, there were only 1.8% (245/13964) and 13.8% (1921/13964) of PCGs harboring such exons with or without strand information. For introns, there were 2.1% (24/1114, same strand) and 22.8% (254/1114, without strand information) level 1 lncRNA transcripts, in which exons of other genes were located within the boundary of introns. Similarly, less fraction of PCG transcripts had such introns.
Sequencing depth
To assess the effect of sequencing depth, we took a dataset, SRP127585, as an example. In the subsample analysis, less fraction of alternative splicing events of lncRNAs were recovered, if subsampled in less than 60% of the total reads (Figure 5E). At 20% of the total reads, only 4.08% (2/49) of SE events for lncRNA could be recovered, compared to 15.33% (450/2936) of SE events for PCG. As expected, SE events with high-expressed exons were more likely to be recovered in subsample datasets (Pearson correlation coefficient = 0.42, P = 0.0029, Figure 5F). Next, we assessed the exon expression and spliced junction expression as sequencing depth decreases, which might indicate utility of them to characterize splice signals at low coverage. In the results, while the exon expression showed slightly increasing trend along with decreased sequencing depth (Figure 5G), the splice site alignments at low sequencing depth were significantly higher than those from original dataset, with 5.24-fold change at 20% of total reads (Figure 5H), suggesting the splice site alignments were more sensitive to sequencing depth.
Above all, some splicing events showed recurrent signals, and affected sequences were annotated by other database. By contrast, some events might be ambiguous due to overlap with other genes or low expression. This information could be used to prioritize splicing events for further elucidation. Therefore, we scored each splicing event for reliability and expected that splicing events with high score should be validated with priority (see Supplementary Methods).
Discussion
LncRNAs actively participate in various processes of cancer hallmarks, and several pioneer studies supported that the roles of lncRNAs were isoform-dependent. However, isoform-level studies for lncRNAs are still in their infancy. To provide a full view of lncRNAs splicing patterns and potential regulators across human cancers, we developed a user-friendly database, called LncAS2Cancer. To our knowledge, this is the first database to detect and annotate alternative splicing of lncRNAs in human cancers. Currently, LncAS2Cancer included a total of 50 859 alternative splicing events in 4155 lncRNAs from 7161 samples, covering over 30 cancer types. There were several features in LncAS2Cancer: (i) samples from bulk sequencing and scRNA-seq were collected, and the latter allowed users to consider cellular heterogeneity [58]; (ii) patient samples and cancer cell lines under seven conditions were collected (e.g. gene interference, therapy and subcellular localization), which facilitated to infer the condition-specific splicing patterns of lncRNAs, as well as potential regulators; (iii) annotation information from eight sources were integrated to understand the functional impact of affected sequences; (iv) survival analysis was performed to explore whether lncRNA alternative splicing could act as a potential biomarker. In LncAS2Cancer, users could conveniently browse, search, visualize and download the above information.
It was reported that LncRNA locus could locally regulate gene expression by at least three potential mechanisms [59]. In the first case, the process of transcription or splicing, independent of RNA sequence, enabled gene regulation, such as Airn [60]. For the second case, gene regulation solely depended on DNA elements (e.g. enhancer) within the lncRNA locus. A recent study demonstrated that the splicing of such lncRNA could promote enhancer activity and thus required efficient RNA splicing [17]. For the third case, the RNA transcript itself was required to perform regulation function, and specific isoforms may carry out different functions. From the results in LncAS2Cancer, known cancer-associated lncRNAs were enriched in lncRNAs with recurrent differential splicing patterns, which suggested that most of them were involved in the second or third case. Moreover, it was hypothesized that the alternative splicing might generate an enormous repertoire of potential lncRNAs with modular exons. Therefore, characterization of splicing patterns of lncRNAs may, in turn, indicate its potential functional sequence. Also, the relative location of functional elements in different lncRNA isoforms, such as SNP [19] and CpG island [61], might suggest distinctive regulatory mechanisms.
