-
PDF
- Split View
-
Views
-
Cite
Cite
Saman Zeeshan, Ruoyun Xiong, Bruce T Liang, Zeeshan Ahmed, 100 Years of evolving gene–disease complexities and scientific debutants, Briefings in Bioinformatics, Volume 21, Issue 3, May 2020, Pages 885–905, https://doi.org/10.1093/bib/bbz038
- Share Icon Share
Abstract
It’s been over 100 years since the word `gene’ is around and progressively evolving in several scientific directions. Time-to-time technological advancements have heavily revolutionized the field of genomics, especially when it’s about, e.g. triple code development, gene number proposition, genetic mapping, data banks, gene–disease maps, catalogs of human genes and genetic disorders, CRISPR/Cas9, big data and next generation sequencing, etc. In this manuscript, we present the progress of genomics from pea plant genetics to the human genome project and highlight the molecular, technical and computational developments. Studying genome and epigenome led to the fundamentals of development and progression of human diseases, which includes chromosomal, monogenic, multifactorial and mitochondrial diseases. World Health Organization has classified, standardized and maintained all human diseases, when many academic and commercial online systems are sharing information about genes and linking to associated diseases. To efficiently fathom the wealth of this biological data, there is a crucial need to generate appropriate gene annotation repositories and resources. Our focus has been how many gene–disease databases are available worldwide and which sources are authentic, timely updated and recommended for research and clinical purposes. In this manuscript, we have discussed and compared 43 such databases and bioinformatics applications, which enable users to connect, explore and, if possible, download gene–disease data.
Introduction
Despite all of the scientific knowledge, much of medicine is still based on the treatment of symptoms and performing learned trials based on treatments, which works for most patients. Genetic research is assisting in producing tailored solutions to each individual, rather than what works for the average population, and understanding who is at risk for critical diseases like diabetes, high blood pressure or cancer. The variability in human genome sequence is a result of the biological code responsible for the development and functioning of a human being [1–6]. The complexity of human deoxyribonucleic acid (DNA) is a measure of the information contained within the DNA, and the maximal information possible in a solution of genomic DNA purified from a tissue or cell is equivalent to the total number of base pairs (bps) present in the haploid genome [7–12]. The majority (~62%) of the human genome comprises of intergenic regions, the non-protein coding parts of the genome that lie between genes, used to be called `junk DNA’, but now genome research over the past few years has revealed functions associated with these regions, suggesting that every part of the genome may have some importance [13–23]. Intergenic DNA may also include gene regulatory sequences [24–31], such as promoters [32–37], enhancers [38–43] and silencers [44–46] that have yet to be characterized. Ribonucleic acid (RNA) [47–54] is the transcribed form of DNA and messenger RNA (mRNA) is the protein-coding form of RNA [55,56]. Non-coding RNAs, such as transfer RNA (tRNA) [57–59], micro RNA (miRNA) [60–62], ribosomal RNA (rRNA) [63–67] and long non-coding RNA (lncRNA) [68–71], play various roles in the cell, from protein translation to gene regulation.
Not every sequence in DNA resolves in disease and the major goal of medical genetics is to identify genes that lead to human disease when altered [72–79]. Most are simple differences called as polymorphism that may not change the expression and meaning of the gene, but alterations at the wrong place could change the gene instructions and ultimately create a malfunction protein, which may cause disease. The first characterized mammalian gene hemoglobin (HBB) [80], which encodes the beta-globin polypeptide, was fully characterized in humans in 1970s [81]. While many years earlier in 1949, Linus Pauling had shown that a defect in the structure of hemoglobin causes sickle cell anemia and that amounts of normal and defective hemoglobin in carriers pointed to a single gene defect [82]. It took scientists years to establish the link between a mutant beta globin gene that sickles hemoglobin and causes sickle cell anemia. It was the discovery of genes, which led to the understanding of the disease. However, an exact count of human genes has not been determined yet. In 1964, the human geneticist Friedrich Vogel was the first to estimate the number of human genes at 6.7 million, but he made clear that his estimate was based on many assumptions [83–86]. There were many laboratories around the world that surmised the number of human genes even before the complete sequence of the genome. While the complete human genome sequence has provided a means for uncovering most genes, many will probably remain hidden from view in the near future. The human genes have a discontinuous structure [87], with the protein coding regions, or exons, interrupted by non-coding regions, or introns. An average human gene has nine exons, and the longest known human gene called titin (TTN) [88] has 365 exons spanning 109 224 bp and encodes a protein of 35 991 amino acids [89]. For some time, many researchers used a broad estimate of gene count at more than 50 000 including 21 000 protein coding genes [90]. However, this number has repeatedly been overturned with advancements in genetics and genomics research.
Human diseases are at the heart of extensive research encompassing genomics, bioinformatics, systems biology and systems medicine. To get new insights into disease taxonomy, etiology and pathogenesis, it’s important to understand how the diseases are related to each other [91]. In the past, various efforts have been made in deciphering disease to facilitate predictive diagnosis and thereby guide treatment factors [92], which include drawing disease relationships using clinical manifestations [93], healthcare records [94], images and data generated using wearable technology and artificial intelligence [95] and information encapsulated within related genes [96], proteins [97], biological [98], chemo-centric views [99] and metabolic pathways, microRNA, phenotypic characteristics and microbes, etc. Studying genome and epigenome [100] led to the fundamentals of development and progression of human diseases [97] (e.g. cancer [101], heart disease, diabetes, polydactyly, spina bifida [102], etc.), which includes chromosomal diseases [102–106] (entire or segment of large chromosome is missing, duplicated or altered), monogenic diseases [107–114] (single-gene disorder effecting another gene’s function), multifactorial disorders (mutations in multiple genes) and mitochondrial disorders [115–119] (due to mutations in non-chromosomal DNA located within the mitochondria). All human diseases are maintained by the World Health Organization (WHO) with the standard creation of International Classification of Diseases (ICD) codes [120–125]. There are many databases available worldwide that claim to provide information about genes and link to related classified diseases. Our aim was to study how gene–disease based information is publically shared, why there are so many databases for such purposes, which sources are authentic, timely updated and recommended for research and clinical purposes.
The clinical interpretation of the significance of specific gene variants can be unique to a patient. Variability in interpretation for sequence variants is due, in part, to the lack of standard curated information to support clinical decision making. Currently, investigation of multiple databases is required to assess the potential significance of even one sequence variant, and that is a cumbersome, time-consuming and increasingly unfeasible process with regard to identification and reports of variants in actionable genes because of the absence of a standard centralized platform for connecting genes to their disease phenotype. In this review, we look at the progress of genomics from pea plant genetics to the human genome project and highlight molecular, technical and computational developments (Figure 1). Furthermore, we discuss and compare 43 gene–disease databases (ClinVar, CNVD, Cochrane Library, Cosmic, dbSNP, DGIdb, Disease Ontology, DiseaseEnhancer, DISEASES, DrugBank, ExPASy, FDA Approved Drugs, FMA, GeneGo, GeneReviews, Genetics Home Reference, GenomeRNAi, GEO DataSets, GTR, HMDB, IUPHAR, KEGG, LifeMap, LncRNADisease, LOVD, MedlinePlus, MeSH, MGI, miR2Disease, NCBI Bookshelf, NCI, NDF-RT, NIH Clinical Center, NINDS, Novus Biologicals, OMIM, Orphanet, R&D Systems, Reactome, Sino Biological, SNOMED-CT, UniProtKB/Swiss-Prot, Tocris) and present personalized web- and desktop-based bioinformatics applications (MSigDB, DigSee, DAVID, DisGeNET, HGMD, Gene2Function, SwissVar, eDGAR, GeneAlacard), which enable users to connect, explore and, if possible, download gene–disease data (Table 1).
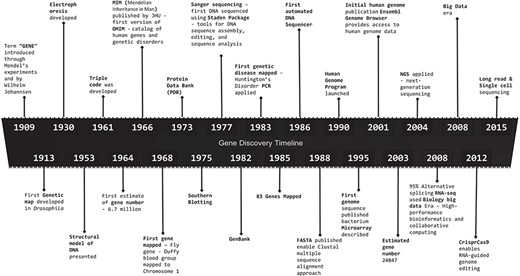
Gene discovery timeline, presenting major discoveries and inventions in the field of genomics, starting from 1909 to 2015. 1909: Term `gene’ introduced through Mendel’s experiments and by Wilhelm Johannsen. 1913: First Genetic map developed in Drosophila. 1930: Electrophoresis developed. 1953: Structural model of DNA presented. 1961: Triple code was developed. 1964: First estimate of gene number—6.7 million. 1966: Mendelian Inheritance in Man (MIM) published by John Hopkins University (JHU)—first version of OMIM—catalog of human genes and genetic disorders. 1968: First gene mapped—Fly gene—Duffy blood group mapped to Chromosome 1. 1973: PDB. 1975: Southern blotting. 1977: Sanger sequencing—first DNA sequenced using Staden Package—tools for DNA sequence assembly, editing and sequence analysis. 1982: GenBank. 1983: First genetics disease mapped—Huntington’s Disorder PCR applied. 1985: 83 genes mapped. 1986: First automated DNA sequencer. 1988: FASTA published enable Clustal multiple sequence alignment approach. 1990: Human Genome Program launched. 1995: First genome sequence published bacterium microarray described. 2001: Initial human genome publication Ensembl Genome Browser provides access to human genome data. 2003: Estimated gene number 24847. 2004: NGS applied. 2008: 95% Alternative splicing RNA-seq used Biology big data Era—High-performance bioinformatics and collaborative computing. 2008: Big data era. 2012: CrisprCas9 enables RNA-guided genome editing. 2015: Long-read and single-cell sequencing.
