-
PDF
- Split View
-
Views
-
Cite
Cite
Yajing Hao, Lili Zhang, Yiwei Niu, Tanxi Cai, Jianjun Luo, Shunmin He, Bao Zhang, Dejiu Zhang, Yan Qin, Fuquan Yang, Runsheng Chen, SmProt: a database of small proteins encoded by annotated coding and non-coding RNA loci, Briefings in Bioinformatics, Volume 19, Issue 4, July 2018, Pages 636–643, https://doi.org/10.1093/bib/bbx005
- Share Icon Share
Abstract
Small proteins is the general term for proteins with length shorter than 100 amino acids. Identification and functional studies of small proteins have advanced rapidly in recent years, and several studies have shown that small proteins play important roles in diverse functions including development, muscle contraction and DNA repair. Identification and characterization of previously unrecognized small proteins may contribute in important ways to cell biology and human health. Current databases are generally somewhat deficient in that they have either not collected small proteins systematically, or contain only predictions of small proteins in a limited number of tissues and species. Here, we present a specifically designed web-accessible database, small proteins database (SmProt, http://bioinfo.ibp.ac.cn/SmProt), which is a database documenting small proteins. The current release of SmProt incorporates 255 010 small proteins computationally or experimentally identified in 291 cell lines/tissues derived from eight popular species. The database provides a variety of data including basic information (sequence, location, gene name, organism, etc.) as well as specific information (experiment, function, disease type, etc.). To facilitate data extraction, SmProt supports multiple search options, including species, genome location, gene name and their aliases, cell lines/tissues, ORF type, gene type, PubMed ID and SmProt ID. SmProt also incorporates a service for the BLAST alignment search and provides a local UCSC Genome Browser. Additionally, SmProt defines a high-confidence set of small proteins and predicts the functions of the small proteins.
Introduction
Identification of coding elements in the genome is a fundamental step towards understanding the building blocks of living systems. Previous genome annotation pipelines mainly focused on proteins longer than 100 amino acids [1, 2]. However, recent studies have shown that many proteins shorter than 100 amino acids (small proteins) also play important roles in diverse functions including development [3], muscle contraction [4, 5] and DNA repair [6]. For example, the functional protein TAL influences development in Drosophila melanogaster [7], and recently, a small protein, MLN, encoded by a putative long non-coding RNA (lncRNA), has been found to regulate muscle performance in human [4]. Identification of the previously neglected small proteins may contribute in important ways to cellular and organismal biology, emphasizing the need for an unbiased and comprehensive strategy to evaluate translation empirically [8].
Deep transcriptome sequencing has revealed the existence of many transcripts that lack long or conserved open reading frames (ORFs), and such transcripts have generally been termed as lncRNAs [9]. Some well-known lncRNAs have been shown to play key roles in diverse biological processes including chromatin remodelling and imprinting [10], cancer metastasis [11] and cell proliferation [12]. Although a number of lncRNAs have established regulatory functions, the vast majority of lncRNAs do not have known functions. While their existence is undisputed, their coding potential and functionality have remained controversial [13–15]. To distinguish lncRNAs from coding mRNAs, previous studies mainly relied on computational approaches [16–20] by evaluating the transcript features, such as ORF length, ORF coverage and protein sequence conservation. However, these approaches may give rise to misclassifications in that lncRNAs containing short conserved regions might be misclassified as protein-coding transcripts (false negatives), whereas actual protein-coding transcripts containing short or weakly conserved ORFs might be misclassified as non-coding (false positives).
