-
PDF
- Split View
-
Views
-
Cite
Cite
Chih-Hsiang Yang, Jennifer Zink, Britni R Belcher, Martina Kanning, Genevieve F Dunton, Age-varying Bi-directional Associations Between Momentary Affect and Movement Behaviors in Children: Evidence From a Multi-wave Ecological Momentary Assessment Study, Annals of Behavioral Medicine, Volume 55, Issue 9, September 2021, Pages 918–931, https://doi.org/10.1093/abm/kaaa124
- Share Icon Share
Abstract
The bi-directional associations between affective states and movement behaviors (e.g., physical activity, sedentary behavior) have been observed in children. It is unclear if the strength of these bi-directional associations varies with age as children transition from childhood to adolescence.
This multi-wave ecological momentary assessment (EMA) study investigates the acute time-varying associations between affect and movement behaviors among youth.
Children (N = 195, baseline mean age = 10.72, range = 8–12 years, mean BMI-z = 0.49, 51% female) participated in a six-wave EMA study across three years. Each EMA survey captured momentary positive and negative affect. Time spent in moderate-to-vigorous physical activity (MVPA) and sedentary time in the 15-min window before and after each EMA survey was calculated using accelerometry data. Time-varying effect models (TVEM) examined the acute bi-directional associations between momentary positive/negative affect and MVPA/sedentary time across ages 8 to 14.
Children provided 14,246 valid activity-matched EMA surveys across all waves. TVEM plots revealed that the directionality and the strength (time-varying slopes) of associations between momentary affect and activity levels vary across ages. Positive affect was associated with higher MVPA levels and lower sedentary time at younger ages, whereas negative affect linked to lower MVPA levels and more sedentary time at older ages.
The acute associations between momentary affect and (in)activity levels may vary as a function of children's age. Applying TVEM to intensive longitudinal data could provide valuable information for developing age-tailored interventions that promote healthy lifestyles among children and adolescents.
Introduction
As youth developmentally transition from childhood to adolescence, physical activity levels decline, and time spent sedentary increases [1–3]. Paralleling these changes in activity levels, adolescence can also be a period of vulnerability for the development of anxiety and depressive symptoms for some youth [4, 5]. It has been postulated that activity levels and emotional health are associated with one another during childhood and adolescence, which can pose major health concerns [6, 7]. In addition to implications for mental health, decreases in physical activity and increases in sedentary time act synergistically to elevate the risk for non-communicable diseases such as Type 2 diabetes and cardiovascular disease as adolescents transition into adulthood [8–11]; and the rising emotional distress can also lead to more serious mental disorders with lifelong consequences [12, 13]. More critically, recent research suggests the decline in mental health and activity levels may bi-directionally stimulate each other and worsen overall health over time [6, 7].
In addition to examining the longitudinal relationships between activity levels and psychological well-being among children and adolescents, researchers have begun to investigate the acute affect-activity associations that occur across smaller time scales (e.g., hours, minutes) in everyday settings [14–16]. This is important to consider because acute associations between affect and activity may accumulate over time and contribute to children's overall levels of physical activity and mental health status. Recent advancements in technology have allowed investigators to apply real-time data capture techniques, such as ecological momentary assessment (EMA), to understand dynamic, subtle, and immediate relationships between activity levels and affective states in naturalistic settings [17, 18]. Children's affective states vary within each day and are subject to retrospective recall bias [19]. EMA offers a time-sensitive and ecologically valid way to capture how children's affective states may be related to their activities in everyday life [14]. Through leveraging the timestamps recorded from both the EMA device (e.g., smartphones) and the activity monitor (e.g., accelerometer) that create the sequential order between affect and activity, researchers can investigate how affective states may serve as antecedents or consequences of different movement behaviors [17, 20]. For example, an EMA study found that children (8–12-years old) experienced greater positive affect following moderate-to-vigorous physical activity (MVPA) and they reported lower positive affect after time spent in sedentary behaviors [16]. Other EMA studies in youth demonstrated that the acute associations between MVPA, sedentary time and affective states may be bi-directional such that affective states can also be determinants of subsequent activity levels [14, 21].
The aforementioned intensive longitudinal studies have observed the existence of within-day momentary, bi-directional associations between children's activity levels and affective experiences in free-living settings. However, it remains unclear whether the strength of acute associations between activity levels, and affective states vary within individuals as a function of children's developmental age. The transition from childhood to adolescence involves continuous physiological and psychosocial changes within individuals [22, 23], which may impact the affective response to activity or the behavioral response to affective experience over time. For instance, when children are younger, their everyday activity and behaviors are usually more responsive to their feelings, but they have lower volition in activity decision [24]. However, when children are older, they have higher awareness of body image, they change in their sports motivation, and they increase reward-seeking behaviors due to the development of specific brain region(s) (i.e., the striatum) that facilitates voluntary movements and produces feelings of pleasure [25, 26]. Given both the activity and affective differences in children and adolescents, it is conceivable that the associations between activity levels and acute affective states may also be dynamic and may vary across this developmental period. No study to date has taken a developmental perspective by investigating age-varying acute associations in children's everyday activity levels and affect; this type of information is critically needed because it can inform behavioral intervention strategies tailored to children at specific ages to slow down or prevent declines in their activity levels and mental health.
