-
PDF
- Split View
-
Views
-
Cite
Cite
Jianjian Wang, Yuze Cao, Xiaoyu Lu, Tianfeng Wang, Shuang Li, Xiaotong Kong, Chunrui Bo, Jie Li, Xiaolong Wang, Heping Ma, Lei Li, Huixue Zhang, Shangwei Ning, Lihua Wang, MicroRNAs and nervous system diseases: network insights and computational challenges, Briefings in Bioinformatics, Volume 21, Issue 3, May 2020, Pages 863–875, https://doi.org/10.1093/bib/bbz032
- Share Icon Share
Abstract
The nervous system is one of the most complex biological systems, and nervous system disease (NSD) is a major cause of disability and mortality. Extensive evidence indicates that numerous dysregulated microRNAs (miRNAs) are involved in a broad spectrum of NSDs. A comprehensive review of miRNA-mediated regulatory will facilitate our understanding of miRNA dysregulation mechanisms in NSDs. In this work, we summarized currently available databases on miRNAs and NSDs, star NSD miRNAs, NSD spectrum width, miRNA spectrum width and the distribution of miRNAs in NSD sub-categories by reviewing approximately 1000 studies. In addition, we characterized miRNA–miRNA and NSD–NSD interactions from a network perspective based on miRNA–NSD benchmarking data sets. Furthermore, we summarized the regulatory principles of miRNAs in NSDs, including miRNA synergistic regulation in NSDs, miRNA modules and NSD modules. We also discussed computational challenges for identifying novel miRNAs in NSDs. Elucidating the roles of miRNAs in NSDs from a network perspective would not only improve our understanding of the precise mechanism underlying these complex diseases, but also provide novel insight into the development, diagnosis and treatment of NSDs.
Introduction
The nervous system (NS) is one of the most complex biological systems, comprising a large variety of cell types and sophisticated synaptic communications that function coordinately [1]. NSDs, a common class of disorders affecting the NS, are remarkably complex; although the precise molecular mechanisms underlying most NSDs remain poorly understood. For example, Alzheimer disease (AD) is the main cause of dementia; however, the exact pathogenesis of this disease remains unclear and no curative treatments are available [2]. Epilepsy is one of the most common NSDs globally, and it is characterized by recurrent seizures that generate abnormal and hypersynchronous neuronal activity in the brain [3]. In recent years, many studies have focused on exploring the mechanisms underlying the involvement of miRNAs in NSDs.
miRNAs (~22 nucleotide) represent a large family of functional, small, non-coding RNAs (ncRNAs) that repress target mRNA expression and are involved in a wide range of biological processes [4]. miRNAs are responsible for NS function including translation, RNA metabolism, gene regulation and development [5]. Aberrant expression of miRNAs has been implicated in a broad spectrum of NSDs. For example, Parkinson disease (PD) is a chronic neurodegenerative disorder, characterized by motor and nonmotor symptoms. Overexpressed miRNAs (miR-103a-3p, miR-30b-5p and miR-29a-3p) serve as potential biomarkers in a relatively large cohort of PD patients [6]. Glioblastoma (GBM) is the most common and lethal brain tumor. miRNAs play critical roles in the GBM process, including cellular proliferation, apoptosis, invasion, angiogenesis and stemness [7]. Emerging evidence indicates that a subset of immune-associated miRNAs including miR-132, miR-124 and miR-155 contribute to a wide range of neuroimmune diseases, such as multiple sclerosis (MS) and myasthenia gravis (MG) [8, 9].
Database . | N_NSDs . | N_NSD miRNAs . | N_miRNA–NSD associations . | Function . | URL . |
---|---|---|---|---|---|
NSDNA [13] | 109 | 1956 | 9160 | specifically experimentally supported associations about NSDs and ncRNAs | http://www.bio-bigdata.com/nsdna/ |
HMDD [14] | 57 | 272 | 1101 | experimentally supported human miRNA–disease associations | http://www.cuilab.cn/hmdd |
miR2Disease [15] | 26 | 179 | 450 | experimentally supported human miRNA–disease associations | http://www.mir2disease.org/ |
MNDR [16] | 123 | 1418 | 13648 | comprehensive database that provides experimental and predicted ncRNA–disease associations | www.rna-society.org/mndr/ |
PhenomiR [17] | 15 | 170 | 445 | experimentally supported human miRNA–disease associations and bioprocesses | http://mips.helmholtz-muenchen.de/phenomir |
MSDD [18] | 25 | 37 | 55 | experimentally supported human miRSNP–disease associations | http://www.bio-bigdata.com/msdd/ |
Database . | N_NSDs . | N_NSD miRNAs . | N_miRNA–NSD associations . | Function . | URL . |
---|---|---|---|---|---|
NSDNA [13] | 109 | 1956 | 9160 | specifically experimentally supported associations about NSDs and ncRNAs | http://www.bio-bigdata.com/nsdna/ |
HMDD [14] | 57 | 272 | 1101 | experimentally supported human miRNA–disease associations | http://www.cuilab.cn/hmdd |
miR2Disease [15] | 26 | 179 | 450 | experimentally supported human miRNA–disease associations | http://www.mir2disease.org/ |
MNDR [16] | 123 | 1418 | 13648 | comprehensive database that provides experimental and predicted ncRNA–disease associations | www.rna-society.org/mndr/ |
PhenomiR [17] | 15 | 170 | 445 | experimentally supported human miRNA–disease associations and bioprocesses | http://mips.helmholtz-muenchen.de/phenomir |
MSDD [18] | 25 | 37 | 55 | experimentally supported human miRSNP–disease associations | http://www.bio-bigdata.com/msdd/ |
N_NSDs represents the number of NSDs; N_NSD miRNAs represents the number of NSD-related miRNAs; N_miRNA–NSD associations represent the number of miRNA–NSD associations.