In recent years, the use of comparative genomics [21, 22], proteomics [23, 24] and a combination of evolutionary conservation and ribosome profiling data [25, 26] has indicated that translation is far more pervasive than hitherto acknowledged and has identified many coding transcripts previously assumed to be non-coding RNAs [27–29]. For example, Ingolia et al. presented that many ribosomes occupied regions of the transcriptome assumed to be non-coding [30]. Bazzini et al. leveraged the periodicity of ribosome movement on the mRNA to define actively translated ORFs by ribosome footprinting, and found several hundred smORFs (small open reading frames, sORFs) encoded by transcripts without defined coding sequences [26]. Besides, Sebastiaan et al. performed systematic RNA sequencing on ribosome-associated RNA pools obtained through ribosomal fractionation and demonstrated that lncRNAs may have a function in ribosome complexes [29]. Recently, Calviello et al. used a rigorous statistical approach to identify translated regions on the basis of the characteristic three-nucleotide periodicity of Ribo-seq data and found distinct ribosomal signatures for several hundred upstream ORFs and ORFs in annotated non-coding genes [31]. Additionally, several small proteins apparently originating from intergenic regions have been shown to be functional [3–6]. These small proteins have diverse regulatory roles; therefore, a small protein database will provide valuable information on small proteins for the scientific community as well as offer new avenues of research into the functions of what has hitherto been regarded as lncRNAs.
Currently, there are few databases for small proteins, but none of them focus on small proteins encoded by non-coding RNA loci. UniProt [32] and CCDS [33–35] pay more attention on proteins longer than 100 amino acids, although there exist some small proteins. sORF.org [36] only harbours sORFs calculated from ribosome profiling data in three cell lines. Although these existing databases offer some information about the small proteins, an in-depth investigation of small proteins across popular species is still lacking.
Here, we present a specifically designed database, small proteins database (SmProt). The current release of SmProt incorporates 255 010 small proteins computationally or experimentally identified in 291 cell types/tissues originating from eight species (human, mouse, rat, fruit fly, zebrafish, yeast, Caenorhabditis elegans and Escherichia coli). SmProt provides a user-friendly website for users to search, browse, submit, blast, export and download data on small proteins. Furthermore, the database incorporates a service for the BLAST alignment search and an integrated local UCSC Genome Browser service for visualizing the genomic locations of small proteins, which greatly improved the user experience. SmProt also defines a high-confidence set of small proteins and predicts the functions of the small proteins curated from ribosome profiling calculation and literature mining. Altogether, we strongly believe that the current SmProt database will facilitate further investigations on small proteins.
Methods and materials
The pipeline for construction of SmProt is depicted in Figure 1. The small proteins curated in SmProt were obtained from four different processing pipelines. The genes encoding the collected small proteins re-annotated with specific IDs, and the small proteins were categorized based on their genomic locations and data sources. The detailed procedure is explained in the following sections.
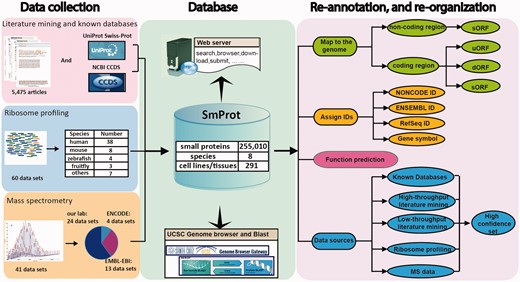
Pipeline for construction of the SmProt database (see main text for details).
Manual curation of small proteins from the scientific literature and other databases
To obtain small proteins from literature, we searched PubMed using a set of key words (listed in Supplementary Materials). We found 5475 articles (union set) published up to 23 December 2015 and obtained the abstracts of these articles from the PubMed database. Then three persons reviewed each abstract independently and obtained the paper containing small proteins’ information. Then we integrated the results. The articles were retained if all the three people agreed to keep. We double checked the qualification by reading the full article if one abstract received only two votes out of three. The remaining articles were then divided into ‘high-throughput’ or ‘low-throughput’, the former term applied to articles dealing with batch discovery of small proteins, and the latter applied to papers focusing on a smaller number of specific small proteins. The basic information on the small proteins was extracted manually, and only small proteins with strong support from experimental evidence were kept for further consideration. From the ‘high-throughput’ literature, we also extracted detailed information according to the information provided in the articles. From the ‘low-throughput’ literature, we also extracted various information such as experimental design, start codons, general description of the small proteins, functions and possible associated diseases. We have attempted to make the amount of information in SmProt as comprehensible as possible, and have included up to 28 pieces of information for each small protein entry. We also obtained small proteins from the CCDS and UniProt database to prevent missing small proteins without support from publications that may have been directly submitted to the databases. As for UniProt, we only retained the small proteins manually annotated and reviewed by UniProtKB curators, and the type of evidence that supports the existence of the protein from either transcript level or protein level. For the small proteins with lengths <100 amino acids in CCDS database, their feature information was adopted with no additional changes, as the proteins curated in CCDS are already of high quality.
