-
PDF
- Split View
-
Views
-
Cite
Cite
Xin Qi, Yuxin Lin, Jiajia Chen, Bairong Shen, Decoding competing endogenous RNA networks for cancer biomarker discovery, Briefings in Bioinformatics, Volume 21, Issue 2, March 2020, Pages 441–457, https://doi.org/10.1093/bib/bbz006
- Share Icon Share
Abstract
Crosstalk between competing endogenous RNAs (ceRNAs) is mediated by shared microRNAs (miRNAs) and plays important roles both in normal physiology and tumorigenesis; thus, it is attractive for systems-level decoding of gene regulation. As ceRNA networks link the function of miRNAs with that of transcripts sharing the same miRNA response elements (MREs), e.g. pseudogenes, competing mRNAs, long non-coding RNAs, and circular RNAs, the perturbation of crucial interactions in ceRNA networks may contribute to carcinogenesis by affecting the balance of cellular regulatory system. Therefore, discovering biomarkers that indicate cancer initiation, development, and/or therapeutic responses via reconstructing and analyzing ceRNA networks is of clinical significance. In this review, the regulatory function of ceRNAs in cancer and crucial determinants of ceRNA crosstalk are firstly discussed to gain a global understanding of ceRNA-mediated carcinogenesis. Then, computational and experimental approaches for ceRNA network reconstruction and ceRNA validation, respectively, are described from a systems biology perspective. We focus on strategies for biomarker identification based on analyzing ceRNA networks and highlight the translational applications of ceRNA biomarkers for cancer management. This article will shed light on the significance of miRNA-mediated ceRNA interactions and provide important clues for discovering ceRNA network-based biomarker in cancer biology, thereby accelerating the pace of precision medicine and healthcare for cancer patients.
Introduction
Over the past decade, the complexity of the human transcriptome has been highlighted by high-throughput transcriptome profiling studies, which have revealed that thousands of transcripts in human genome act as non-coding RNAs (ncRNAs) rather than protein-coding genes [1]. Generally, ncRNAs can be divided into small RNAs [such as microRNAs (miRNAs) and small interfering RNA (siRNAs)] and long non-coding RNAs (lncRNAs) based on sequence length. Importantly, the discovery of extensive ncRNAs provides new insights into the centrality of RNAs in gene regulation.
miRNAs are endogenous ~22 nt RNAs that play key post-transcriptional regulatory roles by targeting mRNAs for cleavage or translational repression. This regulation depends on base-pairing complementarity between the mRNA molecule and the ‘seed’ region at the 5′ end of the miRNA [2]. Increasing evidence indicates that miRNAs are widely involved in the regulation of diverse biological processes, including cell proliferation, differentiation, development and metabolism, and that aberrant miRNA expression could contribute to the initiation and progression of human cancers [3–5].
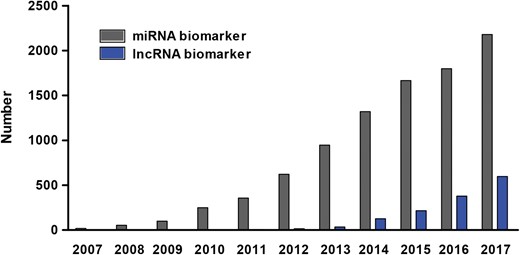
Number of papers on miRNA and lncRNA biomarkers published over the past decade. To count the number of papers on miRNA biomarker (grey bar), papers containing the term ‘microRNA’ or ‘miRNA’ and ‘biomarker’ in the title or abstract were searched in PubMed. Similarly, papers on lncRNA biomarker (blue bar) were counted by searching the key words ‘long non-coding RNA’ or ‘lncRNA’ or ‘lincRNA’ and ‘biomarker’ in the title or abstract in PubMed.
According to their action mechanisms, individual miRNAs may regulate numerous target transcripts that share common miRNA response elements (MREs) by binding to complementary sequences in their 3′ UTR. Artificial miRNA sponge transcripts that contain multiple complementary binding sites to a miRNA of interest have been widely used to inhibit miRNA activity by transgene approach [6, 7]. The miRNA target multiplicity as well as the application of miRNA sponges in a variety of experimental systems has laid an important foundation for the competing endogenous RNAs (ceRNAs) hypothesis, which postulates that both coding and non-coding endogenous RNA molecules sharing MREs can competitively prevent the action of miRNAs on their mRNA targets, thereby regulating gene expression in trans [8]. Although it so far has been considered that effective ceRNA crosstalk is restricted to a small subset of RNA molecules under physiological conditions due to multiple factors, emerging evidence from exploring ceRNA functions has dramatically altered our view of how genes are regulated at the post-transcriptional level. Moreover, perturbing key members of cancer-associated ceRNA networks, e.g., miRNAs, lncRNAs and mRNAs, may disrupt the stability and robustness of the network structure, thereby leading to carcinogenesis [9, 10].
Biomarkers are a class of measurable and evaluable medical signs that can objectively indicate disease initiation, progression and treatment effects [11]. They have been extensively used as powerful tools for patient diagnosis, disease staging and monitoring, and predicting clinical prognosis [12]. Especially, biomarkers possess great potential for cancer management, if they could provide an early indication of tumorigenesis or enable real-time tracking of cancer progression/therapeutic response [13]. Thus, a systematic screening and application of biomarkers to cancer research is urgently needed to promote precision medicine and healthcare.
As shown in Figure 1, components of ceRNA network such as miRNAs and lncRNAs have been considered as biomarkers for cancer diagnosis and treatment in accumulating studies. For example, miR-21, an oncogenic miRNA that negatively modulates multiple tumor-suppressor genes, can serve as a biomarker in various human cancers, including glioblastoma, breast cancer, lung cancer, gastric cancer and colorectal cancer [14]. Similarly, the lncRNAs HOTAIR and H19 have been widely characterized to be overexpressed in multiple cancers, where they can serve as diagnostic and/or prognostic biomarkers [15–21]. Cancer is a complex disease caused by the disorder of various signaling networks in tumorigenesis process; hence, systematical investigation of the interactions within cancer-associated ceRNA networks will further benefit our understanding of carcinogenesis.
To decode the functional role of ceRNAs in cancer biology and highlight the current thoughts on ceRNA-based biomarker discovery, herein, we present a comprehensive review on biomarker characterization under the framework of ceRNA network reconstruction and analysis. First, we review the emerging function of ceRNAs in cancer pathogenesis and the important determinants for ceRNA activity. Then, we propose an integrative pipeline for ceRNA network reconstruction from a systems biology perspective, and summarize the experimental approaches for identi-fying and validating ceRNA interactions. In particular, we elucidate computational approaches for ceRNA biomarker identification and exploration, as well as the application of ceRNA biomarkers to cancer management. Finally, future perspectives and challenges regarding ceRNA biomarkers in precision medicine are discussed.
Decoding ceRNA-mediated carcinogenesis
ceRNAs are endogenous transcripts that contain shared MREs and regulate each other by reducing miRNA availabi-lity through competing for binding to shared miRNAs [8]. Recently, accumulating evidence of ceRNA regulation in diverse species supports that a variety of RNAs can function as ceRNAs, including pseudogenes, protein-coding transcripts, lncRNAs and circular RNAs (circRNAs) (Figure 2A) [22, 23]. Thus, analyzing ceRNA networks may facilitate the functional characterization of both non-coding and protein-coding transcripts.

Schematic diagram of ceRNA mechanism. (A) Endogenous transcripts sharing MREs, such as lncRNAs, pseudogenes, circRNAs and mRNAs, could act as ceRNAs to sponge miRNAs, thereby modulating the de-repression of miRNA targets. (B) Representation of the impact of altered ceRNA abundance on miRNA-mediated target gene expression.
The functions of ceRNAs in cancer
Recently, an increasing number of studies have demonstrated that the four types of ceRNAs are extensively involved in carcinogenesis by regulating key oncogene or tumor suppressor gene expression via a miRNA-mediated manner (Table 1). The first experimental evidence for ceRNA activity came from the functional investigation of pseudogene PTENP1 in regulating its cognate gene PTEN by competing for shared miRNAs. PTEN is a crucial tumor suppressor that is commonly mutated at considerable frequency in various human cancers. Overexpression of PTENP1 3′ UTR increased PTEN expression, leading to cancer cell growth inhibition; whereas knockdown of PTENP1 decreased PTEN1 expression, accelerating cancer cell proliferation [24]. Furthermore, other examples of lncRNAs and circRNAs acting as ceRNAs have been increasingly reported. For example, lncRNA H19 up-regulated the expression level of VIM, ZEB1 and ZEB2 by acting as decoys of miR-138 and miR-200a, thereby promoting epithelial-to-mesenchymal transition (EMT) and tumor growth in colorectal cancer [25]. circRNA circ-ITCH was found to promote p21 and PTEN expression by acting as sponges for miR-17 and miR-224, thereby inhibiting bladder cancer progression [26]. In addition to ncRNAs, protein-coding mRNAs that contain MREs on their 3′ UTR have also emerged as key ceRNAs in cancer. It has been experimentally validated that 13 protein-coding transcripts can act as ceRNA to control PTEN expression in a 3′ UTR-dependent manner and promote cancer cell growth in glioblastoma [27].