Database . | N_NSDs . | N_NSD miRNAs . | N_miRNA–NSD associations . | Function . | URL . |
---|---|---|---|---|---|
NSDNA [13] | 109 | 1956 | 9160 | specifically experimentally supported associations about NSDs and ncRNAs | http://www.bio-bigdata.com/nsdna/ |
HMDD [14] | 57 | 272 | 1101 | experimentally supported human miRNA–disease associations | http://www.cuilab.cn/hmdd |
miR2Disease [15] | 26 | 179 | 450 | experimentally supported human miRNA–disease associations | http://www.mir2disease.org/ |
MNDR [16] | 123 | 1418 | 13648 | comprehensive database that provides experimental and predicted ncRNA–disease associations | www.rna-society.org/mndr/ |
PhenomiR [17] | 15 | 170 | 445 | experimentally supported human miRNA–disease associations and bioprocesses | http://mips.helmholtz-muenchen.de/phenomir |
MSDD [18] | 25 | 37 | 55 | experimentally supported human miRSNP–disease associations | http://www.bio-bigdata.com/msdd/ |
Database . | N_NSDs . | N_NSD miRNAs . | N_miRNA–NSD associations . | Function . | URL . |
---|---|---|---|---|---|
NSDNA [13] | 109 | 1956 | 9160 | specifically experimentally supported associations about NSDs and ncRNAs | http://www.bio-bigdata.com/nsdna/ |
HMDD [14] | 57 | 272 | 1101 | experimentally supported human miRNA–disease associations | http://www.cuilab.cn/hmdd |
miR2Disease [15] | 26 | 179 | 450 | experimentally supported human miRNA–disease associations | http://www.mir2disease.org/ |
MNDR [16] | 123 | 1418 | 13648 | comprehensive database that provides experimental and predicted ncRNA–disease associations | www.rna-society.org/mndr/ |
PhenomiR [17] | 15 | 170 | 445 | experimentally supported human miRNA–disease associations and bioprocesses | http://mips.helmholtz-muenchen.de/phenomir |
MSDD [18] | 25 | 37 | 55 | experimentally supported human miRSNP–disease associations | http://www.bio-bigdata.com/msdd/ |
N_NSDs represents the number of NSDs; N_NSD miRNAs represents the number of NSD-related miRNAs; N_miRNA–NSD associations represent the number of miRNA–NSD associations.
To better understand the regulatory role of miRNAs in complex disease networks, researchers have constructed miRNA and disease networks and found some important principles underlying these networks. Xu et al. [10] developed a miRNA–miRNA synergistic network (MFSN) and showed that disease-related miRNAs have more synergism than non-disease miRNAs. Lu et al. [11] observed that most of the miRNAs sharing the same dysfunctions in diseases were located in the same cluster by constructing miRNA–disease networks. Chen et al. [12] integrated miRNA–miRNA and disease–disease similarity networks for predicting potential miRNA–disease associations. However, a systematic review of miRNA-mediated regulatory networks in the context of NSDs remains unavailable.
Here we first reviewed the functions of miRNAs in NSDs with respect to star miRNAs, NSD spectrum width (NSW), miRNA spectrum width and the distribution of miRNAs in NSD sub-categories. Then we displayed miRNA–miRNA and NSD–NSD networks and summarized the synergistic regulation of miRNAs in NSDs from a network perspective. Moreover, we provided important findings regarding miRNA modules, NSD modules and their interactions underlying the regulatory mechanism. The regulatory roles of miRNAs summarized in the present work will provide critical insight into the pathogenesis, diagnosis and therapeutic strategies for NSDs.
The roles of miRNAs in NSDs
In the past decade, miRNAs have been the most widely studied ncRNAs in NSDs. Extensive evidence indicates that aberrant expression and function of miRNAs contribute to various NSDs. There are currently valuable and high quality databases that help researchers acquire global insight into miRNAs in NSDs. We first summarized the currently available miRNA and NSD databases (Table 1) and then characterized the roles of miRNAs in NSDs based on these benchmark data sets.
Summary of databases on miRNAs and NSDs
NSDNA is a manually curated database that specifically provides experimentally supported ncRNA–NSD associations [13]. The current version of NSDNA recorded 9260 human miRNA–NSD entries, including 1956 miRNAs and 109 NSDs. HMDD v2.0 and miR2Disease are valuable resources for the study of miRNAs and human diseases and contain 1101 and 450 miRNA–NSD associations, respectively [14, 15]. MNDR v2.0 is a comprehensive database that provides experimental and predicted ncRNA–disease associations [16]. The current version of MNDR recorded 10641 experimental miRNA–NSD associations and 3007 predicted miRNA–NSD associations in Homo sapiens. PhenomiR is a database that provides experimentally supported human miRNA–disease associations and bioprocesses [17]. PhenomiR documents 445 miRNA–NSD associations. MSDD is a manually curated database that provides experimentally supported associations about miRNA polymorphisms (miRSNPs) and human diseases [18]. The current version of MSDD contains 55 miRSNP–NSD associations covering 37 miRSNPs and 25 NSDs.
Star miRNAs in NSDs
Accumulating evidence indicates that miRNAs are almost involved in every stage of neural development [19]. Because of the crucial roles of miRNAs in the NS, the dysfunction of miRNAs contributes to various NSDs. Some NSD-related miRNAs are considered star miRNAs because of their versatile roles, and they have been extensively studied in NSDs. In this paper, the star miRNA (SM) of a given NSD miRNA(i) was defined as |${SM}_i=\sqrt{d_i\ast {l}_i}$|. Here di is the number of NSDs associated with miRNA(i) and li is the number of studies about miRNA(i) in NSDs. SM is a parameter that evaluates the popularity of a miRNA in NSDs. A higher SM-value indicates a more popular miRNA. We summarized the top five star miRNAs for their pathological mechanisms underlying complex NSDs in the following paragraphs.
hsa-miR-21-5p
The associations between miR-21-5p and NSDs have been extensively studied, and miR-21-5p was found to be associated with a wide range of NSDs. miR-21-5p functions as an oncogene in brain tumors and is involved in tumor cell proliferation, activation and apoptosis [20]. Overexpression of miR-21-5p promotes GBM tumorigenesis by downregulating insulin-like growth factor-binding protein-3 (IGFBP3) and is associated with poor patient survival [21]. Emerging studies indicate that miR-21-5p is upregulated in activated immune cells and plays critical roles in the pathogenesis of autoimmune NSDs [22]. For example, miR-21-5p expression is significantly upregulated in the peripheral blood mononuclear cells of patients with MS [23]. miR-21-5p plays an essential role in protecting against hypoxia/ischemia-induced neuronal death and is significantly increased in ischemic stroke patients [24]. In addition, upregulated miR-21-5p is a biomarker for the diagnosis of traumatic brain injury [25].