Small proteins predicted in silico from ribosome profiling data sets
Ribosome profiling, that is, deep sequencing of ribosome-protected RNA fragments, has emerged as an efficient technique for comprehensive and quantitative assaying of translation activity. A variety of algorithms and metrics have been developed to use ribosome profiling data for annotation of translated regions of the genome. For the construction of the database, we took advantage of the publicly available ribosome profiling data sets obtained from GEO database [37] and European Nucleotide Archive (http://www.ebi.ac.uk/ena) using a set of key words (listed in Supplementary Materials) to identify small proteins. First, we downloaded 60 ribosome profiling data sets covering 26 cell lines or tissues from eight different species (Supplementary Table S1). At the same time, we also downloaded RNA-seq data sets in the GEO database or European Nucleotide Archive that originated from the same cell lines/tissues and species. The ribosome profiling sequencing (Ribo-seq) reads were stripped of adaptor sequences using Trimmomatic [38], and reads shorter than 28 bases were discarded before removing reads aligning to rRNA sequences using Bowtie2 [39] with the default parameters. Additionally, using the FASTX-Toolkit (http://hannonlab.cshl.edu/fastx_toolkit/), sequences were discarded if containing a base with quality score of ≤20. The RNA-seq reads were pre-processed likewise. Pre-processed Ribo- and RNA-seq reads were aligned to the relevant genome (hg19, mm10, rn6, saccer3, dr7, dm3, EB1 and ce10) with the split-aware aligner STAR [40]. A maximum of four mismatches were allowed, and multimapping to up to eight different positions was permitted. RiboTaper was used to obtain small proteins as previously described [31], retaining only small proteins that passed the multimapping filter.
Data sources . | Description . |
---|---|
Low-throughput Literature Mining | Literature is obtained from PubMed. We used a set of keywords to retrieve literature from PubMed, and then extracted detailed information manually from the literature that focused on several specific small proteins. |
High-throughput Literature Mining | Literature is obtained from PubMed. We used a set of keywords to retrieve literature from PubMed, and then extracted detailed information manually from the literature that focused on batch discovery of small proteins. |
MS data | MS data sets are collected from ENCODE project, EMBL-EBI PRIDE Archive or our lab. Then we analysed these data to obtain small proteins encoded by ncRNAs. |
Ribosome profiling | Ribosome profiling data sets are collected from GEO or ENA database, and RiboTaper software was used for small proteins identification. |
Databases | We also collected small proteins from databases. We only obtained the reliable small proteins (such as manually annotated and reviewed) and reprocessed according to the flow chart. |
Data sources . | Description . |
---|---|
Low-throughput Literature Mining | Literature is obtained from PubMed. We used a set of keywords to retrieve literature from PubMed, and then extracted detailed information manually from the literature that focused on several specific small proteins. |
High-throughput Literature Mining | Literature is obtained from PubMed. We used a set of keywords to retrieve literature from PubMed, and then extracted detailed information manually from the literature that focused on batch discovery of small proteins. |
MS data | MS data sets are collected from ENCODE project, EMBL-EBI PRIDE Archive or our lab. Then we analysed these data to obtain small proteins encoded by ncRNAs. |
Ribosome profiling | Ribosome profiling data sets are collected from GEO or ENA database, and RiboTaper software was used for small proteins identification. |
Databases | We also collected small proteins from databases. We only obtained the reliable small proteins (such as manually annotated and reviewed) and reprocessed according to the flow chart. |
Data sources . | Description . |
---|---|
Low-throughput Literature Mining | Literature is obtained from PubMed. We used a set of keywords to retrieve literature from PubMed, and then extracted detailed information manually from the literature that focused on several specific small proteins. |
High-throughput Literature Mining | Literature is obtained from PubMed. We used a set of keywords to retrieve literature from PubMed, and then extracted detailed information manually from the literature that focused on batch discovery of small proteins. |