History of genetics: molecular, technological and computational
DNA was discovered in 1869 by Johann Friedrich Miescher, a Swiss biochemist working in Tübingen, Germany [126]. Three years before Miescher’s discovery, Gregor Mendel had published the results of his breeding experiments using pea plants, carried out in the monastery gardens at Brno, a central European city in what is now the Czech Republic. Based on his experiments on pea plant hybridization in 1865, the hereditary characters were defined as some certain intracellular substance that could cause differences in traits [127]. The establishment of the concept of particulate inheritance inspired thinking about the nature of this material. Mendel's paper in the Proceedings of the Society of Natural Sciences in Brno describes his hypothesis that inheritance is controlled by unit factors, the entities that geneticists today call genes. Later in 1909, one of Mendel’s students, Wilhelm Johannsen, finally used the word `gene’ to describe units of heredity. Since then, and for more than a hundred years, people have explored the structure, localization, quantity and function of genes.
The physical basis of heredity was the first critical question that early researchers aimed to address. Although Mendel didn’t know the nature of those characters, he considered these characters to be physical entities [127]. In 1869, when Miescher was analyzing cellular components, he discovered a substance he named `nuclein’ that precipitated in an acidic solution and crystallized in an alkaline solution. In fact, this was the crude extract of nucleic acids. However, due to the limitations of chemical knowledge at that time, Miescher was unable to determine the exact chemical nature of `nuclein’ [128]. Until 1944, the Avery–MacLeod–McCarty experiment proposed that DNA, not protein, is the substance that causes cellular transformation, clarifying the chemical nature of genes [129].
The concept of base-pairing was presented by Erwin Chargaff that the DNA nucleotide base adenine (A) always shares the same number of thymines (T), as do guanines (G) with cytosines (C). Soon after, this was explained by James Watson’s and Francis Crick’s structure of the DNA double helix, in which A pairs with T and C pairs with G [130]. Marshal Nirenberg then defined the DNA genetic code as three DNA base combinations or codons for each of the 20 amino acids that make up proteins [131]. With the unveiling of the DNA double helix, the structure of genetic material was finally confirmed, and with the identification of codons for amino acids, scientists had revealed that DNA did in fact harbor a code.
At almost the same time that the term `nuclein’ was introduced to define genetic material, chromosome behavior based on the process of meiosis proposed by August Weismann. This early version of chromosomal behavior echoed Mendel's law of segregation and independent assortment. However, shortly after the birth of chromosome theory, a new phenomenon was found in sweet peas to contradict the law of independent assortment. Some genes were found to exhibit `coupling’, which further developed into the phenomenon of `linkage’. Based on this finding, in 1913 Alfred Sturtevant and Thomas Hunt Morgan produced the first genetic linkage map in the fruit fly, Drosophila Melanogaster [132]. Researchers soon shifted their focus from model organisms to humans and mapping of the first human gene; the Duffy blood group gene mapping to human chromosome 1 was the first gene assigned to a human autosome [133]. Researchers used inheritance studies and chromosomal markers to show the first disease-associated single gene, Huntington's disease gene (HTT), which was mapped to human chromosome 4 [134]. After 46 human chromosomes per human cell was confirmed as the normal number of chromosomes by Joe Hin Tjio in 1955, Jerome Lejeune discovered Down syndrome was caused by trisomy 21 (three copies of chromosome 21 instead of the normal two copies) [135]. In 1985, 831 genes were presented at the 8th workshop of Human Genome Mapping [136].
The transition from the linkage map to the physical map was necessary because the gene's physical carrier chromosome had been discovered and genetic research resisted the bounds of abstraction. The complex structure of the human genome was well characterized and also viewed by some to be an insurmountable obstacle to generating the complete human sequence and grounds for early resistance to the Human Genome Project—conceived in 1984 [137]. Despite these obstacles, a great international scientific effort was launched. The Human Genome Project aimed to determine the sequence of DNA as well as identify and map all the genes of the human genome. Other genome sequencing efforts occurred concurrently, and after years of work by countless scientists all over the world, the first fully sequenced genome was finally published in 1995, that of the bacterium Haemophilus Influenzae. Six years later, the initial human genome publication marked a milestone in the field of genetic research. This international effort led to the completion of the human reference genome in 2003 (International Human Genome Sequencing Consortium 2004), and with a finished human genome reference in hand, it became possible to decipher the functional elements of the genome.
In addition to the physical entities and relative positions of genes, another significant aspect of the genome is the function of genes. The first report on gene function appeared in 1941, and since then, genes and proteins have been inextricably linked. George Beadle and Edward Tatum demonstrated that one gene was responsible for one enzyme [138]. With the process of deciphering the genetic code, the `central dogma’—`one gene to one mRNA to one protein’—had been confirmed [139]. This was proposed by Francis Crick, and this laid the foundation for modern genetics. However, scientists soon discovered that the flow of genetic information in cells is obviously more complex. The research results of Sydney Brenner, François Jacobs and Matthew Meselson in 1961, greatly expanded our understanding of gene function by identifying the function of mRNA [140]. In the study of the regulatory mechanism of Escherichia coli lactose metabolism, they found that some genes couldn’t form a synthetic protein template, but only regulate or manipulate, which put forward the operon theory. From then on, genes were divided into structural genes, regulatory genes and operator genes based on gene function [26].
Richard Roberts and Phil Sharp showed that eukaryotic genes have many interruptions called introns, and, in 1978, Walter Gilbert proposed the same idea [141–143]. He believed that a gene was a mosaic of DNA sequences containing two segments: one segment expressed and present in mature mRNA called the `exon’ and the other segment, although expressed at the same time, would be deleted in the mature mRNA, called the `intron‘ [144]. The proposal of alternative splicing explained that a single gene can produce more than one transcript by the combination of different exons [145]. The expression of these different RNAs from one gene makes possible the enormous protein diversity found in humans involving 95% of all genes.
Sequencing to precise gene editing
Since the order of nucleic acids in a polynucleotide chain defines the genetic information, the ability to sequence DNA is critical to decipher the code. With the development of electrophoresis technology, Southern blotting was invented to detect a specific DNA sequence [146]. However, electrophoresis can only separate DNA fragments of different sizes and cannot perform the sequencing process. By adding chain-terminating dideoxynucleotides to the DNA synthesis process, the basis of the Sanger sequencing method, the sequencing process was carried out by electrophoresis [147]. Although time-consuming and labor-intensive, Sanger sequencing has been widely used for more than 40 years and helped scientists complete the first sequence of the human genome. A chain-terminating reaction by a two-enzyme (sulfurylase/luciferase) system avoided heavy electrophoresis and enabled real-time sequencing. Isolation of a DNA copying enzyme, DNA polymerase, by Arthur Kornberg in 1955 was a major milestone towards next-generation sequencing (NGS) [148]. NGS based on pyrosequencing (a light-detecting method) has opened a new era of high-throughput sequencing with an easier sequencing step and reduced time consumed. The latest generation of sequencing technology freed up the requirement of DNA amplification and enabled single-molecule sequencing (SMS) that avoids amplification-related biases and errors. After a DNA template is attached to a substrate by one nucleotide base, the appropriate fluorescent reversible terminator dNTP (so called 'virtual terminator') is washed, imaged once, cleaved and cycled to the next one [149]. Over the years, innovations in sequencing protocols, molecular biology and automation have increased the technical capabilities of sequencing while reducing costs.
With the continuous development and improvement of whole-genome sequencing (WGS) and the initiation of large-scale annotation projects, researchers are consistently applying new genome sequence data to their research. Identification of the first genetic mutation for cystic fibrosis was one of the most significant discoveries in the history of human genetics [150, 151]. This was followed by thousands of cloned genes and vast amounts of accumulated genomic data, and while this has made discovery easier, it has also led to our current challenge of how to convert these huge amounts of data into meaningful genomic functions or clinically relevant information. Targeted gene inactivation using homologous recombination provided a good way to help scientists define the function of genes, a main goal of understanding the human genome. However, this method is also subject to several limitations: it lacks efficiency, it is time-consuming and laborious and it increases the chance of introducing mutations. Gene-targeted knockout techniques such as RNA interference (RNAi; targeting RNA with complementary RNA) provide scientists with a fast and inexpensive method for conducting high-throughput studies. However, the knockout effect of RNAi technology is not generally complete, and the results of each test and each laboratory can be different. In addition, there is an unpredictable off-target effect, so it can only be used in experiments that require temporary suppression of gene function.
Artificial nucleases, zinc-finger nucleases, transcription activator-like effector nucleases and meganucleases, combined with a sequence-specific DNA binding domain and a non-specific DNA cleavage domain can artificially modify the genome with high efficiency and high precision by cutting DNA to produce a double-stranded break, and then relying on an endogenous non-homologous end-joining mechanism for repair. Recently, a new type of genome modification technology was developed, called Clustered Regularly Interspaced Short Palindromic Repeats (CRISPR) or CRISPR/Cas (Cas refers to the nuclease component). CRISPR/Cas is a genetic system used by bacteria to protect against viruses and other foreign bodies, and scientists can leverage this to edit genes by creating RNAs that direct a Cas9 nuclease to cleave and modify specific matching genomic DNA loci [152]. Compared with other editing methods, CRISPR/Cas is easier to manipulate, more efficient and has a higher probability of homozygous mutants. In addition, multiple mutations can be introduced simultaneously at different sites.