Time-varying effect modeling (TVEM), a statistical approach that is especially useful for studies where repeated measures are taken across time (e.g., multi-wave EMA studies), can fill this critical gap in the literature by increasing our understanding of whether the strength of affect-activity level associations changes over the course of child development. TVEM is a flexible modeling approach extended from linear regression that permits the intercept (the mean levels of an outcome) and the slope(s) (the association[s] between predictor[s] and an outcome) to change as a function of time. It estimates the intercept and slope coefficients using all available data from participants at a given time, so it allows missing data across time (e.g., participants dropping in and out of waves). Further, this modeling approach makes no assumptions regarding the “shape” (e.g., linear, quadratic) of the intercept or slope(s), allowing researchers to estimate the regression coefficients as flexible, nonparametric functions of time [27]. Therefore, by using TVEM, investigators are able to identify segment(s) of time when associations are likely to be the strongest or weakest.
Taken together, the present study aimed to examine whether the strength and direction of the acute associations between MVPA, sedentary time, and positive and negative affective states varied across children's chronological age. Previous findings suggest that children's physical activity, sedentary behavior, and mental health may change over time as children get older. Therefore, we generally hypothesized that the strength of the acute within-subject associations between activity behaviors and affective experiences would also vary over time during the transition from childhood to adolescence. Given the lack of previous research on how biological and psychosocial developmental changes impact affective response to activity or behavioral response to affective experience, determining at which ages that effects would be stronger or weaker were an exploratory goal. TVEM was thus applied to our multi-wave EMA and accelerometry data collected during the transition from late childhood to early adolescence to test these hypotheses.
Methods
Participants
Data were analyzed from 195 ethnically-diverse children (51% female; 56% Hispanic, baseline mean age = 10.16 years, SD = 0.87) who participated in the Mothers' and Their Children's Health (MATCH) Study. The MATCH study is a repeated-measures longitudinal observational study designed to investigate the within-day effects of maternal stress on children's obesity risk over time among mother-child dyads [28]. Children in this study were recruited between August 2014 and March 2016 from public elementary schools and after-school programs in the Los Angeles metropolitan area. Children were eligible to participate in the MATCH study if they were in the third to sixth grade upon enrollment and were able to read English. Children were excluded from the study if they had health issues that limited physical activity, were enrolled in special education programs, were using oral or inhalant corticosteroids for asthma, were classified as underweight by a Body Mass Index (BMI) percentile <5%, or if they were taking medications for thyroid function or psychological conditions (i.e., mood disorders, Attention Deficit Hyperactivity Disorder). The MATCH study consisted of six assessment waves conducted at approximately six-month intervals across three years. The current analysis included only the children's data from the mother-child dyads across the six measurement waves. A detailed description of the MATCH recruitment process and participant eligibility are reported elsewhere [28].
Procedures
Parental consent, child assent, and self-reported demographics were obtained during an initial in-person visit with the staff at neighborhood schools and community centers. All EMA items were explained to participants to ensure that they understood each of them during their visits. Participants were encouraged to ask any questions that they may have had regarding the EMA items and the study protocol. In each of the six assessment waves, they were asked to complete EMA surveys and to wear accelerometers for seven consecutive days. The EMA surveys were delivered via a customized smartphone application operated on Android-based devices. Participants without a compatible phone to receive the EMA prompts were loaned a MotoG smartphone (Motorola, USA). They met with the staff on the first day of each wave to pick up the study devices, complete demographic questionnaires, and undergo anthropometric assessments (i.e., height and weight). They met with the staff again on the last day of each wave to return the devices to receive compensation.
At each measurement wave, the EMA prompts were delivered during non-school hours, with approximately two hours between prompts. Children received random EMA survey prompts up to three times per weekday between 3 pm to 8 pm, and up to seven times per weekend day between 7 am to 8 pm. Upon receiving the prompt, children completed the short electronic survey on their smartphone to report their current affective states (i.e., positive and negative affect) and their social context (i.e., alone or not). Each EMA survey required about 2 min to complete, and children were asked to ignore EMA prompts that occurred during incompatible activities, such as during sleeping or bathing. Across the seven days of EMA at each measurement wave, children also wore Actigraph GT3X accelerometers (Actigraph Corp., Pensacola, FL) on their right hip to collect activity data. Children were instructed to wear the accelerometers during all non-sleep time except for swimming or bathing. Two institutional review boards approved all study procedures.