MS data | MS data sets are collected from ENCODE project, EMBL-EBI PRIDE Archive or our lab. Then we analysed these data to obtain small proteins encoded by ncRNAs. |
Ribosome profiling | Ribosome profiling data sets are collected from GEO or ENA database, and RiboTaper software was used for small proteins identification. |
Databases | We also collected small proteins from databases. We only obtained the reliable small proteins (such as manually annotated and reviewed) and reprocessed according to the flow chart. |
Data sources . | Description . |
---|---|
Low-throughput Literature Mining | Literature is obtained from PubMed. We used a set of keywords to retrieve literature from PubMed, and then extracted detailed information manually from the literature that focused on several specific small proteins. |
High-throughput Literature Mining | Literature is obtained from PubMed. We used a set of keywords to retrieve literature from PubMed, and then extracted detailed information manually from the literature that focused on batch discovery of small proteins. |
MS data | MS data sets are collected from ENCODE project, EMBL-EBI PRIDE Archive or our lab. Then we analysed these data to obtain small proteins encoded by ncRNAs. |
Ribosome profiling | Ribosome profiling data sets are collected from GEO or ENA database, and RiboTaper software was used for small proteins identification. |
Databases | We also collected small proteins from databases. We only obtained the reliable small proteins (such as manually annotated and reviewed) and reprocessed according to the flow chart. |
Small proteins calculated from mass spectrometry data sets
Mass spectrometry (MS) data can provide direct evidence for small proteins. Theoretically, evidence of small proteins supported by the MS can be regarded as more reliable than evidence obtained by other methods. To obtain small proteins that are encoded by annotated non-coding transcripts and supported by MS data sets, we downloaded the genome locations of small proteins in four different cell types (H1-hESC, K562, GM12878 and neural in vitro differentiated cells male embryo) from the ENCODE project [41]. We also obtained raw MS data sets from our lab and from EMBL-EBI PRIDE Archive [42]. Peppy [43] with default parameters was used to match the MS/MS spectra to the reference genome and obtain the best genomic location for each spectrum. We obtained the non-coding RNA transcripts from the NONCODE database [44–48] and only retained small proteins mapping to site located entirely within the locus of an annotated non-coding RNA, using the IntersectBed from BedTools [49]. The MS data in SmProt is an additional evidence for the presence of small proteins encoded by non-coding RNAs. If the small proteins, obtained from literature mining and ribosome profiling prediction, are also identified based on MS data, then the MS data is the more confident evidence. We also provided the genomic locations of all the MS/MS spectra in the genome browser web page regardless of whether they intersected with non-coding RNA loci.
Re-annotation and re-organization
After obtaining small proteins from the four data sources mentioned above, we used gene symbols to annotate the genes encoding the small proteins. We also assigned a NONCODE ID, RefSeq ID [50] and ENSEMBL ID [51] to each of the genes. In addition, we re-organized the types of small proteins based on their location relative to the genes encoded the small proteins. The small proteins were assigned as sORF if they were encoded by annotated non-coding RNAs or not translated entirely from the 5ʹ UTR and 3ʹ UTR of protein coding genes. When translating entirely within the 5ʹ UTR (or the 3ʹ UTR) of an mRNA, the small proteins were assigned as uORF (or dORF). As different processing pipelines had different confidences, we re-organized the data processing pipelines and defined five different data sources as described in Table 1. We assigned the data source to each small protein. The small protein entries in SmProt were designated systematically. Small proteins from the same organism curated from different data sources were assigned a number starting with ‘SPRO’ followed by a symbol representing the organism. For example, ‘SPROMUS000018’ denotes a small protein from mouse (‘MUS’ standing for ‘Mus musculus’). In addition, we defined a high confidence set of small proteins, which was obtained from low-throughput literature mining, databases, high-throughput literature mining supported by MS data or ribosome profiles supported by MS data, these representing the highest quality small protein entries in the database.