hsa-miR-146a-5p
miR-146a-5p, a brain-enriched miRNA, plays a central role in both the NS and immune systems [8]. Aberrant expression of miR-146a-5p is correlated with the onset and prognosis of autoimmune NSDs, such as MS [26], MG [27] and neuromyelitis optica [28]. Emerging evidence suggests that there is a close association between miR-146a-5p and neurodegenerative diseases. miR-146a-5p is significantly upregulated in the brain of AD patients, and miR-146a-5p targeted interleukin-1 receptor-associated kinase-1(IRAK-1) coupled to the NF-κB-induced upregulation of IRAK-2 expression drives an extensively sustained inflammatory response in AD [29]. Recently, miR-146a-5p was shown to increase cell apoptosis by targeting low-density lipoprotein receptor-related protein-2(Lrp2) expression, leading to the reduction of Akt activation and induction of proapoptotic caspase-3 in AD [30]. Recent studies suggest the potential involvement of miR-146a-5p in the modulation of inflammatory and immune processes in epilepsy. For example, increased expression of miR-146a-5p upregulates the inflammatory factor IL-1β in epilepsy by downregulating complement factor H (CFH), a potent inhibitor of the amplification cascade of complement activation [31].
hsa-miR-124-3p
miR-124-3p, another brain-enriched miRNA preferentially expressed in neurons, is a key regulator of neurogenesis [32]. Impairment of neurogenesis is a possible cause for the onset of neurodegenerative disorders [33]. Moreover, miR-124-3p is involved in the regulation of signaling molecules involved in memory and synaptic plasticity [34]. These findings suggest that miR-124-3p contributes to the pathogenesis of neurodegenerative diseases. The pathology of AD is characterized by the accumulation of β-amyloid peptide (Aβ), which is proteolytically cleaved from the amyloid precursor protein (APP). miR-124-3p is involved in the fine-tuning of APP alternative splicing, affecting the production of neurotoxic Aβ [35]. Amyotrophic lateral sclerosis (ALS) is another progressive neurodegenerative disease characterized by degeneration of motor neurons, resulting in paralysis and death within several years from the onset of symptoms. miR-124-3p is downregulated in ALS patients and considered a molecular denominator for multiple forms of ALS [36]. miR-124-3p is also highly expressed in immune cells and mediates the development of neuroimmune disorders [37]. Ponomarev et al. [38] showed that increased miR-124-3p promotes microglia quiescence and suppresses MS in a mouse model by deactivating macrophages through the C/EBP-α-PU.1 pathway.
hsa-miR-20a-5p
miR-20a-5p is an oncomiR that is associated with the development of multiple cancers, including brain tumors. Overexpression of miR-20a-5p promotes glioma invasiveness by directly targeting the tissue inhibitor of metalloproteinases-2 (TIMP-2) [39]. miR-20a-5p is involved in the Sonic Hedgehog pathway in medulloblastoma, which is the most common malignant brain tumor in children [40]. In recent years, circulating miRNAs have drawn a great deal of attention as potential biomarkers for the diagnosis and prognosis of various NSDs. For example, elevated levels of serum miR-20a-5p are associated with poor survival in GBM patients [41]. Meanwhile, circulating miR-20a-5p is as promising marker to monitor carotid artery stenosis progression and may improve therapeutic strategies to prevent stroke [42]. Cheng et al. [43] suggested that serum miR-20a-5p is a potential peripheral monitoring factor in AD.
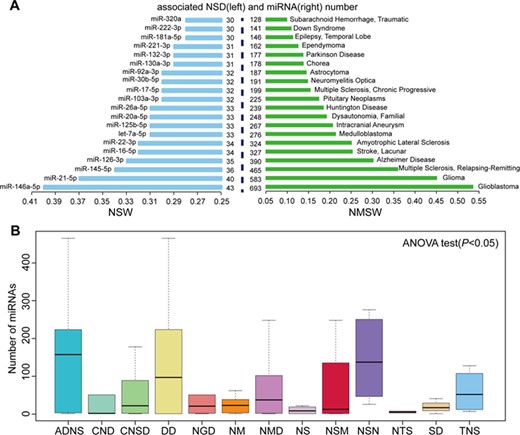
Summaries of the roles of miRNAs in NSDs. (A) The top 20 NSWs (left) and NMSWs (right). (B) Box plot of miRNA distribution in NSD sub-categories. ADNS, autoimmune diseases of the nervous system; CNSD, central nervous system diseases; CND, cranial nerve diseases; DD, demyelinating diseases; NSM, nervous system malformations; NSN, nervous system neoplasms; NS, neurocutaneous syndromes; NGD, neurodegenerative diseases; NM, neurological manifestations; NMD, neuromuscular diseases; NTS, neurotoxicity syndromes; SD, sleep disorders; TNS, trauma, nervous system.
hsa-miR-145-5p
miR-145-5p plays a critical role in vascular smooth muscle cell (VSMC) differentiation and vascular pathogenesis. Loss of miR-145-5p expression alters VSMC maintenance and vascular homeostasis, leading to incomplete differentiation [44]. Moreover, miR-145-5p controls the phenotypes of VSMCs [45]. Because of its involvement in the vascular system, miR-145-5p has been studied in the field of cerebrovascular diseases. Downregulation of miR-145-5p regulates the proliferation and contractility of VSMCs by targeting Krüppel-like factor 5 (KLF5) in intracranial aneurysm [46]. Deregulated miR-145-5p is involved in the growth and instability of carotid plaques, which break and embolize in cerebral vessels and are responsible for stroke [47]. Thus, targeting miR-145-5p may provide a novel therapeutic approach for the treatment of cerebrovascular diseases. In addition, miR-145-5p is involved in NSDs by targeting not only genes, but also long non-coding RNAs (lncRNAs). miR-145-5p is sponged by lncRNA TUG1 to promote the self-renewal of glioma stem cells [48].