Genomics to proteomics
Bioinformatics began with protein research, because it was widely believed that proteins carried genetic information; this changed after a 1952 phage infection experiment, which proved that DNA was the real genetic material. The first bioinformatics software, COMPROTEIN, was developed by Margaret Dayhoff to determine the primary structure of a protein using Edman sequencing data. Then the first protein database, Protein Data Bank (PDB), was created. When researchers confirmed the importance of DNA, the focus of research quickly turned to gene sequencing. The first software dedicated to analyzing Sanger sequencing reads was published by Roger Staden in 1979 and was used to search for overlaps between Sanger gel readings; verify, edit and link sequence readings to contigs; and annotate and manipulate sequence files. In 1985, Richard Stallman published the GNU's Not Unix (GNU) declaration and later grew into a free software philosophy, advocating the idea that users can freely run, copy, distribute, research, change and improve software. With the impression of this concept, and the accumulation of various gene sequencing data, worldwide laboratories and databases cooperated to create a large number of public databases that are still in use now. With the completion of The Human Genome Project in 2003, the foundational data source of these gene databases was finally established. The second generation of sequencing and the emergence of corresponding processing tools have made the whole biological information field increasingly powerful and have marked the era of biological big data analysis. But with big data, new problems have emerged, such as the generation of vast numbers of tools now available that makes it difficult to identify the one needed for a specific task. Also, increasing numbers of laboratories have a need to analyze big data, even if they don't specialize in bioinformatics research. In the future, bioinformatics development will focus on unity and user-friendliness.
Gene to disease databases and tools
Genome-scale science is becoming increasingly common with the advancement of high-throughput technologies. A key challenge in this realm is the interpretation of NGS data. Scientists are faced with the daunting challenge of identifying candidate genes that are relevant to their biological system of interest. Most often, a researcher only has direct knowledge of a few, if any, of the candidate genes. Many complex diseases, such as cancer, develops with the sporadic acquisition of genome alterations. These alterations may cause functional cellular issues or be inert. Not all mutations contribute equally to the cancer type in which they are found. The proportion of mutations causally implicated in cancer is still unknown. Although the number of unique variants for each cancer genome can be very high, only a few variants will be critical for the development of the tumor [153]. This necessitates the need of bioinformatics tools linked to comprehensive knowledgebase.
The clinical treatment of diseases is based on patient phenotype in the context of others treated previously. Shifting a clinician’s focus from symptoms and physiology—phenotype—to individual genetic variations—genotype—as the source of the disease has been the greatest challenge for crafting personalized medicine. It is true that the relationships between genes and diseases are complex, but their implications can reshape both research and clinical practice. Amid the continuous advancement of sequencing technology and data analytics, WGS has become more popular and affordable. Whole-genome analysis methods integrated with epidemiologic investigations yield the ultimate resolution into disease outbreaks. Also, with the discovery and association of genetic variants with diseases, precise treatment based on individual genome variations has become a big trend, improving disease risk prediction and personalized medicine. Over the past few decades, the new methods in all the pathology specialties, genome-based predictive diagnostics for creating a genomic fingerprint of each individual has become central in allowing for the rapid detection and characterization of disease.
There are numerous databases and tools with information about genes, diseases, variants, proteins and pathways. The pioneer databases of genes, variants and phenotypes are the Online Mendelian Inheritance in Man (OMIM) established in 1966 and GenBank in 1982 [154, 155]. The wealth of data generated at this time gave rise to several other such public and private databases. Databases have different sponsors, operate independently from one another and use different standards [156]. According to a report of 2014 Molecular Biology Database Collection in the journal Nucleic Acids Research, a total of 1552 databases were publicly accessible online [157]. There are some databases providing online services without publication in peer-reviewed journals or being administered by commercial companies [e.g. Human Gene Mutation Database (HGMD)], some databases are inaccessible over time, not maintained/updated or even never used. Moreover, none of these databases are free from errors, which often occur at multiple levels. Since the major public databases store data in a generalized fashion, often these databases do not contain more specialized types of information that would be of interest to specific segments within the biological community. To address this, many smaller, specialized databases have emerged, developed to fulfill specific needs [157]. An annotated list of such databases can be found in the yearly Database Issue of Nucleic Acids Research [158]. Considering the continuously proliferating number of biological databases, it becomes increasingly daunting to navigate this huge number of databases [159]. Users must navigate from database to database, which makes the interpretation of genes time-consuming and inefficient.
We screened databases that provide gene to disease searches from among 43 databases and nine tools for further analysis (Table 1). Screening these databases allowed us to identify inconsistences and potential concerns for their use. The first criteria regarding the most basic function of a gene search was the failure to automatically generate related disease names corresponding to an input gene. The second filtration measure was the inability to take multiple genes as an input or upload a list of genes of interest to search. This feature is convenient for big data analytics. The third point of comparison was not providing information about disease-related ICD codes, whether directly or indirectly for every gene. Lastly, we assessed the simplicity of search and ease of navigation, which holds great importance for promoting database extensibility and usability. Our screening results are presented in Table 2.
![]() |
![]() |
Elements highlighted in blue stand for affirmative and elements highlighted in red, negative.
![]() |
![]() |
Elements highlighted in blue stand for affirmative and elements highlighted in red, negative.
ClinVar
ClinVar at the National Center for Biotechnology Information (NCBI) is a freely available archive of variants for reported conditions [160]. The database includes germline and somatic variants of any size, type or genomic location. Interpretations are submitted by clinical testing laboratories, research laboratories, locus-specific databases, OMIM, GeneReviewsTM, UniProt, expert panels and practice guidelines. ClinVar currently holds >158 000 submitted interpretations, representing >125 000 variants [160]. Interpretations in the database affect more than 26 000 genes, including structural variants that may include many genes; for variants that affect a single gene, almost 4800 genes are represented in it. It allows users to enter a gene name, e.g. BRCA1 (Figure 2A), and it will retrieve all related variations and associated conditions by name, as well as their review status and last time reviewed (Supplementary Data, Figure 1). In the help page, they claim their review status can be divided into eight continuous grades, but they do not present their results according to these grades. Even though there is an option to filter based on review status, it would be more helpful if the results could be presented with the grades rating from high to low. Also, their website can search one gene at a time and cannot take multiple gene inputs, which leaves researchers with a lot of work to collect and integrate information. Their results mainly come from three different sources: clinical testing, research and literature. Besides literature, there are no adequate ways and systems to measure the accuracy of results from clinical testing, and research relies on the data provider. Therefore, some information might be ambiguous and vague.
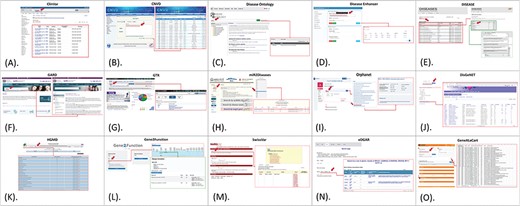
Gene to disease databases and tools. (A). ClinVar search page and single gene input. (B). CNVD search page and multiple gene input. (C). DO search page and single gene input. (D). DiseaseEnhancer search page and multiple gene search. (E). DISEASE search page and single gene input. (F). GARD search page and single gene input. (G). GTR search page and single gene input. (H). miR2Diseases search page and single gene input. (I). Orphanet search page and single gene input. (J). DisGeNET search page and multiple gene input. (K). HGMD search page and single gene input. (L). Gene2Function search page and single gene input. (M). SwissVar search page and multiple gene search. (N). eDGAR search page and multiple gene search. (O). GeneAlaCart search page and multiple gene search. High-resolution images are attached in supplementary data.
Copy Number Variations in Disease
Copy Number Variations in Disease (CNVD) is a systematic and comprehensive database for copy number variations and related diseases, in which all the records are manually extracted from experimental data published in CNV-related articles [161]. Hence, CNVD is a reliable resource for studying disease-associated copy number variations. It incorporates 251 697 records, containing 183 219 CNV segments, 844 related diseases and 46 348 genes mined from articles published from 2006 to 2014. CNVD allows users to search the database in several ways: by gene name, disease name, chromosome location or copy number variation region. In query results, each record contains such information: species, chromosomes, the start and end locations of the CNV, related disease, genes in the CNV region and PubMed ID of the source article. Even though CNVD is based on genes with copy number variations, results still present gene name and related disease clearly. The results can be downloaded in batches or individually, according to users' needs. CNVD can perform two-way searches both from genes to diseases and from diseases to genes, facilitating gene annotation (Figure 2B). Also, its ability to perform multiple gene searches brings great convenience to research (Supplementary Data, Figure 2). However, it does not group redundant results, which means that the same results will recur if they have different sources. This integration step requires the user to complete it themselves, adding an unexpected amount of extra work. The entire database is based solely on experimental data published in CNV-related articles, so the data volume may be too small for consideration.
Disease Ontology
The Human Disease Ontology (DO) is a biomedical resource of standardized common and rare disease concepts with stable identifiers organized by disease etiology [162]. The current version of DO expands the utility of ontology for the examination and comparison of genetic variation, phenotype, protein, drug and epitope data through the lens of human disease [162]. It provides hierarchical open-source ontology for the integration of biomedical data that is associated with human disease. Users can create basic or advanced queries. The basic search field explores every field in the query ontology to derive a DOID, name, synonym, xref or a block of text that could be found in the definition field. For example, when entering BRCA1, the result is a related disease and it’s DOID. DO is a professional website for disease research, which also includes ICD codes for each disease. But they are not linked to the gene and have to be searched separately for every disease (Figure 2C). As it is not a gene research-specific website, the inability to perform searches for multiple inputs and the relatively small gene pools are a relative downside (Supplementary Data, Figure 3).
DiseaseEnhancer
DiseaseEnhancer is a well-curated database for disease-associated enhancers. As of July 2017, it includes 847 disease-associated enhancers in 143 human diseases [163]. Database features include basic enhancer information (e.g. genomic location and target genes), disease types and associated variants of the enhancer and their associated phenotypes (e.g. gain/loss of enhancer and the alterations of transcription factor binding) [163]. Genetic alterations/variants of enhancers make an essential contribution to disease progression. This site is based on enhancer-gene interactions rather than directly based on genome. But as gene-associated enhancers play a role in defining disease phenotype, this website only provides an indirect connection (Figure 2D). However, the number of disease results is very small (Supplementary Data, Figure 4). Although this database can perform two-way searches for disease-to-gene and gene-to-disease and also takes multiple gene inputs, this database can only be considered as a partial reference.