Functional annotation of small proteins
To enable researchers to have a better understanding of small proteins’ functions, we predicted the functions of small proteins, obtained from databases, high-throughput literature mining and ribosome profiles, through InterProScan [52] with the default parameters. The small proteins obtained from low-throughput literature mining almost had specific functions, while the small proteins obtained from MS data are served as evidence for the presence of small proteins encoded by non-coding RNAs. All results have been added to the SmProt database.
Results
Database content
Currently, SmProt contains 255 010 small protein entries representing eight popular species (Table 2). Each small protein entry in the SmProt database has three main data components: General Information, Detailed Information and References. The General Information provides users with basic information including small protein ID, predicted functions, sequence, length, genomic location, ORF type, transcript ID, gene symbol, gene type, the organism, transcript ID, gene IDs in the NONCODE, RefSeq and ENSEMBL databases, tissue or cell line and data source. The Reference component contains the PubMed ID (PMID), the article title, authors and the journal where the article is published.
Species . | Number . |
---|---|
Human | 167 785 |
Fruit fly | 39 015 |
Caenorhabditis elegans | 18 357 |
Mouse | 15 581 |
Rat | 8128 |
Zebrafish | 2994 |
Yeast | 1875 |
Escherichia coli | 1275 |
Species . | Number . |
---|---|
Human | 167 785 |
Fruit fly | 39 015 |
Caenorhabditis elegans | 18 357 |
Mouse | 15 581 |
Rat | 8128 |
Zebrafish | 2994 |
Yeast | 1875 |
Escherichia coli | 1275 |
Species . | Number . |
---|---|
Human | 167 785 |
Fruit fly | 39 015 |
Caenorhabditis elegans | 18 357 |
Mouse | 15 581 |
Rat | 8128 |
Zebrafish | 2994 |
Yeast | 1875 |
Escherichia coli | 1275 |
Species . | Number . |
---|---|
Human | 167 785 |
Fruit fly | 39 015 |
Caenorhabditis elegans | 18 357 |
Mouse | 15 581 |
Rat | 8128 |
Zebrafish | 2994 |
Yeast | 1875 |
Escherichia coli | 1275 |
In the Detailed Information, we provide the detailed information on each small protein according to its data source.
Low-throughput literature mining: The Detailed Information tables for small protein entry obtained from low-throughput literature mining include the start codon of the small protein, the experimental method(s) used to obtain or characterize the small protein, the function of the small protein, the disease(s) with which the small protein may be involved and its description in the literature.
High-throughput literature mining: The Detailed Information tables for small protein entry curated from high-throughput literature mining vary according to the literature from which information has been obtained. The information descriptions can be viewed in the user manual in the help web page.
MS Data: The Detailed Information tables for small protein entry curated from MS data include Raw Score, Spectrum ID, Peptide Rank and Peptide Repeat Count.
Ribosome profiling data: The Detailed Information tables for small protein entry curated from ribosome profiling data contain a variety of data, including transcripts per million for both the Ribo-seq and RNA-Seq data, the (relative) positions of start and stop codons for both the small protein and for a possible annotated CDS (coding sequence) in the transcript, the sequence reads number for the small protein in both the Ribo-seq and the RNA-seq data, the P and RNA sites number in the small protein, P values for the small protein (calculated by the multitaper method) for both the Ribo-seq and the RNA-seq data, the number of exons in the small protein and the ribosome profiling data set ID.
