-
PDF
- Split View
-
Views
-
Cite
Cite
Abdul Arif Khan, Zakir Khan, Mohd Abul Kalam, Azmat Ali Khan, Inter-kingdom prediction certainty evaluation of protein subcellular localization tools: microbial pathogenesis approach for deciphering host microbe interaction, Briefings in Bioinformatics, Volume 19, Issue 1, January 2018, Pages 12–22, https://doi.org/10.1093/bib/bbw093
- Share Icon Share
Abstract
Microbial pathogenesis involves several aspects of host–pathogen interactions, including microbial proteins targeting host subcellular compartments and subsequent effects on host physiology. Such studies are supported by experimental data, but recent detection of bacterial proteins localization through computational eukaryotic subcellular protein targeting prediction tools has also come into practice. We evaluated inter-kingdom prediction certainty of these tools. The bacterial proteins experimentally known to target host subcellular compartments were predicted with eukaryotic subcellular targeting prediction tools, and prediction certainty was assessed. The results indicate that these tools alone are not sufficient for inter-kingdom protein targeting prediction. The correct prediction of pathogen’s protein subcellular targeting depends on several factors, including presence of localization signal, transmembrane domain and molecular weight, etc., in addition to approach for subcellular targeting prediction. The detection of protein targeting in endomembrane system is comparatively difficult, as the proteins in this location are channelized to different compartments. In addition, the high specificity of training data set also creates low inter-kingdom prediction accuracy. Current data can help to suggest strategy for correct prediction of bacterial protein’s subcellular localization in host cell.
Introduction
Microbial pathogenesis involves a highly coordinated response of the pathogens with the host for their survival, growth and reproduction. This coordination is multifaceted and involves microbial attachment to the host and the subsequent signaling with host cell machinery. These events are managed through multiple processes including pathogen proteins targeting the host cell. These targeted proteins get localized in several host subcellular compartments [1]. The most important among these are nucleus and mitochondria, which carry genetic material and control host cell survival and death. The bacterial proteins migrating to host nucleus are also known as nucleomodulins [2]. The nucleus is core of entire eukaryotic cellular machinery and controls genetic expression, which governs whole cell physiology. The mitochondrion is also a critically important organelle of eukaryotic cell that controls the energy requirement of cell. It is also involved in regulating intrinsic pathway of apoptosis, thereby controlling cellular senescence and death. These two organelles are common in terms of having their own genetic material susceptible to several bacterial genetic modulator proteins. In addition, several microbial proteins are known to target host cell endomembrane system and cytoplasm. The endomembrane system includes various membrane-bound compartments of eukaryotic cell, which include nuclear membrane, rough and smooth endoplasmic reticulum, golgi, cytoplasmic vesicles, which is connected to each other either directly or by vesicle transport. During microbial pathogenesis, these membrane-bound compartments communicate with each other and involves pathogen protein subcellular targeting among endomembrane system components [3–5]. Targeting of bacterial proteins in host cell cytoplasm is a common event affecting host cell machinery. For example, anthrax lethal toxin produced by bacteria Bacillus anthracis migrate to host cell cytoplasm and influence several host proteins including mitogen-activated protein kinase and kill macrophages and macrophage-like cell lines [6].
Several studies tried to detect pathogen protein targeting host cell to decipher their role in microbial pathogenesis, regulation of host cell physiology including cell death and proliferation [7–9]. As the experimental analysis of whole microbial proteome is always a labor-intensive and extravagant task and every laboratory cannot afford it, therefore computational prediction of microbial proteins targeting host cell is now a routine practice [10–14]. Several computational tools are available for predicting subcellular targeting of certain proteins. However, these tools are based on certain data set derived from same type of organism for which they are designed to predict subcellular targeting, but the capability of these tools for inter-organism prediction needs to be investigated. These tools work on variety of principles including detection of localization signal, evolutionary information, amino acid composition, dipeptide composition, sequence similarity, transmembrane segment, etc. (Figure 1). Each method has its own limitations and advantages, but they claim to have certain prediction ability depending on the type of tools (Table 1). Although prediction reliability of these tools is assessed for certain types of organisms, which is included in their training data set, evaluation of their prediction reliability for microbial proteins is required.