ceRNA . | Shared miRNA . | Competing target . | Cancer type . | Biological effects of ceRNAs . | PMID . |
---|---|---|---|---|---|
lncRNA | |||||
Snhg1 | miR-338 | CST3 | Esophageal cancer | promote cancer cell growth | 28423738 |
UCA1 | miR-216b | FGFR1 | HCC | promote cancer cell growth and metastasis | 25760077 |
Linc-ROR | miR-145 | ARF6 | Breast cancer | promote cancer cell invasion | 25253741 |
PCAT-1 | miR-129-5p | HMGB1 | HCC | promote cancer cell invasion and metastasis | 28931210 |
H19 | miR-342-3p | FOXM1 | GBC | promote cancer cell invasion and proliferation | 27716361 |
MALAT1 | miR-1 | cdc42 | Breast cancer | promote cancer cell migration and invasion | 26926567 |
HOXD-AS1 | miR-130a | E2F8 | Glioma | promote cancer cell migration and invasion | 29341117 |
GAPLINC | miR-211-3p | CD44 | GC | promote cancer cell migration and proliferation | 25277524 |
H19 | miR-484 | ROCK2 | Lung cancer | promote cancer cell migration, invasion, and EMT process | 29219208 |
TP73-AS1 | miR-200a | TFAM | Breast cancer | promote cancer cell proliferation | 28639399 |
MALAT1 | miR-363-3p | MCL-1 | GBC | promote cancer cell proliferation | 27420766 |
BC032469 | miR-1207-5p | hTERT | GC | promote cancer cell proliferation | 26549025 |
TINCR | miR-375 | PDK1 | GC | promote cancer cell proliferation | 28744139 |
PVT1-5 | miR-126 | SLC7A5 | Lung cancer | promote cancer cell proliferation | 29277611 |
MALAT1 | miR-124 | STAT3 | NSCLC | promote cancer cell proliferation | 29215698 |
PCAT1 | miR-3667-3p | c-Myc | PCa | promote cancer cell proliferation | 25425964 |
SNHG1 | miR-199a-3p | CDK7 | PCa | promote cancer cell proliferation | 28400279 |
PVT1 | miR-199a-5p | HIF1α | NSCLC | promote cancer cell proliferation | 29115513 |
HOTTIP | miR-574-5p | EZH1 | SCLC | promote cancer cell proliferation and cell cycle | 29041935 |
LINC00974 | miR-642 | KRT19 | HCC | promote cancer cell proliferation and metastasis | 25476897 |
UCA1 | miR-193a | HMGB1 | Lung cancer | promote cancer cell proliferation and migration | 29355524 |
CCAT1 | let-7 | HMGA2 and c-Myc | HCC | promote cancer cell proliferation and migration | 25884472 |
HNF1A-AS1 | miR-30b-5p | Bcl-2 | Bladder cancer | promote cancer cell proliferation and suppress cancer cell apoptosis | 29100339 |
XLOC_008466 | miR-874 | MMP2 and XIAP | NSCLC | promote cancer cell proliferation, invasion and suppress cancer cell apoptosis | 28501870 |
HIF1A-AS2 | miR-129-5p | DNMT3A | CRC | promote cancer cell proliferation, invasion and EMT formation | 29278853 |
OIP5-AS1 | miR-186a-5p | ZEB1 | Hepatoblastoma | promote cancer cell proliferation, metastasis and EMT progress | 29475118 |
HOTAIR | miR-331-3p | HER2 | GC | promote cancer cell proliferation, migration and invasion | 24775712 |
SNHG1 | miR-326 | NOB1 | Osteosarcoma | promote cancer cell growth, migration and invasion | 29115574 |
HOTAIR | miR-373 | Rab22a | OV | promote cancer cell proliferation, migration and invasion | 27484896 |
CCAT1 | miR-181b | FGFR3 and PDGFRα | Glioma | promote cancer cell proliferation, migration and the EMT process, and suppress cancer cell apoptosis | 28475287 |
H19 | miR-17-5p | YES1 | Thyroid cancer | promote cancer cell proliferation, migration, and invasion | 27093644 |
XIST | miR-137 | PXN | NSCLC | promote cancer cell viability and invasion | 29337100 |
CASC2 | miR-367 | FBXW7 | HCC | suppress cancer cell migration, invasion and EMT progression | 28716020 |
TUSC7 | miR-211 | CDK6 | CRC | suppress cancer cell proliferation | 28214867 |
Gas5 | miR-222-3p | PTEN | PTC | suppress cancer cell proliferation | 29423063 |
TINCR | miR-544a | FBXW7 | Lung cancer | suppress cancer cell proliferation and invasion | 29324317 |
PWRN1 | miR-425-5p | PTEN | GC | suppress cancer cell proliferation and metastasis and promote cancer cell apoptosis | 29535266 |
SNHG5 | miR-32 | KLF4 | GC | suppress cancer cell proliferation and migration | 27871067 |
MEG3 | miR-181a | Bcl-2 | GC | suppress cancer cell proliferation, migration, invasion, and promoted cancer cell apoptosis | 26253106 |
CASC2 | miR-183 | Sprouty2 | PCa | suppress cancer cell proliferation, promote cancer cell apoptosis, and enhance the sensitivity of PCa cancer cells to docetaxel | 29373811 |
lncRNA RP4 | miR-7-5p | SH3GLB1 | CRC | suppress cancer cell proliferation and tumor growth | 29531464 |
H19 | miR-29b-3p | DNMT3B | Bladder cancer | promote cancer EMT and metastasis | 28779971 |
H19 | miR-138/200a | Vimentin, ZEB1, and ZEB2 | CRC | promote cancer cell EMT | 26068968 |
Linc-ROR | miR-145 | ZEB2 | HCC | promote cancer cell invasion and metastasis via induction of EMT | 28680141 |
RSU1P2 | let-7a | IGF1R, N-Myc and EphA4 | Cervical cancer | promote tumorigenesis | 27487126 |
lncRNA-PAGBC | miR-133b/511 | SOX4 and PIK3R3 | GBC | promote tumorigenesis | 28887321 |
LINC01234 | miR-204-5p | CBFB | GC | promote tumorigenesis | 29386218 |
HULC | miR-200a-3p | ZEB1 | HCC | promote tumorigenesis and metastasis | 27285757 |
CCAT1 | miR-218-5p | BMI1 | GBC | promote cancer development | 25569100 |
NEAT1 | miR-506 | STAT3 | GC | promote cancer development | 29363783 |
HULC | miR-186 | HMGA2 | HCC | promote cancer growth | 28765279 |
HOXD-AS1 | miR-130a-3p | SOX4 | Liver cancer | promote cancer metastasis | 28810927 |
NORAD | miR-125a-3p | RhoA | Pancreatic cancer | promote cancer metastasis | 29121972 |
Linc-ROR | miR-145 | FSCN1 | ESCC | promote cancer metastasis and invasion | 29430188 |
Lnc-ATB | miR-141-3p | TGFβ2 | GC | promote cancer progression | 28115163 |
MIAT | miR-155-5p | DUSP7 | Breast cancer | promote cancer progression | 29100300 |
NEAT1 | miR-448 | ZEB1 | Breast cancer | promote cancer progression | 29323713 |
lincRNA-ROR | miR-145 | NA | Colon cancer | promote cancer progression | 27071407 |
HCP5 | miR-22-3p/186-5p/216a-5p | ST6GAL2 | FTC | promote cancer progression | 29515098 |
MALAT1 | miR-202 | Gli2 | GC | promote cancer progression | 27887846 |
XIST | miR-185 | TGF-β1 | GC | promote cancer progression | 29053187 |
TUG1 | miR-145 | Sirt3 | ICC | promote cancer progression | 29371936 |
CCAT1 | miR-181a-5p | HOXA1 | MM | promote cancer progression | 29228867 |
NEAT1 | miR-377-3p | E2F3 | NSCLC | promote cancer progression | 27351135 |
SNHG7 | miR-193b | FAIM2 | NSCLC | promote cancer progression | 29131440 |
UCA1 | miR-204 | ATF2 | PCa | promote cancer progression | 28337266 |
Linc-ROR | miR-205 | ZEB2 | Breast cancer | promote EMT and cancer progression and metastasis | 24922071 |
NEAT1 | miR-193b-3p | CCND1 | Cervical cancer | promote cancer radio-resistance | 29416780 |
TUG1 | miR-186 | CPEB2 | CRC | promote resistance to methotrexate treatment | 28302487 |
UCA1 | miR-195 | ARL2 | Bladder cancer | promote mitochondrial function in cancer cell | 29130995 |
HULC | miR-372 | PRKACB | HCC | NA | 20423907 |
pseudogene | |||||
INTS6P1 | miR-17-5p | INTS6 | HCC | promote cancer cell death and suppress cancer cell mobility | 25686840 |
KRAS1P | NA | K-RAS | PCa | promote cancer cell growth | 20577206 |
BRAFP1 | miR-30a, miR-876, miR-182 | BRAF | Lymphoma | promote cancer development | 25843629 |
CYP4Z2P | miR-211/125a-3p/197/1226/204 | CYP4Z1 | Breast cancer | promote tumor angiogenesis | 25701119 |
PTENP1 | miR-106b/93 | PTEN | GC | suppress cancer cell growth and induce cancer cell apoptosis | 28212532 |
PTENP1 | miR-193a-3p | PTEN | HCC | suppress cancer cell migration and invasion | 29296207 |
PTENP1 | miR-21 | PTEN | OSCC | suppress cancer cell proliferation | 27862321 |
PTENP1 | miR-19b | PTEN | Breast cancer | suppress cancer cell proliferation, migration and invasion and promote cancer cell apoptosis | 28731027 |
PTENP1 | miR-17/19b/20a | PTEN and PHLPP | HCC | suppress cancer cell proliferation, migration/invasion and induce cancer cell autophagy and apoptosis | 25617127 |
CTNNAP1 | miR-141 | CTNNA1 | CRC | suppress cancer cell proliferation and tumor growth | 27487124 |
PTENP1 | miR-21 | PTEN | ccRCC | suppress cancer progression | 25249556 |
circRNA | |||||
circRNA-MYLK | miR-29a | VEGFA | Bladder cancer | promote cancer progression | 28687357 |
circGFRA1 | miR-34a | GFRA1 | Breast cancer | promote cancer cell proliferation and suppress cancer cell apoptosis | 29037220 |
circDOCK1 | miR-196a-5p | BIRC3 | OSCC | suppress cancer cell apoptosis | 29286141 |
cir-ITCH | miR-7/20a/214 | ITCH | CRC | suppress cancer cell proliferation | 26110611 |
cir-ITCH | miR-7/17/214 | ITCH | ESCC | suppress cancer cell proliferation and tumor growth | 25749389 |
cir-ITCH | miR-17/224 | p21 and PTEN | Bladder cancer | suppress cancer progression | 29386015 |
mRNA | |||||
versican 3′ UTR | miR-133a/199a-3p/144/431 | CD34 and Fibronectin | HCC | promote cancer development | 23180826 |
XIAP 3′ UTR | miR-29a-5p | FSCN1 | Breast cancer | promote cancer progression | 28186968 |
IGF1R/ROCK2/RAP1B 3′ UTR | miR-139-5p | IGF1R/ROCK2/RAP1B | CRC | promote cancer cell proliferation | 24942287 |
AEG-1 3′ UTR | miR-30a | Vimentin and Snail | NSCLC | promote EMT | 25484183 |
FOXO1 3′ UTR | miR-9 | E-cadherin | Breast cancer | suppress cancer metastasis | 25017439 |
USP3 | miR-224 | SMAD4 | CRC | suppress cancer metastasis | 28655924 |
versican 3′ UTR | miR-136/199a-3p/144 | Rb1 and PTEN | Breast cancer | suppress cancer cell proliferation and tumor growth | 21049042 |
StarD13 3′ UTR | miR-125b | TP53INP1 | Breast cancer | suppress cancer cell migration and invasion | 29146309 |
CNOT6L/VAPA/SERINC1 | miR-17/19a/20a/b/106a/b/93 | PTEN | PCa | suppress cancer cell proliferation | 22000013 |
ZEB2 | miR-25/92a/181a/200b | PTEN | Melanoma | suppress tumorigenesis | 22000016 |
EPO/EPOR | miR-125b | ERBB2/HER2 | Breast cancer | NA | 24165569 |
ceRNA . | Shared miRNA . | Competing target . | Cancer type . | Biological effects of ceRNAs . | PMID . |
---|---|---|---|---|---|
lncRNA | |||||
Snhg1 | miR-338 | CST3 | Esophageal cancer | promote cancer cell growth | 28423738 |