NSD spectrum width of miRNAs
Here NSW was used to evaluate the effect of a miRNA in NSDs [14]. For a given miRNA(i), |$NSW(i)=m(i)/N$|, where m(i) represents the number of NSDs associated with miRNA(i), and N represents the total number of NSDs associated with all miRNAs. All NSWs of miRNAs are listed in Supplementary Table S1. The top 20 largest NSWs of miRNAs are shown in Figure 1A. These miRNAs are implicated in at least 30 NSDs and have important functions in NSDs. For example, miR-146a-5p had the highest NSW (0.4) and is involved in 43 NSDs. miR-146a-5p plays multiple roles in various NSDs; it acts as an inflammation-associated molecule in MS [49], a noninvasive biomarker for AD [50] and a risk factor in stroke [51]. miR-21-5p had the second highest NSW (0.37) and is dysfunctional in 40 NSDs. It is worth noting that miR-21-5p is frequently overexpressed and functions as an oncogene in nervous system neoplasms (NSNs) [21]. MiR-146a-5p and miR-21-5p have not only important functions in NSDs but also critical roles in other types of diseases [14].
miRNA spectrum width of NSDs
Similar to NSW, NSD miRNA spectrum width (NMSW) was used to evaluate the severity and complexity of NSDs [14]. For a given NSD(i), NMSW(i) = d(i)/M, where d(i) represents the number of miRNAs associated with NSD(i), and M represents the total number of dysfunctional miRNAs in all NSDs. All NMSWs of NSDs were listed in Supplementary Table S2. We found that 36% of NSDs were associated with more than 50 unique miRNAs. This observation suggested that NSDs are not only regulated by many miRNAs, but also have a complex regulatory genetic network. Figure 1A shows the top 20 largest NMSWs of NSDs. GBM and glioma had the highest NMSW values (0.54 and 0.45), with 693 and 583 unique miRNAs involved, respectively. These two diseases are the most common and malignant brain tumors. miRNAs contribute to the tumorigenesis of brain tumors, acting as oncogenes such as miR-21-5p [52] or tumor suppressors such as miR-218-5p [53]. MS had the third largest NMSW (0.36), with 465 miRNAs involved. MS is an autoimmune NSD that affects the brain and spinal cord and is a common cause of neurological disability in young adults [54]. A number of miRNAs (such as miR-21-5p, miR-146a/b, miR-155-5p and miR-27a-3p) are overexpressed in the blood or brain of MS patients and contribute to the development of MS [55]. For example, miR-155-5p functions in the pathological processes of MS by targeting cell–cell complex molecules (annexin-2 and claudin-1) and focal adhesion components (DOCK-1 and syntenin-1) [56].
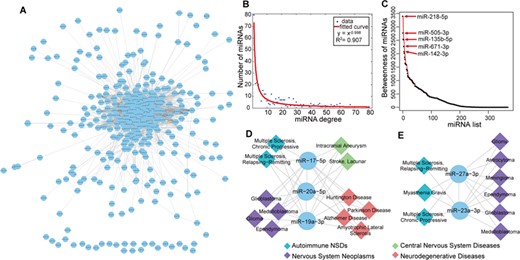
Synergistic regulation of miRNAs in NSD. (A) The human NSD-associated miRNA–miRNA network. Circles represent miRNAs. The line between two miRNAs indicates that they are significantly shared NSDs. (B) The curve fitting of miRNA degree distribution. The x-axis represents miRNA degree and the y-axis represents the number of miRNAs. (C) The miRNA betweenness distribution of the HNMN. The top five miRNAs regarding betweenness are indicated in the plot. (D) and (E) Two examples of clustered miRNA pairs that are synergistically involved in NSDs.
The distribution of miRNAs in NSD sub-categories
Next we explored the distribution of miRNAs in NSD sub-categories. The NSDs were grouped into 13 sub-categories according to MeSH disease categories, and the average number of involved miRNAs in each NSD sub-category was calculated. The results are shown in Supplementary Table S3, and the miRNA distribution was significant by one-way ANOVA test (P < 0.05; Figure 1B). We found that NSN was the most common disease involving miRNAs. NSNs are common lethal tumors characterized by aggressive growth, high heterogeneity and poor prognosis, such as GBM, glioma and medulloblastoma [57]. Because miRNAs are involved in cellular proliferation, apoptosis evasion, invasion and angiogenesis, a number of NSN-related miRNAs have been identified [7].
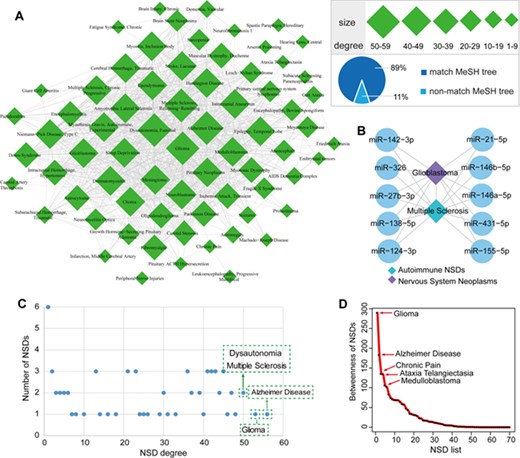
The HNNN and its global properties. (A) The human NSD–NSD network. Quadrilaterals represent NSDs, and the size of the nodes corresponds to the node degree. The line between two NSDs indicates that they significantly share common miRNAs. (B) GBM and MS are connected by sharing immune-regulatory miRNAs. (C) Scatter plot of NSD degree distribution. The x-axis represents NSD degree and the y-axis represents the number of NSDs. The top four NSDs regarding degrees are indicated in the plot. (D) The NSD betweenness distribution of the HNNN. The top five NSDs regarding betweenness are indicated in the plot.
Synergistic regulation of miRNAs in NSD
NSDs are generally affected by several miRNAs rather than by a single miRNA; therefore, it is important to understand the synergistic regulation of miRNAs in complex NSDs. miRNAs with similar functions are often involved in similar diseases [11]. Here we constructed a human NSD-associated miRNA–miRNA network (HNMN) in which two miRNAs were connected if they significantly shared common NSDs. Furthermore, we constructed a human NSD–NSD network (HNNN) in which two NSDs were connected if they significantly shared common miRNAs.