Different prediction tools used during the study and their prediction approach, training data set and reliability as mentioned in literature
Sr. No. . | Prediction tool . | Database size and validation process . | Reliability/prediction performance (as per literature) . | ||||
---|---|---|---|---|---|---|---|
. | Sensitivity . | Specificity . | Accuracy . | ||||
1 | cNLS mapper [15, 16] |
| Class ½ | 99 | 94 | 98 | |
Class 3 | 100 | 100 | 100 | ||||
Class 4 | 87 | 97 | 92 | ||||
Bipartite | 87 | 82 | 85 | ||||
Values are based on test peptide sequence from synthetic NLS mutant | |||||||
2 | PSORT II [17, 18] |
| 57% for yeast sequences and 86% f or Escherichia coli sequences | ||||
3 | WOLF PSORT [19] |
|
| ||||
4 | TargetP [20] | Uses N terminal sequence information only |
| ||||
Plant | Chloroplast transit peptide (cTP): 141; mitochondrial targeting peptide (mTP): 368; secretory: 269; nuclear: 102; cytosolic: 195 | ||||||
Non-plant | Cytosolic: 438; mTP: 371; secretory: 715; nuclear: 1214 | ||||||
5 | Mitoprot [21] |
|
| ||||
6 | BaCeILo [22] |
|
| ||||
7 | HSLPred [23] |
|
| ||||
8 | ESLPred [24] |
|
| ||||
9 | SubLoc v 1.0 [25] |
|
| ||||
10 | EffectiveDB [26] |
|
| ||||
11 | TMPred [28] | It predicts membrane-spanning regions of certain protein with their orientation | Average prediction reliability for photosynthetic reaction centre, bacteriorhodopsin, and cytochrome c oxidase: 84.5% [29] |
Sr. No. . | Prediction tool . | Database size and validation process . | Reliability/prediction performance (as per literature) . | ||||
---|---|---|---|---|---|---|---|
. | Sensitivity . | Specificity . | Accuracy . | ||||
1 | cNLS mapper [15, 16] |
| Class ½ | 99 | 94 | 98 | |
Class 3 | 100 | 100 | 100 | ||||
Class 4 | 87 | 97 | 92 | ||||
Bipartite | 87 | 82 | 85 | ||||
Values are based on test peptide sequence from synthetic NLS mutant | |||||||
2 | PSORT II [17, 18] |
| 57% for yeast sequences and 86% f or Escherichia coli sequences | ||||
3 | WOLF PSORT [19] |
|
| ||||
4 | TargetP [20] | Uses N terminal sequence information only |
| ||||
Plant | Chloroplast transit peptide (cTP): 141; mitochondrial targeting peptide (mTP): 368; secretory: 269; nuclear: 102; cytosolic: 195 | ||||||
Non-plant | Cytosolic: 438; mTP: 371; secretory: 715; nuclear: 1214 | ||||||
5 | Mitoprot [21] |
|
| ||||
6 | BaCeILo [22] |
|
| ||||
7 | HSLPred [23] |
|
| ||||
8 | ESLPred [24] |
|
| ||||
9 | SubLoc v 1.0 [25] |
|
| ||||
10 | EffectiveDB [26] |
|
| ||||
11 | TMPred [28] | It predicts membrane-spanning regions of certain protein with their orientation | Average prediction reliability for photosynthetic reaction centre, bacteriorhodopsin, and cytochrome c oxidase: 84.5% [29] |
EC = endothelial cell; PM = plasma membrane.
Different prediction tools used during the study and their prediction approach, training data set and reliability as mentioned in literature
Sr. No. . | Prediction tool . | Database size and validation process . | Reliability/prediction performance (as per literature) . | ||||
---|---|---|---|---|---|---|---|
. | Sensitivity . | Specificity . | Accuracy . | ||||
1 | cNLS mapper [15, 16] |
| Class ½ | 99 | 94 | 98 | |
Class 3 | 100 | 100 | 100 | ||||
Class 4 | 87 | 97 | 92 | ||||
Bipartite | 87 | 82 | 85 | ||||
Values are based on test peptide sequence from synthetic NLS mutant | |||||||
2 | PSORT II [17, 18] |
| 57% for yeast sequences and 86% f or Escherichia coli sequences | ||||
3 | WOLF PSORT [19] |
|
| ||||
4 | TargetP [20] | Uses N terminal sequence information only |
| ||||
Plant | Chloroplast transit peptide (cTP): 141; mitochondrial targeting peptide (mTP): 368; secretory: 269; nuclear: 102; cytosolic: 195 | ||||||
Non-plant | Cytosolic: 438; mTP: 371; secretory: 715; nuclear: 1214 | ||||||
5 | Mitoprot [21] |
|
| ||||
6 | BaCeILo [22] |
|
| ||||
7 | HSLPred [23] |
|
| ||||
8 | ESLPred [24] |
|
| ||||
9 | SubLoc v 1.0 [25] |
|
| ||||
10 | EffectiveDB [26] |
|
| ||||
11 | TMPred [28] | It predicts membrane-spanning regions of certain protein with their orientation | Average prediction reliability for photosynthetic reaction centre, bacteriorhodopsin, and cytochrome c oxidase: 84.5% [29] |
Sr. No. . | Prediction tool . | Database size and validation process . | Reliability/prediction performance (as per literature) . | ||||
---|---|---|---|---|---|---|---|
. | Sensitivity . | Specificity . | Accuracy . | ||||
1 | cNLS mapper [15, 16] |
| Class ½ | 99 | 94 | 98 | |
Class 3 | 100 | 100 | 100 | ||||
Class 4 | 87 | 97 | 92 | ||||
Bipartite | 87 | 82 | 85 | ||||
