-
PDF
- Split View
-
Views
-
Cite
Cite
Vincent Berardi, Christine B Phillips, Mindy L McEntee, Chad Stecher, Michael Todd, Marc A Adams, The Impact of Monetary Incentives on Delay Discounting Within a Year-Long Physical Activity Intervention, Annals of Behavioral Medicine, Volume 58, Issue 5, May 2024, Pages 341–352, https://doi.org/10.1093/abm/kaae009
- Share Icon Share
Abstract
Delay discounting is the depreciation in a reward’s perceived value as a function of the time until receipt. Monetary incentive programs that provide rewards contingent on meeting daily physical activity (PA) goals may change participants’ delay discounting preferences.
Determine if monetary incentives provided in close temporal proximity to meeting PA goals changed delay discounting, and if such changes mediated intervention effects.
Inactive adults (n = 512) wore accelerometers during a 12-month intervention where they received proximal monetary incentives for meeting daily moderate-to-vigorous PA (MVPA) goals or delayed incentives for study participation. Delay discount rate and average MVPA were assessed at baseline, end of intervention, and a 24-month follow-up. Using structural equation modeling, we tested effects of proximal versus delayed rewards on delay discounting and whether any changes mediated intervention effects on MVPA. PA self-efficacy was also evaluated as a potential mediator, and both self-efficacy and delay discounting were assessed as potential moderators of intervention effects.
Proximal rewards significantly increased participants’ delay discounting (β = 0.238, confidence interval [CI]: −0.078, 0.380), indicating greater sensitivity to reinforcement timing. This change did not mediate incentive-associated increases in MVPA at the end of the 12-month intervention (β = −0.016, CI: −0.053, 0.019) or at a 24-month follow-up (β = −0.020, CI: −0.059, 0.018). Moderation effects were not found.
Incentive-induced increases in delay discounting did not deleteriously impact MVPA. This finding may help assuage concerns about using monetary incentives for PA promotion, but further research regarding the consequences of changes in delay discounting is warranted.
Lay Summary
This study examined the effects of providing proximal monetary incentives for meeting daily exercise goals on people’s tendency to value immediate versus delayed rewards. Inactive adults (n = 512) participated in a year-long program where they wore an accelerometer each day and received either (i) small monetary rewards in close temporal proximity to instances of meeting daily exercise goals or (ii) larger rewards at 2-month intervals for ongoing participation. Those receiving proximal incentives showed an increased preference for immediate rewards, yet this reported change did not compromise long-term physical activity gains. In a comparison analysis, we found that proximal monetary incentives were not associated with changes in exercise self-efficacy. Overall, the findings suggest that monetary incentives for exercise do not negatively impact people’s activity levels, though more research is needed to fully understand the implications of changes in reward timing preferences.
Introduction
Regular physical activity (PA) yields a suite of benefits for physical and mental health that prevent chronic disease and all-cause mortality [1], yet many U.S. adults do not meet federal PA guidelines [2]. Improvement in PA since the U.S. Department of Health and Human Services first released its Physical Activity Guidelines for Americans in 2008 has been modest [3, 4], indicating that more than a decade of interventions have yet to make sufficient progress on a population level. Emerging PA interventions that leverage wearable technology offer treatment approaches that can now be more personalized, optimized, and ultimately promise to be more effective, but this requires a better understanding of the time-varying determinants of PA behavior. One such factor is delay discounting, defined as the degree to which the consequences of a behavior are devalued as a function of the delay in experiencing those consequences [5]. Delay discounting has been suggested as a critical psychological attribute for understanding PA initiation and maintenance [6, 7].
When assessing delay discounting, a stronger preference for immediate rewards is often interpreted as an indicator of impulsivity [8], with neurological studies linking this characteristic to the brain’s limbic area associated with emotion processing [9]. In contrast, prioritizing longer-term goals over smaller, more immediate rewards is associated with the activation of the prefrontal cortex and posterior parietal cortex regions associated with executive functioning [9]. A large body of work has shown that higher discount rates (i.e., less tolerance for delayed reinforcers) are associated with a number of maladaptive behaviors and outcomes, including drug abuse [10], gambling [11], high-risk sexual practices [12], and lower levels of self-reported PA [13, 14]. These observations have led to delay discounting being described as a universal, “trans-disease process” [15].
Delay discounting rates tend to be relatively stable over time [16], leading to them being treated as a personality trait [8]. However, as seen in multiple systematic reviews detailing the effectiveness of various discount-altering techniques [17–19], discounting rates can be mutable. Of particular relevance to PA promotion are monetary incentive interventions, where participants are given proximal incentives contingent on increasing PA [20, 21]. While monetary incentive interventions have been shown to be effective for encouraging PA [22] and other health behaviors [23–25], potential mechanisms of action, such as modifying participants’ tolerance for delayed reinforcement, are largely understudied, with some notable exceptions [26–29]. Thus far, studies examining the impact of incentive interventions on delay discounting have primarily concerned programs that provide monetary incentives for abstaining from tobacco smoking, as was the case for five of the seven studies [30–33] outlined in the above-referenced systematic reviews [17–19], with the other two trials concerning cannabis [34] and opioid use [35]. Another study not included in the reviews incentivized substance-free behavior for heavy drinkers [36]. Evidence concerning the ability of monetary incentives to change discounting is mixed, with two of the smoking interventions [32, 33] and the opioid intervention [35] decreasing discounting, and an additional smoking trial doing so only for women [31]. It is unknown if monetary incentive interventions for PA, which reinforce adopting a healthy behavior rather than abstaining from deleterious behavior, impact delay discounting, and whether such a change would represent a behavior change mechanism.