Databases: The original ID and the detailed information about the small protein such as evidence, experiment and so on.
Web interface
All data were organized into a set of relational MySQL tables. The database query and user interface were developed using HTML, PHP (http://www.php.net/), CSS and JavaScript. Figure 2 illustrates the user interface of the database.
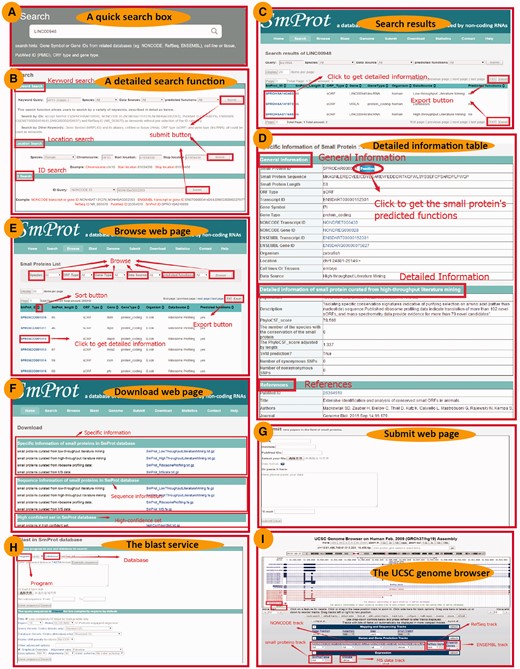
User interface of the SmProt database. (A) A quick search box on the home page. (B) A detailed search function provided in the Search web page. (C) The detailed information of search results. (D) The detailed information of small proteins curated in SmProt. (E) The Browse web page. (F) The Download web page. (G) The Submit web page. (H) The BLAST service provided in the Blast web page. (I) The local UCSC Genome Browser for visualization.
Searching and browsing
The SmProt website includes several user-friendly search boxes, which make data retrieval easy and efficient. A quick search box is available on the home page for fast searching by Gene Symbol or Gene IDs from related databases (e.g. NONCODE, RefSeq, ENSEMBL), cell line or tissue, PMID, ORF type and gene type (Figure 2A). A detailed search function is also provided (Figure 2B). The search function is divided into three parts. (1) Keyword search, allowing searching by a variety of keywords, including gene symbol, gene ID, cell line/tissue, gene type or ORF type. Keyword search results can be filtered by data sources and species. (2) Location search, by which small protein loci overlapping biological features of interest (e.g. chromosome, species, genomic region) will be obtained. (3) The option for ID search can be used if the small protein-related ID exists in any of the major databases (i.e. NONCODE, ENSEMBL, RefSeq or PubMed), or if the ID is already created in the SmProt database. The search results are obtained by clicking the ‘submit’ button (Figure 2C). If users want to view the detailed information of any particular small protein occurring in the search results, they can click the corresponding SmProt_ID, which links to the Detailed Information table (Figure 2D).
The database can be browsed by clicking the ‘Browse’ tab on the navigation menu. In the Browse web page, basic information including SmProt_ID, protein size (SmProt_length), protein type (ORF_Type), the name/ID (Gene) or type (gene type) of genomic loci encoding the small proteins, organism, the data source of the small proteins (Data Source) and PMID (Figure 2E). In the Browse web page, users can browse their interested species, ORF type, gene type and data source through Browse button to retrieve results, which would be showed as below. The result list can be viewed either by changing the number of records or by clicking on the page numbers at the bottom right corner of the table. The results list also can be sorted by specific key words by clicking the ‘∧’ or ‘∨’ in the corresponding column. Users can export detailed information using the export button and click the ‘SmProt_ID’ to obtain the detailed information concerning any specific small protein.