Values are based on test peptide sequence from synthetic NLS mutant | |||||||
2 | PSORT II [17, 18] |
| 57% for yeast sequences and 86% f or Escherichia coli sequences | ||||
3 | WOLF PSORT [19] |
|
| ||||
4 | TargetP [20] | Uses N terminal sequence information only |
| ||||
Plant | Chloroplast transit peptide (cTP): 141; mitochondrial targeting peptide (mTP): 368; secretory: 269; nuclear: 102; cytosolic: 195 | ||||||
Non-plant | Cytosolic: 438; mTP: 371; secretory: 715; nuclear: 1214 | ||||||
5 | Mitoprot [21] |
|
| ||||
6 | BaCeILo [22] |
|
| ||||
7 | HSLPred [23] |
|
| ||||
8 | ESLPred [24] |
|
| ||||
9 | SubLoc v 1.0 [25] |
|
| ||||
10 | EffectiveDB [26] |
|
| ||||
11 | TMPred [28] | It predicts membrane-spanning regions of certain protein with their orientation | Average prediction reliability for photosynthetic reaction centre, bacteriorhodopsin, and cytochrome c oxidase: 84.5% [29] |
EC = endothelial cell; PM = plasma membrane.
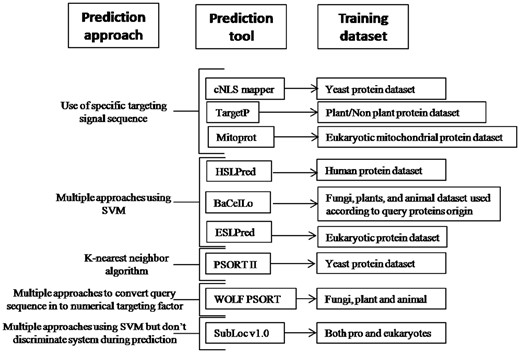
Graphical outline for different prediction methods used by different tools and their training data sets.
An estimation of the reliability and accuracy of these inter-organism predictions is always a challenging task. Therefore, we designed this study for evaluating the ability of eukaryotic subcellular localization prediction tools to predict prokaryotic proteins as a query. This calibration is highly important in maintaining prediction accuracy of these tools for their use in microbial pathogenesis-related studies.
Materials and methods
Protein sequences
The 119 bacterial proteins experimentally known to target host subcellular compartments were selected for the study. These proteins included 44 (nuclear), 29 (mitochondrial), 32 (endomembrane system), 14 (cytosolic) proteins either known to target or interact with respective subcellular targeting location in host cell. Possible care was taken to avoid similar sequence with multiple accession numbers, but derived from similar bacterial strain. Although in some cases, proteins from two different organisms were included in the study, their origin from different bacteria made them suitable candidates for inclusion in the study. The protein sequences were retrieved from Uniprot, whereas the protein sequences, which were not found in Uniprot, were retrieved from NCBI protein database (details available in Supplementary tables). Both plant and animal pathogens (including human pathogens) were selected for prediction.
Selection of tools
The pathogen’s protein targeting in host cell is governed by multiple host pathogen factors. Under certain situations, pathogen proteins can passively localize to host subcellular compartments, and this property of proteins is governed by their molecular weight [30, 31]; therefore, we detected molecular weight of protein to understand their passive subcellular targeting. The pathogen proteins targeting host subcellular compartment are also regulated by presence of certain localization signals, so the tools predicting these localization signals were included in the study. The prediction tools based on single prediction approach cannot consider influence of other factors on subcellular targeting, therefore prediction tools detecting bacterial protein secretion mechanism, and host subcellular targeting by multiple approaches including transmembrane helices detection, evolutionary information, sequence similarity were also used. As the aim of this study was to detect prediction certainty of prokaryotic protein targeting in eukaryotic host cells, tools working on diverse principles and training data set were selected (Figure 1). A total of 11 tools working on different prediction approaches and training data set were used to predict pathogen protein targeting in host cell (Table 1; Figure 1). Among these, classical nuclear localization signal (cNLS) mapper detects nuclear targeting and therefore was used only for nuclear proteins, and MitoProt, which detects mitochondrial targeting, was used for host mitochondrial-targeted proteins only. Remaining seven prediction tools were known to predict both nuclear and mitochondrial subcellular targeting and therefore used for all proteins irrespective of their types. TargetP detects only mitochondrial, chloroplast and secretary pathway localization signal, but include data set of nuclear proteins also, and so, it was also used for all types of proteins to understand their effect on protein localization prediction (Table 1). TMPred was used for detection of transmembrane helices in query proteins.