UCA1 | miR-216b | FGFR1 | HCC | promote cancer cell growth and metastasis | 25760077 |
Linc-ROR | miR-145 | ARF6 | Breast cancer | promote cancer cell invasion | 25253741 |
PCAT-1 | miR-129-5p | HMGB1 | HCC | promote cancer cell invasion and metastasis | 28931210 |
H19 | miR-342-3p | FOXM1 | GBC | promote cancer cell invasion and proliferation | 27716361 |
MALAT1 | miR-1 | cdc42 | Breast cancer | promote cancer cell migration and invasion | 26926567 |
HOXD-AS1 | miR-130a | E2F8 | Glioma | promote cancer cell migration and invasion | 29341117 |
GAPLINC | miR-211-3p | CD44 | GC | promote cancer cell migration and proliferation | 25277524 |
H19 | miR-484 | ROCK2 | Lung cancer | promote cancer cell migration, invasion, and EMT process | 29219208 |
TP73-AS1 | miR-200a | TFAM | Breast cancer | promote cancer cell proliferation | 28639399 |
MALAT1 | miR-363-3p | MCL-1 | GBC | promote cancer cell proliferation | 27420766 |
BC032469 | miR-1207-5p | hTERT | GC | promote cancer cell proliferation | 26549025 |
TINCR | miR-375 | PDK1 | GC | promote cancer cell proliferation | 28744139 |
PVT1-5 | miR-126 | SLC7A5 | Lung cancer | promote cancer cell proliferation | 29277611 |
MALAT1 | miR-124 | STAT3 | NSCLC | promote cancer cell proliferation | 29215698 |
PCAT1 | miR-3667-3p | c-Myc | PCa | promote cancer cell proliferation | 25425964 |
SNHG1 | miR-199a-3p | CDK7 | PCa | promote cancer cell proliferation | 28400279 |
PVT1 | miR-199a-5p | HIF1α | NSCLC | promote cancer cell proliferation | 29115513 |
HOTTIP | miR-574-5p | EZH1 | SCLC | promote cancer cell proliferation and cell cycle | 29041935 |
LINC00974 | miR-642 | KRT19 | HCC | promote cancer cell proliferation and metastasis | 25476897 |
UCA1 | miR-193a | HMGB1 | Lung cancer | promote cancer cell proliferation and migration | 29355524 |
CCAT1 | let-7 | HMGA2 and c-Myc | HCC | promote cancer cell proliferation and migration | 25884472 |
HNF1A-AS1 | miR-30b-5p | Bcl-2 | Bladder cancer | promote cancer cell proliferation and suppress cancer cell apoptosis | 29100339 |
XLOC_008466 | miR-874 | MMP2 and XIAP | NSCLC | promote cancer cell proliferation, invasion and suppress cancer cell apoptosis | 28501870 |
HIF1A-AS2 | miR-129-5p | DNMT3A | CRC | promote cancer cell proliferation, invasion and EMT formation | 29278853 |
OIP5-AS1 | miR-186a-5p | ZEB1 | Hepatoblastoma | promote cancer cell proliferation, metastasis and EMT progress | 29475118 |
HOTAIR | miR-331-3p | HER2 | GC | promote cancer cell proliferation, migration and invasion | 24775712 |
SNHG1 | miR-326 | NOB1 | Osteosarcoma | promote cancer cell growth, migration and invasion | 29115574 |
HOTAIR | miR-373 | Rab22a | OV | promote cancer cell proliferation, migration and invasion | 27484896 |
CCAT1 | miR-181b | FGFR3 and PDGFRα | Glioma | promote cancer cell proliferation, migration and the EMT process, and suppress cancer cell apoptosis | 28475287 |
H19 | miR-17-5p | YES1 | Thyroid cancer | promote cancer cell proliferation, migration, and invasion | 27093644 |
XIST | miR-137 | PXN | NSCLC | promote cancer cell viability and invasion | 29337100 |
CASC2 | miR-367 | FBXW7 | HCC | suppress cancer cell migration, invasion and EMT progression | 28716020 |
TUSC7 | miR-211 | CDK6 | CRC | suppress cancer cell proliferation | 28214867 |
Gas5 | miR-222-3p | PTEN | PTC | suppress cancer cell proliferation | 29423063 |
TINCR | miR-544a | FBXW7 | Lung cancer | suppress cancer cell proliferation and invasion | 29324317 |
PWRN1 | miR-425-5p | PTEN | GC | suppress cancer cell proliferation and metastasis and promote cancer cell apoptosis | 29535266 |
SNHG5 | miR-32 | KLF4 | GC | suppress cancer cell proliferation and migration | 27871067 |
MEG3 | miR-181a | Bcl-2 | GC | suppress cancer cell proliferation, migration, invasion, and promoted cancer cell apoptosis | 26253106 |
CASC2 | miR-183 | Sprouty2 | PCa | suppress cancer cell proliferation, promote cancer cell apoptosis, and enhance the sensitivity of PCa cancer cells to docetaxel | 29373811 |
lncRNA RP4 | miR-7-5p | SH3GLB1 | CRC | suppress cancer cell proliferation and tumor growth | 29531464 |
H19 | miR-29b-3p | DNMT3B | Bladder cancer | promote cancer EMT and metastasis | 28779971 |
H19 | miR-138/200a | Vimentin, ZEB1, and ZEB2 | CRC | promote cancer cell EMT | 26068968 |
Linc-ROR | miR-145 | ZEB2 | HCC | promote cancer cell invasion and metastasis via induction of EMT | 28680141 |
RSU1P2 | let-7a | IGF1R, N-Myc and EphA4 | Cervical cancer | promote tumorigenesis | 27487126 |
lncRNA-PAGBC | miR-133b/511 | SOX4 and PIK3R3 | GBC | promote tumorigenesis | 28887321 |
LINC01234 | miR-204-5p | CBFB | GC | promote tumorigenesis | 29386218 |
HULC | miR-200a-3p | ZEB1 | HCC | promote tumorigenesis and metastasis | 27285757 |
CCAT1 | miR-218-5p | BMI1 | GBC | promote cancer development | 25569100 |
NEAT1 | miR-506 | STAT3 | GC | promote cancer development | 29363783 |
HULC | miR-186 | HMGA2 | HCC | promote cancer growth | 28765279 |
HOXD-AS1 | miR-130a-3p | SOX4 | Liver cancer | promote cancer metastasis | 28810927 |
NORAD | miR-125a-3p | RhoA | Pancreatic cancer | promote cancer metastasis | 29121972 |
Linc-ROR | miR-145 | FSCN1 | ESCC | promote cancer metastasis and invasion | 29430188 |
Lnc-ATB | miR-141-3p | TGFβ2 | GC | promote cancer progression | 28115163 |
MIAT | miR-155-5p | DUSP7 | Breast cancer | promote cancer progression | 29100300 |
NEAT1 | miR-448 | ZEB1 | Breast cancer | promote cancer progression | 29323713 |
lincRNA-ROR | miR-145 | NA | Colon cancer | promote cancer progression | 27071407 |
HCP5 | miR-22-3p/186-5p/216a-5p | ST6GAL2 | FTC | promote cancer progression | 29515098 |
MALAT1 | miR-202 | Gli2 | GC | promote cancer progression | 27887846 |
XIST | miR-185 | TGF-β1 | GC | promote cancer progression | 29053187 |
TUG1 | miR-145 | Sirt3 | ICC | promote cancer progression | 29371936 |
CCAT1 | miR-181a-5p | HOXA1 | MM | promote cancer progression | 29228867 |
NEAT1 | miR-377-3p | E2F3 | NSCLC | promote cancer progression | 27351135 |
SNHG7 | miR-193b | FAIM2 | NSCLC | promote cancer progression | 29131440 |
UCA1 | miR-204 | ATF2 | PCa | promote cancer progression | 28337266 |
Linc-ROR | miR-205 | ZEB2 | Breast cancer | promote EMT and cancer progression and metastasis | 24922071 |
NEAT1 | miR-193b-3p | CCND1 | Cervical cancer | promote cancer radio-resistance | 29416780 |
TUG1 | miR-186 | CPEB2 | CRC | promote resistance to methotrexate treatment | 28302487 |
UCA1 | miR-195 | ARL2 | Bladder cancer | promote mitochondrial function in cancer cell | 29130995 |
HULC | miR-372 | PRKACB | HCC | NA | 20423907 |
pseudogene | |||||
INTS6P1 | miR-17-5p | INTS6 | HCC | promote cancer cell death and suppress cancer cell mobility | 25686840 |
KRAS1P | NA | K-RAS | PCa | promote cancer cell growth | 20577206 |
BRAFP1 | miR-30a, miR-876, miR-182 | BRAF | Lymphoma | promote cancer development | 25843629 |
CYP4Z2P | miR-211/125a-3p/197/1226/204 | CYP4Z1 | Breast cancer | promote tumor angiogenesis | 25701119 |
PTENP1 | miR-106b/93 | PTEN | GC | suppress cancer cell growth and induce cancer cell apoptosis | 28212532 |
PTENP1 | miR-193a-3p | PTEN | HCC | suppress cancer cell migration and invasion | 29296207 |
PTENP1 | miR-21 | PTEN | OSCC | suppress cancer cell proliferation | 27862321 |
PTENP1 | miR-19b | PTEN | Breast cancer | suppress cancer cell proliferation, migration and invasion and promote cancer cell apoptosis | 28731027 |
PTENP1 | miR-17/19b/20a | PTEN and PHLPP | HCC | suppress cancer cell proliferation, migration/invasion and induce cancer cell autophagy and apoptosis | 25617127 |
CTNNAP1 | miR-141 | CTNNA1 | CRC | suppress cancer cell proliferation and tumor growth | 27487124 |
PTENP1 | miR-21 | PTEN | ccRCC | suppress cancer progression | 25249556 |
circRNA | |||||
circRNA-MYLK | miR-29a | VEGFA | Bladder cancer | promote cancer progression | 28687357 |
circGFRA1 | miR-34a | GFRA1 | Breast cancer | promote cancer cell proliferation and suppress cancer cell apoptosis | 29037220 |
circDOCK1 | miR-196a-5p | BIRC3 | OSCC | suppress cancer cell apoptosis | 29286141 |
cir-ITCH | miR-7/20a/214 | ITCH | CRC | suppress cancer cell proliferation | 26110611 |
cir-ITCH | miR-7/17/214 | ITCH | ESCC | suppress cancer cell proliferation and tumor growth | 25749389 |
cir-ITCH | miR-17/224 | p21 and PTEN | Bladder cancer | suppress cancer progression | 29386015 |
mRNA | |||||
versican 3′ UTR | miR-133a/199a-3p/144/431 | CD34 and Fibronectin | HCC | promote cancer development | 23180826 |
XIAP 3′ UTR | miR-29a-5p | FSCN1 | Breast cancer | promote cancer progression | 28186968 |
IGF1R/ROCK2/RAP1B 3′ UTR | miR-139-5p | IGF1R/ROCK2/RAP1B | CRC | promote cancer cell proliferation | 24942287 |
AEG-1 3′ UTR | miR-30a | Vimentin and Snail | NSCLC | promote EMT | 25484183 |
FOXO1 3′ UTR | miR-9 | E-cadherin | Breast cancer | suppress cancer metastasis | 25017439 |
USP3 | miR-224 | SMAD4 | CRC | suppress cancer metastasis | 28655924 |
versican 3′ UTR | miR-136/199a-3p/144 | Rb1 and PTEN | Breast cancer | suppress cancer cell proliferation and tumor growth | 21049042 |
StarD13 3′ UTR | miR-125b | TP53INP1 | Breast cancer | suppress cancer cell migration and invasion | 29146309 |
CNOT6L/VAPA/SERINC1 | miR-17/19a/20a/b/106a/b/93 | PTEN | PCa | suppress cancer cell proliferation | 22000013 |
ZEB2 | miR-25/92a/181a/200b | PTEN | Melanoma | suppress tumorigenesis | 22000016 |
EPO/EPOR | miR-125b | ERBB2/HER2 | Breast cancer | NA | 24165569 |
GC: gastric cancer; CRC: colorectal cancer; FTC: follicular thyroid carcinoma; PTC: papillary thyroid carcinoma; PCa: prostate cancer; GBC: gallbladder cancer; ICC: intrahepatic cholangiocarcinoma; MM: multiple myeloma; SCLC: small cell lung cancer; HCC: hepatocellular carcinoma; ESCC: esophageal squamous cell carcinoma; ccRCC: clear-cell renal cell carcinoma; OSCC: oral squamous cell carcinoma; NSCLC: non-small cell lung cancer; OV: ovarian cancer; EMT: epithelial-to-mesenchymal transition.