Download, export and submit
Specific information and sequence information of the small proteins stored in the database can be downloaded in TXT or FASTA format from the Download web page (Figure 2F). The high-confidence set in SmProt database has been also provided in the Download web page. Downloading can be performed either from the download page or while browsing and searching specific data. The queried data can be exported as a TXT or EXCEL file, using the export button on the top right of each data table. To maintain an up-to-date and comprehensive resource, SmProt also encourages users to submit newly published small proteins in the Submit web page in the requested data format (Figure 2G).
Integration with a service for the BLAST alignment search and a UCSC genome browser
In the SmProt database, we have integrated the online BLAST service (NCBI wwwBLAST version 2.2.24), which allows for sequence similarity searches of both nucleotides and proteins to be run in the blast web page (Figure 2H). Importantly, for a small protein with no recognized gene name or IDs, it is also possible to search in SmProt simply based on its sequence. Additionally, SmProt also has integrated a local UCSC Genome Browser (http://genome.ucsc.edu/) for visualization of the genomic locations of the small proteins in the SmProtTable track (Figure 2I). Small proteins curated from MS data are shown as an independent track in the genome browser. For a small protein with no recognized gene name or IDs, users can also search in SmProt based on its genomic location in genome browser. Associated tracks like NONCODE lncRNA, NONCODE Gene, RefSeq Genes and Ensembl Genes are also shown in the genome browser.
Discussion and feature development
Comparison with existing databases
By integrating data on small proteins from the literature, MS data and ribosome profiling data, SmProt provides an easy access to unbiased and comprehensive sets of small proteins derived from eight species. Compared with the existing small protein database, sORFs.org [36], which is a repository of small open reading frames (sORFs) identified by ribosome profiling, and only harbours sORFs calculated from ribosome profiling data in three cell lines, SmProt database excels in the following aspects: (i) Multiple lines of data sources. SmProt not only collected small proteins that have been computationally predicted from ribosome profiling data but also manually curated information from the scientific literature and known databases. Small protein loci were also predicted based on MS data obtained from public databases or generated experimentally in our laboratory. (ii) Substantially expanded data volumes. The current release of SmProt incorporates small proteins computationally or experimentally identified in 291 cell types and tissues derived from eight species. In comparison, sORFs.org used only ribosome profiling data from three cell lines from three species. (iii) A more stringent data analysis approach, yielding more reliable prediction results. In SmProt, we used the RiboTaper (published in Nature Methods in 2016) to identify translated regions on the basis of the characteristic three-nucleotide periodicity of the Ribo-seq data. (iv) Comprehensive annotation of all small proteins. Basic information about each small protein as well as more specific and source-dependent information is made easily available. SmProt provides up to 28 pieces of information for each small protein, which helps users to better evaluate the search results. (v) Multiple options for keyword searches. To facilitate data extraction, SmProt provides multiple search options, including species, genome location, gene name, cell type/tissue and ORF Type. Moreover, SmProt allows users to search by gene symbol, ENSEMBLID, RefSeq ID and NONCODE ID. In contrast, sORFs.org only allows users to search by ENSEMBL ID in the gene term. (vi) SmProt provides a user-friendly website, incorporating a service for BLAST alignment search and integrating a local UCSC genome browser for the visualization of the small protein genomic locations.
As compared with the databases containing small proteins, SmProt is a specialized database with a specific focus on small protein collection, and is the first database to pay attention to small proteins encoded by loci annotated as non-coding RNAs. The proteins curated in UniProt and CCDS are mainly proteins longer than 100 amino acids encoded by the known protein-coding genes. For the eight species selected in SmProt, the number of small proteins with high confidence in CCDS and UniProt is 1517 and 1481, respectively. However, 95.8% of these small proteins are already obtained in the SmProt. And the left 4.3% proteins without curation in SmProt are mainly submitted directly to the databases. In order not to ignore these small proteins, we also obtained the small proteins from these two databases and processed to add the unique features in SmProt.