Cross-sectional studies have found a negative relationship between delay discounting and PA [13, 14, 37], but to date, we are not aware of prospective studies that have examined this association in the context of incentive interventions. Contingent rewards are more effective when provided in close temporal proximity to a behavior [38], so we hypothesize that the impact of monetary incentives on delay discounting will be more acute when they are provided more immediately in response to performing a target PA behavior (e.g., meeting a daily exercise goal). But the direction of this potential effect is unknown. On the one hand, monetary incentives can promote the repetition of forgoing the short-term rewards produced by sedentary behavior (e.g., a lack of exertion, stimuli from television) in favor of the more nebulous delayed rewards associated with more movement (e.g., improved appearance or health). This may strengthen executive functioning and reduce discounting, which would mirror the changes observed in drug-abstinence incentive programs and align with work positing changes in discounting as the mechanism by which contingency-based interventions operate [39]. On the other hand, participants could become accustomed to repeatedly experiencing proximal, differential reinforcement for PA and begin to anticipate rewards, resulting in greater sensitivity to reinforcement timing, that is, higher discounting. This outcome would bolster critiques of contingent monetary incentives as undermining, or “crowding out,” intrinsic motivation [40]. Another factor that complicates possible associations among monetary incentives, discounting, and PA is that, in contrast to viewing exercise as a sacrifice to be endured in return for future health outcomes, PA possesses inherent, temporally discounted reinforcing qualities [41, 42].
This study explores potentially complex relationships among proximal monetary incentives, delay discounting, and PA within a year-long intervention for insufficiently active adults. We considered a mediational process where incentives induce changes in discounting that, if present, in turn initiate changes in PA (proximal incentives → discounting → PA). Several comparative analyses were also performed. First, because self-efficacy has a well-documented relationship with monetary incentives and PA [28, 29, 43], to contextualize any observed delay discounting effects, we estimated a proximal incentives → PA self-efficacy → PA mediation model. Next, we estimated an adaptive goals → discounting → PA mediation model. While adaptive goals have been shown to support sustained PA engagement, they do not have a theoretical connection to delay discounting, allowing this model to assess the face validity of any observed delay discounting effects. Lastly, based on findings from previous studies [7, 13, 14, 44], we also examined the potential moderation of the effect of proximal incentives on PA due to participants’ baseline levels of delay discounting and PA self-efficacy.
Critically, all analyses are performed prospectively on longitudinal data, enabling the specification of temporal relationships among treatment, potential mediators/moderators, and PA [45]. Given the popularity of monetary incentives for PA promotion and that delay discounting is thought to be a universal risk factor associated with multiple health outcomes, a better understanding of the interplay among these elements can inform how practitioners intervene to increase adoption of PA and other health promoting behaviors.
Methods
Procedures and Sample Description
Data for this study were collected from May 2016 through May 2019 as part of the Walking Interventions Through Texting (WalkIT) Arizona study, a 2 × 2 factorial trial with a year-long intervention aimed at increasing moderate-to-vigorous PA (MVPA) and an additional year-long, no-intervention follow-up. The Institutional Review Board at Arizona State University approved protocols for this study. The full details of this study intervention methods have been previously published [46], but an abbreviated description of the methodology is provided here. Insufficiently active adults (n = 512) were recruited primarily via social media with enrollment balanced over neighborhood walkability, neighborhood median household income, and calendar year (to account for extreme summer temperatures in Arizona). Sample demographics are provided in Table 1. Over the course of a 1-year intervention, participants were asked to wear an ActiGraph GT9X wrist-worn accelerometer (a solid-state device whose measures do not drift with time) during awake time and to sync the device daily with WalkIT Arizona data servers. Each participant’s device was individually calibrated to detect their unique MVPA signature. On each day, participants were assigned a daily MVPA goal via text message and, after syncing, were informed of whether this goal was met. Select participants (see below) received financial reinforcement for meeting daily goals and syncing the device in a timely manner. The assignment of goals, assessment of goal compliance, and disbursement of financial reinforcers were managed via an automated, cloud-based infrastructure.