ceRNA . | Shared miRNA . | Competing target . | Cancer type . | Biological effects of ceRNAs . | PMID . |
---|---|---|---|---|---|
lncRNA | |||||
Snhg1 | miR-338 | CST3 | Esophageal cancer | promote cancer cell growth | 28423738 |
UCA1 | miR-216b | FGFR1 | HCC | promote cancer cell growth and metastasis | 25760077 |
Linc-ROR | miR-145 | ARF6 | Breast cancer | promote cancer cell invasion | 25253741 |
PCAT-1 | miR-129-5p | HMGB1 | HCC | promote cancer cell invasion and metastasis | 28931210 |
H19 | miR-342-3p | FOXM1 | GBC | promote cancer cell invasion and proliferation | 27716361 |
MALAT1 | miR-1 | cdc42 | Breast cancer | promote cancer cell migration and invasion | 26926567 |
HOXD-AS1 | miR-130a | E2F8 | Glioma | promote cancer cell migration and invasion | 29341117 |
GAPLINC | miR-211-3p | CD44 | GC | promote cancer cell migration and proliferation | 25277524 |
H19 | miR-484 | ROCK2 | Lung cancer | promote cancer cell migration, invasion, and EMT process | 29219208 |
TP73-AS1 | miR-200a | TFAM | Breast cancer | promote cancer cell proliferation | 28639399 |
MALAT1 | miR-363-3p | MCL-1 | GBC | promote cancer cell proliferation | 27420766 |
BC032469 | miR-1207-5p | hTERT | GC | promote cancer cell proliferation | 26549025 |
TINCR | miR-375 | PDK1 | GC | promote cancer cell proliferation | 28744139 |
PVT1-5 | miR-126 | SLC7A5 | Lung cancer | promote cancer cell proliferation | 29277611 |
MALAT1 | miR-124 | STAT3 | NSCLC | promote cancer cell proliferation | 29215698 |
PCAT1 | miR-3667-3p | c-Myc | PCa | promote cancer cell proliferation | 25425964 |
SNHG1 | miR-199a-3p | CDK7 | PCa | promote cancer cell proliferation | 28400279 |
PVT1 | miR-199a-5p | HIF1α | NSCLC | promote cancer cell proliferation | 29115513 |
HOTTIP | miR-574-5p | EZH1 | SCLC | promote cancer cell proliferation and cell cycle | 29041935 |
LINC00974 | miR-642 | KRT19 | HCC | promote cancer cell proliferation and metastasis | 25476897 |
UCA1 | miR-193a | HMGB1 | Lung cancer | promote cancer cell proliferation and migration | 29355524 |
CCAT1 | let-7 | HMGA2 and c-Myc | HCC | promote cancer cell proliferation and migration | 25884472 |
HNF1A-AS1 | miR-30b-5p | Bcl-2 | Bladder cancer | promote cancer cell proliferation and suppress cancer cell apoptosis | 29100339 |
XLOC_008466 | miR-874 | MMP2 and XIAP | NSCLC | promote cancer cell proliferation, invasion and suppress cancer cell apoptosis | 28501870 |
HIF1A-AS2 | miR-129-5p | DNMT3A | CRC | promote cancer cell proliferation, invasion and EMT formation | 29278853 |
OIP5-AS1 | miR-186a-5p | ZEB1 | Hepatoblastoma | promote cancer cell proliferation, metastasis and EMT progress | 29475118 |
HOTAIR | miR-331-3p | HER2 | GC | promote cancer cell proliferation, migration and invasion | 24775712 |
SNHG1 | miR-326 | NOB1 | Osteosarcoma | promote cancer cell growth, migration and invasion | 29115574 |
HOTAIR | miR-373 | Rab22a | OV | promote cancer cell proliferation, migration and invasion | 27484896 |
CCAT1 | miR-181b | FGFR3 and PDGFRα | Glioma | promote cancer cell proliferation, migration and the EMT process, and suppress cancer cell apoptosis | 28475287 |
H19 | miR-17-5p | YES1 | Thyroid cancer | promote cancer cell proliferation, migration, and invasion | 27093644 |
XIST | miR-137 | PXN | NSCLC | promote cancer cell viability and invasion | 29337100 |
CASC2 | miR-367 | FBXW7 | HCC | suppress cancer cell migration, invasion and EMT progression | 28716020 |
TUSC7 | miR-211 | CDK6 | CRC | suppress cancer cell proliferation | 28214867 |
Gas5 | miR-222-3p | PTEN | PTC | suppress cancer cell proliferation | 29423063 |
TINCR | miR-544a | FBXW7 | Lung cancer | suppress cancer cell proliferation and invasion | 29324317 |
PWRN1 | miR-425-5p | PTEN | GC | suppress cancer cell proliferation and metastasis and promote cancer cell apoptosis | 29535266 |
SNHG5 | miR-32 | KLF4 | GC | suppress cancer cell proliferation and migration | 27871067 |
MEG3 | miR-181a | Bcl-2 | GC | suppress cancer cell proliferation, migration, invasion, and promoted cancer cell apoptosis | 26253106 |
CASC2 | miR-183 | Sprouty2 | PCa | suppress cancer cell proliferation, promote cancer cell apoptosis, and enhance the sensitivity of PCa cancer cells to docetaxel | 29373811 |
lncRNA RP4 | miR-7-5p | SH3GLB1 | CRC | suppress cancer cell proliferation and tumor growth | 29531464 |
H19 | miR-29b-3p | DNMT3B | Bladder cancer | promote cancer EMT and metastasis | 28779971 |
H19 | miR-138/200a | Vimentin, ZEB1, and ZEB2 | CRC | promote cancer cell EMT | 26068968 |
Linc-ROR | miR-145 | ZEB2 | HCC | promote cancer cell invasion and metastasis via induction of EMT | 28680141 |
RSU1P2 | let-7a | IGF1R, N-Myc and EphA4 | Cervical cancer | promote tumorigenesis | 27487126 |
lncRNA-PAGBC | miR-133b/511 | SOX4 and PIK3R3 | GBC | promote tumorigenesis | 28887321 |
LINC01234 | miR-204-5p | CBFB | GC | promote tumorigenesis | 29386218 |
HULC | miR-200a-3p | ZEB1 | HCC | promote tumorigenesis and metastasis | 27285757 |
CCAT1 | miR-218-5p | BMI1 | GBC | promote cancer development | 25569100 |
NEAT1 | miR-506 | STAT3 | GC | promote cancer development | 29363783 |
HULC | miR-186 | HMGA2 | HCC | promote cancer growth | 28765279 |
HOXD-AS1 | miR-130a-3p | SOX4 | Liver cancer | promote cancer metastasis | 28810927 |
NORAD | miR-125a-3p | RhoA | Pancreatic cancer | promote cancer metastasis | 29121972 |
Linc-ROR | miR-145 | FSCN1 | ESCC | promote cancer metastasis and invasion | 29430188 |
Lnc-ATB | miR-141-3p | TGFβ2 | GC | promote cancer progression | 28115163 |
MIAT | miR-155-5p | DUSP7 | Breast cancer | promote cancer progression | 29100300 |
NEAT1 | miR-448 | ZEB1 | Breast cancer | promote cancer progression | 29323713 |
lincRNA-ROR | miR-145 | NA | Colon cancer | promote cancer progression | 27071407 |
HCP5 | miR-22-3p/186-5p/216a-5p | ST6GAL2 | FTC | promote cancer progression | 29515098 |
MALAT1 | miR-202 | Gli2 | GC | promote cancer progression | 27887846 |
XIST | miR-185 | TGF-β1 | GC | promote cancer progression | 29053187 |
TUG1 | miR-145 | Sirt3 | ICC | promote cancer progression | 29371936 |
CCAT1 | miR-181a-5p | HOXA1 | MM | promote cancer progression | 29228867 |
NEAT1 | miR-377-3p | E2F3 | NSCLC | promote cancer progression | 27351135 |
SNHG7 | miR-193b | FAIM2 | NSCLC | promote cancer progression | 29131440 |
UCA1 | miR-204 | ATF2 | PCa | promote cancer progression | 28337266 |
Linc-ROR | miR-205 | ZEB2 | Breast cancer | promote EMT and cancer progression and metastasis | 24922071 |
NEAT1 | miR-193b-3p | CCND1 | Cervical cancer | promote cancer radio-resistance | 29416780 |
TUG1 | miR-186 | CPEB2 | CRC | promote resistance to methotrexate treatment | 28302487 |
UCA1 | miR-195 | ARL2 | Bladder cancer | promote mitochondrial function in cancer cell | 29130995 |
HULC | miR-372 | PRKACB | HCC | NA | 20423907 |
pseudogene | |||||
INTS6P1 | miR-17-5p | INTS6 | HCC | promote cancer cell death and suppress cancer cell mobility | 25686840 |
KRAS1P | NA | K-RAS | PCa | promote cancer cell growth | 20577206 |
BRAFP1 | miR-30a, miR-876, miR-182 | BRAF | Lymphoma | promote cancer development | 25843629 |
CYP4Z2P | miR-211/125a-3p/197/1226/204 | CYP4Z1 | Breast cancer | promote tumor angiogenesis | 25701119 |
PTENP1 | miR-106b/93 | PTEN | GC | suppress cancer cell growth and induce cancer cell apoptosis | 28212532 |
PTENP1 | miR-193a-3p | PTEN | HCC | suppress cancer cell migration and invasion | 29296207 |
PTENP1 | miR-21 | PTEN | OSCC | suppress cancer cell proliferation | 27862321 |
PTENP1 | miR-19b | PTEN | Breast cancer | suppress cancer cell proliferation, migration and invasion and promote cancer cell apoptosis | 28731027 |
PTENP1 | miR-17/19b/20a | PTEN and PHLPP | HCC | suppress cancer cell proliferation, migration/invasion and induce cancer cell autophagy and apoptosis | 25617127 |
CTNNAP1 | miR-141 | CTNNA1 | CRC | suppress cancer cell proliferation and tumor growth | 27487124 |
PTENP1 | miR-21 | PTEN | ccRCC | suppress cancer progression | 25249556 |
circRNA | |||||
circRNA-MYLK | miR-29a | VEGFA | Bladder cancer | promote cancer progression | 28687357 |
circGFRA1 | miR-34a | GFRA1 | Breast cancer | promote cancer cell proliferation and suppress cancer cell apoptosis | 29037220 |
circDOCK1 | miR-196a-5p | BIRC3 | OSCC | suppress cancer cell apoptosis | 29286141 |
cir-ITCH | miR-7/20a/214 | ITCH | CRC | suppress cancer cell proliferation | 26110611 |
cir-ITCH | miR-7/17/214 | ITCH | ESCC | suppress cancer cell proliferation and tumor growth | 25749389 |
cir-ITCH | miR-17/224 | p21 and PTEN | Bladder cancer | suppress cancer progression | 29386015 |
mRNA | |||||
versican 3′ UTR | miR-133a/199a-3p/144/431 | CD34 and Fibronectin | HCC | promote cancer development | 23180826 |
XIAP 3′ UTR | miR-29a-5p | FSCN1 | Breast cancer | promote cancer progression | 28186968 |
IGF1R/ROCK2/RAP1B 3′ UTR | miR-139-5p | IGF1R/ROCK2/RAP1B | CRC | promote cancer cell proliferation | 24942287 |
AEG-1 3′ UTR | miR-30a | Vimentin and Snail | NSCLC | promote EMT | 25484183 |
FOXO1 3′ UTR | miR-9 | E-cadherin | Breast cancer | suppress cancer metastasis | 25017439 |
USP3 | miR-224 | SMAD4 | CRC | suppress cancer metastasis | 28655924 |
versican 3′ UTR | miR-136/199a-3p/144 | Rb1 and PTEN | Breast cancer | suppress cancer cell proliferation and tumor growth | 21049042 |
StarD13 3′ UTR | miR-125b | TP53INP1 | Breast cancer | suppress cancer cell migration and invasion | 29146309 |
CNOT6L/VAPA/SERINC1 | miR-17/19a/20a/b/106a/b/93 | PTEN | PCa | suppress cancer cell proliferation | 22000013 |
ZEB2 | miR-25/92a/181a/200b | PTEN | Melanoma | suppress tumorigenesis | 22000016 |
EPO/EPOR | miR-125b | ERBB2/HER2 | Breast cancer | NA | 24165569 |
ceRNA . | Shared miRNA . | Competing target . | Cancer type . | Biological effects of ceRNAs . | PMID . |
---|---|---|---|---|---|
lncRNA | |||||
Snhg1 | miR-338 | CST3 | Esophageal cancer | promote cancer cell growth | 28423738 |