Host subcellular targeting prediction
The bacterial proteins known to target host subcellular compartments were subjected as query for prediction by above tools. The default parameters were used for prediction, as these are most frequently used. For cNLS mapper prediction, the prediction was performed in the entire protein with NLS cutoff value 2.0. The plant and animal/human pathogen proteins were searched in their respective database wherever desired. With some tools like ESLPred and HSLPred, the protein subcellular localization is detected through various properties of query sequence under individual prediction approach, but the hybrid method involves inclusion of all approaches of prediction. We used hybrid method approach for prediction of subcellular targeting, as it is found to have highest prediction accuracy in comparison with other individual approaches (Table 1). TargetP has another variation SignalP, which predicts subcellular targeting of bacterial proteins. Nevertheless, we used only TargetP, as we wanted to predict targeting of bacterial proteins in eukaryotic system [20].
Detection of bacterial secretion of proteins
Some tools are able to detect release of protein by potential secretion system of bacteria. In addition, this can also indicate about targeting of certain proteins in host subcellular compartments. The protein secretion with its subcellular targeting makes more sense for actual protein targeting in practical scenario. Therefore, we predicted secretion system in bacteria through EffectiveDB.
Results
Nuclear targeting prediction
The results indicate that the detection of NLS is insufficient to guarantee about nuclear localization of proteins. After considering NLS cutoff value 5 as strong nuclear targeting signal, we found only 27% nuclear protein with monopartite and bipartite NLS. These NLS-containing proteins included 83.3% sequences with >40 kDa molecular weight. In contrast, 56.25% proteins without NLS was found to have <40 kDa molecular weight. The present distribution of transmembrane helices in proteins was found to be almost equal in both NLS-containing and not containing proteins. Figure 2 indicates about prediction performance of nuclear proteins with different protein subcellular localization prediction tools using bacterial proteins as query. BaCeILo and ESLPred were found to give better inter-kingdom prediction reliability with >40 kDa nuclear proteins. In case of protein with <40 kDa molecular weight and presence of transmembrane helices, BaCeILo was not able to predict nuclear targeting proteins accurately. Prediction reliability of PSORT II and WOLF PSORT was not good until second prediction choice was also considered as significant. Both these proteins subcellular targeting prediction tools give a number of hits or percent chance for subcellular targeting of query protein with WOLF PSORT and PSORT II, respectively. Inclusion of second predicted location choice markedly increased the prediction reliability (Figure 2). However, these tools were also not able to give 100% prediction certainty, and some false-negative predictions occurred depending on different factors associated with query protein, but ESLpred maintained almost uniform prediction reliability among the tools analyzed. Supplementary Table S1 gives details about overall prediction of bacterial proteins experimentally known to target host nucleus. During the analysis of NLS distribution among different molecular weight proteins, it was found that majority of nuclear-targeted proteins lies between 0 and 150 kDa. Among these, the highest molecular weight protein with accession number Q2GGH1 was found to have good mono- and bipartite NLS cutoff value. However, monopartite NLS was comparatively less than bipartite NLS with few exceptions, but in case of proteins with increasing molecular weight, the monopartite NLS cutoff value was higher than bipartite NLS cutoff (Figure 3).
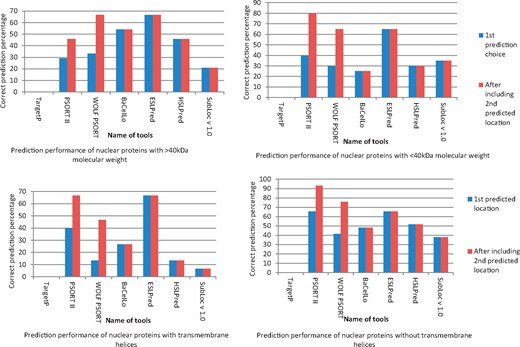
Prediction of host nuclear targeting of experimentally known bacterial proteins localizing host nucleus and their relation with associated factors.
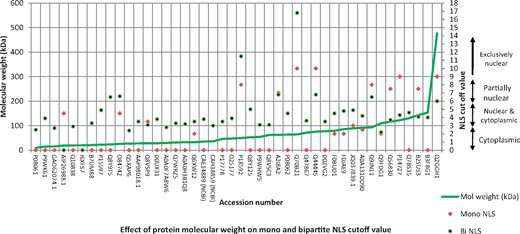
Prediction of NLS in bacterial proteins experimentally known to target host nucleus and their relation with molecular weight of proteins.