Variable . | n . | % . | Mean . | SD . |
---|---|---|---|---|
Gender | ||||
Female | 330 | 64.5 | ||
Male | 182 | 35.5 | ||
Ethnicity | ||||
White, non-Hispanic | 363 | 70.9 | ||
Non-White | 117 | 22.9 | ||
Prefer not to answer | 32 | 6.3 | ||
Age | 45.5 | 9.1 | ||
BMI | 33.8 | 7.3 | ||
Delay discountinga | ||||
Baselineb | −5.26 | 1.47 | ||
12 monthsb | −4.94 | 1.48 | ||
24 monthsb | −4.84 | 1.49 | ||
PA self-efficacy | ||||
Baselineb | 5.91 | 1.94 | ||
12 monthsb | 4.91 | 2.34 | ||
24 monthsb | 4.94 | 2.53 | ||
Mean daily bout minutes | ||||
Baselineb (M0) | 15.6 | 11.1 | ||
12 monthsb (M12) | 26.6 | 20.1 | ||
24 monthsb (M24) | 21.4 | 18.3 |
Variable . | n . | % . | Mean . | SD . |
---|---|---|---|---|
Gender | ||||
Female | 330 | 64.5 | ||
Male | 182 | 35.5 | ||
Ethnicity | ||||
White, non-Hispanic | 363 | 70.9 | ||
Non-White | 117 | 22.9 | ||
Prefer not to answer | 32 | 6.3 | ||
Age | 45.5 | 9.1 | ||
BMI | 33.8 | 7.3 | ||
Delay discountinga | ||||
Baselineb | −5.26 | 1.47 | ||
12 monthsb | −4.94 | 1.48 | ||
24 monthsb | −4.84 | 1.49 | ||
PA self-efficacy | ||||
Baselineb | 5.91 | 1.94 | ||
12 monthsb | 4.91 | 2.34 | ||
24 monthsb | 4.94 | 2.53 | ||
Mean daily bout minutes | ||||
Baselineb (M0) | 15.6 | 11.1 | ||
12 monthsb (M12) | 26.6 | 20.1 | ||
24 monthsb (M24) | 21.4 | 18.3 |
BMI body mass index; PA physical activity.
aCalculated from the Monetary Choice Questionnaire as the log-transformed geometric mean of discounting values for small, medium, and large reward magnitudes.
bDue to dropout, the means at each time point summarize a different number of participants, with baseline/12 months/24 months sample sizes as follows—delay discounting: 512/418/356; PA self-efficacy: 512/418/386; and mean daily bout minutes: 512/387/365.
Variable . | n . | % . | Mean . | SD . |
---|---|---|---|---|
Gender | ||||
Female | 330 | 64.5 | ||
Male | 182 | 35.5 | ||
Ethnicity | ||||
White, non-Hispanic | 363 | 70.9 | ||
Non-White | 117 | 22.9 | ||
Prefer not to answer | 32 | 6.3 | ||
Age | 45.5 | 9.1 | ||
BMI | 33.8 | 7.3 | ||
Delay discountinga | ||||
Baselineb | −5.26 | 1.47 | ||
12 monthsb | −4.94 | 1.48 | ||
24 monthsb | −4.84 | 1.49 | ||
PA self-efficacy | ||||
Baselineb | 5.91 | 1.94 | ||
12 monthsb | 4.91 | 2.34 | ||
24 monthsb | 4.94 | 2.53 | ||
Mean daily bout minutes | ||||
Baselineb (M0) | 15.6 | 11.1 | ||
12 monthsb (M12) | 26.6 | 20.1 | ||
24 monthsb (M24) | 21.4 | 18.3 |
Variable . | n . | % . | Mean . | SD . |
---|---|---|---|---|
Gender | ||||
Female | 330 | 64.5 | ||
Male | 182 | 35.5 | ||
Ethnicity | ||||
White, non-Hispanic | 363 | 70.9 | ||
Non-White | 117 | 22.9 | ||
Prefer not to answer | 32 | 6.3 | ||
Age | 45.5 | 9.1 | ||
BMI | 33.8 | 7.3 | ||
Delay discountinga | ||||
Baselineb | −5.26 | 1.47 | ||
12 monthsb | −4.94 | 1.48 | ||
24 monthsb | −4.84 | 1.49 | ||
PA self-efficacy | ||||
Baselineb | 5.91 | 1.94 | ||
12 monthsb | 4.91 | 2.34 | ||
24 monthsb | 4.94 | 2.53 | ||
Mean daily bout minutes | ||||
Baselineb (M0) | 15.6 | 11.1 | ||
12 monthsb (M12) | 26.6 | 20.1 | ||
24 monthsb (M24) | 21.4 | 18.3 |
BMI body mass index; PA physical activity.
aCalculated from the Monetary Choice Questionnaire as the log-transformed geometric mean of discounting values for small, medium, and large reward magnitudes.
bDue to dropout, the means at each time point summarize a different number of participants, with baseline/12 months/24 months sample sizes as follows—delay discounting: 512/418/356; PA self-efficacy: 512/418/386; and mean daily bout minutes: 512/387/365.
Prior to the start of the intervention, participants were enrolled in a measurement-only baseline period, scheduled for 10 days. Participants were recruited by high/low neighborhood walkability and high/low socioeconomic status strata and block randomized into one of four treatment groups defined by two levels of daily goals (static vs. adaptive) and two levels of reinforcement timing conditions (proximal vs. delayed). In the static goal condition, the daily MVPA goal was 30 min, and in the adaptive goal condition, the goal was based on a moving-average-type approach using each individual’s unique MVPA recorded over the previous 9 days [47, 48]. In the proximal reinforcement condition, participants were provided with a message indicating that they had earned a monetary incentive immediately after meeting their daily goal and syncing their accelerometer with project servers. (Syncing was required to be completed by noon the next day.) The reinforcement value was initially $1.00. Once a participant met 24 daily goals, the magnitude of rewards began to vary, and after 48 goals were met, reinforcement was governed by a sequence of variable ratio schedules such that 67%–92% of daily goals met were rewarded. In all cases, the average daily reward was held constant at $1.00. (Reinforcement schedules are discussed in greater detail in Ref. [49].) In the delayed reinforcement condition, participants received monetary incentives at 60-day intervals for wearing and syncing their device with an escalating reward structure that ranged from $15 to $95. Monetary incentives were provided via e-gift cards redeemable at an establishment of the participant’s choosing (e.g., Amazon, Target, Sephora, Home Depot, Starbucks, etc.) and were immediately sent when the total accrued reward exceeded $5.00.