We identified 2718 miRNA–miRNA interactions among 370 unique miRNAs in the HNMN (Figure 2A). We paid attention to the topological characteristics of the HNMN, including the degree and betweenness. Supplementary Table S4 shows the degrees of miRNAs in the HNMN. The results suggested that a few miRNAs interact with a large number of miRNAs, whereas many miRNAs interact with a small number of miRNAs. miR-218-5p had the highest degree, suggesting that it shares the most significant common NSDs with other miRNAs. miR-218-5p plays a crucial role in NS development and drives motor neuron fate [58]. Examination of the miRNA degree distribution revealed a power law with a slope of −0.998 and R2 = 0.907, indicating that the HNMN has a scale-free, small world and modular architecture (Figure 2B). This feature was consistent with previously reported human miRNA–miRNA biological networks, such as MFSN [10] and miRNA functional similarity network [59]. Next we calculated the miRNAs’ betweenness, which is a measure of the total number of shortest paths passing through a certain node (Supplementary Table S5). A miRNA’s betweenness represents the centrality in the HNMN. The top five miRNAs regarding betweenness were miR-218-5p, miR-505-3p, miR-135b-5p, miR-671-3p and miR-142-3p, suggesting that these miRNAs play important roles in the connectivity of the HNMN (Figure 2C).
Certain miRNAs tend to cluster in the genome and are expected to be involved in similar diseases [59]. Here we observed NSD miRNAs cluster tendency in the HNMN. We found that 25% of NSD-related miRNAs in the HNMN are clustered. These neighboring interacting miRNAs may synergistically participate in NSDs and share common mechanisms. For example, three members of the cluster, miR-20a-5p, miR-17-5p and miR-19a-3p, are located in the region of chromosome 13q31.3. These three interacting miRNAs are co-regulated in four NSD sub-categories, including neurodegenerative disease, brain tumor, cerebrovascular disease and autoimmune NSD (Figure 2D). In particular, the four most prevalent neurodegenerative diseases [AD, ALS, Huntington disease (HD) and PD] are usually incurable and show a similar pathogenic mechanism, such as protein aggregation, mitochondrial dysfunction and axonal transport defects [60]. Another example, the miR-23a-3p/miR-27a-3p cluster, which is located in chromosome 19p13.12, is involved in autoimmune NSDs and brain tumors through a synergistic effect (Figure 2E). These two miRNAs are pro-inflammatory and promote cancer progression [61]. Therefore, targeting the miR-23a-3p/miR-27a-3p cluster may be a potential treatment for ameliorating neuroinflammation in autoimmune NSDs and brain tumors.
We identified 909 NSD–NSD interactions among 70 unique NSDs in the HNNN (Figure 3A). Supplementary Table S6 shows the degrees of NSDs in the HNNN. We found that these NSDs are tightly connected through associations with common miRNAs. AD had the highest degree with 56 interacting NSDs. Glioma had the second highest degree, interacting with 53 NSDs. This suggests the presence of cross-talk among some NSDs through common miRNAs. For example, GBM and MS are connected by sharing more than 100 miRNAs. Studies provide evidence for the relationship between neuroimmunology and brain tumors [62]. Through reviewing reliable literatures, we list 10 miRNAs that play important regulatory roles in immune mechanisms and GBM (Figure 3B). Importantly, some studies provide evidence of the safety of immunotherapy in patients with GBM [63]. As a good example, miR-138 exerts anti-GBM efficacy by targeting immune checkpoints which may have potential as a novel immunotherapeutic agent [64]. Thus, these immune-regulatory miRNAs are important for the development of miRNA-based strategies related to immunotherapy for malignant brain tumors.
The degree of distribution of the HNNN indicates that most NSDs are connected to each other, and a few NSDs such as AD or glioma represent hubs that are connected to a large number of distinct NSDs (Figure 3C). Then we calculated the betweenness of NSDs in the HNNN (Supplementary Table S7). The top five NSDs regarding betweenness were glioma, AD, chronic pain, ataxia telangiectasia and medulloblastoma, indicating that these NSDs have high connectivity in the HNNN (Figure 3D).
Next we mapped NSD–NSD pairs in the HNNN to NSD sub-categories. We found that 89% of NSDs were consistent with NSD sub-categories (Figure 3A). For example, chorea and HD were located in the sub-category “Central Nervous System Diseases” in the MeSH tree. Chorea and HD share similar clinical features and pathophysiology, which is characterized by involuntary movements of the body mainly involving the basal ganglia region [65, 66]. This suggests that these NSD pairs with similar phenotypes and pathogenesis tend to share significant common miRNAs. Interestingly, we identified certain NSD pairs that did not map to NSD sub-categories despite being biologically connected. For example, friedreich ataxia (belonging to neurodegenerative diseases) and gait ataxia (belonging to neurologic manifestations) were connected in the HNNN. There is certain overlap in the clinical features of these two diseases [67, 68].
The modular structure of the networks
Next we characterized the modular structure of the HNMN and HNNN. Here a miRNA (or NSD) module was defined as a clique, which is a maximal complete subgraph and communities where two k-cliques share k-1 nodes in the HNMN (or HNNN). All modules and communities in the HNMN (or HNNN) were identified using CFinder [69]. Finally, we observed important miRNA modules, NSD modules and their interactions underlying the regulatory mechanism.