DISEASES
DISEASES integrates the results from text mining disease–gene associations, cancer mutation data and genome-wide association studies from existing databases [164]. Developed by The University of Copenhagen, this database provides disease–gene associations mined from literature. Searching for a gene such as BRCA1 generates a primary results page with all matched names in the system including primary name, type and Ensembl ID. Clicking on the gene of interest text in the list of primary results leads to a second detailed page with disease information for that gene. The three-tiered output consists of text-mined, curated and experimental results. For each disease, corresponding publications show up upon clicking on its name. DISEASES is a useful database because of its unique data collection method and large capacity [164]. However, it is too time-consuming for researchers with large-scale gene searches. It is too cumbersome to get to the disease information for a gene, and even more complex to find the referenced publication. Also, there is no way for users to extract the results in an organized way (Figure 2E). DISEASES database do contain ICD codes in their system, but they are provided with diseases, not directly linked to genes (Supplementary Data, Figure 5).
Genetic and Rare Diseases Information Center
Genetic and Rare Diseases Information Center (GARD) maintains a list of rare diseases and related terms to help the public find reliable disease information. It is a program of the National Center for Advancing Translational Sciences to provide access to current, reliable and easy to understand information about rare and genetic diseases in English or Spanish. GARD at the National Institutes of Health (NIH) coordinates research and information on rare diseases. It is a resource more suitable for general patients, rather than researchers. When a gene is searched, the output shows all related diseases, with some results not directly relevant or are repetitive (Figure 2F). Also, the search is limited to one gene at a time (Supplementary Data, Figure 6).
Genetic Testing Registry
The National Institute of Health Genetic Testing Registry (GTR) maintains comprehensive information about testing offered worldwide for genetic-based disorders [165]. The database provides details of each test (e.g. its purpose, target populations, methods, measurements, analytical validity, clinical validity, clinical utility and ordering information) and laboratory (e.g. location, contact information, certifications and licenses) [165]. Until October 2018, the GTR [47] website states that it has explored multiple research studies in all 55 475 tests, 11 260 conditions, 16 452 genes and 509 laboratories. GTR provides a central location for voluntary submission of genetic test information by providers [165]. It takes a single gene input and simply gives the conditions found to be associated with it. However, as stated on the website, NIH does not independently verifies information submitted to the GTR; it relies on submitters to provide information that is accurate and not misleading (Figure 2G). However, GTR includes information from resources such as ClinVar and MedGen from within the NIH and many resources from outside the NIH [165]. So additional proof checking and validation may be needed for data on this website (Supplementary Data, Figure 7).
miR2Disease
miR2Disease is a concisely curated database of human diseases involving microRNA deregulation [166]. A search provides details on a microRNA and its disease relationship, including a microRNA ID, the associated disease name, a brief description of the microRNA–disease relationship, an expression pattern of the microRNA, the detection method for microRNA expression, experimentally verified target gene(s) of the microRNA and a literature reference. It provides a user-friendly interface for convenient retrieval of each entry by microRNA ID, disease name or target gene [166]. There are currently 1881 human microRNAs reported in Gencode Release 29 (GRCh38.p12). According to the website (Figure 2H), from its creation in 2008 until 2018, miR2Disease has documented 3273 curated relationships between 349 human microRNAs and 163 human diseases. Even though miR2Diseases has a very small scope, it still provides useful information. However, users can only make choices from a list of existing miRNA target genes, rather than manually entering genes. This limitation makes miR2Diseases short of our target database scope (Supplementary Data, Figure 8).
Orphanet
Orphanet was established to gather scarce knowledge on rare diseases to improve the diagnosis, care and treatment of patients with these diseases. Orphanet is a multi-stakeholder global consortium of 40 countries, coordinated by the core resource team at the French National Institute of Health and Medical Research in Paris. It covers over 6000 existing rare diseases from sources including OMIM, ICD10, MeSH, MedDRA, GARD, UMLS and a classification of diseases elaborated using existing published expert classifications. It is designed for all audiences and it can perform multi-directional searches, although only one gene can be searched at a time. For every gene search, a series of associated proteins appears, which upon clicking show the information related to the disease. Clicking on the disease gives the ICD codes for that specific disease (Figure 2I). This is inconvenient for basic gene research as the search involves a lot of steps to gather all the disease-related information for a particular gene. Orphanet has the potential to be a great reference database (Supplementary Data, Figure 9).
DisGeNET
DisGeNET is a discovery platform that contains a comprehensive catalog of genes and variants associated to human diseases [167]. It covers the whole landscape of human diseases, including Mendelian, complex, environmental and rare diseases, as well as disease-related traits. Furthermore, the platform includes a web interface with analytical tools and packages to interact with the data. DisGeNET allows users to input multiple genes at the same time [167]. The system will give a separate introduction to each gene and combine their disease associations in one line, which can be downloaded with all the related information (Figure 2J). However, the related diseases are ordered by the assigned score rather than by corresponding gene name. Gene and disease related information can be obtained from their relevant pages, which similar to other databases is a multi-step process (Supplementary Data, Figure 10).
The Human Gene Mutation Database
The Human Gene Mutation Database (HGMD) constitutes a comprehensive collection of published germline mutations in genes that underlie, or are closely associated with, human inherited diseases [168]. As of March 2017, the database contained in excess of 203 000 different gene lesions identified in over 8000 genes manually curated from over 2600 journals. With new mutation entries currently accumulating at a rate exceeding 17 000 per year, HGMD represents the de facto central unified gene–disease repository of heritable mutations causing human genetic disease [168]. The HGMD search option allows users to jump to a specific mutation data set if the exact gene symbol is known. Users have to register for access to HGMD mutation data. There are five ways HGMD may be searched (Figure 2K). The primary information includes gene symbol, location and gene description, which refers to the related diseases. By clicking on a gene name, users can get complete information that includes diseases and phenotypes. However, the details cannot be accessed unless the professional version with Array Studio feature is purchased. It also does not allow multiple inputs and cannot group disease aliases (Supplementary Data, Figure 11).
Gene2Function
Gene2Function (G2F) is an online resource that maps orthologs among human genes and common genetic model species supported by Model Organism databases (MODs) and displays summary information for each ortholog. G2F makes it easy to survey the wealth of information available for orthologs and navigate from one species to another and connects users to detailed reports and information at individual MODs and other sources. G2F supports searches with one gene or one disease term. For a gene search, species of interest must be selected in addition to the gene name, gene symbol or other identifier for the gene itself. A search with a gene symbol displays the search term you entered, predicted orthologs in other species, information regarding confidence in the ortholog relationship and summary information. For a human disease, the result count for associated genes comes up with details for each gene (Figure 2L). After one more click on the counting number, the name of related disease will show up. The advantage of G2F is that it can conduct two-way searches, both from gene to disease and disease to gene. However, as with many other annotation tools, it cannot take multiple gene input. Also, it is impossible to jump directly to the associated disease; it is one more `click’ away (Supplementary Data, Figure 12).
SwissVar
SwissVar provides access to a comprehensive collection of single amino acid polymorphisms (SAPs) and diseases in the UniProtKB/Swiss-Prot knowledgebase via a unique search engine [169]. It represents nearly 4160 genes with disease annotations and 20 412 human proteins. SwissVar is a website for protein-disease research, therefore, this database is not directly related to gene–disease research (Figure 2M) [169]. However, it provides profound information on protein coding genes. Users can only input single genes and the result will come out with protein accessions for the protein formed by the gene and diseases caused by the protein (Supplementary Data, Figure 13).
eDGAR
eDGAR is a database for disease–gene associations with annotated relationships among genes derived from OMIM, Humsavar and ClinVar [170]. For each disease-associated gene, eDGAR collects all information on its annotation [170]. The present release of eDGAR includes 2672 diseases, related to 3658 different genes, for a total of 5729 disease–gene associations [170]. eDGAR includes a network-based enrichment method for detecting statistically significant functional terms associated to groups of genes. It is a small tool for 3658 protein coding genes and their relationship with diseases. It takes multiple gene input, and provides a list of related diseases, which can be exported as well (Figure 2N). For the source of this relationship, users must explore different pages, which can be time-consuming (Supplementary Data, Figure 14).
GeneCardSuite
GeneCardsSuite is a set of biomedical databases and tools, which includes: GeneCards [171], MalaCards [173], GeneALaCart [174] and GeneAnalytics [178]. GeneCards is a gene-centric database, with its authors claiming to have integrated data for 152 704 human genes from 125 sources [171]. Some data in GeneCards can be easily interpreted, while others require few extra mining steps for analysis. The suite consists of companion databases and analysis tools including GeneCards for comprehensive human gene annotation [172], MalaCards for gene–disease links [173], GeneALaCart for batch queries [174] and GeneAnalytics for finding functional partners and for gene set distillations [178]. The premium tools are only available upon licensed purchase. MalaCards is the human disease database featuring 19 289 human diseases and their associated 65 000 aliases, with annotations integrated from 69 sources in 15 sections [174–176]. One of these is the genes section, unifying every disease with its related gene. It employs sophisticated data-mining strategies modeled after the widely used GeneCards database [177]. It effectively addresses some of the major challenges facing disease bioinformatics. GeneCards and MalaCards delineate and share a multi-tiered, scored gene–disease network and high-quality gene–disease pairs, coming from manually curated trustworthy sources that include 4500 genes for 8000 diseases [176].