Mitochondrial targeting prediction
During analysis of bacterial proteins known to target host mitochondria, the MitoProt P value greatly influenced prediction ability of subcellular localization prediction tools. The bacterial proteins known to target host mitochondria with MitoProt P value >0.5 were found to have increased prediction reliability with sorting signals detecting tools like TargetP, PSORT II, WOLF PSORT and EffectiveDB. The prediction reliability was also increased with tools working on multiple approaches like BaCeIlo. The presence of transmembrane segments also reduced prediction reliability with TargetP, PSORT II, WOLF PSORT and EffectiveDB and the tool working on multiple approaches like BaCeILo. In contrast, HSLPred, ESLPred and SubLoc were not able to predict proteins without transmembrane helices (Figure 4). WOLF PSORT and PSORT II increased prediction accuracy after adding second choice as significant. Under certain situation, the first prediction choice by these tools almost miss all mitochondrial proteins. This makes an impression that second prediction choice should not be neglected in such predictions, as this can also give valuable information. During analysis of MitoProt P value and its relation with molecular weight of bacterial protein known to target host mitochondria, no consistent relation was found except with the proteins with molecular weight >250 kDa giving low MitoProt P value (Figure 5). Supplementary Table S2 provides details about overall prediction of bacterial proteins experimentally known to target host cell mitochondria.
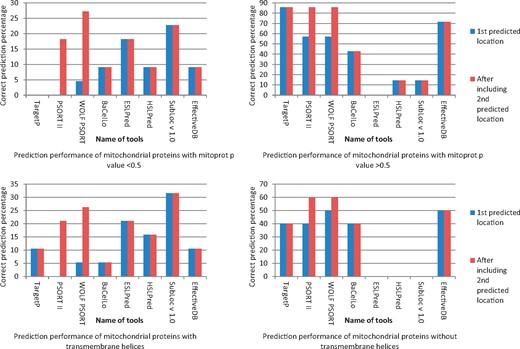
Prediction of host mitochondrial targeting of experimentally known bacterial proteins localizing host mitochondria and their relation with associated factors.
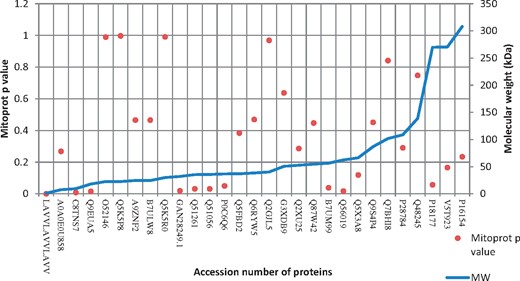
Prediction of MitoProt P value in bacterial proteins experimentally known to target host mitochondria and their relation with molecular weight of proteins.
Endomembrane system and cytoplasmic targeting prediction
The detection of bacterial protein targeting in endomembrane system components was found to be highly influenced by presence of transmembrane helices in query proteins. However, the prediction performance or these targeting locations was poor, but detection of such locations in proteins without transmembrane helices was 0%. The correct prediction with cytoplasmic proteins was highest with SubLoc v 1.0 followed by BaCeILo and HSLPred (Figure 6). Supplementary Tables S3 and S4 give details about protein targeting prediction by different subcellular localization prediction tools for host endomembrane system and cytosol targeting proteins, respectively.
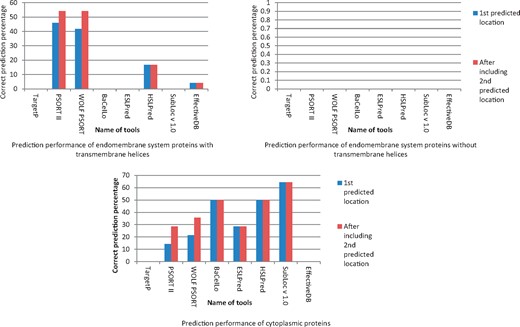
Prediction of host endomembrane system targeting of experimentally known bacterial proteins localizing host endomembrane system and their relation with associated factors.
During detection of secretion system in query proteins, it was found that among our listed secretion system (based on literature) and EffectiveDB predicted secretion system, 90.9% (nuclear), 83.33% (mitochondrial), 91.66% (endomembrane system) and 66.6% (cytosol) proteins showed correct prediction. The overall prediction assessment ability of these subcellular localization prediction tools is presented (Figure 7) as heat plot.

Overall prediction performance of in silico tools for analysis of bacterial protein localization in host subcellular compartments.