Measures
Based on prior work by Barnett et al. [50], participants underwent individual calibrations of the ActiGraph GT9X using indirect calorimetry at baseline, which allowed each minute of recorded wear time to be characterized as either an MVPA or a non-MVPA minute based on >3 metabolic equivalents. An MVPA bout began when 3 out of 5 min (not necessarily contiguous) were scored as MVPA and ended when 3 out of 5 min were scored as non-MVPA. A similar approach has been used for NHANES hip-worn accelerometer data, where MVPA bouts were defined as 8 (potentially noncontiguous) min of MVPA out of any 10 min [51, 52]. Since no consensus for scoring MVPA bouts was present in the literature, our approach was based on measurement fidelity and was designed to avoid nonambulatory arm activities (e.g., washing dishes) being counted as MVPA. It ensured that bouts contained a minimum of three MVPA minutes, which was more conservative than including all MVPA minutes, while still largely aligning with the 2018 US Physical Activity Guidelines Advisory Committee Scientific Report’s statement that “bouts of any length contribute to the health benefits associated with…physical activity” [53]. While our definition is not an analytic standard, it reflects the unique needs of this novel intervention. Analyses only considered MVPA bouts on valid wear days, defined as (i) the accelerometer being worn for at least 600 min and/or (ii) the participant’s daily MVPA goal for that day being met. The second criterion was instituted to minimize potential demoralization by allowing participants to be eligible to receive the expected financial reward for occasions when they met their daily MVPA goals, even if they did not meet the 600-min wear criterion. Nonvalid wear days were excluded from all analyses.
Delay discounting was assessed via the 27-item Monetary Choice Questionnaire (MCQ) [54], used in conjunction with the Kaplan et al. automated scorer [55]. For each participant, this instrument yields discounting values in units of days for small, medium, and large reward magnitudes. An overall composite score was created by calculating the geometric mean of these three values, that was then log-transformed to account for a positive skew in their distribution. Higher values on this metric are indicative of greater discounting, that is, less tolerance for reinforcement delay. Possible logged geometric mean values range from −8.74 to −1.39, where the minimum and maximum values indicate exclusively choosing either delayed or immediate rewards, respectively.
Self-efficacy for PA was measured via a questionnaire based on the validated instrument developed by Marcus et al. [56], but modified for a recruited sample from Arizona (e.g., items mentioning “rain” were changed to discuss “poor weather”). Participants were provided with six scenarios (poor weather, tired, busy day, sad/stressed, on vacation, 5 days-per-week) and asked about their confidence that they could be moderately physically active for 30 min. Responses were scored on a 10-point Likert scale from 0 (strongly disagree) to 9 (strongly agree) and the average across all items was calculated as the self-efficacy score.
MVPA, delay discounting, and self-efficacy were assessed at three time points corresponding to baseline, the end of the 12-month intervention phase, and a 24-month follow-up. To minimize participant burden, mid-intervention measures of delay discounting and self-efficacy were not collected. Average daily MVPA min/day was calculated over (i) a 10-day baseline period immediately preceding the start of the intervention phase, consistent with our previous research [48]; (ii) the last 14 valid days (or maximum number of valid days available) from intervention phase days 305–365 (to allow for participants who stopped generating accelerometer data before the trial ended); and (iii) during a 13-day follow-up period beginning approximately 24 months after the beginning of the trial. Delay discounting was measured at the beginning of the intervention, at the end of the intervention, and at the end of the follow-up PA assessment period.
Statistical Approach
Two WalkIT Arizona treatment characteristics were investigated: (i) proximal versus delayed rewards and (ii) adaptive versus static goals. Mediation and moderation analyses were guided by the Macarthur approach, which resolves ambiguity in the widely used Baron and Kenny definitions of these relationships by explicitly requiring moderating variables to precede treatment and mediating variables to follow treatment [45]. These temporal distinctions cannot be made with cross-sectional data, so it is critical to perform prospective studies that use longitudinal data, particularly for mediation [57].
Best practices for longitudinal mediation require the estimation of effects of variables on each other, as well as autoregressive effects, that is, the effects of the variables on themselves over time [58]. Structural equation models (SEMs) were used for this task (and for moderation analyses), since they can analyze repeated measures and simultaneously assess multiple causal paths, including direct and indirect effects [59, 60]. In accordance with Goldsmith et al. [60], the observed outcome and potential moderator/mediator measures at each time point were assumed to represent a true-score latent variable plus an error (residual) component. Accordingly, all factor loadings for observed variables on their corresponding latent variables were fixed at 1, and residual variances were fixed to be constant over time. Moderation/mediation effects among the latent variables were investigated and, for clarity, all figures and tables summarizing results show latent variables only.