Feature development
The overall goal of the SmProt database is to provide a comprehensive resource to facilitate further studies of small proteins and their functions. The SmProt will also provide a new tool for exploring the functional mechanism of annotated lncRNAs. The small protein research field is probably only beginning to unfold. In the future, we will continue to update the database and integrate more species. Furthermore, we will also continue to expand the storage space and improve the computer server performance for storing and analysing these data. We expect that by these continuous efforts on developing and improving SmProt, we will contribute to the general understanding of small proteins and their roles in cellular function.
Key Points
To provide informative data source as well as valuable information on small proteins for the whole scientific community, we developed a small protein database with an integration of 255 010 small proteins curated form five different data sources in eight popular species.
Comprehensive annotation of all small proteins. Basic information about each small protein as well as more specific and source-dependent information is well described. Importantly, we also provided the small proteins’ functions.
SmProt provided a user-friendly website, incorporating a service for BLAST alignment search and integrating a local UCSC genome browser for the visualization of the small protein genomic locations. Additionally, SmProt defines a high-confidence set of small proteins, which can be downloaded in the Download web page.
SmProt also curated small proteins encoded by non-coding regions, which offers new avenues of research into the functions of what has hitherto been regarded as lncRNAs.
Supplementary Data
Supplementary data are available online at http://bib.oxfordjournals.org/.
Acknowledgement
We are very grateful to Dr Geir Skogerbø for helpful suggestions and critical reading of this manuscript. We also thank Dr Xiaowei Chen and Dr Zhen Fan for discussion on high-throughput sequencing.
Funding
National Natural Science Foundation of China (grant number 31520103905) and the National High Technology Research and Development Program (‘863’ Program) of China (grant number 2015AA020108, 2014AA021502).
Yajing Hao is a PhD candidate at Key Laboratory of RNA Biology, Institute of Biophysics and University of the Chinese Academy of Sciences, Beijing, China. She has been working in the field of small proteins’ associated study, especially the small proteins encoded by lncRNAs.
Lili Zhang is a master candidate at Key Laboratory of RNA Biology, Institute of Biophysics and University of the Chinese Academy of Sciences, Beijing, China.
Yiwei Niu is a master candidate at Key Laboratory of RNA Biology, Institute of Biophysics and University of the Chinese Academy of Sciences, Beijing, China.
Tanxi Cai is an associate professor at Key Laboratory of Protein and Peptide Pharmaceuticals and Laboratory of Proteomics, Institute of Biophysics, Chinese Academy of Sciences, Beijing, China. His research interests are focused on proteomics and lipidomics.
Jianjun Luo is an associate professor at Key Laboratory of RNA Biology, Institute of Biophysics, Chinese Academy of Sciences, Beijing, China. His research interests are focused on systematic identification and functional studies of non-coding RNAs and small proteins.
Shunmin He is an associate professor at Key Laboratory of the Zoological Systematics and Evolution, Institute of Zoology, Chinese Academy of Sciences, Beijing, China. His research interests are focused on transcriptomics and bioinformatics.
Bao Zhang is a master candidate at Key Laboratory of RNA Biology, Institute of Biophysics and University of the Chinese Academy of Sciences, Beijing, China.
Dejiu Zhang is an assistant professor at Key Laboratory of RNA Biology, Institute of Biophysics, Chinese Academy of Sciences, Beijing, China. His research interest is focused on ribosome-related studies.
Yan Qin is a professor at Key Laboratory of RNA Biology, Institute of Biophysics, Chinese Academy of Sciences, Beijing, China. Her research interests are focused on the process of translation/protein biosynthesis.
Fuquan Yang is a professor at Key Laboratory of Protein and Peptide Pharmaceuticals and Laboratory of Proteomics, Institute of Biophysics, Chinese Academy of Sciences, Beijing, China. His research interests are focused on proteomics and lipidomics.
Runsheng Chen is a professor at Key Laboratory of RNA Biology, Institute of Biophysics, Chinese Academy of Sciences, Beijing, China. His research interests are focused on transcriptomics and bioinformatics.
References
Author notes
These authors Yajing Hao and Lili Zhang contributed equally to this work.