Discussion
Pathogen protein targeting host cell is an important part of microbial pathogenesis. Among the host subcellular compartments, the nucleus and mitochondria are important components that form the core of cell survival [14, 32, 33]. The pathogen tries to hijack the host cellular machinery such that the host cell survival and death are coordinated as per pathogen requirement. In addition, pathogen protein targeting host cell cytoplasm and other membrane-bound subcellular organelles has several implications in microbial pathogenesis [1]. Several subcellular protein targeting prediction tools are available, and their number and prediction performance are gradually improving. Our selection of tools for this study depends on diverse approaches for prediction as well as variable training data set (Figure 1; Table 1).
The protein targeting can be passive through diffusion, where protein passively travels through available space and stops wherever it finds its proper restricting target [30]. In contrast, under most of the cases, the protein targeting depends on presence of certain localization signals present in protein itself [34]. We tried to use both prediction strategies during our study. We observed molecular weight of nuclear proteins, as it is known that <40 kDa molecular weight proteins can passively localize to host cell nucleus [31]. The prediction tools based on detection of certain localization signal were further included in the study to cover protein targeting prediction by this mechanisms (Figure 1). However, these localization signals are not always present with certain category proteins. For example, only a part of nuclear protein carries NLS and therefore additional factors are involved in subcellular localization of certain protein [35]. Therefore, we additionally included other tools working on support vector machine (SVM) and consider multiple factors for protein subcellular targeting prediction. SVMs are computational supervised models to classify data on the basis of learning algorithm associated with SVM [36]. These SVMs work on different prediction approach as well as training data set to provide maximum accuracy to available protein subcellular localization tools (Table 1). The subcellular localization of query protein is also influenced by the presence of transmembrane domain and therefore it was also included in the study.
However, the protein localization depends on multiple factors mentioned above, but the targeting of pathogen protein in host cell required additional measures. The pathogen uses special secretion system to export their proteins in host cell [37]. The subcellular targeting prediction of pathogen proteins in host cell is incomplete without prediction of their secretion system to export particular type of proteins by pathogen. Therefore, we included EffectiveDB, which is a combination of tools detecting secretion system as well as subcellular targeting of query protein.
Our study predicted presence of NLS in only 27% proteins. This observation is consistent with the finding that only a part of nuclear proteins has NLS, and these proteins can use alternate strategies for targeting host nucleus [38]. Although low molecular weight proteins can be translocated to host nucleus as per the fact that <40 kDa molecular weight proteins can passively enter into nucleus, high molecular weight proteins required higher NLS cutoff value (Figure 3) and justify the results. The detection of NLS has multiple advantages and disadvantages. However, being simple in nature with either one (mono) or two (bipartite) stretches of basic amino acids, but in the proteins with multiple predicted NLS, detection of actual functional NLS is not possible and therefore it can be a contributing factor behind inaccurate predictions. Moreover, the NLS activity is calculated as an isolated peptide instead of considering the structure of native protein. The cNLS mapper is based on yeast data set to predict nuclear localization [39]. Sometimes, the nuclear proteins are known to target multiple locations [40] and create complex situations for prediction tools. This problem is far grave with detection of distant protein as a query. During our study, we used bacterial proteins as a query to detect their targeting in eukaryotic host cell. Therefore, the disparity in targeting prediction is certain, and added parameters should be measured for getting more precise prediction as mentioned in Figure 2.
According to results, ESLPred was able to give comparatively consistent inter-kingdom prediction accuracy for nuclear proteins. This may be because of a number of reasons including the multiple approaches used by ESLPred. The HSLPred and ESLPred are almost similar in prediction approaches, but the difference lies in their training data set. The HSLPred works on specific human protein data set, while ESLPred works on the basis of broad group of eukaryotic protein data set (Table 1). As mentioned in Figure 2, the inter-kingdom subcellular targeting prediction performance of ESLPred was always higher in comparison with HSLPred for nuclear proteins. This indicates that although highly specific training data set can provide high prediction accuracy for that particular organism query proteins [41, 42], the detection of prokaryotic pathogen protein targeting in eukaryotic host cell indicates that highly specific training data set creates low inter-kingdom prediction accuracy. Therefore, organism-specific protein subcellular targeting prediction tools cannot solve the problem of in silico detection of one organism’s protein targeting in another distant organism.
During detection of pathogen protein targeting in host cell mitochondria, the tools detecting mitochondrial targeting signals (e.g. TargetP, PSORT II, WOLF PSORT and EffectiveDB) gave good inter-kingdom prediction performance. It has been already suggested that bacteria use mitochondrial targeting signals to target their proteins in host mitochondria [1]. This evidence fairly supports the result indicating high MitoProt P value proteins are showing good inter-kingdom mitochondrial targeting prediction accuracy (Figure 4) and poor prediction of mitochondrial protein with low MitoProt P value.