Measures
Positive and Negative Affect
The affective state questions were adapted from the validated Positive and Negative Affect Schedule for children [29, 30]. Participants self-rated how (i) happy, (ii) joyful, (iii) mad, (iv) sad, and (v) stressed they were at each EMA prompt on a i (not at all) to iv (extremely) scale. For positive affect, scores from the happy and joyful items were averaged at each prompt. For negative affect, scores from the mad, sad, and stressed items were averaged at each prompt. The within-subject internal consistency was high for the two positive affect items (ω = 0.90) and the three negative affect items (ω = 0.85).
Moderate-to-Vigorous Physical Activity and Sedentary Time
The accelerometers were set to collect data at a frequency of 30 Hz with a 30-sec epoch. Children's MVPA and sedentary time were defined by activity count thresholds applied previously in national surveillance studies [31, 32]. These thresholds were also adjusted for children's age using the Freedson prediction equation [33, 34]. Any periods that consisted of ≥60 continuous minutes of zero activity counts were considered as non-wear time.
Demographics, Social, and Temporal Factors
Self-reports of children's sex, date of birth at baseline, and their objectively measured weights and heights at each wave were used in the current analysis. Children's ages were calculated in years from the lapse between their self-reported date of birth and the first day of each measurement wave. Children's BMI (kilogram per meter square) was calculated at each measurement wave and further transformed to standardized BMI-z scores using the SAS program and growth charts provided by the Centers for Disease Control and Prevention [35]. Time of day (in 24 hr) and day of the week (weekends/weekdays) were derived from the date and time stamps of the EMA prompts. In each EMA survey, children also reported whether they were alone (vs. not alone). These demographic and temporal factors were included in the analysis as covariates based on their potential impact on daily physical activity and sedentary behavior patterns [31, 36–39].
Data Merging and Processing
Children's EMA and accelerometer data were merged by the timestamps for each child. The number of minutes of MVPA and sedentary time accumulated in the 15-min window before and after each EMA prompt was calculated to test the acute bi-directional associations [20, 40]. Partially completed EMA responses (i.e., children skipped EMA item(s) at a given prompt; occurred less than 5% of total EMA occasions) for any given prompt were also included in the final dataset. Only windows that contained 15 min of valid accelerometer wear time on valid wear days (having ≥10 hr of valid accelerometer wear time) were included in the current analyses.
Data Analysis
This study applied TVEM to test whether the acute associations between affect and activity levels in children varied across developmental age. In the current study, each child was enrolled in the study for approximately three years, starting from their baseline age (which ranged from 8.31 to 11.49 years at wave one). For example, a child who enrolled in the study at age 8 provided data up to around age 11, and a child who enrolled at age 10 provided data up to around 13 years old. Thus, for the available study sample, the age range of data spanned across six developmental years covering the transition period from late childhood to early adolescence (range = 8.31–14.57 years). The TVEM analysis in the current study was conducted using the SAS macro (%TVEM_normal) [41]. The parameter-function estimation was based on the P-spline method with 10 knots (dividing points), which is considered sufficient for capturing rather complicated time-varying phenomena or shapes [42].
Two sets of TVEMs (eight models in total) were carried out to examine age-varying bi-directional associations between momentary affect and activity levels in children. The first set of TVEMs (four separate models) was specified to predict children's momentary positive or negative affect as a function of their prior MVPA or sedentary time (in the 15-min window before the EMA prompt). The second set of TVEMs (also four separate models) was specified to predict children's MVPA or sedentary time in the 15-min window after the matched EMA prompt as a function of momentary positive or negative affect at that prompt. To account for children's general levels of affect and activity across the study period, children's mean levels of positive or negative affect across all EMA prompts were used as time-invariant covariates (i.e., between-subject effects) in the first set of models. Similarly, children's mean levels of MVPA or sedentary time in the 15-min prompt-matched windows before and after the EMA prompts were included as time-invariant covariates (i.e., between-subject effects) in the second set of models. Children's BMI-z score at each wave, whether the EMA response occurred on a weekend day (vs. weekday), the social context (alone or not) reported during the EMA response, and time of EMA response (0–24 hr) was entered as time-varying covariates in all models. Children's sex (a time-invariant covariate) reported at baseline was also controlled in both sets of models. The general equations for the two sets of TVEMs can be presented as follows:
where Momentary Affectij, Activity Levelsij, BMI-zij, Weekendij, Aloneij, Response timeij are intensively measured longitudinal variables for child i from assessment occasion j measured at time(age) tij. Momentary Affectij represents either the positive affect or negative affect at each EMA prompt. Activity Levelsij represents either children's MVPA or sedentary minutes in the 15 min before (first set of models) or after (second set of models) each EMA prompt. Mean Affecti represents each child's mean levels of positive or negative affect across all EMA prompts. Sexi represents a child's sex at baseline. β 0(t) is the mean level or the intercept function of momentary affect or activity for children with average levels of all covariates at time t. β 1(t)–β 7(t) are the slope functions describing the time-varying associations between the predictors and outcomes. ɛ ij represents the error term for child i at occasion j.