GeneAnalytics’s authors claim to have automatically mined data from more than 120 data sources [178]. Its broad descriptor categories enable users to focus on areas of interest, each rich with annotation and supporting evidence. The GeneAnalytics analysis provides gene annotations from GeneCards and diseases category is powered by MalaCards. The disease category includes several filters that enable the user to focus on the results of interest. GeneALaCart is a gene-set-orientated batch query engine, a fundamental tool in GeneCardsSuite [171]. It provides batch query support, whereby the user submits a gene list, along with the desired GeneCards annotation fields, and receives tabulated output, which can be visually examined or serve as input to automated scripts for more sophisticated analyses [172]. Licensed subscription purchase is necessary for searching 10 000 gene entries. Upon selecting disorders/diseases as our field of interest, the resulting output comes from both MalaCardsDisorders and UniprotDisorders. The results give gene aliases, related diseases, sources and, in most cases, the PubMed ID of referenced literature. GeneALaCart generates a file of GeneCards annotations associated with a list of genes. For each batch query, a list of gene identifiers is supplied and an annotation of interest is selected; GeneALaCart then extracts the information from GeneCardsSuite companion databases and gives a one-to-one gene–disease association [172]. In contrast to GeneALaCart, GeneAnalytics runs a disease filter such that it gives all the diseases matched to the queried gene set. Each disease is further linked to all its connected genes. It is difficult to separate out the input genes of interest from all the disease-related genes. The result is not a direct gene to disease outcome (Supplementary Data, Figure 15). For this reason, GeneALaCart is a preferable tool for large-scale gene searches (Figure 2O).
Discussion
The completion of the Human Genome Project laid the foundation for systematically studying the human genome from evolutionary history to personalized medicine. It gave rise to an explosive growth of biological data with an increasing number of biological databases and tools developed to assist human research. Here we present a review from the time of gene discovery to the present day of human gene databases and compare them on the basis of their usability. As of 2017, well over one million human genomes have been sequenced worldwide. In the realm of current advancements, genetic and disease-related databases have appeared at a great pace. These repositories have been developed both academically and commercially. They are based on an array of resources: referenced literature and gene, protein and mutation databases. We explored all databases that claim to perform gene-to-disease searches for analysis. Most of the databases focus on collecting and storing different types of data, rather than on how to present data to users directionally and succinctly, so we turned our attention to some of the tools available for better annotation. We also investigated all the tools available that offer gene-to-disease searches, especially those taking multiple gene input. A basic feature-based comparison for all the selected tools and databases is presented in Table 3.
Type . | Name . | Types . | Data sources . | Gene capacity . | Latest update . | Search results . | User friendly . |
---|---|---|---|---|---|---|---|
Databases | ClinVar | Relationships among variations and human disorders | Clinical testing; research; extraction from the literature | 26 000 genes | 2018 | Variation; genes; conditions; clinical significance; review status | Yes |
CNVD | Copy number variations and related diseases | PubMed; OMIM; Ensembl; CNV Project; DGV; and HGSVP | 46 348 genes | 2014 | Species; Chr; position; region; disease; PMID | Yes | |
Disease Ontology | Human disease ontology | MeSH; ICD; NCI’s thesaurus; SNOMED and OMIM | Not found | 2018 | DOID; disease name | Yes | |
DiseaseEnhancer | Disease-associated enhancers | 1866 publications | 308 genes | 2018 | ID;Chr; position; disease; target gene | Yes | |
DISEASES | Disease–gene associations; cancer mutations; genome-wide association studies | GHR; UniProtKB; GWAS results from DistiLD and COSMIC | 17 606 genes | 2018 | Genes; identifiters; disease name; scores; confidencity; Publication | No | |
GARD | Rare diseases and related terms | NIH coordinates research and information | Not found | 2018 | Diseases list | Yes | |
GTR | Genetic test information by providers | ClinVar; Genetics & Medicine; GeneReviews; MedGen; OMIM; Orphanet; NHGRI Glossary | 16 451 genes | 2018 | Associated conditions; copy number response; genomic context; variation; related articles in PubMed | Yes | |
miR2Disease | miRNA deregulation in human diseases | Researchers submission; PubMed text-mining | 349 miRNAs | 2008 | miRNA; disease; relationship type; target gene; reference | No | |
Orphanet | Rare diseases and orphan drugs | OMIM; ICD10; MeSH; MedDRA; GARD; and UMLS | 15 470 genes | 2018 | Genes; disease; ORPHA ID; ICD | No | |
Tools | DisGeNET | Genotype–phenotype relationships | CTD; UniProt; ClinVar; Orphanet; RGD; MGD; GAD | 17 381 genes | 2018 | Gene; disease; disease class; semantic type; PMIDs; SNPs | No |
HGMD | Germline mutations | GDB; OMIM; 250 journals | 6 662 genes | 2017 | Disease/phenotype; number of mutations; gene symbol | Yes | |
Gene2Function | Orthologs among human genes and common genetic model species | OMIM; EBI; GWAS; HGNC; MOD; DIOPT; GO; SGD; ORF; NCBI; PDB; Uniprot | 10 499 genes | 2017 | Gene ID; gene symbol; human disease; species name… | No | |
SwissVar | SAPs and diseases | Disease:UniProtKB/SwissProt and their mapping to MeSH terms; variant:ModSNP database | Not found | Not found | Accession; disease; variant | Yes | |
eDGAR | Gene–disease relationships | OMIM; Humsavar; ClinVa | 3 658 genes | 2016 | gene; number of associated diseases; associated diseases identifiers; associated diseases names | Yes | |
GeneAlaCart | Gene; genomics; proteins; gene ontology; pathways; drugs; diseases | 76 sources https://www.malacards.org/pages/info#whats_in_a_malacard | 71 150 genes | 2018 | Symbol; aliases; disorder; score; Is Cancer Census; sources | Yes |
Type . | Name . | Types . | Data sources . | Gene capacity . | Latest update . | Search results . | User friendly . |
---|---|---|---|---|---|---|---|
Databases | ClinVar | Relationships among variations and human disorders | Clinical testing; research; extraction from the literature | 26 000 genes | 2018 | Variation; genes; conditions; clinical significance; review status | Yes |
CNVD | Copy number variations and related diseases | PubMed; OMIM; Ensembl; CNV Project; DGV; and HGSVP | 46 348 genes | 2014 | Species; Chr; position; region; disease; PMID | Yes | |
Disease Ontology | Human disease ontology | MeSH; ICD; NCI’s thesaurus; SNOMED and OMIM | Not found | 2018 | DOID; disease name | Yes | |
DiseaseEnhancer | Disease-associated enhancers | 1866 publications | 308 genes | 2018 | ID;Chr; position; disease; target gene | Yes | |
DISEASES | Disease–gene associations; cancer mutations; genome-wide association studies | GHR; UniProtKB; GWAS results from DistiLD and COSMIC | 17 606 genes | 2018 | Genes; identifiters; disease name; scores; confidencity; Publication | No | |
GARD | Rare diseases and related terms | NIH coordinates research and information | Not found | 2018 | Diseases list | Yes | |
GTR | Genetic test information by providers | ClinVar; Genetics & Medicine; GeneReviews; MedGen; OMIM; Orphanet; NHGRI Glossary | 16 451 genes | 2018 | Associated conditions; copy number response; genomic context; variation; related articles in PubMed | Yes | |
miR2Disease | miRNA deregulation in human diseases | Researchers submission; PubMed text-mining | 349 miRNAs | 2008 | miRNA; disease; relationship type; target gene; reference | No | |
Orphanet | Rare diseases and orphan drugs | OMIM; ICD10; MeSH; MedDRA; GARD; and UMLS | 15 470 genes | 2018 | Genes; disease; ORPHA ID; ICD | No | |
Tools | DisGeNET | Genotype–phenotype relationships | CTD; UniProt; ClinVar; Orphanet; RGD; MGD; GAD | 17 381 genes | 2018 | Gene; disease; disease class; semantic type; PMIDs; SNPs | No |
HGMD | Germline mutations | GDB; OMIM; 250 journals | 6 662 genes | 2017 | Disease/phenotype; number of mutations; gene symbol | Yes | |
Gene2Function | Orthologs among human genes and common genetic model species | OMIM; EBI; GWAS; HGNC; MOD; DIOPT; GO; SGD; ORF; NCBI; PDB; Uniprot | 10 499 genes | 2017 | Gene ID; gene symbol; human disease; species name… | No | |