Mediation
While theoretical considerations typically guide SEM model selection, when assessing mediation in longitudinal data, several theoretically plausible models exist, many of which make non-testable assumptions. Therefore, we followed the approach outlined by Goldsmith et al. [60] and fit several candidate models (simplex with contemporaneous and/or lagged effects, latent growth models with linear or empirical growth trajectories, and latent change score models) to the data and selected the one that best fit the data, as indicated by the lowest Akaike Information Criteria. The simplex model with contemporaneous mediation performed best, so it was used for assessing longitudinal mediation. The interested reader is directed to Goldsmith et al. [60] for a more thorough description of all models that were considered.
A simplex model, as illustrated in Fig. 1, is a repeated measures SEM with first-order autoregressive latent components. Baseline, 12-month, and 24-month MVPA (Mi) and delay discount measures (ki) are denoted as M0(k0), M12(k12), and M24(k24), respectively. In Fig. 1, the effect of proximal reinforcement (r = 1) versus delayed reinforcement (r = 0) on change in delay discounting at 12 months is given by the path
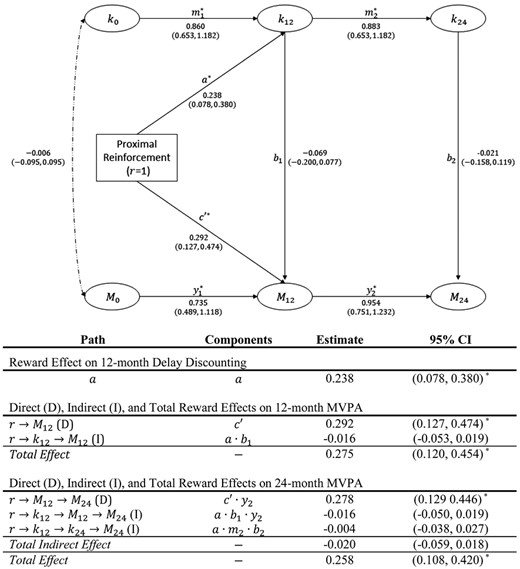
Path diagram and results for simplex model with contemporaneous mediation for proximal versus delayed reward as treatment and delay discounting as a potential mediator. Note. Measurements over three time points are shown in ovals and are designated as ki for delay discounting and Mi for MVPA with i = 0, 12, and 24 months. Each path has an alpha or alpha-numeric designation as well as an estimated coefficient and 95% confidence interval (CI). The table below the figure summarizes the delay discounting outcome as well as direct (D), individual indirect (I), total indirect, and total effects at both the 12- and 24-month measurement occasions. CIs that do not contain 0 and are therefore statistically significant are marked with an asterisk. MVPA moderate-to-vigorous physical activity.
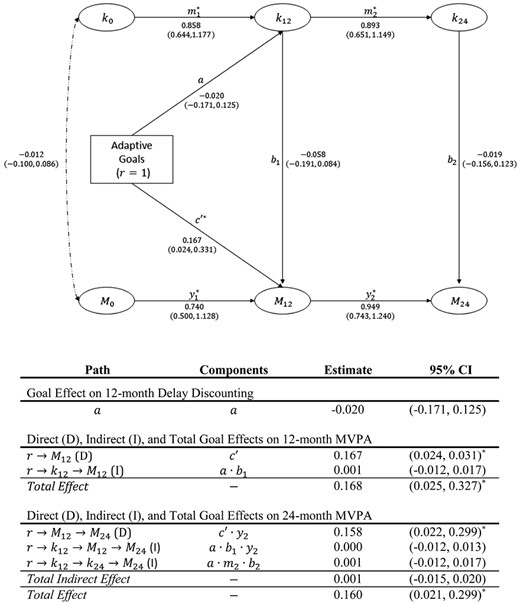
Path diagram and results for simplex model with contemporaneous mediation for adaptive versus static goals as treatment and delay discounting as a potential mediator. Note. See Fig. 1 caption/notes for a description of figure components.
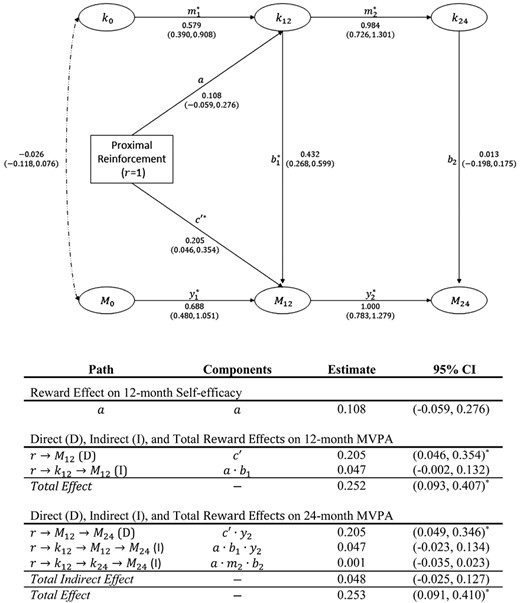
Path diagram and results for simplex model with contemporaneous mediation for proximal versus delayed reward as treatment and self-efficacy as a potential mediator. Note. See Fig. 1 caption/notes for a description of figure components. Note that k12 represents self-efficacy measured at the 12-month time point.