Results
Children's accelerometry data were included if they provided at least one valid wear day (had at least 10 hr of valid accelerometer wear time on a given day). Each child in this study provided an average of 25.53 valid accelerometer wear days (SD = 11.49, range = 2 to 42 days) containing at least one EMA self-report. After removing the EMA non-response occasions and the non-valid time windows with less than 15 min of valid accelerometry wear time (28% of all prompt-matched occasions), a total of 14,246 prompt-matched occasions with both valid EMA and accelerometry data were identified and included for analysis. The mean number of prompt-matched EMA-accelerometry occasions provided by each child was 73.05 (SD = 35.69; range = 5–149). Matched EMA-accelerometry occasions were distributed across children's ages as follows: 466 (age 8.31 to <9); 2,227 (age 9 to <10); 4,032 (age 10 to <11); 4,142 (age 11 to <12); 2,483 (age 12 to <13); and 896 (age 13 to 14.57). Table 1 summarizes the descriptive statistics of the time-variant and time-invariant covariates included in the current analyses. Overall, children reported generally low levels of negative affect and generally high levels of positive affect at any given EMA prompt. The mean levels of MVPA or sedentary time accumulated in the 15-min time window before vs. after the EMA prompt were similar. Nearly half of the EMA reports were provided on weekend days, and children reported being alone in approximately one-tenth of the EMA prompts.
Descriptive statistics for the covariates in the time-varying effect modeling (N = 195)
Variable . | Mean (%) . | Median . | SD . | Min . | Max . |
---|---|---|---|---|---|
Age by measurement wave (years) | 11.23 | 11.21 | 1.23 | 8.31 | 14.57 |
EMA response timea | 5.29 | 5 | 1.49 | 1 | 7 |
Momentary negative affect | 1.25 | 1 | 0.50 | 1 | 4 |
Momentary positive affect | 3.01 | 3 | 0.94 | 1 | 4 |
MVPA before prompt (minute) | 0.89 | 0.5 | 1.93 | 0 | 15 |
MVPA after the prompt (minute) | 0.87 | 0.5 | 1.86 | 0 | 15 |
Sedentary time before the prompt (minute) | 8.85 | 9 | 4.12 | 0 | 15 |
Sedentary time after the prompt (minute) | 8.93 | 9.5 | 4.06 | 0 | 15 |
Wave-based BMI (z-score) | 0.49 | 0.44 | 1.10 | −2.63 | 2.63 |
Boys (coded as 1) | (48.6) | – | – | 0 | 1 |
Weekend day (coded as 1) | (47.0) | – | – | 0 | 1 |
Alone (coded as 1) | (11.5) | – | – | 0 | 1 |
Variable . | Mean (%) . | Median . | SD . | Min . | Max . |
---|---|---|---|---|---|
Age by measurement wave (years) | 11.23 | 11.21 | 1.23 | 8.31 | 14.57 |
EMA response timea | 5.29 | 5 | 1.49 | 1 | 7 |
Momentary negative affect | 1.25 | 1 | 0.50 | 1 | 4 |
Momentary positive affect | 3.01 | 3 | 0.94 | 1 | 4 |
MVPA before prompt (minute) | 0.89 | 0.5 | 1.93 | 0 | 15 |
MVPA after the prompt (minute) | 0.87 | 0.5 | 1.86 | 0 | 15 |
Sedentary time before the prompt (minute) | 8.85 | 9 | 4.12 | 0 | 15 |
Sedentary time after the prompt (minute) | 8.93 | 9.5 | 4.06 | 0 | 15 |
Wave-based BMI (z-score) | 0.49 | 0.44 | 1.10 | −2.63 | 2.63 |
Boys (coded as 1) | (48.6) | – | – | 0 | 1 |
Weekend day (coded as 1) | (47.0) | – | – | 0 | 1 |
Alone (coded as 1) | (11.5) | – | – | 0 | 1 |
Note. aEMA response time was coded into 7 time windows between 7 am and 8 pm: (1) 7:00–8:00, (2) 9:00–10:00, (3) 11:00–12:00, (4) 13:00–14:00, (5) 15:00–16:00, (6) 17:00–18:00, (7) 19:00–20:00.