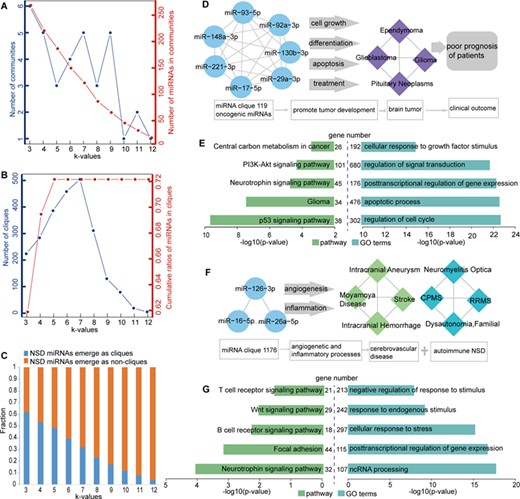
The modular structure of the HNMN. (A) The number of communities and the number of miRNAs in communities at different k-values. The left blue y-axis represents the number of communities at different k-values, corresponding to the blue line. The right red y-axis represents the number of miRNAs in communities at different k-values, corresponding to the red line. (B) The number of cliques and cumulative ratios of miRNAs in cliques at different k-values. The left blue y-axis represents the number of cliques at different k-values, corresponding to the blue line. The right red y-axis represents cumulative ratios of miRNAs in cliques at different k-values, corresponding to the red line. (C) The fraction of NSD miRNAs mapped in cliques (blue) and not mapped in cliques (orange) at different k-values. (D) All of the 10 miRNAs in miRNA clique 119 are co-involved in four brain tumors. (E) Pathway enrichment analysis (left) and GO annotation (right) of miRNAs’ targets in miRNA clique 119. (F) All of the 3 miRNAs in miRNA clique 1176 are co-involved in cerebrovascular disease and autoimmune NSDs. (G) Pathway enrichment analysis (left) and GO annotation (right) of miRNAs’ targets in miRNA clique 1176.
The modular structure of HNMN
For the HNMN, with an increase in the k-value, there is a decrease tendency in the number of miRNA in communities. This is because a low k-value generates a large number of extensive communities that show a high degree of overlap. With an increase in the k-value, the communities are more tightly connected (Figure 4A). With an increase in the k-value, there is an increase in the number of cliques. However, there was a sharp decrease in the number of cliques with k-values >7 (Figure 4B). Approximately 72% of miRNAs are involved in at least one clique, and the cumulative ratios of miRNAs in cliques reached the highest ratio with a k-value = 5 (Figure 4B). These features suggested that some NSD-related miRNAs are tightly connected and synergistically involved in NSDs.
Next we observed that NSD-related miRNAs regulate NSDs in a module manner (Figure 4C). When the k-value was 3, 62% of NSD miRNAs emerged as cliques. For example, all of the 10 miRNAs in miRNA clique 119 were co-involved in four brain tumors. These 10 miRNAs are generally overexpressed and considered to be oncogenic in brain tumors. These oncogenic miRNAs promote brain tumor development by regulating cell growth, differentiation, apoptosis, and treatment, leading to poor prognosis of patients [70–72] (Figure 4D). Another example is miRNA clique 1176, which was involved in two different NSD sub-categories. All miRNAs (miR-126-3p, miR-16-5p and miR-26a-5p) in this clique play important roles in cerebrovascular disease (belonging to central nervous system diseases) and autoimmune NSDs (Figure 4F). For example, miR-126-3p have important roles in inflammatory and angiogenetic processes. Through these processes, miR-126-3p are implicated in stroke, intracranial aneurysm, MS, among others [73]. To further confirm the roles of two miRNA cliques exampled above, we performed pathway enrichment analysis and Gene Ontology (GO) annotation of miRNAs’ targets using functional annotation tools in DAVID (P < 0.05) [74]. The experimentally validated targets of miRNAs in clique 119 and 1176 were downloaded from miRTarBase database [75], respectively. We found that the targets were significantly enriched in NSD-related pathways and GO terms, which were these two miRNA cliques co-involved (Figure 4E and G). These findings further highlighted the fundamental characteristics of miRNAs in the pathology of NSDs.
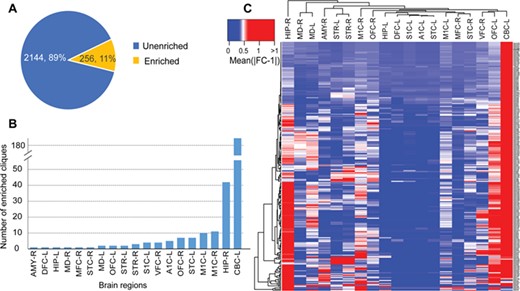
Regional expression profiles of the miRNA cliques in the brain. (A) Proportion of miRNA cliques enriched in various regions of the brain. (B) Distribution of enriched miRNA cliques in different brain regions. (C) The heatmap of differentially expressed miRNA cliques in different brain regions. AMY, amygdaloid complex; DFC, dorsolateral prefrontal cortex; HIP, hippocampus; MD, mediodorsal nucleus of thalamus; MFC, anterior (rostral) cingulate (medial prefrontal) cortex; STC, posterior (caudal) superior temporal cortex; OFC, orbital frontal cortex; STR, striatum; S1C, primary somatosensory cortex; VFC, ventrolateral prefrontal cortex; A1C, primary auditory cortex (core); M1C, primary motor cortex; CBC, cerebellar cortex; L, left; R, right.
Furthermore, we explored the regional expression profiles of the miRNA cliques in the brain and identified the important brain regions that the miRNA cliques might regulate. First, we downloaded the regional expression profiles of miRNAs in the brain from the Allen Brain Atlas (http://www.brain-map.org) [76]. T-test was used to identify the differentially expressed miRNAs in various regions of the brain (P < 0.05, |fold change (FC)-1| > 0.5). Then Fisher exact test was performed to identify significantly enriched miRNA cliques in different brain regions (P < 0.05). As a result, we found 11% of miRNA cliques were significantly enriched in various regions of the brain, suggesting that these miRNA cliques may play important regulatory roles in the development of the brain (Figure 5A). These brain-regional enriched miRNA cliques distributed in 19 brain regions, mainly in the left cerebellar cortex (CBC-L) and the right hippocampus (Figure 5B). Furthermore, hierarchical clustering of differentially expressed miRNA cliques showed that the majority of miRNA cliques were significantly enriched in CBC-L, which is a suggestion of critical regulatory mechanism mediated by miRNA in CBC-L (Figure 5C). This finding was supported by the previous studies that numerous miRNAs contributed to the cerebellar development [77]. For example, miR-9 has been shown to be involved in the establishment of the cerebellar anlage [78] and maturation of cell types in the developing cerebellum [79]. Owing to the critical roles of miRNAs in the cerebellum, the dysfunction of miRNAs during the development of the cerebellum drives to various NSDs, such as medulloblastoma [80], tuberous sclerosis [81] and spinocerebellar ataxias [82]. For example, medulloblastoma originating in the cerebellum is the most common pediatric brain tumor. It has been reported that miR-17~92 is consistently overexpressed in medulloblastoma, while silencing of miR-17~92 cluster inhibits the progression of medulloblastoma [40, 80].