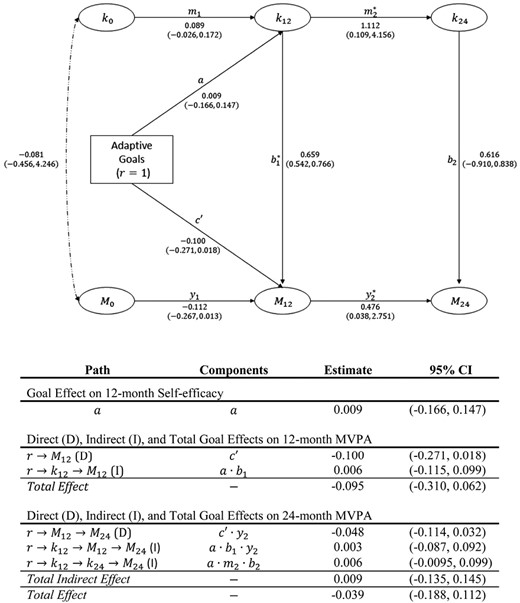
Path diagram and results for simplex model with contemporaneous mediation for adaptive versus static goals as treatment and self-efficacy as a potential mediator. Note. See Fig. 1 caption/notes for a description of figure components. Note that k12 represents self-efficacy measured at the 12-month time point.
Statistical analyses were performed in Mplus Version 7. All independent and dependent variables were standardized. Paramters' maximum likelihood estimates were generated via full-information maximum likelihood procedures. Confidence intervals (CIs) for effects were obtained via bootstrapping with 1,000 samples, which was sufficient for estimates to converge. Following Goldsmith et al. [60], covariances between baseline delay discounting and non-baseline MVPA measures were not estimated.
Moderation
The MacArthur approach to moderation requires a moderator variable to precede the treatment, so only baseline measures were candidate moderators and only the M12 measure, from when the treatment was active, was considered as an outcome. Following recommendations [61], an SEM with was fit with paths to 12-month MVPA from (i) the intervention indicator (either proximal vs. delayed reward or static vs. adaptive goals), (ii) baseline discounting or self-efficacy, and (iii) their interaction. Additionally, an autoregressive path for the association between baseline and 12-month MVPA was included (see Fig. 5).
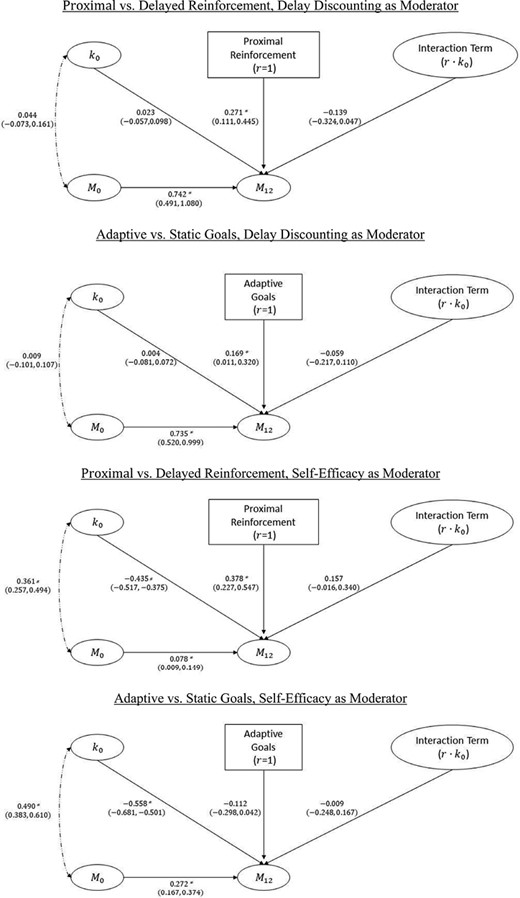
Path diagram and results for moderation models. Note. r represent treatment status, k0 represents moderator during the baseline time interval, and Mi for MVPA with i = 0, 12 during baseline and final 60 days, respectively. Each path has an estimated coefficient and 95% confidence interval (CI). CIs that do not contain 0 are marked with an asterisk. MVPA moderate-to-vigorous physical activity.
Results
Mediation
Figure 1 illustrates the results of the simplex model with contemporaneous mediation of proximal versus delayed rewards via changes in delay discounting. At baseline, delay discounting and MVPA were not significantly correlated (r = −0.006, 95% CI: −0.095, 0.095). Compared to delayed reinforcement, proximal rewards (i.e., r = 1) significantly increased 12-month MVPA (M12) via direct effects by 0.292 standard deviations (CI: 0.127, 0.474) and 24-month MVPA (M24) by 0.278 standard deviations (CI: 0.129, 0.446). These values correspond to a relative difference of 5.88 and 5.09 min/day, respectively. Proximal rewards also significantly increased discounting (i.e., made participants less tolerant of delay) by 0.238 standard deviations (CI: 0.078, 0.380). Notably, intervention-induced changes in delay discounting were persistent, as shown by the similar magnitude path coefficients between baseline and 12 months (0.860, CI: 0.653, 1.182) and 12 and 24 months (0.883, CI: 0.662, 1.108). Delay discounting was not significantly associated with MVPA at either the 12-month (b1 = −0.069, CI: −0.200, 0.077) or 24-month (b2 = −0.021, CI: −0.158, 0.119) measurement occasions. Indirect effects of proximal rewards on MVPA via delay discounting were not significant.