Descriptive statistics for the covariates in the time-varying effect modeling (N = 195)
Variable . | Mean (%) . | Median . | SD . | Min . | Max . |
---|---|---|---|---|---|
Age by measurement wave (years) | 11.23 | 11.21 | 1.23 | 8.31 | 14.57 |
EMA response timea | 5.29 | 5 | 1.49 | 1 | 7 |
Momentary negative affect | 1.25 | 1 | 0.50 | 1 | 4 |
Momentary positive affect | 3.01 | 3 | 0.94 | 1 | 4 |
MVPA before prompt (minute) | 0.89 | 0.5 | 1.93 | 0 | 15 |
MVPA after the prompt (minute) | 0.87 | 0.5 | 1.86 | 0 | 15 |
Sedentary time before the prompt (minute) | 8.85 | 9 | 4.12 | 0 | 15 |
Sedentary time after the prompt (minute) | 8.93 | 9.5 | 4.06 | 0 | 15 |
Wave-based BMI (z-score) | 0.49 | 0.44 | 1.10 | −2.63 | 2.63 |
Boys (coded as 1) | (48.6) | – | – | 0 | 1 |
Weekend day (coded as 1) | (47.0) | – | – | 0 | 1 |
Alone (coded as 1) | (11.5) | – | – | 0 | 1 |
Variable . | Mean (%) . | Median . | SD . | Min . | Max . |
---|---|---|---|---|---|
Age by measurement wave (years) | 11.23 | 11.21 | 1.23 | 8.31 | 14.57 |
EMA response timea | 5.29 | 5 | 1.49 | 1 | 7 |
Momentary negative affect | 1.25 | 1 | 0.50 | 1 | 4 |
Momentary positive affect | 3.01 | 3 | 0.94 | 1 | 4 |
MVPA before prompt (minute) | 0.89 | 0.5 | 1.93 | 0 | 15 |
MVPA after the prompt (minute) | 0.87 | 0.5 | 1.86 | 0 | 15 |
Sedentary time before the prompt (minute) | 8.85 | 9 | 4.12 | 0 | 15 |
Sedentary time after the prompt (minute) | 8.93 | 9.5 | 4.06 | 0 | 15 |
Wave-based BMI (z-score) | 0.49 | 0.44 | 1.10 | −2.63 | 2.63 |
Boys (coded as 1) | (48.6) | – | – | 0 | 1 |
Weekend day (coded as 1) | (47.0) | – | – | 0 | 1 |
Alone (coded as 1) | (11.5) | – | – | 0 | 1 |
Note. aEMA response time was coded into 7 time windows between 7 am and 8 pm: (1) 7:00–8:00, (2) 9:00–10:00, (3) 11:00–12:00, (4) 13:00–14:00, (5) 15:00–16:00, (6) 17:00–18:00, (7) 19:00–20:00.
Figure 1 presents the time-varying intercept functions, representing the mean levels of affect and activity time, separately, across ages 8.31 to 14.57, adjusting for average levels for all covariates. As shown in panels 1a and 1b, children's mean positive affect remained relatively high (above 3) until age 11.72, and then subsequently decreased until age 14.57; children's mean negative affect was relatively low and stable (around 1.25) across ages, except for a slight increase after age 13.68 (above 1.30). Panels 1c and 1d showed similar decreasing trends in mean MVPA during the 15-min time window, regardless of before or after an EMA prompt. Around age 8, children had an average of approximately 1.6 min (≈100 secs) of MVPA during any given prompt-matched 15-min time window. Around age 14, their time spent in MVPA decreased to approximately half a minute (≈ 30 secs) in a 15-min time window. Similarly, panels 1e and 1f show almost identical increasing trends in children's mean sedentary time during any given 15-min time window before or after an EMA prompt. Children initially spent about half of the time (≈7.5 mins) being sedentary in the 15-min window around age 8. Their sedentary time then gradually increased and eventually occupied over two-thirds of the 15-min window (≈11 mins) around age 14.
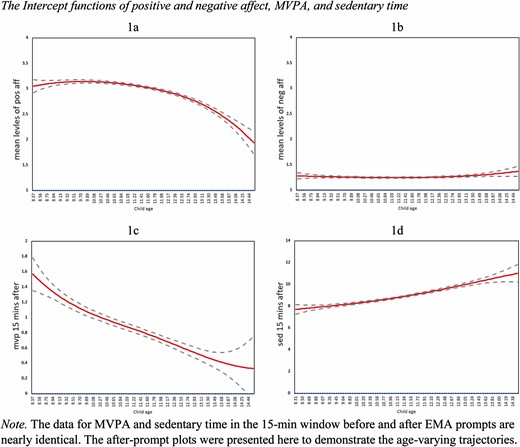
The intercept functions of positive and negative affect, MVPA, and sedentary time in the EMA prompt-matched window across ages.
Figure 2 presents the age-varying associations (slope) between children's activity levels before the EMA prompts and subsequent affective responses, adjusted for covariates. The solid line depicts the estimated regression coefficients over time, along with their corresponding 95% confidence intervals shown as the dashed lines. If at any point in time, the confidence interval (dashed lines) does not include zero (the horizontal dotted line), there is a statistically significant non-zero regression coefficient at that specific time. As shown in panels 2a and 2b, greater MVPA levels in the 15-min window before the prompt significantly predicted higher positive affect and lower negative affect after adjusting for covariates, but these associations were only significant for specific age ranges. The positive association between MVPA and higher subsequent positive affect was observed from ages 9.45 to 12.36 (p's < .05), whereas the negative association between MVPA and lower subsequent negative affect was observed from age 11.41 to age 13.18 (p's<.05). Panels 2c and 2d show that sedentary time during the 15-min window before the prompt predicted lower levels of both positive and negative affect, but these associations were only significant for specific age ranges. Between ages 10.96 and 13.05 (p's < .05), greater sedentary time in the 15 min before the prompt was associated with lower subsequent positive affect. However, greater sedentary time before the EMA prompt was associated with lower subsequent negative affect between age 9.07 and 10.52 (p's < .05).