The prediction performance of proteins with transmembrane helices was comparatively poor (Figure 4). Perhaps, the transmembrane helices detection by prediction tools creates additional complexity in the query proteins and reduces their mitochondrial targeting prediction ability. For example, it is found with Legionella pneumophila protein LncP (which is experimentally known to target host mitochondria), that it has four strong transmembrane segments (Supplementary Table S2). It has found that this protein targets mitochondria and makes a specific channel for transfer of metabolites. It is involved in evacuation of adenosine triphosphate molecules from mitochondrial matrix during infection [43]. It is obvious now that detection of pathogen’s protein (with strong mitochondrial sorting signal and without transmembrane domain) targeting in host subcellular compartments is comparatively easier than vice versa. The influence of mitochondrial targeting signal in prediction ability assessment is further supported by the fact that BaCeILo performance was higher among similar category tools detecting targeting of bacterial proteins with >0.5 MitoProt P value. The BaCeILo considers N and C termini sequences in addition to evolutionary information for SVM, while ESLPred and HSLPred use different approaches of prediction (Table 1). MitoProt P value was less with >250 kDa molecular weight mitochondrial proteins (Figure 5). This indicates that alternative mechanisms for mitochondrial targeting are possible and should be covered for prediction tools detecting pathogen’s protein targeting in host cell mitochondria. After analysis of these proteins by TMPred, it was found that these all contain transmembrane domain and can use mechanism like LcnP of L. pneumophila.
It can be concluded for detection of pathogen’s protein targeting in host cell mitochondria that detection of transmembrane helices and mitochondrial targeting signals should be used as additional parameters to customize the predictions. In addition, the host pathogen protein targeting prediction tools should incorporate these parameters to improve prediction accuracy for microbial pathogenesis-related studies.
The prediction performance of endomembrane system proteins was poorest among all subcellular targeting location analyzed, especially in the proteins without transmembrane helices. None of the prediction tool was able to predict correct subcellular targeting of endomembrane system proteins without transmembrane helices (Figure 6). However, the prediction performance of protein with transmembrane helices was comparatively higher, but not good. There may be several reasons behind this poor inter-kingdom prediction reliability. The endomembrane system involves protein trafficking through vesicles in multiple compartments [44], and it is already known that pathogen’s proteins are trafficked through endomembrane system during infection [45, 46]. Owing to this reason, we selected endomembrane system as a whole with the intention to get better prediction reliability. The proteins targeting any endomembrane system component was included as correct prediction, but still the prediction performance was poor. The majority of the tools used in the study were not detecting endomembrane system targeting. Only PSORT II and WOLF PSORT were detecting this targeting location on the basis of sorting signals, and HSLPred was detecting host cell plasma membrane targeting only. This may be the reason for low inter-kingdom prediction certainty for this location. It is required to have a tool with including endomembrane compartment sorting signals, transmembrane domain and other parameters for efficient prediction of pathogen’s protein targeting in host cell. The poor prediction performance of such protein deserves an independent study on properties of these proteins and their inclusion in prediction tools algorithm to increase prediction certainty.
During detection of pathogen’s protein targeting in host cell cytoplasm, Subloc v 1.0 was found to have comparatively higher inter-kingdom prediction certainty. SubLoc is also based on SVM to predict subcellular targeting of query proteins. However, it has two variations to predict prokaryotic and eukaryotic proteins separately, but as it does not ask for a particular system to predict, the chances of giving good accuracy with prokaryotic proteins in eukaryotic system are higher and logical [25]. It analyzes query protein without asking its source (animal, plant, bacteria, etc.). It can be the reason behind comparatively good prediction performances of SubLoc for cytoplasmic proteins.
The prediction of secretion system by EffectiveDB was comparatively good. This tool is primarily designed for detecting secretion of query protein by bacteria, but also detects host subcellular targeting [26]. This utility makes it an ideal candidate for microbial pathogenesis-related studies. Bacteria uses several secretion systems to transport their effectors in host cell, and the information about subcellular targeting can be better assumed with their secretion prediction specifically for extracellular pathogens. However, the prediction of secretion system adds valuable input in microbial pathogenesis, but the detection of subcellular targeting through only N-terminals targeting sequence may be the reason behind limited subcellular targeting prediction certainty of EffectiveDB [27]. This fact is also reflected in another study analyzing prediction accuracy of k-nearest neighbors classifier (PSORT II method), that it gives 60% prediction accuracy for 10 yeast classes and therefore may be the reason behind certain false predictions of PSORT II [47].