The model of lncRNAs and PCGs might look similar [62], and it is intuitive to identify their alternative splicing using tools that were originally designed for PCGs. However, Melé et al. found the splicing was the most discriminant difference between long intergenic noncoding RNAs (lincRNAs) and mRNAs, when chromatin environment and transcriptional regulation were also considered [63]. Using the datasets in LncAS2Cancer, we carefully compared the gene models and splicing signals between lncRNAs and PCGs and found that they were quite different in several aspects. First, the annotations of lncRNA transcripts were in poorer quality. Several studies have struggled to provide a better annotation of lncRNAs, such as GENCODE and FANTOM, which underlined the basis of the characterization of lncRNA splicing patterns [29, 62, 64]. Second, the constitutive exons of lncRNAs were significantly less than those of PCG, and this situation may complicate their identification, such as challenge of multiple hypotheses test. Third, the overlap among exons within the same lncRNA locus or between lncRNAs and other genes was higher, which might affect the signal-to-noise ratio. In LncAS2Cancer, users could check these regions in genome browser to exclude potential false positive. For experiment design, strand-specific RNA sequencing may be helpful. Finally, as lncRNAs were generally lower expressed, the identification of alternative splicing was more sensitive to sequencing depth, especially for the supporting of junction reads, and thus deeper sequencing is badly required. Another strategy may be to integrate other information [65], such as splicing motifs and functional elements.
LncAS2Cancer will be regularly updated as the growing of public data. In the future version of this database, new features will be integrated. For example, long-read sequencing, which can capture the whole transcripts, will be considered in the future. In addition, studies revealed that functional noncoding isoforms could be transcribed from PCG locus [66], and such isoforms will be collected for LncAS2Cancer in the future. Further, to understand the potential functions of affected sequences, multi-omics data can also be considered, such as RNA methylation [67] and ChIP-seq [68].
Conclusion
In conclusion, LncAS2Cancer is a comprehensive data repository for lncRNA splicing patterns in more than 30 cancer types from both bulk RNA sequencing and single-cell RNA sequencing datasets. Manual annotations, as well as databases about mutation, miRNA binding site and protein binding site, were integrated to explore the functional roles of affected sequence. Survival analysis was performed to indicate potential biomarkers of lncRNA isoforms. The comparison between lncRNAs and PCGs revealed the potential confounders for interpreting splicing patterns of lncRNAs. Considering recurrent signals, annotation information and potential confounders, we proposed a reliability score to prioritize splicing events for further elucidation. Overall, LncAS2Cancer provided a user-friendly interface to search, browse, visualize and download detailed information. We believe that it will empower researchers to investigate the diverse functional roles of lncRNA isoforms in human cancers.
We developed a comprehensive database for the alternative splicing of lncRNAs in human cancers, called LncAS2Cancer, which included a total of 50 859 alternative splicing events in 4155 lncRNAs across over 30 cancer types.
With the help of annotations in LncAS2Cancer, users could explore function of affected sequence, as well as their potential regulators.
LncAS2Cancer provided a user-friendly interface, where users could browse, search, visualize and download interesting information in different ways.
We compared the alternative splicing between lncRNAs and protein-coding genes and described the potential confounders when identifying splicing patterns of lncRNAs, which provided suggestion for the identification and explanation of splicing signals.
Yulan Deng is a post-doctor in the Department of Thoracic Surgery, West China Hospital, Sichuan University.
Hao Luo is a laboratory technician in the Department of Thoracic Surgery, West China Hospital, Sichuan University.
Zhenyu Yang is a MM student in the Department of Thoracic Surgery, West China Hospital, Sichuan University.
Lunxu Liu is a professor in the Department of Thoracic Surgery, West China Hospital, Sichuan University.
Funding
National Natural Science Foundation of China (grant number 31801119, 81672311); Key Science and Technology Support Program of Sichuan Province, China (grant number 2016FZ0118); Project funded by China Postdoctoral Science Foundation (Grant number 2019T120835, 2019M650243); Post-Doctor Research Project, West China Hospital, Sichuan University (grant number 2019HXBH047); 1.3.5 project for disciplines of excellence, West China Hospital, Sichuan University (grant number ZYGD18021, ZYJC18009).
References
Author notes
Yulan Deng, Hao Luo and Zhenyu Yang are contributed equally to this work.