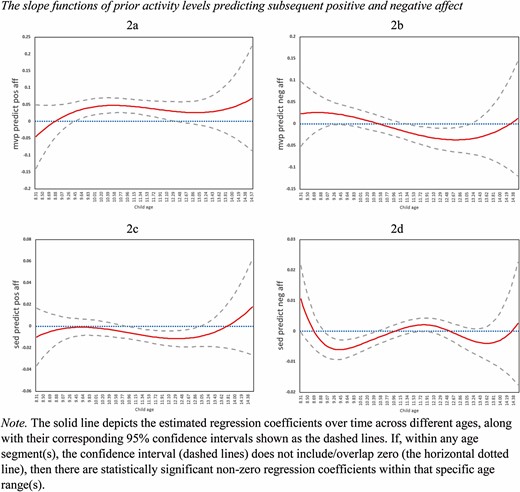
The slope functions of prior MVPA and sedentary levels predicting subsequent positive and negative affect across ages.
Figure 3 depicts the age-varying association between children's EMA-reported affective states and subsequent activity levels in the 15 min after the prompt, adjusted for covariates. Panels 3a and 3b show that greater positive affect predicted more MVPA and less sedentary time in the 15 min after the prompt, but these associations were only significant for specific age ranges. Between age 9.19 and 10.84 (p's < .05), higher positive affect was associated with less subsequent sedentary time in the 15 min after the prompt. Similarly, higher positive affect was associated with greater subsequent MVPA in the 15 min after the prompt between age 9 and 11.12 (p's < .05). Relatively small but significant associations between negative affect and activity levels are shown in both panels 3c and 3d. Higher negative affect was associated with less subsequent MVPA in the 15 min after the prompt from age 10.90 and 11.66 (p's < .05), and greater subsequent sedentary time between age 11.21 and 11.72 (p's < .05).
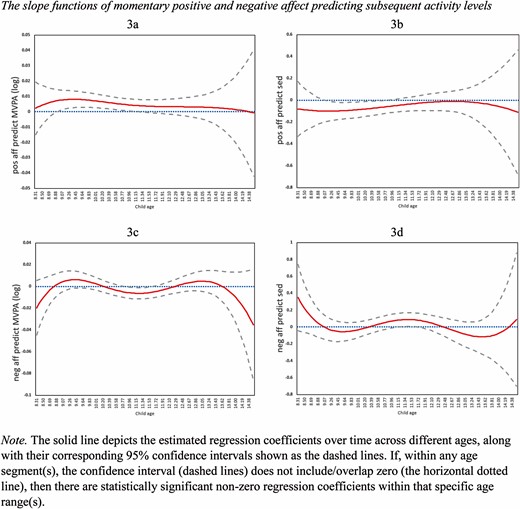
The slope functions of momentary positive and negative affect predicting subsequent MVPA and sedentary levels.
Figure 4 summarizes each of the eight observed age-varying associations between affect and activity levels in children. In general, bi-directional associations were observed for MVPA and among younger children (rows A and B). In contrast, associations were typically uni-directional for sedentary time and for older children (rows E–H). Collectively, all the observed associations presented in this figure supported our general hypotheses that the bi-directional associations between children's affect and movement behaviors vary with age during the transition from childhood to adolescence.
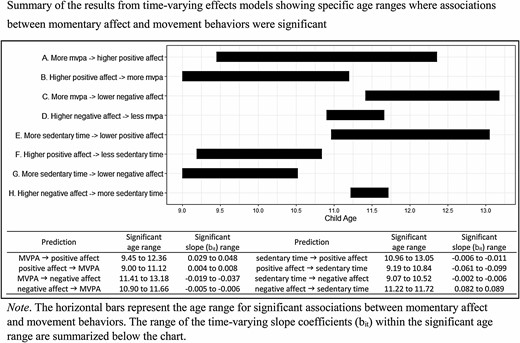
Summary of the age-varying bi-directional associations between momentary affect and movement behaviors.
Discussion
This study investigated the potential age-varying bi-directional associations between affective states and activity levels from late childhood to early adolescence as they occurred naturally in the free-living environment. Results from the TVEMs supported our general hypotheses that the strength of the acute bi-directional associations between affect and movement behaviors are not static and are likely to change across this developmental period. These preliminary findings have the potential to pinpoint critical developmental windows whereby activity levels and affective states are more tightly (and loosely) linked in youth. Accumulating work of this kind can aid practitioners in identifying periods of vulnerability or opportunity for delivering tailored interventions that best meet the needs of children undergoing specific developmental transitions.