UCA1 | miR-216b | FGFR1 | HCC | promote cancer cell growth and metastasis | 25760077 |
Linc-ROR | miR-145 | ARF6 | Breast cancer | promote cancer cell invasion | 25253741 |
PCAT-1 | miR-129-5p | HMGB1 | HCC | promote cancer cell invasion and metastasis | 28931210 |
H19 | miR-342-3p | FOXM1 | GBC | promote cancer cell invasion and proliferation | 27716361 |
MALAT1 | miR-1 | cdc42 | Breast cancer | promote cancer cell migration and invasion | 26926567 |
HOXD-AS1 | miR-130a | E2F8 | Glioma | promote cancer cell migration and invasion | 29341117 |
GAPLINC | miR-211-3p | CD44 | GC | promote cancer cell migration and proliferation | 25277524 |
H19 | miR-484 | ROCK2 | Lung cancer | promote cancer cell migration, invasion, and EMT process | 29219208 |
TP73-AS1 | miR-200a | TFAM | Breast cancer | promote cancer cell proliferation | 28639399 |
MALAT1 | miR-363-3p | MCL-1 | GBC | promote cancer cell proliferation | 27420766 |
BC032469 | miR-1207-5p | hTERT | GC | promote cancer cell proliferation | 26549025 |
TINCR | miR-375 | PDK1 | GC | promote cancer cell proliferation | 28744139 |
PVT1-5 | miR-126 | SLC7A5 | Lung cancer | promote cancer cell proliferation | 29277611 |
MALAT1 | miR-124 | STAT3 | NSCLC | promote cancer cell proliferation | 29215698 |
PCAT1 | miR-3667-3p | c-Myc | PCa | promote cancer cell proliferation | 25425964 |
SNHG1 | miR-199a-3p | CDK7 | PCa | promote cancer cell proliferation | 28400279 |
PVT1 | miR-199a-5p | HIF1α | NSCLC | promote cancer cell proliferation | 29115513 |
HOTTIP | miR-574-5p | EZH1 | SCLC | promote cancer cell proliferation and cell cycle | 29041935 |
LINC00974 | miR-642 | KRT19 | HCC | promote cancer cell proliferation and metastasis | 25476897 |
UCA1 | miR-193a | HMGB1 | Lung cancer | promote cancer cell proliferation and migration | 29355524 |
CCAT1 | let-7 | HMGA2 and c-Myc | HCC | promote cancer cell proliferation and migration | 25884472 |
HNF1A-AS1 | miR-30b-5p | Bcl-2 | Bladder cancer | promote cancer cell proliferation and suppress cancer cell apoptosis | 29100339 |
XLOC_008466 | miR-874 | MMP2 and XIAP | NSCLC | promote cancer cell proliferation, invasion and suppress cancer cell apoptosis | 28501870 |
HIF1A-AS2 | miR-129-5p | DNMT3A | CRC | promote cancer cell proliferation, invasion and EMT formation | 29278853 |
OIP5-AS1 | miR-186a-5p | ZEB1 | Hepatoblastoma | promote cancer cell proliferation, metastasis and EMT progress | 29475118 |
HOTAIR | miR-331-3p | HER2 | GC | promote cancer cell proliferation, migration and invasion | 24775712 |
SNHG1 | miR-326 | NOB1 | Osteosarcoma | promote cancer cell growth, migration and invasion | 29115574 |
HOTAIR | miR-373 | Rab22a | OV | promote cancer cell proliferation, migration and invasion | 27484896 |
CCAT1 | miR-181b | FGFR3 and PDGFRα | Glioma | promote cancer cell proliferation, migration and the EMT process, and suppress cancer cell apoptosis | 28475287 |
H19 | miR-17-5p | YES1 | Thyroid cancer | promote cancer cell proliferation, migration, and invasion | 27093644 |
XIST | miR-137 | PXN | NSCLC | promote cancer cell viability and invasion | 29337100 |
CASC2 | miR-367 | FBXW7 | HCC | suppress cancer cell migration, invasion and EMT progression | 28716020 |
TUSC7 | miR-211 | CDK6 | CRC | suppress cancer cell proliferation | 28214867 |
Gas5 | miR-222-3p | PTEN | PTC | suppress cancer cell proliferation | 29423063 |
TINCR | miR-544a | FBXW7 | Lung cancer | suppress cancer cell proliferation and invasion | 29324317 |
PWRN1 | miR-425-5p | PTEN | GC | suppress cancer cell proliferation and metastasis and promote cancer cell apoptosis | 29535266 |
SNHG5 | miR-32 | KLF4 | GC | suppress cancer cell proliferation and migration | 27871067 |
MEG3 | miR-181a | Bcl-2 | GC | suppress cancer cell proliferation, migration, invasion, and promoted cancer cell apoptosis | 26253106 |
CASC2 | miR-183 | Sprouty2 | PCa | suppress cancer cell proliferation, promote cancer cell apoptosis, and enhance the sensitivity of PCa cancer cells to docetaxel | 29373811 |
lncRNA RP4 | miR-7-5p | SH3GLB1 | CRC | suppress cancer cell proliferation and tumor growth | 29531464 |
H19 | miR-29b-3p | DNMT3B | Bladder cancer | promote cancer EMT and metastasis | 28779971 |
H19 | miR-138/200a | Vimentin, ZEB1, and ZEB2 | CRC | promote cancer cell EMT | 26068968 |
Linc-ROR | miR-145 | ZEB2 | HCC | promote cancer cell invasion and metastasis via induction of EMT | 28680141 |
RSU1P2 | let-7a | IGF1R, N-Myc and EphA4 | Cervical cancer | promote tumorigenesis | 27487126 |
lncRNA-PAGBC | miR-133b/511 | SOX4 and PIK3R3 | GBC | promote tumorigenesis | 28887321 |
LINC01234 | miR-204-5p | CBFB | GC | promote tumorigenesis | 29386218 |
HULC | miR-200a-3p | ZEB1 | HCC | promote tumorigenesis and metastasis | 27285757 |
CCAT1 | miR-218-5p | BMI1 | GBC | promote cancer development | 25569100 |
NEAT1 | miR-506 | STAT3 | GC | promote cancer development | 29363783 |
HULC | miR-186 | HMGA2 | HCC | promote cancer growth | 28765279 |
HOXD-AS1 | miR-130a-3p | SOX4 | Liver cancer | promote cancer metastasis | 28810927 |
NORAD | miR-125a-3p | RhoA | Pancreatic cancer | promote cancer metastasis | 29121972 |
Linc-ROR | miR-145 | FSCN1 | ESCC | promote cancer metastasis and invasion | 29430188 |
Lnc-ATB | miR-141-3p | TGFβ2 | GC | promote cancer progression | 28115163 |
MIAT | miR-155-5p | DUSP7 | Breast cancer | promote cancer progression | 29100300 |
NEAT1 | miR-448 | ZEB1 | Breast cancer | promote cancer progression | 29323713 |
lincRNA-ROR | miR-145 | NA | Colon cancer | promote cancer progression | 27071407 |
HCP5 | miR-22-3p/186-5p/216a-5p | ST6GAL2 | FTC | promote cancer progression | 29515098 |
MALAT1 | miR-202 | Gli2 | GC | promote cancer progression | 27887846 |
XIST | miR-185 | TGF-β1 | GC | promote cancer progression | 29053187 |
TUG1 | miR-145 | Sirt3 | ICC | promote cancer progression | 29371936 |
CCAT1 | miR-181a-5p | HOXA1 | MM | promote cancer progression | 29228867 |
NEAT1 | miR-377-3p | E2F3 | NSCLC | promote cancer progression | 27351135 |
SNHG7 | miR-193b | FAIM2 | NSCLC | promote cancer progression | 29131440 |
UCA1 | miR-204 | ATF2 | PCa | promote cancer progression | 28337266 |
Linc-ROR | miR-205 | ZEB2 | Breast cancer | promote EMT and cancer progression and metastasis | 24922071 |
NEAT1 | miR-193b-3p | CCND1 | Cervical cancer | promote cancer radio-resistance | 29416780 |
TUG1 | miR-186 | CPEB2 | CRC | promote resistance to methotrexate treatment | 28302487 |
UCA1 | miR-195 | ARL2 | Bladder cancer | promote mitochondrial function in cancer cell | 29130995 |
HULC | miR-372 | PRKACB | HCC | NA | 20423907 |
pseudogene | |||||
INTS6P1 | miR-17-5p | INTS6 | HCC | promote cancer cell death and suppress cancer cell mobility | 25686840 |
KRAS1P | NA | K-RAS | PCa | promote cancer cell growth | 20577206 |
BRAFP1 | miR-30a, miR-876, miR-182 | BRAF | Lymphoma | promote cancer development | 25843629 |
CYP4Z2P | miR-211/125a-3p/197/1226/204 | CYP4Z1 | Breast cancer | promote tumor angiogenesis | 25701119 |
PTENP1 | miR-106b/93 | PTEN | GC | suppress cancer cell growth and induce cancer cell apoptosis | 28212532 |
PTENP1 | miR-193a-3p | PTEN | HCC | suppress cancer cell migration and invasion | 29296207 |
PTENP1 | miR-21 | PTEN | OSCC | suppress cancer cell proliferation | 27862321 |
PTENP1 | miR-19b | PTEN | Breast cancer | suppress cancer cell proliferation, migration and invasion and promote cancer cell apoptosis | 28731027 |
PTENP1 | miR-17/19b/20a | PTEN and PHLPP | HCC | suppress cancer cell proliferation, migration/invasion and induce cancer cell autophagy and apoptosis | 25617127 |
CTNNAP1 | miR-141 | CTNNA1 | CRC | suppress cancer cell proliferation and tumor growth | 27487124 |
PTENP1 | miR-21 | PTEN | ccRCC | suppress cancer progression | 25249556 |
circRNA | |||||
circRNA-MYLK | miR-29a | VEGFA | Bladder cancer | promote cancer progression | 28687357 |
circGFRA1 | miR-34a | GFRA1 | Breast cancer | promote cancer cell proliferation and suppress cancer cell apoptosis | 29037220 |
circDOCK1 | miR-196a-5p | BIRC3 | OSCC | suppress cancer cell apoptosis | 29286141 |
cir-ITCH | miR-7/20a/214 | ITCH | CRC | suppress cancer cell proliferation | 26110611 |
cir-ITCH | miR-7/17/214 | ITCH | ESCC | suppress cancer cell proliferation and tumor growth | 25749389 |
cir-ITCH | miR-17/224 | p21 and PTEN | Bladder cancer | suppress cancer progression | 29386015 |
mRNA | |||||
versican 3′ UTR | miR-133a/199a-3p/144/431 | CD34 and Fibronectin | HCC | promote cancer development | 23180826 |
XIAP 3′ UTR | miR-29a-5p | FSCN1 | Breast cancer | promote cancer progression | 28186968 |
IGF1R/ROCK2/RAP1B 3′ UTR | miR-139-5p | IGF1R/ROCK2/RAP1B | CRC | promote cancer cell proliferation | 24942287 |
AEG-1 3′ UTR | miR-30a | Vimentin and Snail | NSCLC | promote EMT | 25484183 |
FOXO1 3′ UTR | miR-9 | E-cadherin | Breast cancer | suppress cancer metastasis | 25017439 |
USP3 | miR-224 | SMAD4 | CRC | suppress cancer metastasis | 28655924 |
versican 3′ UTR | miR-136/199a-3p/144 | Rb1 and PTEN | Breast cancer | suppress cancer cell proliferation and tumor growth | 21049042 |
StarD13 3′ UTR | miR-125b | TP53INP1 | Breast cancer | suppress cancer cell migration and invasion | 29146309 |
CNOT6L/VAPA/SERINC1 | miR-17/19a/20a/b/106a/b/93 | PTEN | PCa | suppress cancer cell proliferation | 22000013 |
ZEB2 | miR-25/92a/181a/200b | PTEN | Melanoma | suppress tumorigenesis | 22000016 |
EPO/EPOR | miR-125b | ERBB2/HER2 | Breast cancer | NA | 24165569 |
GC: gastric cancer; CRC: colorectal cancer; FTC: follicular thyroid carcinoma; PTC: papillary thyroid carcinoma; PCa: prostate cancer; GBC: gallbladder cancer; ICC: intrahepatic cholangiocarcinoma; MM: multiple myeloma; SCLC: small cell lung cancer; HCC: hepatocellular carcinoma; ESCC: esophageal squamous cell carcinoma; ccRCC: clear-cell renal cell carcinoma; OSCC: oral squamous cell carcinoma; NSCLC: non-small cell lung cancer; OV: ovarian cancer; EMT: epithelial-to-mesenchymal transition.