Mediation effects were also not significant in the comparison mediation analyses, largely due to the fact that, in contrast to the primary analysis, treatments did not impact potential mediators. As shown in Fig. 2, adaptive versus static goals did not have a significant effect on 12-month delay discounting, and discounting, in turn, was not significantly associated with 12-month MVPA. When considering self-efficacy as a potential mediator, neither the proximal reward nor adaptive goal treatments impacted self-efficacy (Figs. 3 and 4, respectively). However, in both cases, self-efficacy and MVPA were significantly associated at the 12-month time point (b1 = 0.432, CI: 0.268, 0.599 for proximal rewards; b1 = 0.659, CI: 0.542, 0.766 for adaptive goals), although overall indirect effects were not significant.
Moderation
As shown in Fig. 5, for participants in the proximal reward condition, change in MVPA from baseline to 12 months was 0.271 standard deviations higher (CI: 0.111, 0.445) than for those in the delayed reward condition, consistent with previously reported results for this study, where a 16.54 min/day increase in MVPA for the proximal reinforcement group was found [62]. Neither baseline discounting (k0: 0.023, CI: −0.057, 0.098) nor the
Similarly, comparative analyses did not yield significant moderation effects. However, these analyses did find that baseline self-efficacy was negatively associated with 12-month MVPA (−0.435, CI: −0.517, −0.375 for proximal rewards; −0.558, CI: −0.681, −0.501 for adaptive goals).
Discussion
Our results indicate that, compared to the delayed reinforcement condition, proximal monetary incentives contingent on meeting MVPA goals over the course of a year significantly increased: (i) MVPA by 5.88/5.09 min/day at 12/24 months and (ii) delay discounting by 0.292/0.278 standard deviations. The first effect is consistent with our previous work that used a statistical model that analyzed an entire year’s worth of daily accelerometer data (in contrast to summary metrics at three time points herein) to demonstrate increases in MVPA of 16.54 and 9.91 min/day, respectively, for the proximal and delayed reward conditions (difference = 6.63 min) [62]. For context, a review/meta-analysis of interventions that used wearables and/or smartphone apps to objectively measure PA found that the weighted average change score in treatment groups was 7.45 min (9.96 min when eliminating studies with high risk of bias) [63]. Outcomes from both treatment groups in our study exceed this average, which is notable considering the intervention occurred over 1 year, compared to durations ranging from 8 weeks to 6 months in the meta-analysis studies. Furthermore, the US Federal PA Guideline of 150 weekly minutes of MVPA corresponds to 21.4 min/day, so the relative differences observed between the treatment and active control conditions in this study (~5 min/day) represent roughly 25% of the guideline.
The increase in delay discounting is a moderate-sized effect and suggests that proximal rewards made participants significantly less tolerant of delays in reinforcement. We speculate that as participants became accustomed to repeatedly experiencing proximal and differential reinforcement for MVPA, they began to anticipate rewards, resulting in greater sensitivity to reinforcement timing and therefore higher discounting. This result does not support the hypothesis that monetary incentives activate executive functioning skills [39], which stands in contrast to findings from drug-abstinence programs. This discrepancy may be due to differences associated with incentivizing participants to adopt a behavior, rather than abstaining from one via the differential reinforcement of other activities. It might also be associated with the fact that for some participants, PA offers immediate gratification and can be reinforcing in its own right, which has been observed for both animals [64] and humans [65]. Experiments have shown that PA, as a reinforcer, is temporally discounted at a rate similar to that seen for consumable rewards such as food and cigarettes [41] and different from money [42], which is notable since discounting in this study was assessed via the monetary choice questionnaire.
Of key importance is that the increases in delay discounting produced by proximal incentives in this study did not impact MVPA, that is, this change did not mediate the higher levels of MVPA associated with the proximal incentives program. Because higher discounting is typically associated with lower levels of PA [13, 37, 66–68], one could conclude that proximal incentives improved PA outcomes in spite of, rather than due to, changes in delay discounting. However, caution should be used when comparing the cross-sectional studies that have linked higher discounting to less PA and the longitudinal results presented herein. A similar misalignment might also explain why our longitudinal analyses did not show that pre-intervention discounting moderated the proximal incentive’s effect on MVPA, which runs counter to a moderating effect found with a cross-sectional analysis of a study with a similar intervention approach [7].
Whereas proximal reinforcement significantly changed delay discounting, self-efficacy for PA was not impacted by either proximal reinforcement or adaptive goals, which may be a function of our intervention not explicitly targeting self-efficacy. Interestingly, the mediation models showed a positive relationship between PA self-efficacy and MVPA at 12 months, but the moderation models found a negative association between baseline PA self-efficacy and MVPA. This finding could reflect self-efficacy being irrelevant when participants are insufficiently active (at baseline), but becoming more salient once PA is more consistently performed. Future work should investigate this phenomenon and other intervention factors that could impact self-efficacy during intensive, adaptive interventions.