Our findings of generally decreasing trends of mean activity levels across childhood to mid-adolescence are consistent with the developmental literature [43, 44]. Since children's MVPA and sedentary time in the current study were assessed within 15-min windows, our results provide novel evidence that the decreases in activity levels typically found at a macro timescale can also be observed at a micro timescale. Given that children in the current study were generally in good psychological health (children with mood disorders or mental illnesses were excluded), it may explain the low mean levels of negative affect observed across all ages. However, the apparent decreasing trend in mean levels of positive affect and a slightly increasing trend in mean negative affect also supports findings from population-based studies that report decreased psychological well-being as youth age across development [45, 46].
The age-varying associations between children's MVPA and subsequent affective responses suggest that more MVPA predicted higher positive affect during younger ages and lower negative affect during older ages. These results may be in part due to engagement in more structured and organized types of sports and physical activities as children age. MVPA may be more leisurely and intrinsically motivated in younger children, which may bring about more positive affect [47, 48]. In contrast, older children need to spend more time studying and therefore have less time to play [49]. Further, MVPA may be more task-oriented, mandated, and competitive for older children, such as after-school sports programs and activity classes (e.g., dance, karate) [50]. The findings that MVPA is associated with subsequently lower levels of negative affect in older but not younger children suggest that physical activity may confer an added benefit towards mental health among older youth; one reason for this may be that negative affect tends to be more variable during early-to mid-adolescence due to the development of emotion regulation skills and thus may be more malleable during this period [51]. As negative affect experiences are more frequent while transitioning into adolescence, the beneficial role of MVPA on mental health may be more salient as physical activity help divert adolescents' attention from stressors [52]. This is supported by studies demonstrating that children and adolescents who participated in exercise training or physical activity interventions reported significantly lower levels of stress and depression compared to peers in the control groups [53–55].
The age-varying associations between children's prior sedentary time and affect suggest that more prior sedentary time predicted lower negative affect during younger ages and lower positive affect during older ages. These results may reflect the different types and purposes of sedentary behaviors that children engage in during different developmental periods. One epidemiological study among youth during a similar developmental period showed that children's screen-based sedentary behaviors steadily increase from 9 to 14 years old [56]. These increases in screen-based sedentary time usually consist of more academic-oriented behaviors as children get older [57, 58], which may lead to the decreases in children's positive affect in response to their sedentary behaviors [59]. Similarly, as older children may spend more of their sedentary time engaged in social media, concerns have been raised that this may especially impair mental health in adolescents [60, 61]. On the other hand, younger children may engage in less deleterious forms of sedentary behaviors (arts and crafts, board games, or playing casual video games), which may, on the contrary, benefit emotional outcomes [62, 63].
Children's momentary positive affect was associated with greater subsequent MVPA and less subsequent sedentary time at younger ages (around 9 to 11 years). When children are younger, they may be more likely to express their positive emotions with bodily movements; for example, they gesticulate for daily events that bring about excitement and joy [64]. This result may also be partially explained by the stronger link between intrinsic motivation and physical activity for children at younger ages than older ages [65]. Children generally engage in physical activity for fun, and they may therefore look forward to it and feel excited about it, whereas adolescents' motivation for physical activity may be less intrinsic and more relevant to external rewards and social purposes [66]. Thus, the role of positive affect on subsequent physical activity may be relatively weaker among older children as compared to younger children. In contrast, the association between prior momentary negative affect and subsequent activity levels was only evident during a narrow developmental window; more sedentary time and less MVPA were predicted by higher momentary negative affect only around 11 years old. Children around 11 years old usually undergo the transition from elementary school to middle school. This is also a critical developmental period in which children's biological/metabolic changes during puberty may play a role in their activity levels [67, 68]. Further, negative affect experiences during this period may be due to adjustment to a new environment and enhanced academic competition, which, in turn, may result in greater inactivity [69, 70]. However, due to the narrow significant region observed in the TVEM plots (panels 3c and 3d), the age-varying association between momentary negative affect and children's subsequent activity levels should be interpreted with caution.
Overall, our results suggested that the bi-directional associations between movement behaviors and affective states differentially occurred across ages in children. Consistent with a previous EMA systematic review synthesizing the bi-directional affect-activity associations in different populations including children [21], we also found relatively more evidence that prior physical activity and sedentary levels predicted subsequent affect across ages. We further found that when children are younger, being more active and less sedentary was associated with more positive affect and less negative affect. However, during adolescence, more physical activity and less sedentary time seemed to be protective against negative affect and being more sedentary reduced positive affect. These findings suggest that being physically active may connect closely to better psychological well-being among younger youth, whereas physical inactivity may be more strongly linked to negative aspects of mental health among adolescents compared to children.