SwissVar | SAPs and diseases | Disease:UniProtKB/SwissProt and their mapping to MeSH terms; variant:ModSNP database | Not found | Not found | Accession; disease; variant | Yes | |
eDGAR | Gene–disease relationships | OMIM; Humsavar; ClinVa | 3 658 genes | 2016 | gene; number of associated diseases; associated diseases identifiers; associated diseases names | Yes | |
GeneAlaCart | Gene; genomics; proteins; gene ontology; pathways; drugs; diseases | 76 sources https://www.malacards.org/pages/info#whats_in_a_malacard | 71 150 genes | 2018 | Symbol; aliases; disorder; score; Is Cancer Census; sources | Yes |
Type . | Name . | Types . | Data sources . | Gene capacity . | Latest update . | Search results . | User friendly . |
---|---|---|---|---|---|---|---|
Databases | ClinVar | Relationships among variations and human disorders | Clinical testing; research; extraction from the literature | 26 000 genes | 2018 | Variation; genes; conditions; clinical significance; review status | Yes |
CNVD | Copy number variations and related diseases | PubMed; OMIM; Ensembl; CNV Project; DGV; and HGSVP | 46 348 genes | 2014 | Species; Chr; position; region; disease; PMID | Yes | |
Disease Ontology | Human disease ontology | MeSH; ICD; NCI’s thesaurus; SNOMED and OMIM | Not found | 2018 | DOID; disease name | Yes | |
DiseaseEnhancer | Disease-associated enhancers | 1866 publications | 308 genes | 2018 | ID;Chr; position; disease; target gene | Yes | |
DISEASES | Disease–gene associations; cancer mutations; genome-wide association studies | GHR; UniProtKB; GWAS results from DistiLD and COSMIC | 17 606 genes | 2018 | Genes; identifiters; disease name; scores; confidencity; Publication | No | |
GARD | Rare diseases and related terms | NIH coordinates research and information | Not found | 2018 | Diseases list | Yes | |
GTR | Genetic test information by providers | ClinVar; Genetics & Medicine; GeneReviews; MedGen; OMIM; Orphanet; NHGRI Glossary | 16 451 genes | 2018 | Associated conditions; copy number response; genomic context; variation; related articles in PubMed | Yes | |
miR2Disease | miRNA deregulation in human diseases | Researchers submission; PubMed text-mining | 349 miRNAs | 2008 | miRNA; disease; relationship type; target gene; reference | No | |
Orphanet | Rare diseases and orphan drugs | OMIM; ICD10; MeSH; MedDRA; GARD; and UMLS | 15 470 genes | 2018 | Genes; disease; ORPHA ID; ICD | No | |
Tools | DisGeNET | Genotype–phenotype relationships | CTD; UniProt; ClinVar; Orphanet; RGD; MGD; GAD | 17 381 genes | 2018 | Gene; disease; disease class; semantic type; PMIDs; SNPs | No |
HGMD | Germline mutations | GDB; OMIM; 250 journals | 6 662 genes | 2017 | Disease/phenotype; number of mutations; gene symbol | Yes | |
Gene2Function | Orthologs among human genes and common genetic model species | OMIM; EBI; GWAS; HGNC; MOD; DIOPT; GO; SGD; ORF; NCBI; PDB; Uniprot | 10 499 genes | 2017 | Gene ID; gene symbol; human disease; species name… | No | |
SwissVar | SAPs and diseases | Disease:UniProtKB/SwissProt and their mapping to MeSH terms; variant:ModSNP database | Not found | Not found | Accession; disease; variant | Yes | |
eDGAR | Gene–disease relationships | OMIM; Humsavar; ClinVa | 3 658 genes | 2016 | gene; number of associated diseases; associated diseases identifiers; associated diseases names | Yes | |
GeneAlaCart | Gene; genomics; proteins; gene ontology; pathways; drugs; diseases | 76 sources https://www.malacards.org/pages/info#whats_in_a_malacard | 71 150 genes | 2018 | Symbol; aliases; disorder; score; Is Cancer Census; sources | Yes |
Type . | Name . | Types . | Data sources . | Gene capacity . | Latest update . | Search results . | User friendly . |
---|---|---|---|---|---|---|---|
Databases | ClinVar | Relationships among variations and human disorders | Clinical testing; research; extraction from the literature | 26 000 genes | 2018 | Variation; genes; conditions; clinical significance; review status | Yes |
CNVD | Copy number variations and related diseases | PubMed; OMIM; Ensembl; CNV Project; DGV; and HGSVP | 46 348 genes | 2014 | Species; Chr; position; region; disease; PMID | Yes | |
Disease Ontology | Human disease ontology | MeSH; ICD; NCI’s thesaurus; SNOMED and OMIM | Not found | 2018 | DOID; disease name | Yes | |
DiseaseEnhancer | Disease-associated enhancers | 1866 publications | 308 genes | 2018 | ID;Chr; position; disease; target gene | Yes | |
DISEASES | Disease–gene associations; cancer mutations; genome-wide association studies | GHR; UniProtKB; GWAS results from DistiLD and COSMIC | 17 606 genes | 2018 | Genes; identifiters; disease name; scores; confidencity; Publication | No | |
GARD | Rare diseases and related terms | NIH coordinates research and information | Not found | 2018 | Diseases list | Yes | |
GTR | Genetic test information by providers | ClinVar; Genetics & Medicine; GeneReviews; MedGen; OMIM; Orphanet; NHGRI Glossary | 16 451 genes | 2018 | Associated conditions; copy number response; genomic context; variation; related articles in PubMed | Yes | |
miR2Disease | miRNA deregulation in human diseases | Researchers submission; PubMed text-mining | 349 miRNAs | 2008 | miRNA; disease; relationship type; target gene; reference | No | |
Orphanet | Rare diseases and orphan drugs | OMIM; ICD10; MeSH; MedDRA; GARD; and UMLS | 15 470 genes | 2018 | Genes; disease; ORPHA ID; ICD | No | |
Tools | DisGeNET | Genotype–phenotype relationships | CTD; UniProt; ClinVar; Orphanet; RGD; MGD; GAD | 17 381 genes | 2018 | Gene; disease; disease class; semantic type; PMIDs; SNPs | No |
HGMD | Germline mutations | GDB; OMIM; 250 journals | 6 662 genes | 2017 | Disease/phenotype; number of mutations; gene symbol | Yes | |
Gene2Function | Orthologs among human genes and common genetic model species | OMIM; EBI; GWAS; HGNC; MOD; DIOPT; GO; SGD; ORF; NCBI; PDB; Uniprot | 10 499 genes | 2017 | Gene ID; gene symbol; human disease; species name… | No | |
SwissVar | SAPs and diseases | Disease:UniProtKB/SwissProt and their mapping to MeSH terms; variant:ModSNP database | Not found | Not found | Accession; disease; variant | Yes | |
eDGAR | Gene–disease relationships | OMIM; Humsavar; ClinVa | 3 658 genes | 2016 | gene; number of associated diseases; associated diseases identifiers; associated diseases names | Yes | |
GeneAlaCart | Gene; genomics; proteins; gene ontology; pathways; drugs; diseases | 76 sources https://www.malacards.org/pages/info#whats_in_a_malacard | 71 150 genes | 2018 | Symbol; aliases; disorder; score; Is Cancer Census; sources | Yes |
We discussed nine databases and six tools outlining the concept behind the gene–disease feature presented by them. The pathway leading from genes to disease varies depending on the type of data. Our focus was on data types and sources as well as the presentation of results. Some databases are based on simple literature sites, such as OMIM. These databases do not intuitively give corresponding disease information, but require users to constantly shuttle between different pages and options until they find the most appropriate results. Not all databases and tools specifically focus on the relationship between genes and diseases. We found DISEASES, eDGAR and GeneAlaCart to directly give relevant disease output. Other databases, however, discussed the relationship between genes and disease but only indirectly. ClinVar uses variations to bridge related genes and diseases; in CNVD, copy number variations are used; miRNAs are used by miR2Disease; enhancers used by DiseaseEnhancer; germline mutations are the focus of HGMD; and protein variants by SwissVar. GARD and Orphanet are centered on rare diseases; and DO covers human disease ontologies.
When collecting the data sources, gene capacity, along with the last maintenance update year, we noticed that some databases have not been updated in the past few years and some have very limited data (e.g. over a few hundred or a thousand genes). Most databases or tools have multiple sources, which at times prove to be a double-edged sword. On one hand, more data sources enrich the capacity of the databases; on the other hand, too many data sources may make it difficult to avoid entry of uncertain or erroneous data. Finally, we focused on the initial search output and user-friendly interface. Many tools have tried to simplify searches, but because of complexity, compromises on result quality were made. To establish our viewpoint, we performed gene-based test searches of five genes across all the databases and tools we discussed here in detail (Table 4).