The modular structure of the HNNN
For the HNNN, we identified NSD cliques and communities. We observed with an increase in the k-value, there is a decrease in the number of NSDs in communities. This is because a low k-value generates a large number of extensive communities that show a high degree of overlap. With an increase in the k-value, the communities are more tightly connected (Figure 6A). The cumulative rates of diseases in cliques showed that 83% of NSDs were involved in at least one clique (Figure 6B).
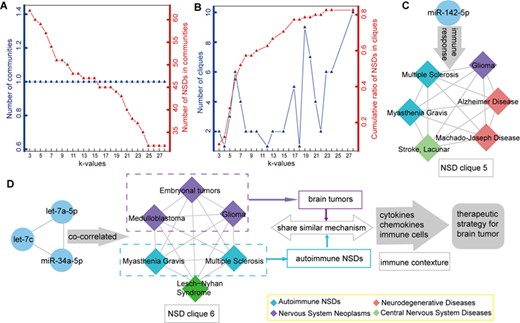
The modular structure of the HNNN. (A) The number of communities and number of NSDs in communities at different k-values. The left blue y-axis represents the number of communities at different k-values, corresponding to the blue line. The right red y-axis represents the number of NSDs in communities at different k-values, corresponding to the red line. (B) The number of cliques and cumulative ratios of NSDs in cliques at different k-values. The left blue y-axis represents the number of cliques at different k-values, corresponding to the blue line. The right red y-axis represents the cumulative ratios of NSDs in cliques at different k-values, corresponding to the red line. (C) miR-142-5p was disordered in all NSDs of clique 5. miR-142-5p is involved in all NSDs of clique 5 by regulating immune responses of them. (D) The NSD clique 6 and its related miRNA.
In addition, we observed some regulatory mechanisms of miRNAs in NSD cliques. For example, miR-142-5p was altered in all NSDs of NSD clique 5. It has become increasingly clear that immune mechanisms are involved in all NSDs of clique 5. Therefore, miR-142-5p has gained considerable attention for its role in regulating immune responses in NSD clique 5 [83] (Figure 6C). Another example is NSD clique 6, which consisted of six NSDs and was co-correlated with three miRNAs (let-7c, let-7a-5p and miR-34a-5p). The NSDs in clique 6 belonged to the sub-category of neoplasms and autoimmune NSDs (except Lesch–Nyhan syndrome). Autoimmune NSDs and brain tumors share a similar mechanism in the context of the immune system, such as cytokines, chemokines and immune cells [84, 85]. This suggested that NSDs in one clique may share a common mechanism. Because of the relationship between immunity and brain tumors, immunotherapy may be a promising therapeutic strategy for the treatment of brain tumors (Figure 6D).
Conclusion and computational challenges
NSDs are common complex disorders, and researchers have made great efforts to explore the cause of these diseases for many years. Despite considerable advances in our understanding of the molecular mechanisms, diagnosis and treatment of NSDs, a substantial percentage of patients with NSDs, such as ALS, HD and AD, are usually incurable. Improved knowledge on the roles and functions of miRNAs has shed light on the mechanisms underlying these diseases. Here we summarized the regulatory principles of miRNAs in NSDs from a network perspective. The regulatory roles of miRNAs described in the present work will help improve our understanding of the miRNA dysregulation mechanisms in NSDs.
Advances in bioinformatics have led to the use of computational models as an important means for predicting novel miRNAs involved in various diseases including NSDs. The framework of the state-of-the-art computational methods for predicting novel miRNA–disease associations is summarized as follows [86]. These methods could help biologists select the most promising disease-associated miRNAs, saving time and cost for future experimental validation. For example, You et al. [87] developed a computational model called Path-Based MiRNA–Disease Association (PBMDA), which effectively predicts potential disease-related miRNAs with high accuracy. Mørk et al. [88] proposed miRPD based on the miRNA–protein–disease heterogeneous association network for predicting miRNA–disease associations. These computational models are also crucial for identifying novel miRNAs in NSDs. For example, miR-195-5p and miR-125b-5p are predicted to be associated with PD based on the PBMDA and miRPD computational model [16]. In fact, miR-195-5p was confirmed to be differentially expressed in PD cell line [89]. Thus, miR-125b-5p can be a candidate miRNA for future experimental validation in PD. In addition, Chandrasekaran et al. [90] identified more AD-related miRNAs based on network topology analysis. Chatterjee et al. [91] also predicted novel miRNAs by constructing miRNA-gene regulatory network in AD. Moreover, Bradley [92] identified a set of miRNAs with potential for decreased expression in invasive GBM by three prediction algorithms. Although computational models promoted the progress of miRNA–NSD research, there are challenges and difficulties associated with predicting potential disease-related miRNAs. Chen et al. [86] summarized the following four important factors that limit computational models: lack of known miRNA–disease associations, unavailable negative miRNA–disease associations, relatively limited biological data and the associations of new miRNAs with diseases. Additional efforts are necessary to solve these challenges and difficulties in the future.
Technological developments in miRNA biology have stimulated efforts to develop miRNA-based therapeutic strategies. Currently, a number of miRNA-based therapeutics are undergoing extensive preclinical testing, and some therapeutics have reached clinical development [93]. Although numerous dysregulated miRNAs have been identified in NSDs, knowledge of their therapeutic potential is still in its infancy. The challenges associated with the development of miRNA-based therapeutics in NSD include delivery of miRNAs, potential off-target effects and safety and the ability to cross the blood brain barrier among others. Although there is considerable concern about miRNA-based therapeutics, recent advances in the field are promising for individuals suffering from devastating NSDs. For example, Koval et al. developed anti-miR-155 (oligonucleotide-based miR-155 inhibitor), which significantly extends survival in an ALS rodent model. Therefore, miR-155 is a promising new therapeutic target for human ALS [94].