Overall, ceRNAs in cancers can be functionally classified as: (1) promoting/suppressing cancer cell proliferation, migration and invasion. For example, HOTAIR functions as ceRNA to promote cell proliferation, migration and invasion in ovarian cancer [28] and gastric cancer [29], while MEG3 serves as ceRNA to control gastric cancer progression by inhibiting cell proliferation, migration and invasion [30]. (2) promoting/suppressing cancer cell EMT. H19 has been found as an oncogenic ceRNA to regulate EMT in multiple cancer types including colorectal cancer [25], bladder cancer [31] and lung cancer [32]. (3) promoting/suppressing tumorigenesis. lncRNA RSU1P2 [33], lncRNA-PAGBC [34] and LINC01234 [35] were found to promote tumorigenesis by acting as ceRNA, while ZEB2 could suppress tumorigenesis in melanoma via ceRNA mechanism [36]. (4) promoting/suppressing cancer progression, metastasis or development. For example, lncRNA TUG1 [37], and NEAT1 [38] have been identified as crucial ceRNAs in promoting cancer progression and development, respectively, while circRNA cir-ITCH could inhibit bladder cancer progression by functioning as ceRNA [26]. (5) promoting/suppressing sensitivity to drug treatment. For example, lncRNA CASC2 could increase the sensitivity of prostate cancer cells to docetaxel [39], and lncRNA TUG1 could enhance the resistance of colorectal cancer to methotrexate treatment [40] (Table 1).
Molecular mechanisms of ceRNAs in cancer
According to the ceRNA hypothesis, RNA molecules that share MREs can serve as natural decoys for miRNAs, thereby up-regulating miRNA target gene expression (Figure 2B). However, whether ceRNA crosstalk can occur under physiological cellular conditions is influenced by multiple factors, such as the expression level and subcellular localization of ceRNA components, miRNA/ceRNA affinity, and the number and location of MREs. Accordingly, a growing number of studies have explored the crucial determinants of ceRNA crosstalk [41].
Relative abundance and subcellular localization of miRNAs, ceRNAs and Argonaute
The expression levels of miRNAs and ceRNAs are important determinants of ceRNA activity. Indeed, significant changes in ceRNA abundance are needed to substantially inhibit miRNA repression on target genes [8]. Wee et al. [42] analyzed the effect of miRNA and its sponge RNA concentration on miRNA activity and proposed that a ceRNA can only function when its miRNA partner is at moderate or low abundance; the most abundant miRNAs are not susceptible to be regulated by ceRNAs. A study on the optimal condition for ceRNA activity using a mathematical mass-action model showed that the optimal ceRNA-mediated crosstalk occurred when miRNA and ceRNA levels were near equimolar; this was validated experimentally by using the paradigm of PTEN and its ceRNA VAPA [43]. As an essential component of the RNA-induced silencing complex (RISC), argonaute (AGO) plays a central role in miRNA-mediated gene expression regulation [42]. Competition for AGO has been shown to affect the emergence of ceRNA crosstalk [44]. Thus, altering AGO abundance may also affect ceRNA activity.
Additionally, as subcellular localization can influence the physical accessibility of ceRNAs to corresponding miRNAs [45], effective ceRNA-mediated regulation requires cellular co-localization of ceRNA network components including sponge RNAs and shared miRNAs. Thus, overlapping subcellular localization is necessary for effective ceRNA crosstalk.
miRNA/ceRNA binding affinity
Another factor that influences ceRNA activity is the miRNA/ceRNA binding affinity, which is primarily determined by the extent of base pairing between the ceRNA MRE and the miRNA seed region (nucleotides 2–8 at the 5′ end of the miRNA). According to their preferential complementarity to miRNA seed regions, miRNA target sites can be classified into three canonical types: 8mer sites (base pairing to miRNA positions 2–8 with an A opposite position 1), 7mer sites (base pairing to miRNA positions 2–8 or positions 2–7 with an A opposite position 1) and 6mer sites (base pairing to miRNA positions 2–7) [46, 47]. The most effective canonical miRNA complementary sites are the 8mer sites, followed by 7mer and 6mer sites [48]. Denzler et al. [49] found that 7mer and 6mer sites were 50% and 20% as effective as 8mer site in competing for miRNA binding, respectively. Thus, ceRNAs containing high-affinity MREs tend to be more effective in sequestrating miRNAs.
Besides the degree of complementarity between MREs and miRNA seed regions, the number and location of MREs also impact ceRNA activity. It is conceivable that a ceRNA containing more MREs would have stronger ability in competitively binding miRNAs. Moreover, Denzler et al. found that proximal MREs could act cooperatively to sequester miRNA and therefore influence the potency of ceRNA-mediated gene de-repression. For example, the de-repression of let-7 targets is strengthened by let-7 MREs harboring a adjacent miR-122 site [49].
RNA editing
RNA editing is broadly defined as a post-transcriptional process that introduce changes in RNA sequences through site-specific insertion, deletion or base substitution of nucleotides, excluding events such as RNA splicing and polyadenylation [50]. In higher eukaryotes, the most prevalent type of RNA editing is adenosine-to-inosine (A-to-I) editing, which is catalyzed by adenosine deaminase acting on RNA (ADAR) enzymes [51]. Given the important role in altering miRNA and target sequences, RNA editing could influence ceRNA crosstalk in the following ways. First, editing within miRNAs can alter their sequence, processing, expression level and/or target spectrum, which in turn can impact miRNA-mediated gene regulation. Yang et al. [52] found that editing of the miRNA-142 precursor by ADAR1 and ADAR2 suppressed its processing, leading to decreased miRNA-142 expression level. Kawahara et al. [53] found that a single A-to-I conversion in the middle of miR-376 seed region redirected it to silence a set of target genes that were not repressed by the unedited miRNA. Second, RNA editing could affect ceRNA crosstalk by modifying MREs in ceRNA sequences. Several studies have shown that numerous pre-mRNAs in the human transcriptome are subject to A to I editing, which often occurred in their introns and 5′ and 3′ UTRs [54, 55]. As MREs are also frequently located in 3′ UTRs, RNA editing may change the MRE sequences binding to miRNAs of interest [56].
Interplay between miRNAs and RNA-binding proteins
Interaction between miRNAs and RNA-binding proteins (RBPs) also influence ceRNA activity, as RBPs play critical roles in multiple post-transcriptional processes including RNA splicing, localization, degradation and translation efficiency [57]. An increasing number of studies have shown that RBPs (e.g. HUR) can cooperate or compete with miRNAs to down-regulate target genes [58, 59]. Furthermore, RBPs may block miRNA-ceRNA binding by occupying miRNA-binding sites, as RBP binding sites are frequently enriched near miRNA-binding sites or overlap with them. Lebedeva et al. [60] found that HuR and miRNA binding sites tended to reside nearby, and that HuR knockdown specifically up-regulated miR-7 expression.
In summary, the molecular mechanism of ceRNA is that RNA molecules with shared miRNA binding sites could co-regulate each other by reducing the activity of the bound miRNA via sequestration. Notably, the effectiveness of ceRNA-mediated gene regulation under physiological cellular conditions is mainly determined by the relative abundance of ceRNAs and their shared miRNA. Other factors such as miRNA/ceRNA binding affinity, RNA editing and interplay between miRNA and RBP could also influence ceRNA activity. Given that ceRNAs could sponge miRNAs with unequal efficiency [61], ceRNA function may vary greatly under different cellular conditions.
ceRNA network reconstruction
Identifying miRNA-mediated ceRNA networks opens a holistic window to explore the biological functions and regulatory mechanisms of both miRNAs and ceRNAs. Combining computational prediction with experimental validation increases the efficiency and accuracy of systems-level detection of ceRNA–ceRNA associations.
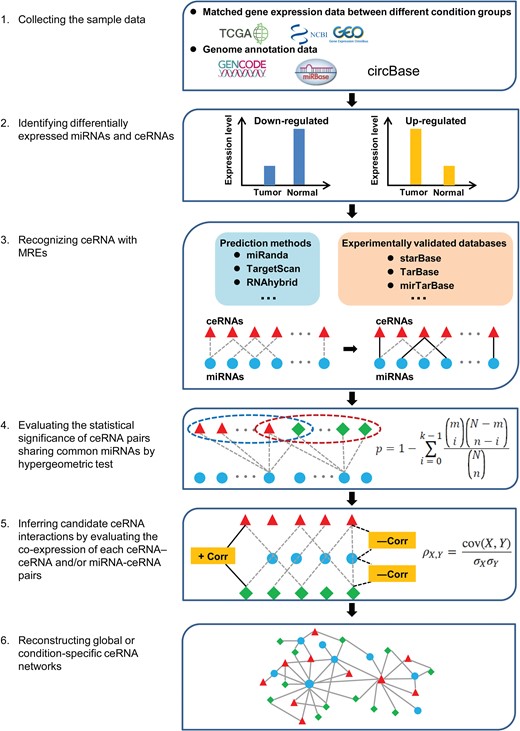
Integrative pipeline for reconstructing ceRNA networks at the transcriptome-wide level. The reconstruction of ceRNA network involves six steps, wherein differentially expressed miRNAs/ceRNAs are extracted based on the selected sample data, and putative miRNA-ceRNA associations are inferred and integrated to construct global/condition-specific networks via statistical analyses.
Data integration and ceRNA network reconstruction
According to computational approach, ceRNA network mainly included two types: global ceRNA network consisting of RNA pairs with co-regulating miRNAs and context-specific ceRNA network consisting of RNA pairs not only sharing common miRNAs but also with positive expression correlations in specific condition or cancer type. The process of reconstructing context-specific ceRNA networks using computational methods can be largely divided into six steps: (1) collecting sample data, (2) identifying differentially expressed miRNAs and ceRNAs, (3) recognizing ceRNAs with MREs, (4) evaluating the statistical significance of ceRNA pairs sharing common miRNAs, (5) inferring candidate ceRNA interactions and (6) reconstructing global or condition-specific ceRNA networks (Figure 3) [62, 63].