WalkIT Arizona used monetary incentives to encourage adopting a health behavior, which can lead to apprehension about (i) the psychological effects associated with the continued presentation of contingent rewards and (ii) the sustainability of an intervention strategy that requires an ongoing financial commitment. Our results address the psychological concern by showing that incentive-induced decreases in discounting did not impact MVPA behavior. This finding is consistent with Promberger and Marteau’s [69] review that reported no evidence for hypothesized motivational changes (e.g., crowding out) due to monetary incentives when complex, health-related behaviors were studied. The sustainability concern is assuaged by our finding that MVPA increases were found for both the 12-month end-of-treatment and 24-month follow-up measurements, meaning that the proximal incentives protocol impacted behavior up to 1 year after reinforcement had terminated. However, these study results do raise a new concern about the potential for incentive-induced increases in delay discounting to generate negative outcomes in other health domains, a possibility that should be explored in future research.
This study’s investigation into the operational mechanisms for a successful behavioral intervention represents a step toward critically advancing behavior science. The transition from observing an outcome to understanding the underlying mechanisms that govern it is a natural part of the scientific process. Yet, the identification of health behavior mechanisms has not received sufficient attention. As behavior science matures as a discipline (often aided by technological advances), such investigations are needed and will likely allow interventions to be better understood and more easily optimized.
From a methodological standpoint, a significant strength of this study is its prospective examination of delay discounting and self-efficacy as mediators/moderators in a large sample of adults within a factorial design that collected measures longitudinally over 2 years. However, it is important to acknowledge the study’s limitations. First, the sample was recruited from a single geographic region and consisted of mainly overweight adults, which limits the generalizability of findings. However, the stratified sampling approach resulted in a similar number of individuals from high- and low-income areas randomized to the factorial conditions, thereby enhancing external validity. Second, although participants were blinded to the hypotheses during the study, neither researchers nor participants were blinded to experimental conditions. Third, despite implementing rigorous accelerometry quality control procedures, potential measurement error cannot be entirely dismissed, especially when assessing PA daily over the course of an entire year. Fourth, outside of responding to technical difficulties, participants’ interaction with project personnel over the course of the intervention only occurred at a 6-month assessment point. While this enhanced the scalability of our approach, it may have also led to decreased engagement. Furthermore, due to the need to balance participant burden, delay discounting and self-efficacy measures were not collected at the 6-month assessment. Fifth, while participants in the proximal rewards arm were instantly notified that a reward was earned when project servers detected MVPA goal attainment, operational limitations dictated that participants received payments only after $5 had been accrued. In some cases, this led to delays between the earning and presentation of a reward, potentially diluting the strength of the intervention. Furthermore, because two-thirds of participants in the proximal reinforcement arm eventually progressed to a variable ratio reinforcement schedule, differential group outcomes may be attributable to differences in reinforcement timing (proximal vs. delayed), reinforcement ratio (continuous vs. variable), or the combination of the two. Sixth, data from follow-up period were collected via accelerometers mailed to participants after several months with no contact from study personnel; therefore, reactivity to this technology could be a concern. Lastly, although the MCQ is a validated, popular measure of delay discounting used across research fields, it relies on participants making decisions about hypothetical monetary rewards. Thus, the MCQ assumes no differences in discounting of hypothetical and real monetary incentives and likely does not capture the discounting of rewards associated with exercise. Future research should address this shortcoming.
In conclusion, the longitudinal measures of PA and delay discounting collected in this study make it uniquely capable of assessing incentive-related changes in discounting. We found that contingent monetary incentives altered delay discounting on a long-term basis, but this change did not mediate intervention-induced increases in MVPA. These results suggest that focusing PA incentive intervention efforts on executive functioning may not be optimal, but more work is required to fully understand monetary incentive behavior change mechanisms on both health behavior adoption and maintenance.
Funding
This work was supported by the National Cancer Institute at the National Institutes of Health [R01CA198915].
Compliance with Ethical Standards
Authors’ Statement of Conflict of Interest and Adherence to Ethical Standards Vincent Berardi, Christine B. Phillips, Mindy L. McEntee, Chad Stecher, Michael Todd, and Marc A. Adams declare that they have no conflict of interest.
Authors’ Contributions Vincent Berardi (Conceptualization [supporting], Formal analysis [lead], Methodology [lead], Writing – original draft [lead], Writing – review & editing [lead]), Christine B. Phillips (Conceptualization [supporting], Funding acquisition [supporting], Methodology [supporting], Writing – original draft [supporting], Writing – review & editing [supporting]), Mindy McEntee (Data curation [supporting], Methodology [supporting], Writing – original draft [supporting], Writing – review & editing [supporting]), Chad Stecher (Data curation [supporting], Methodology [supporting], Writing – original draft [supporting], Writing – review & editing [supporting]), Michael Todd (Data curation [lead], Funding acquisition [supporting], Methodology [supporting], Writing – original draft [supporting], Writing – review & editing [supporting]), and Marc A. Adams (Conceptualization [lead], Funding acquisition [lead], Project administration [lead], Writing – original draft [supporting], Writing – review & editing [supporting])
Transparency Statements (i) The study was preregistered at clinicaltrials.gov (NCT02717663). (ii) The analysis plan was not formally preregistered. (iii) Deidentified data from this study are not available in an a public archive. Deidentified data from this study will be made available (as allowable according to institutional IRB standards) by emailing the corresponding author. (iv) Analytic code used to conduct the analyses presented in this study are not available in a public archive. They may be available by emailing the corresponding author. (v) Materials used to conduct the study are not publicly available.