Methods
Curated human NSD–miRNA associations
The NSD–miRNA associations were obtained from manual literature curation and available resources. We first manually curated experimentally supported NSD–miRNA associations by screening the PubMed database (July 2018). Then, NSD–miRNA associations were also extracted from the experimental resources summarized in Table 1. The miRNA names and NSD names were normalized based on the miRBase [95] and MeSH [96] databases, respectively. After removing redundancy, we got 7980 human miRNA–NSD associations from approximately 1000 studies, including 107 NSDs and 1294 miRNAs.
Construction of human NSD-associated miRNA–miRNA and NSD–NSD networks
For each miRNA–miRNA pair, N denoted the total number of NSDs that were associated with all miRNAs, n represented the number of NSDs that were associated with one miRNA, m denoted the number of NSDs that were associated with the other miRNA and x represented the number of overlapped NSDs that were associated with the two miRNAs. For each NSD–NSD pair, N denoted the total number of miRNAs that are involved in all NSDs, n represented the number of miRNAs that were associated with one NSD, m denoted the number of miRNAs that were associated with the other NSD and x represented the number of overlapped miRNAs that were associated with the two NSDs. P-values were adjusted by the Benjamini and Hochberg false discovery rate (FDR). Considering that the threshold for FDR was adopted 1 × 10−4, the number of miRNA cliques and NSD cliques (see clique modules search) in the networks were more suitable (Supplementary Figure S1). Therefore, we considered that the miRNA–miRNA pair or NSD–NSD pair was significantly connected if the FDR was less than 1 × 10−4. After assembling all miRNA pairs and NSD pairs, we constructed the HNMN and HNNN.
Degree and betweenness of the HNMN and HNNN
To better understand the HNMN and HNNN, two network topological parameters were used to characterize the roles of miRNAs in NSDs, which are degree and betweenness. The degree of a miRNA (or NSD) was defined as the number of connected miRNAs (or NSDs) in the HNMN (or HNNN). The miRNA (or NSD) degree distribution N(k) was defined as the number of miRNAs (or NSDs) with degree k. The pattern of their dependencies can be visualized by fitting a line, in which the power law curve has the form y = βxα. The R2 value is a statistical measure of the linearity of the curve fit and is used to quantify the fit to the power line. When the R2 value is closer to 1, the fit result is better. The betweenness B(v) was defined as the summation of the numbers of shortest paths between all pairs of miRNAs (or NSDs) through the node v in the HNMN (or HNNN). The shortest path was a path with the smallest number of associations between two nodes in the network.
miRNA clusters
The genome coordinate information of miRNAs was downloaded from miRBase (release 21) [95]. A cluster was defined as a maximum distance of 50 kb between two miRNA genes as previously reported [98].
Clique modules search
CFinder was used to find miRNA and NSD modules in the HNMN and HNNN. CFinder is a software tool for detecting clique modules of complex networks based on the Clique Percolation Method [69]. A k-clique in the HNMN (or HNNN) was a smaller complete subgraph in which every two miRNAs (or NSDs) in the clique were fully connected by an edge, where k refers to the number of nodes in the subgraph. A k-clique-community is defined as the union of all k-cliques that can be reached from each other through a series of adjacent k-cliques. Two k-cliques are said to be adjacent if they share k-1 nodes.
NSDs are remarkably complex, and numerous dysregulated miRNAs are involved in a broad spectrum of NSDs. Currently available miRNA–NSD resources, star NSD miRNAs, NSD spectrum width, miRNA spectrum width and the distribution of miRNAs in NSD sub-categories were summarized based on benchmarking data sets.
We constructed and characterized a human NSD-associated miRNA–miRNA network and an NSD–NSD network.
We summarized the regulatory principles of miRNAs in NSDs, including NSD miRNA cluster tendency, miRNA synergistic regulation and cross-talk among NSDs from a network perspective.
Some important miRNA modules, NSD modules and their interactions underlying the regulatory mechanism were identified.
We discussed computational challenges for identifying novel miRNAs in NSDs.
Funding
National Natural Science Foundation of China (81820108014, 81571166, 81771361, 81701190, 81701155 and 81801190), The applied technique research and development project of Harbin (2016RAXYJ067), The Fundamental Research Funds for the Provincial Universities (2017LCZX57, 2017LCZX65 and 2017LCZX48), The Postdoctoral Foundation of Heilongjiang Province (LBH-Z17138 and LBH-Z18204) and China Postdoctoral Science Foundation Grant (2018 M640307).
Jianjian Wang is a physician at Department of Neurology, The Second Affiliated Hospital of Harbin Medical University.
Yuze Cao is a postdoctoral research fellow at Department of Neurology, Peking Union Medical College Hospital, Chinese Academy of Medical Sciences. Yuze Cao is also a physician at Department of Neurology, The Second Affiliated Hospital of Harbin Medical University.
Xiaoyu Lu is a physician at Department of Neurology, The Second Affiliated Hospital of Harbin Medical University.
Tianfeng Wang is an MS student at Department of Neurology, The Second Affiliated Hospital of Harbin Medical University.
Shuang Li is an MS student at Department of Neurology, The Second Affiliated Hospital of Harbin Medical University.
Xiaotong Kong is a PhD student at Department of Neurology, The Second Affiliated Hospital of Harbin Medical University.
Chunrui Bo is a physician at Department of Neurology, The Second Affiliated Hospital of Harbin Medical University.
Jie Li is a physician at Department of Neurology, The Second Affiliated Hospital of Harbin Medical University.
Xiaolong Wang is a surgeon in Harbin Medical University Cancer Hospital.
Heping Ma is an associate professor in Emory University School of Medicine.
Lei Li is an associate professor at Department of Neurology, The Second Affiliated Hospital of Harbin Medical University.
Huixue Zhang is an associate professor at Department of Neurology, The Second Affiliated Hospital of Harbin Medical University.
Shangwei Ning is an associate professor in the College of Bioinformatics Science and Technology at Harbin Medical University.
Lihua Wang is a professor and dean of Department of Neurology, The Second Affiliated Hospital of Harbin Medical University
References
Author notes
Jianjian Wang, Yuze Cao, and Xiaoyu Lu contributed equally to this work.