The variations in host subcellular targeting prediction of these tools indicate that these in silico prediction tools can miss many nuclear and mitochondrial proteins while predicting their subcellular targeting location elsewhere. However, this does not summarily nullify previous studies predicting bacterial proteins targeting in host cell, but raises skepticism that such prediction should be validated further for evaluating actual protein localization and their subsequent impact on host cell through protein–protein interactions (PPIs). Certainly, there are several factors behind low inter-kingdom prediction accuracy of these tools. For example, sometimes the proteins are not exclusively localized to one location (especially for multi membrane pass proteins) and makes prediction uncertain. Therefore, additional measures are required to increase prediction certainty for such proteins. In addition, the database used for prediction is different from the query sequence, and this can be another reason behind variable inter-kingdom prediction accuracy.
This study also revealed that several measures can be taken to improve prediction accuracy by in silico tools. Every type of subcellular targeting location can be analyzed by different approach-based tools. The use of ESLPred was consistent for nuclear proteins with or without transmembrane (TM), NLS and with variation in molecular weight. However, the detection of pathogen’s proteins targeting host cell mitochondria should be coupled with additional parameters of MitoProt P value and presence of TM, but tools working on detection of sorting signals were good in comparison with tools based on SVM using other approaches. The detection of pathogen’s protein targeting in endomembrane system is still a challenging task, and tools working on sorting signal detection give a slightly better performance, but still it needs methods to incorporate additional parameters to predict accurate pathogen’s protein targeting in host cell (Figure 7). However, it has been experimentally verified that proteins with multiple subcellular targeting location, the targeting should be customized for in silico approach [48]. Current data generated from this study can add valuable inputs in customizing prokaryotic protein’s subcellular targeting prediction in eukaryotic host cell. The data generated during the study provide details about the factors those can be added to provide positive and negative impact on reliability values of these tools. It will also be helpful for development of prediction tools for such complex situations. In addition, researchers trying to predict bacterial proteins through existing tools can also involve these recommendations in their studies for getting better prediction outcomes. Another major addition can be done by predicting host pathogen PPI of query proteins by homology modeling, hidden Markov model or gold standard PPI data. Among these PPI methods, gold standard PPI is the most reliable method, which depends on experimentally validated PPI. Several databases of gold standard PPI are available and should be incorporated to increase the validity of predictions. The high specificity of training data set and less number of prediction approaches in a tool also create low inter-kingdom prediction certainty, and therefore, tools based on these criteria should be avoided for certain cases.
The standard in silico approach involves evaluation of host pathogen PPI by multiple methods and detection of host subcellular targeting by significant interacting proteins. Therefore, the evaluation of microbial proteins influence on host physiology by only in silico protein subcellular targeting data should be discouraged. In conclusion, this article is not intended to raise criticism on actual function of tools analyzed, as these were not tested by us and perhaps already tested by developer before making tools available for public. Nevertheless, the results indicate the potential of these tools to predict certainty of bacterial protein as query should be carefully done and further validated by PPI data. In addition, the results also provide a glimpse about customizing parameters for inter-kingdom protein subcellular targeting prediction in case of microbial pathogenesis-related studies.
Pathogen’s protein targeting in host subcellular compartments is an important part of microbial pathogenesis.
Computational prediction of this targeting is a common practice now.
Detection of prokaryotic protein targeting in eukaryotic host cell should be done carefully.
Host subcellular compartment targeting can be analyzed by different approach-based tools, considering several other host pathogen factors.
Supplementary data
Supplementary data are available online at http://bib.oxfordjournals.org/.
Abdul Arif Khan is working as an Assistant Professor in Department of Pharmaceutics, College of Pharmacy, King Saud University, Riyadh, Saudi Arabia. He has strong research interest in the field of cancer associated infections including study of host-pathogen interactions using system biology approaches. He is involved in using computational approaches to decipher role of microbes in cancer etiology and diagnosis.
Zakir Khan is a Scientist at the Department of Biomedical Sciences, Cedars-Sinai Medical Center, Los Angeles, USA. He has major research interest in understanding of molecular mechanisms for identifying novel targets/strategies in cancer treatment. He is also involved in using computational approaches to understand molecular mechanisms behind cancer etiology.
Mohd Abul Kalam is working as an Assistant Professor at Department of Pharmaceutics, College of Pharmacy, King Saud University, Riyadh, Saudi Arabia. His research area is to conduct rigorous translational nanomedicine for promising improvements of potential therapeutics. His expertise is in nanotechnology including the role for computational approaches in nanotechnology research.
Azmat Ali Khan is working as an Assistant Professor in Pharmaceutical Biotechnology Laboratory, Department of Pharmaceutical chemistry, College of Pharmacy, King Saud University, Riyadh, Saudi Arabia. His research interest focus is on drug delivery via lipid nanoparticles. He is also working on study of host-pathogens interactions using computational and wet lab tools.
Acknowledgments
The authors are grateful to Deanship of Scientific Research and Research Centre, College of Pharmacy, King Saud University.