Type . | Name . | BRCA1 . | GABRG2 . | OR4F29 . | C19orf80 . | RP11-167P11.3 . |
---|---|---|---|---|---|---|
Databases | ClinVar | Hereditary cancer-predisposing syndrome; familial breast–ovarian cancer; hereditary breast and ovarian cancer syndrome; neoplasm of the breast | Childhood absence epilepsy; familial febrile seizures; severe myoclonic epilepsy in infancy; generalized epilepsy with febrile seizures plus | No results | No results | No results |
CNVD | Breast cancer; ovarian cancer; pancreatic cancer | Breast cancer; ovarian cancer; pancreatic cancer; myelofibrosis | Breast cancer; ovarian cancer; schizophrenia | No results | No results | |
Disease Ontology | Hereditary breast and ovarian cancer | No results | No results | No results | ||
DiseaseEnhancer | Breast cancer | No results | No results | No results | No results | |
DISEASES | Prostate cancer; breast cancer; ovarian cancer; fanconi anemia | Generalized epilepsy with febrile seizures plus; childhood absence epilepsy | Hepatocellular carcinoma | Diabetes mellitus | No results | |
GARD | Hereditary breast and ovarian cancer syndrome; familial breast cancer; ovarian cancer; familial prostate cancer; familial pancreatic cancer | Epilepsy with myoclonic-atonic seizures | No results | No results | No results | |
GTR | Familial breast–ovarian cancer; familial cancer of breast; fanconi anemia complementary group S; hereditary breast and ovarian cancer syndrome; pancreatic cancer | Childhood absence epilepsy; familial febrile seizures | No results | No results | No results | |
miR2Disease | Ovarian disease; breast cancer | No results | No results | No results | No results | |
Orphanet | Lethal neonatal spasticity-epileptic encephalopathy syndrome; hereditary breast and ovarian cancer syndrome; uveal melanoma; familial melanoma; fanconi anemia; familial pancreatic carcinoma; familial prostate cancer; primary peritoneal carcinoma | Dravet syndrome; generalized epilepsy with febrile seizures-plus; rolandic epilepsy; childhood absence epilepsy | No results | No results | No results | |
Tools | DisGeNET | Malignant neoplasm of breast; familial breast–ovarian cancer; hereditary breast and ovarian cancer syndrome; mammary neoplasms; ovarian neoplasms; familial breast cancer; breast carcinoma; prostate neoplasms | Febrile convulsions; chronic alcoholic intoxication; absence epilepsy; myoclonic epilepsies; generalized epilepsy with febrile seizures plus; hepatic encephalopathy; schizophrenia | No results | No results | No results |
HGMD | Breast cancer; ovarian cancer; ovarian carcinoma | Generalized epilepsy with febrile seizures plus; epilepsy, childhood absence with febrile seizures; idiopathic generalized epilepsy | No results | No results | No results | |
Gene2Function | Pancreatic cancer; familial breast–ovarian cancer | Generalized epilepsy with febrile seizures plus; childhood absence epilepsy; familial febrile seizures; post bronchodilator; cognitive impairment; severe influenza A | Subjective response to lithium treatment | HDL cholesterol | No results | |
SwissVar | Breast cancer; familial breast–ovarian cancer, ovarian cancer; pancreatic cancer, fanconi anemia, complementation group S | Childhood absence epilepsy; familial febrile seizures; generalized epilepsy with febrile seizures plus | No results | Insulin-dependent diabetes mellitus; non-insulin-dependent diabetes mellitus | No results | |
eDGAR | Breast cancer; ovarian cancer; pancreatic cancer; familial breast–ovarian cancer; fanconi anemia | Childhood absence epilepsy; familial febrile seizures; generalized epilepsy with febrile seizures plus | No results | No results | No results | |
GeneAlaCart | Lynch syndrome; breast cancer; colorectal cancer | Generalized idiopathic epilepsy; Angelman syndrome; febrile seizures | No results | Diabetes mellitus; breast sarcoma; insulin-dependent diabetes mellitus | No results |
Type . | Name . | BRCA1 . | GABRG2 . | OR4F29 . | C19orf80 . | RP11-167P11.3 . |
---|---|---|---|---|---|---|
Databases | ClinVar | Hereditary cancer-predisposing syndrome; familial breast–ovarian cancer; hereditary breast and ovarian cancer syndrome; neoplasm of the breast | Childhood absence epilepsy; familial febrile seizures; severe myoclonic epilepsy in infancy; generalized epilepsy with febrile seizures plus | No results | No results | No results |
CNVD | Breast cancer; ovarian cancer; pancreatic cancer | Breast cancer; ovarian cancer; pancreatic cancer; myelofibrosis | Breast cancer; ovarian cancer; schizophrenia | No results | No results | |
Disease Ontology | Hereditary breast and ovarian cancer | No results | No results | No results | ||
DiseaseEnhancer | Breast cancer | No results | No results | No results | No results | |
DISEASES | Prostate cancer; breast cancer; ovarian cancer; fanconi anemia | Generalized epilepsy with febrile seizures plus; childhood absence epilepsy | Hepatocellular carcinoma | Diabetes mellitus | No results | |
GARD | Hereditary breast and ovarian cancer syndrome; familial breast cancer; ovarian cancer; familial prostate cancer; familial pancreatic cancer | Epilepsy with myoclonic-atonic seizures | No results | No results | No results | |
GTR | Familial breast–ovarian cancer; familial cancer of breast; fanconi anemia complementary group S; hereditary breast and ovarian cancer syndrome; pancreatic cancer | Childhood absence epilepsy; familial febrile seizures | No results | No results | No results | |
miR2Disease | Ovarian disease; breast cancer | No results | No results | No results | No results | |
Orphanet | Lethal neonatal spasticity-epileptic encephalopathy syndrome; hereditary breast and ovarian cancer syndrome; uveal melanoma; familial melanoma; fanconi anemia; familial pancreatic carcinoma; familial prostate cancer; primary peritoneal carcinoma | Dravet syndrome; generalized epilepsy with febrile seizures-plus; rolandic epilepsy; childhood absence epilepsy | No results | No results | No results | |
Tools | DisGeNET | Malignant neoplasm of breast; familial breast–ovarian cancer; hereditary breast and ovarian cancer syndrome; mammary neoplasms; ovarian neoplasms; familial breast cancer; breast carcinoma; prostate neoplasms | Febrile convulsions; chronic alcoholic intoxication; absence epilepsy; myoclonic epilepsies; generalized epilepsy with febrile seizures plus; hepatic encephalopathy; schizophrenia | No results | No results | No results |
HGMD | Breast cancer; ovarian cancer; ovarian carcinoma | Generalized epilepsy with febrile seizures plus; epilepsy, childhood absence with febrile seizures; idiopathic generalized epilepsy | No results | No results | No results | |
Gene2Function | Pancreatic cancer; familial breast–ovarian cancer | Generalized epilepsy with febrile seizures plus; childhood absence epilepsy; familial febrile seizures; post bronchodilator; cognitive impairment; severe influenza A | Subjective response to lithium treatment | HDL cholesterol | No results | |
SwissVar | Breast cancer; familial breast–ovarian cancer, ovarian cancer; pancreatic cancer, fanconi anemia, complementation group S | Childhood absence epilepsy; familial febrile seizures; generalized epilepsy with febrile seizures plus | No results | Insulin-dependent diabetes mellitus; non-insulin-dependent diabetes mellitus | No results | |
eDGAR | Breast cancer; ovarian cancer; pancreatic cancer; familial breast–ovarian cancer; fanconi anemia | Childhood absence epilepsy; familial febrile seizures; generalized epilepsy with febrile seizures plus | No results | No results | No results | |
GeneAlaCart | Lynch syndrome; breast cancer; colorectal cancer | Generalized idiopathic epilepsy; Angelman syndrome; febrile seizures | No results | Diabetes mellitus; breast sarcoma; insulin-dependent diabetes mellitus | No results |
Type . | Name . | BRCA1 . | GABRG2 . | OR4F29 . | C19orf80 . | RP11-167P11.3 . |
---|---|---|---|---|---|---|
Databases | ClinVar | Hereditary cancer-predisposing syndrome; familial breast–ovarian cancer; hereditary breast and ovarian cancer syndrome; neoplasm of the breast | Childhood absence epilepsy; familial febrile seizures; severe myoclonic epilepsy in infancy; generalized epilepsy with febrile seizures plus | No results | No results | No results |
CNVD | Breast cancer; ovarian cancer; pancreatic cancer | Breast cancer; ovarian cancer; pancreatic cancer; myelofibrosis | Breast cancer; ovarian cancer; schizophrenia | No results | No results | |
Disease Ontology | Hereditary breast and ovarian cancer | No results | No results | No results | ||
DiseaseEnhancer | Breast cancer | No results | No results | No results | No results | |
DISEASES | Prostate cancer; breast cancer; ovarian cancer; fanconi anemia | Generalized epilepsy with febrile seizures plus; childhood absence epilepsy | Hepatocellular carcinoma | Diabetes mellitus | No results | |
GARD | Hereditary breast and ovarian cancer syndrome; familial breast cancer; ovarian cancer; familial prostate cancer; familial pancreatic cancer | Epilepsy with myoclonic-atonic seizures | No results | No results | No results | |
GTR | Familial breast–ovarian cancer; familial cancer of breast; fanconi anemia complementary group S; hereditary breast and ovarian cancer syndrome; pancreatic cancer | Childhood absence epilepsy; familial febrile seizures | No results | No results | No results | |
miR2Disease | Ovarian disease; breast cancer | No results | No results | No results | No results | |
Orphanet | Lethal neonatal spasticity-epileptic encephalopathy syndrome; hereditary breast and ovarian cancer syndrome; uveal melanoma; familial melanoma; fanconi anemia; familial pancreatic carcinoma; familial prostate cancer; primary peritoneal carcinoma | Dravet syndrome; generalized epilepsy with febrile seizures-plus; rolandic epilepsy; childhood absence epilepsy | No results | No results | No results | |
Tools | DisGeNET | Malignant neoplasm of breast; familial breast–ovarian cancer; hereditary breast and ovarian cancer syndrome; mammary neoplasms; ovarian neoplasms; familial breast cancer; breast carcinoma; prostate neoplasms | Febrile convulsions; chronic alcoholic intoxication; absence epilepsy; myoclonic epilepsies; generalized epilepsy with febrile seizures plus; hepatic encephalopathy; schizophrenia | No results | No results | No results |
HGMD | Breast cancer; ovarian cancer; ovarian carcinoma | Generalized epilepsy with febrile seizures plus; epilepsy, childhood absence with febrile seizures; idiopathic generalized epilepsy | No results | No results | No results | |
Gene2Function | Pancreatic cancer; familial breast–ovarian cancer | Generalized epilepsy with febrile seizures plus; childhood absence epilepsy; familial febrile seizures; post bronchodilator; cognitive impairment; severe influenza A | Subjective response to lithium treatment | HDL cholesterol | No results | |
SwissVar | Breast cancer; familial breast–ovarian cancer, ovarian cancer; pancreatic cancer, fanconi anemia, complementation group S | Childhood absence epilepsy; familial febrile seizures; generalized epilepsy with febrile seizures plus | No results | Insulin-dependent diabetes mellitus; non-insulin-dependent diabetes mellitus | No results | |
eDGAR | Breast cancer; ovarian cancer; pancreatic cancer; familial breast–ovarian cancer; fanconi anemia | Childhood absence epilepsy; familial febrile seizures; generalized epilepsy with febrile seizures plus | No results | No results | No results | |
GeneAlaCart | Lynch syndrome; breast cancer; colorectal cancer | Generalized idiopathic epilepsy; Angelman syndrome; febrile seizures | No results | Diabetes mellitus; breast sarcoma; insulin-dependent diabetes mellitus | No results |
Type . | Name . | BRCA1 . | GABRG2 . | OR4F29 . | C19orf80 . | RP11-167P11.3 . |
---|---|---|---|---|---|---|
Databases | ClinVar | Hereditary cancer-predisposing syndrome; familial breast–ovarian cancer; hereditary breast and ovarian cancer syndrome; neoplasm of the breast | Childhood absence epilepsy; familial febrile seizures; severe myoclonic epilepsy in infancy; generalized epilepsy with febrile seizures plus | No results | No results | No results |