To explore the role of ceRNAs in cancer initiation and progression, matched gene expression data under different biological backgrounds (e.g. tumors and adjacent normal controls) are primarily chosen for ceRNA network reconstruction. These data can be initially generated by high-throughput sequencing or downloaded from publicly available data repositories, such as TCGA [64] and GEO [65]. Due to individual differences, it should be noted that the collected datasets are from a sufficiently large number of human samples to be statistically meaningful. Additionally, the GENCODE project [66], miRBase [67] and circBase [68] can be used for functional annotation during data processing. As RNA molecules with altered expression pattern were more likely associated with specific biological process, those differentially expressed miRNAs, lncRNAs, circRNAs and mRNAs are supposed to be identified based on statistical analyses in the next step. Notably, multiple methods (edgeR, DESeq and cuffdiff) developed to identify differentially expressed genes may produce distinct results.
The crosstalk among ceRNAs functions through competitive binding to shared miRNAs. Similar to miRNA-target recognition, predicting ceRNAs initially relies on the principle of complementary base-pairing between miRNA seed region and putative ceRNA MREs. Currently, multiple miRNA-target prediction algorithms, including miRanda [69], TargetScan [47], RNAhybrid [70] and RNA22 [71], have been widely used to identify ceRNAs by considering a variety of factors, such as complementarity to the miRNA seed region, evolutionary conservation of binding sites, free energy of the miRNA-ceRNA heteroduplex and flanking secondary structures. Although advances in computational algorithms has been made to improve the accuracy of prediction, the most commonly used miRNA-target prediction algorithms still have more than 40% false positive and false negative rates [72]. Predicted miRNA-ceRNA interactions should be further filtered by experimental validation, as introduced by Starbase v2.0 [73], Tarbase [74] and mirTarBase [75]. In addition, although the combination of prediction algorithms and experiment-supported databases could facilitate the prediction of ceRNA interactions with relatively high accuracy, in practice, there still lacks a gold standard for effective selection of optimal combination methods.
As the crosstalk between ceRNAs was mediated by sharing miRNAs, the statistical significance of each ceRNA pair on sharing common miRNAs can be calculated by a hypergeometric test before network reconstruction. In some cases, only ceRNA pairs that share significant number of miRNAs are selected for further analysis.
According to the ‘ceRNA hypothesis’, the expression correlation information between interacting ceRNA pairs and between ceRNA-miRNA pairs could strengthen the knowledge for computer-aided ceRNA interaction extraction. Notably, it is well known that miRNAs could regulate gene expression at post-transcriptional level by targeting mRNAs for cleavage or translational repression. At the level of co-expression analysis, the miRNAs and their target mRNAs do not always show negative co-expression when miRNA functions via translational repression rather than mRNA degradation. Therefore, according to the research purpose, the conditions for negative correlation between miRNA and target gene are optional when constructing the network. Here, three types of computational approaches are mainly used: (1) pair-wise correlation approach, (2) partial association approach and (3) mathematical modeling approach [76]. The principle of the pair-wise correlation approach is that expression levels of each pair of interacting ceRNAs in a network have a significant positive correlation, which can be measured by Pearson correlation coefficients. For example, Cao et al. [63] identified the miRNA-mediated ceRNA crosstalk between lncRNAs and mRNAs in glioblastoma multiforme by integrating genome-wide lncRNA and mRNA expression data. The main limitation of the first category of methods is that it ignores the expression correlation between ceRNAs and shared miRNAs. Accordingly, partial association methods combine correlations between ceRNAs and the expression levels of miRNAs shared by two candidate ceRNAs to investigate their interactive relationships. Statistically, given the presence of miRNAs, partial correlation methods could be used to evaluate linear relationships between ceRNAs, whereas conditional mutual information (CMI) methods can quantify nonlinearly direct dependencies between ceRNAs [77, 78]. JAMI, an open-source software based on Java, was recently developed to accelerate the computation of ceRNA network analysis via CMI [79]. Based on partial association methods, Zhou et al. [80] constructed a global and progression-related ceRNA network for ovarian cancer by integrating matched miRNA, mRNA and lncRNA expression data. As the term suggests, mathematical modeling approaches focus on predicting ceRNA interactions using different mathematical models. Bosia et al. [81] formulated a stochastic model to characterize the equilibrium and out-of-equilibrium properties of a miRNA-ceRNA interaction network, indicating the way by which ceRNAs and miRNAs could correlatively fluctuate under complex conditions, such as feedback or feedforward loops. Swain et al. [82] proposed a score of competitive endogenous RNA model to predict potential ceRNA pairs and evaluate their sequestering ability based on the ‘tug of war’ concept. Because the large number of components in ceRNA networks can increase the algorithmic complexity of the mathematical models, the methods of the third category is often applicable to small ceRNA network construction. Following the prediction of ceRNA interactions, Cytoscape software can be used to construct and visualize the network based on the indicated ceRNA interactions. It should be noted that further development, optimization and comparative analysis of bioinformatic methods for constructing ceRNA network is needed to provide more insight into ceRNA regulation.
Experimental approaches for identifying and validating ceRNA interactions
Biochemical techniques including miRNA pull-down, high-throughput sequencing of RNA isolated by crosslinking immunoprecipitation (HITS-CLIP), photoactivatable-ribonucleoside-enhanced crosslinking and immunoprecipitation, crosslinking, ligation and sequencing of hybrids, and MS2-tagged RNA affinity purification, have enabled large-scale experimental identification of ceRNAs through detecting endogenous miRNA–RNA interactions [22]. For example, Chan et al. identified ferritin heavy chain 1 (FTH1) as well as its multiple pseudogenes to be the targets of oncogenic miR-638 in prostate cancer by using the miRNA pull-down assay. They uncovered that the ceRNA network of FTH1:pseudogene:miRNA plays an important role in the development and progression of prostate cancer by modulating iron homeostasis [83]. Chi et al. employed HITS-CLIP to extensively identify miRNA-mRNA interactions in the mouse brain, and their studies showed that miRNA binding sites could locate within both the 3′ UTRs and coding sequences [84]. Although miRNA pull-down assay has been widely adopted for probing direct miRNA-target interaction, the limitation of miRNA pull-down assay is that there may be background noise due to binding with non-specific targets and the qPCR-based quantitative strategy is relatively low-throughput [85]. Therefore, miRNA pull-down assay is suitable for validation of one or several miRNA-target pairs of interest. Comparatively, HITS-CLIP is a powerful approach capable of identifying AGO-associated miRNAs and their target MREs on a genome-wide scale [86]. Additionally, the experimental identification and functional validation of individual ceRNAs generally comprises the following several steps: (1) the miRNA-ceRNA interaction should be confirmed by experimental approaches, such as miRNA pull-down assay, luciferase assay or RNA immunoprecipitation [87], (2) the positive co-expression of candidate ceRNA pairs could be investigated by ceRNA overexpression and knockdown (e.g. CRISPR, RNAi) experiments and the results of both should be consistent. Notably, dramatical alteration of ceRNA abundance is required to validate their positive expression correlation and CRISPR experiments may produce off-target effects. Furthermore, the correlated expression should also be confirmed in Dicer-knockout cell lines to test whether the observed ceRNA reciprocal regulations are mediated by miRNAs. Finally, ceRNA function can be explored in vivo (e.g. cell and mouse models) by overexpression and knockout of ceRNAs [61, 88]. Overall, to gain solid results, the function and mechanism of ceRNAs need to be investigated by more than two methods in vitro or in vivo.
Biomarker identification based on ceRNA network analysis
Systematic classification of biomarkers
Biomarkers play an increasingly important role in the diagnosis, subtyping, prognosis and therapy of a broad spectrum of cancers [11]. According to systems biology paradigms, molecular biomarkers are classified into single molecular biomarkers, module biomarkers and network biomarkers [89]. In general, both genetic and epigenetic factors can serve as single molecular biomarkers, such as DNA mutation and methylation, and the abnormal expression of genes, ncRNAs and proteins. Although a large number of single molecular biomarkers have been adopted into clinical practice, they are still too limited to indicate the dynamic nature of living organisms. To address this issue, interactions or associations among individual molecules have been integrated as module biomarkers or network biomarkers, which consist of multiple functional meta-modules. Compared with single molecular biomarkers, module and network biomarkers offer multi-dimensional information for cancer prediction; therefore, they are more sensitive and robust for decoding carcinogenesis at a systems-level [90].
ceRNA network-based biomarker identification and characterization
Recent advances in high-throughput sequencing technology have enabled large-scale screening of protein-coding RNAs and ncRNAs in tissues and blood from patients that are applicable for biomarker discovery. However, the methods traditionally used to identify cancer biomarkers were strictly dependent on gene expression pattern, therefore, they were unable to describe the underlying interactions among different biological players. Consequently, gene signatures for patients with the same clinical types from different research groups tended to be highly heterogeneous [91]. We assume that biomarker identification based on systems-guided ceRNA network analysis will provide more reliable signatures for cancer management, which is an attractive way towards precision medicine. The methodology of computational strategies for ceRNA network-driven biomarker discovery primarily consists of biomarker identification and validation steps (Figure 4).
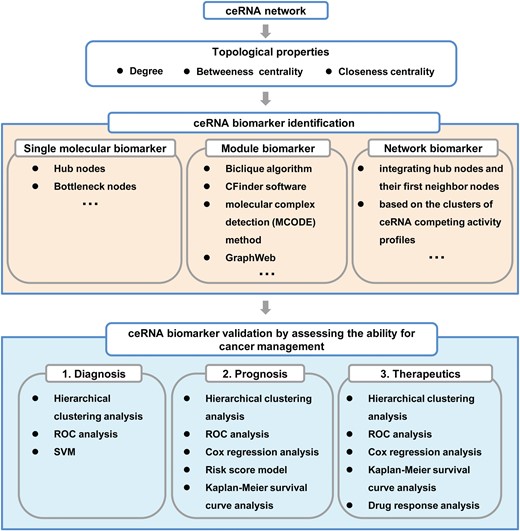
Computational strategies for biomarker discovery based on ceRNA network analysis.
Biomarker identification
Topological properties including degree, betweenness centrality and closeness centrality can be analyzed to characterize reconstructed ceRNA networks and identify key ceRNA nodes [63]. Generally, cancer-associated ceRNA networks tend to be scale-free networks, in which the nodes closely follow a power-law degree distribution [10]. According to network properties, the hub and bottleneck nodes are typically defined as the top 5–10% highest degree nodes and 5–10% highest betweenness centrality nodes, respectively. These nodes always play critical roles in ceRNA networks, and thereby could be considered as candidate single molecular biomarkers. For example, the lncRNA MCM3AP-AS possesses both hub and bottleneck node characteristics, and was identified as a prognostic biomarker in glioblastoma [63]. To further explore module biomarkers, the methods including the Biclique algorithm [62, 63, 94], CFinder software [95, 96], molecular complex detection [80] and GraphWeb [97] can be employed to extract functional modules from ceRNA networks. For example, He et al. identified a ceRNA module comprising 18 miRNAs and 1 lncRNA in laryngeal cancer using CFinder software. They found that this ceRNA module could divide patients into two major subgroups with significantly different survival outcomes, and therefore can be used as a potential prognostic biomarker for laryngeal cancer [95]. Furthermore, to gain a more detailed picture of the extensive regulatory ceRNA–ceRNA and miRNA–ceRNA interactions, sub-networks can be derived from the global ceRNA network by integrating hub nodes and their directly interacting first neighbor nodes [95], or based on the clusters of ceRNA competing activity profiles [62].