Interventions that focus on cultivating positive affect, intrinsic motivation, or enjoyment of physical activity should be implemented earlier on to promote active lifestyle and emotional well-being in childhood. For example, after-school programs that combine audio- and video-based exergaming (e.g., Wii Sports) can enhance enjoyment and physical activity simultaneously among younger youth [71]. On the other hand, intervention approaches that aim to enhance physical activity and reduce general sedentary time or screen-based sedentary behavior may confer mental health benefits among youth. For instance, mindfulness-based practices tailored for children and youth are feasible to be implemented in the school setting to help students manage negative affective experiences (stress, anxiety, depression) [72, 73]. Other school-based interventions, such as incorporating standing desks in the classroom setting, may be effective for reducing sedentary time [74]. In addition, family-based interventions that involve parents have also demonstrated efficacy for reducing adolescents' sedentary time and engagement in screen-based sedentary behaviors [75]. Future studies should investigate if these promising mindfulness and sedentary behavior interventions also lead to improved physical activity and mental health outcomes among youth.
Our summary TVEM plots (Fig. 4) demonstrate that affect and activity levels are bi-directionally related for MVPA and younger children, whereas associations for sedentary time and older children were primarily uni-directional; this pattern of findings may help explain inconsistencies in the EMA literature on acute associations affect and activity levels [20]. Similarly, traditional modeling methods (e.g., multilevel modeling, generalized estimating equations), may not have captured the same nuances in the timing of associations that we found, highlighting the value of implementing TVEM when it is plausible that the strength of associations may vary over time (e.g., chronological age).
Strengths of the current study include the real-time data collection strategy, objective activity measures, longitudinal design, and use of a cutting-edge statistical strategy. However, there are also limitations that are worth noting. First, this study applied a multi-wave EMA design to cohorts of children with different baseline ages, which makes it difficult to rule out potential cohort effects on our findings [76]. Future research should collect complete longitudinal EMA data by tracking the same age of children across years to better address the possible confounding between age and cohort effects. Second, children's positive and negative affect were assessed with a small set of items in the current study, which were not capable of capturing a broad spectrum of affective experiences. Other affective states (e.g., excitement, fear, contentment, guilt) should be included in future studies to investigate whether the age-varying associations exist based on different affective components [77, 78]. Wearable devices and sensors that passively collect individuals' physiological signals for detecting different psychological states can also be combined with EMA self-reports in future studies to strengthen the affect measures [79]. Third, despite that we used timestamps to determine the sequential order of affective experiences and activity behaviors, causal inferences cannot be made from our findings given the observational nature of the study design. There may a third variable that accounts for the observed age-varying affect-activity association. For example, children's physical activity and sedentary behaviors may be more volitional (have more autonomy to choose what they want to do) as they age. Other time-varying factors that may impact children's affective experiences associated with their physical activity and sedentary behavior at any given time should be included in future longitudinal EMA studies and analysis. Fourth, the study sample only included children living in Southern California; applying TVEM on population-level data are needed to support our findings and provide stronger developmental implications. Lastly, potential mechanisms underlying the observed age-varying associations between children's daily affect and activity levels were not examined in this study. For example, as children approach puberty, the observed associations may be due to the biological, hormonal, or other social-psychological changes that link to emotional regulation and processing during this developmental stage [80, 81]. Regardless of the stated limitations, our results suggest that developmental age should be considered as a meaningful dimension in theories of behavior change for physical activity and sedentary behaviors in youth.
Conclusion
This study utilizes a novel analytic approach on multiple waves of EMA data collected from children during their transition from late childhood to early adolescence. Preliminary results indicate that the strength of the bi-directional associations between momentary affect and activity levels may vary across ages during this developmental stage. These findings suggest that age- or developmental stage-tailored interventions may be needed to promote active lifestyles and well-being among children and adolescents. Overall, this observational study contributes to a larger body of evidence aiming to inform preventive strategies targeting age-related declines in physical activity and mental health and increases in sedentary time.
Acknowledgments
The MATCH study was supported by the National Heart Lung and Blood Institute R01 grant (R01HL119255). Jennifer Zink was supported by the National Cancer Institute (T32CA009492). The authors thank Daniel Chu for processing the EMA and accelerometry data. This work was supported by the American Cancer Society (118283-MRSGT-10-012-01CPPB). The funders had no role in study design, data collection, and analysis, decision to publish, or preparation of the manuscript.
Compliance with Ethical Standards
Authors’ Statement of Conflict of Interest and Adherence to Ethical Standards All authors listed on this manuscript declare that they have no conflicts of interest.
Ethical Approval All procedures performed in studies involving human participants were in accordance with the ethical standards of the institutional and/or national research committee and with the 1964 Helsinki declaration and its later amendments or comparable ethical standards. Informed consent was obtained from all individual participants included in the study.
References