CNVD | Breast cancer; ovarian cancer; pancreatic cancer | Breast cancer; ovarian cancer; pancreatic cancer; myelofibrosis | Breast cancer; ovarian cancer; schizophrenia | No results | No results | |
Disease Ontology | Hereditary breast and ovarian cancer | No results | No results | No results | ||
DiseaseEnhancer | Breast cancer | No results | No results | No results | No results | |
DISEASES | Prostate cancer; breast cancer; ovarian cancer; fanconi anemia | Generalized epilepsy with febrile seizures plus; childhood absence epilepsy | Hepatocellular carcinoma | Diabetes mellitus | No results | |
GARD | Hereditary breast and ovarian cancer syndrome; familial breast cancer; ovarian cancer; familial prostate cancer; familial pancreatic cancer | Epilepsy with myoclonic-atonic seizures | No results | No results | No results | |
GTR | Familial breast–ovarian cancer; familial cancer of breast; fanconi anemia complementary group S; hereditary breast and ovarian cancer syndrome; pancreatic cancer | Childhood absence epilepsy; familial febrile seizures | No results | No results | No results | |
miR2Disease | Ovarian disease; breast cancer | No results | No results | No results | No results | |
Orphanet | Lethal neonatal spasticity-epileptic encephalopathy syndrome; hereditary breast and ovarian cancer syndrome; uveal melanoma; familial melanoma; fanconi anemia; familial pancreatic carcinoma; familial prostate cancer; primary peritoneal carcinoma | Dravet syndrome; generalized epilepsy with febrile seizures-plus; rolandic epilepsy; childhood absence epilepsy | No results | No results | No results | |
Tools | DisGeNET | Malignant neoplasm of breast; familial breast–ovarian cancer; hereditary breast and ovarian cancer syndrome; mammary neoplasms; ovarian neoplasms; familial breast cancer; breast carcinoma; prostate neoplasms | Febrile convulsions; chronic alcoholic intoxication; absence epilepsy; myoclonic epilepsies; generalized epilepsy with febrile seizures plus; hepatic encephalopathy; schizophrenia | No results | No results | No results |
HGMD | Breast cancer; ovarian cancer; ovarian carcinoma | Generalized epilepsy with febrile seizures plus; epilepsy, childhood absence with febrile seizures; idiopathic generalized epilepsy | No results | No results | No results | |
Gene2Function | Pancreatic cancer; familial breast–ovarian cancer | Generalized epilepsy with febrile seizures plus; childhood absence epilepsy; familial febrile seizures; post bronchodilator; cognitive impairment; severe influenza A | Subjective response to lithium treatment | HDL cholesterol | No results | |
SwissVar | Breast cancer; familial breast–ovarian cancer, ovarian cancer; pancreatic cancer, fanconi anemia, complementation group S | Childhood absence epilepsy; familial febrile seizures; generalized epilepsy with febrile seizures plus | No results | Insulin-dependent diabetes mellitus; non-insulin-dependent diabetes mellitus | No results | |
eDGAR | Breast cancer; ovarian cancer; pancreatic cancer; familial breast–ovarian cancer; fanconi anemia | Childhood absence epilepsy; familial febrile seizures; generalized epilepsy with febrile seizures plus | No results | No results | No results | |
GeneAlaCart | Lynch syndrome; breast cancer; colorectal cancer | Generalized idiopathic epilepsy; Angelman syndrome; febrile seizures | No results | Diabetes mellitus; breast sarcoma; insulin-dependent diabetes mellitus | No results |
The first gene we tested was breast cancer 1 (BRCA1), which has been studied since the 1990s. This gene is associated with breast and other cancers, has been widely studied and is relatively well understood. We compared the gene results of BRCA1 searches from each database and every database and tool generated corresponding results. The next gene we tested was GABRG2, which encodes Gamma-aminobutyric acid receptor subunit gamma-2. GABA is an important inhibitory neurotransmitter in the brain. Most of the databases and tools contained disease information for GABRG2, but three databases did not include this gene. The third gene, OR4F29, encodes the olfactory receptor family 4 subfamily F member 29 involved in an olfactory signaling pathway. The fourth gene tested is C19orf80, which may be more recognizable by its alias, ANGPTL8; the last gene is RP11-167P11.3, which codes for a novel protein recently found to be involved in a primary immunodeficiency disorder [179]. Although the last three genes have been reported in the literature for their relationship with disease, their search results in most of the databases were not positive. Only three databases can recognize C19orf80 by the alias name, AN3GPTL8, and none of the databases contain information about RP11-167P11.3. The last three genes tested are absent from DisGeNET, HGMD and eDGAR databases. Even though GeneAlaCart utilizes the most extensive companion databases in the GeneCardsSuite, it still has some inconsistencies (Supplementary Data, Figure 15). OR4F29 has no related disease record in MalaCards. However, we were able to find results for this gene in other repositories. For some genes, such as PVRL4, a manual search in MalaCards output relevant diseases, but when uploaded directly in GeneAlaCart, it does not generate any related disease. Thirdly, some disease-related protein coding genes are completely missing in some databases. For example, AC004381.6, is found to be related to breast cancer in the literature, but there was no disease-related information available in GeneCardsSuite. The output contains incomplete information. This affirms that there is still a lot of missing data in the current database, even though they claim to be updated every year.
This review is meant to provide an overview of the current status of gene–disease association data in public resources to overcome the difficulties users face when searching for specific disease–gene information. All databases presented are great resources for retrieving human gene annotation data. However, as soon as a query gets more specific and complicated, an integrative approach becomes necessary. Currently, researchers typically have to browse and search several databases to obtain the required information. These databases are based on many literature, gene, protein and mutation databases but not all databases annotate genes with their Ensembl gene ID [180, 181]. Most importantly, there is no repository that can directly link genes to the currently used International Statistical Classification of Diseases and Related Health Problems codes based on their disease phenotype. Relation to an accurate ICD code can point to more accurate treatments toward diseases.
Mapping the genes to their diseases and, at the same time, mapping the diseases to their respective ICD codes will provide a crosscut to find drugs and the therapeutics for specific patients. As genetic variant data accumulates at exponential scales, it has become fundamentally essential to make it available for healthcare setting to map on the clinical data. With the growing trend and demand for adapting these databases into biomedical research, there is a crucial need to develop integrated, standard, and curated platform to support the clinical decision making process [182, 183]. There are reference gene annotation databases like GenCode and Ensembl but no such standard exists for gene–disease associations [181, 184]. There is no resource available in literature or database reported, which can annotate multiple genes to WHO classified disease phenotype codes.
Creating a database with smart distillation and abundant distribution of authentic genes linked to classified diseases through their description and IDs (e.g. ICD-9 and ICD-10 codes) can support both clinical and research environments. Such a database must not be redundant and should only include human reference genome and disease based information collected from valid sources. It’s very important to facilitate interested users with efficient, user friendly, easy navigation and free portable access to the database using platforms that have proven to be efficient tools in several areas including healthcare, e.g. smart devices and iOS- and Android-based applications. Effectiveness of developing a platform by mapping diseased genes to medical records can facilitate precision medicine via predictive diagnostic, precise assessment and management with customized treatments and tailored medications [185–191]. It will not only produce meaningful health outcomes but also revolutionize healthcare by integrating metabolomics (metabolites) [192], transcriptomics (RNA), proteomics (proteins) and epigenomics to reveal the functional significance of genomic variations [193–198].
This is the era of big data, where human-related biological databases continue to grow not only in count but also in volume, posing unprecedented challenges in data storage, processing, exchange and curation. NGS advancements have made it possible to sequence single cell [199–207] and produce long reads using PacBio [208–215], Oxford Nanopore [215–222] and Illumina technologies using 10× Genomics Kits [3, 223–229]. Adoption of NGS with WGS [230–236] in a diagnostic context has the potential to improve disease risk detection in support of precision medicine and discovery [185, 186]. Moreover, several bioinformatics pipelines have been developed to strengthen WGS variant interpretation by efficiently processing and analyzing sequenced data [237–244]. Many published results show how genomics can be proactively incorporated into medical practice and raise the clinical utilization of information in these databases [198, 245–250].
Conclusion
Despite many important genetic discoveries, the genetics of complex diseases like cancer remains far from clear. With a progressive understanding of the molecular and genomic factors at play in diseases, more effective medical treatments are anticipated for many disorders like cancer. The scientific approach would be to perform individualized analysis of individual genomes giving rise to a new form of preventive and personalized medicine in healthcare [251–264]. Availability of gene based designer drugs, precise targeting of molecular fingerprints for tumor, appropriate drug therapy, predicting individual susceptibility to disease, diagnosis and treatment of mental illness are all a few of the many transformations expected in the decade to come. To efficiently fathom the wealth of biological data, there is a crucial need to generate appropriate gene annotation repositories and resources.
Author contributions
Z.A. proposed and led the study and drafted the manuscript. S.Z. and R.X. conducted review research and evaluated reported databases, tools and technologies. B.L. guided and supported the study. All authors participated in writing and reviewing the manuscript.
Authentic and classified gene–disease data is highly significant at every level of biological research and healthcare.
To efficiently fathom the wealth of genomics and clinical data, there is a crucial need to develop appropriate gene–disease annotation repositories and resources.
Evolving gene–disease complexities need to be well addressed to better implement precision medicine worldwide.
Innovative and robust big data platforms are necessary to improve the quality of healthcare by intelligently analyzing and sharing clinical and genomics data for discovering new therapeutic targets and designing diagnostic tools.
Acknowledgements
We are grateful to the Ahmed laboratory, Department of Genetics and Genome Sciences, The Pat and Jim Calhoun Cardiology Center, School of Medicine, University of Connecticut Health Center and The Jackson Laboratory for Genomic Medicine. We appreciate all colleagues who provided insight and expertise that greatly assisted this research.
References
Author notes
Saman Zeeshan and Ruoyun Xiong are equally contributing first authors.