Notably, one specific cancer could involve a number of miRNAs, mRNAs, lncRNAs and even pseudogenes. For example, hepatocellular carcinoma is associated with thousands of mRNAs and lncRNAs [98], as well as more than 300 human miRNAs according to the human microRNA disease database (HMDD v3.0) [92]. As a result, there will be a large number of ceRNAs for hepatocellular carcinoma. Therefore, it is of great significance to evaluate which ceRNAs are the most important ones that need further investigations. Fortunately, two computational algorithms, Gene Importance Calculator (GIC) and microRNA essentiality score (miES), has been developed to efficiently measure the essentiality of mRNA, lncRNA and miRNA with excellent performance (AUC ≥ 0.9) [99, 100]. Therefore, applying those methods will dramatically contribute to narrow down the huge number of ceRNAs and characterize ceRNAs with important functions.
Biomarker validation
As the promise of biomarkers for assessing clinical outcomes, hierarchical clustering analysis has often been employed to investigate the power of identified ceRNA biomarkers for distinguishing subgroups of samples (e.g. cancers versus healthy controls) based on expression patterns [80]. Moreover, the sensitivity and specificity of identified biomarker candidates can be calculated through receiver operating characteristic curve analysis [95, 96]. Regarding prognostic biomarkers, ceRNAs can be fitted in a multivariate Cox regression model with overall survival time as the dependent variable and module genes as co-variables. Moreover, a risk score model of ceRNA molecules can be constructed based on the score measured by the regression coefficients derived from the multivariate Cox regression analysis. Then, patients are randomly divided into training and test datasets, in which the high-risk group should have significantly shorter survival time than low-risk group, as shown in Kaplan–Meier survival curves [95]. With respect to therapy-responsive ceRNA biomarkers, it’s also essential to perform drug response analysis [101]. Furthermore, the potential of identified ceRNA biomarkers could be validated using other independent datasets.
Translational research of ceRNA biomarkers for cancer management
Currently, the potential applications of ceRNA biomarkers to cancer research are a hot topic, especially in terms of cancer diagnosis, prognosis and drug response evaluation (Figure 4). For example, ceRNA biomarkers can be used to clinically diagnose cancer patients. Shao et al. constructed a dysregulated ceRNA network in lung adenocarcinoma by integrating miRNA regulation with the expression data of cancerous and normal tissues. They found that the module with lncRNA RP11-457 M11.2 as a hub gene can serve as a promising diagnostic biomarker with the average AUC value of 0.95 [102]. Similarly, Zhou et al. developed a 7-lncRNA signature that could significantly distinguish pancreatic ductal adenocarcinoma from nonmalignant pancreas samples with high performance based on the construction of a dysregulated lncRNA-associated ceRNA network [103].
Additionally, ceRNA biomarkers play important roles in cancer prognosis. For example, Sui et al. constructed a lung squamous cell carcinoma-specific ceRNA network using the RNA-seq data from TCGA database. They found a 2-lncRNA (FMO6P and PRR26) signature that could serve as a candidate prognostic biomarker for predicting the overall survival of lung squamous cell carcinoma patients [104]. Zhao et al. identified a ceRNA module comprising 12 lncRNAs, 2 miRNAs and 15 mRNAs in pancreatic adenocarcinoma using a ceRNA network-driven method. They found that this ceRNA module could divide patients into two subgroups with significantly different survival outcomes [97]. Similarly, Wang et al. identified nine cancer-specific sub-networks based on a global lncRNA-associated ceRNA network. They further discovered that the H19- and BRCA1/2-associated ceRNA module (HB_module) in the BRCA sub-network could distinguish two groups of breast cancer patients with different clinical outcomes, as shown by the Kaplan–Meier survival curves; therefore the HB_module could act as potential prognostic biomarkers for breast cancer [62].
Furthermore, the current effectiveness of chemotherapy for cancer is largely limited by individual differences in drug responses; thus, predicting individual responses will improve treatment and guide personalized therapy. A recent study by Zhang et al. revealed that ceRNA biomarkers can be used to predict and monitor clinical drug responses. Individual drug response was predicted based on the pan-cancer ceRNA network by integrating the expression profiles of miRNA, lncRNA and mRNA with the survival data of patients treated with drugs. They found that the dysregulation of drug response-related ceRNA (DRCE) expression could affect individual responses to cisplatin by influencing drug response-associated pathways. Moreover, a case study in breast invasive carcinoma showed that two DRCEs (NEAT1_hsa-miR-130b_TP53INP1 and NEAT1_hsa-miR-18a_NBR1) have the potential to modulate the effect of tamoxifen in treating patients with TP53 mutation [101].
Discussion and perspective
With the recent advances in theoretical and experimental techniques, unprecedented progress has been made in ceRNA discovery and characterization for cancer. Accumulating efforts have proven that protein-coding genes and transcribed ncRNAs such as lncRNA, circRNA and pseudogenes held the power as oncogenes or tumor suppressors through their ceRNA activity (Table 1). Computational methods, such as GIC [99] and miES [100], could be used to narrow down the huge number of ceRNAs by selecting essential RNA molecules. Several studies have revealed that the perturbation of ceRNA networks could result in cancer initiation and progression [26, 27, 36, 43, 87, 105]. For example, Estrogen receptor β has been shown to promote renal cell carcinoma proliferation and invasion by modulating the lncRNA HOTAIR:miR-128/200c/204/217:oncogene (e.g. ADAM9, CCND2, EZH2)-associated ceRNA network [106]. Based on the mathematical model (MAT3UTR) analysis, Park et al. [107] found that tumor-suppressor genes could be repressed `in trans’ through mRNA 3′ UTR shortening-mediated ceRNA network disruption. Thus, the understanding of gene regulation based on miRNA-mediated ceRNA interactions not only facilitates the characterization of non-coding transcriptome, but also benefits the decoding of cancer pathogenesis.
Precision medicine seeks to optimize clinical treatment decisions by classifying individual patients, which heavily relies on identifying biomarkers [108]. As multiple components of a perturbed regulatory network may contribute to carcinogenesis as well as therapeutic response, molecular interactions within networks have advantages over individual genes alone in providing clinically meaningful information [109]. Indeed, systems biology-based network analysis has been employed to identify biomarkers related to breast cancer metastasis with higher accuracy compared with those without network selection [110]. In particular, biomarkers identified based on analyses of ceRNA network properties (e.g. topological parameters, dynamical features and interactive relations), could greatly contribute to developing computational strategies and synergistic drug combinations for cancer management [10], thereby facilitating the advancing progress of precision medicine. First, using discriminating profiles between different sample groups (e.g. cancer and normal controls), single ceRNAs, ceRNA modules or subnetworks identified on the basis of network reconstruction have been increasingly used as biomarkers for early cancer diagnosis [102, 103]. Second, prognosis is an important aspect considered when making clinical decisions in precision medicine. A growing number of studies have shown that ceRNA network-based biomarkers could improve our understanding of tumor biology by stratifying clinically informative cancer subgroups (e.g. high-risk and low-risk subgroups) with distinct prognostic features, such as survival time and metastasis risk [62, 96–97, 111]. Third, ceRNA network-based biomarkers are powerful tools for systematical evaluating drug responses [101], thereby promoting the development of synergistic drug combinations that improve therapeutic responses and reduce the risk of drug resistance. Furthermore, emerging evidence has shown that ceRNAs also play important roles in other diseases, such as cardiovascular diseases [112]. Thus, with the elucidation of ceRNA mechanisms, systems-based ceRNA biomarkers will not only provide useful information in guiding clinical decision-making, but also facilitate the selection of applicable therapeutic or interventional strategies for patients with cancer and other diseases in the emerging era of precision medicine.
Compared with networks without ceRNAs, the analysis of ceRNA network could improve the understanding of carcinogenesis in the following aspects. Firstly, based on ceRNA network analysis, different types of biomarkers could be identified for cancer management. Compared with networks solely considering miRNAs or mRNAs, single miRNA/lncRNA biomarkers and ceRNA-ceRNA module biomarkers could be reasonably detected through integrative ceRNA network analysis. For example, Shao et al. [113] only identified single miRNA biomarkers for lung adenocarcinoma diagnosis by miRNA-mRNA regulatory network analysis, while Sui et al. [111] and Shao et al. [102], respectively, managed to discover lncRNA biomarkers and even ceRNA module biomarkers for lung cancer through ceRNA-network driven methods. Secondly, in methodology, ceRNA-based network analysis would help develop new methods and approaches that cater the biological rules for biomarker mechanism study. For example, in ceRNA networks, the regulation among miRNAs and mRNAs and the competition from ceRNAs could be functionally investigated and weighted, which were important for the understanding of cancer pathogenesis [10]. Thirdly, due to the complexity of biological processes, the analysis of ceRNA-based network was propitious to decoding carcinogenesis at the systems biology level. The interactions among different biological molecules covered broad ranges of biological activities and states. Thus, the analysis of ceRNA could bring the dynamical insights in cancer development and improve the predictive power of methods for prognostic study.
However, there are some key questions about the current ceRNA theory to be addressed. First, as ceRNA activity is influenced by multiple factors, such as the abundance of ceRNA components, miRNA/ceRNA affinity, RNA editing and interactions with RBPs and TFs, a precise understanding of the physiological cellular conditions in which ceRNA interactions can effectively occur is of critical importance [22, 23]. Second, the molecular mechanism by which dysregulation of ceRNAs leads to the dramatic perturbation of ceRNA crosstalk in specific cancer condition needs to be carefully elucidated in vivo [61]. Moreover, for clinical practice, the contribution of ceRNA biomarkers in cancer pathogenesis should be comprehensively evaluated in model animals, especially regarding tumor heterogeneity.
In summary, emerging data from studies exploring the function and mechanism of ceRNAs have dramatically increased our understanding of how gene expression is regulated by miRNAs. Despite the fact that the clinical implementation of biomarkers discovered from ceRNA network analysis remains a challenge, they hold great potential for providing global insight into the regulation of cell physiology and cancer pathogenesis, thereby improving molecular therapeutic approaches for precision medicine.
ceRNAs, endogenous transcripts sharing MREs, have emerged as an important class of post-transcriptional regulators in cancer pathogenesis by acting as miRNA sponges.
Perturbation of key components in ceRNA networks (e.g. ceRNAs and miRNAs), could contribute to carcinogenesis by affecting the robustness of the network system.
Compared with traditional methods, cancer biomarkers identified by systems biology level ceRNA network analysis have promising advantages in precision medicine under a holistic framework of gene regulation.
ceRNA biomarkers will be powerful for clinical cancer management in the future, including early diagnosis, prognosis, tracking and evaluating therapeutic responses in the era of precision medicine.
Funding
National Key Research & Development Program of China (2016YFC1306605), the National Natural Science Foundation of China (grant Nos. 31670851, 31470821, 91530320, 31770903, 31600670), and Jiangsu Planned Projects for Postdoctoral Research Funds (2018K173C).
Xin Qi is a post-doctor at Center for Systems Biology, Soochow University. Her research interest is biomarker discovery based on competing endogenous RNA network analysis.
Yuxin Lin is a PhD candidate at Center for Systems Biology, Soochow University. His research interest is in developing computational models for molecular biomarker discovery.
Jiajia Chen is an associate professor at School of Chemistry, Biology and Material Engineering, Suzhou University of Science and Technology. Her research interest is computational systems biology.
Bairong Shen is a professor and executive director at Institutes for Systems Genetics, West China Hospital, Sichuan University. His research interest is bioinformatics and medical systems biology.
Reference
Author notes
These authors contributed equally to this work.